The Genetic Architecture of Obsessive-Compulsive Disorder: Contribution of Liability to OCD From Alleles Across the Frequency Spectrum
Abstract
Objective:
Obsessive-compulsive disorder (OCD) is known to be substantially heritable; however, the contribution of genetic variation across the allele frequency spectrum to this heritability remains uncertain. The authors used two new homogeneous cohorts to estimate the heritability of OCD from inherited genetic variation and contrasted the results with those of previous studies.
Methods:
The sample consisted of 2,090 Swedish-born individuals diagnosed with OCD and 4,567 control subjects, all genotyped for common genetic variants, specifically >400,000 single-nucleotide polymorphisms (SNPs) with minor allele frequency (MAF) ≥0.01. Using genotypes of these SNPs to estimate distant familial relationships among individuals, the authors estimated the heritability of OCD, both overall and partitioned according to MAF bins.
Results:
Narrow-sense heritability of OCD was estimated at 29% (SE=4%). The estimate was robust, varying only modestly under different models. Contrary to an earlier study, however, SNPs with MAF between 0.01 and 0.05 accounted for 10% of heritability, and estimated heritability per MAF bin roughly followed expectations based on a simple model for SNP-based heritability.
Conclusions:
These results indicate that common inherited risk variation (MAF ≥0.01) accounts for most of the heritable variation in OCD. SNPs with low MAF contribute meaningfully to the heritability of OCD, and the results are consistent with expectation under the “infinitesimal model” (also referred to as the “polygenic model”), where risk is influenced by a large number of loci across the genome and across MAF bins.
Obsessive-compulsive disorder (OCD) is a serious and often long-lasting psychiatric disorder characterized by intrusive and unwanted thoughts, images, or urges (obsessions) that are typically linked to ritualized acts (compulsions) (1–4). OCD affects 1%–3% of the population, and multiple studies provide reliable evidence for a significant genetic contribution to risk (1, 3–6) as well as a role for environmental factors in risk (7, 8). The heritability of OCD, historically estimated by analysis of twin and family studies and within the context of the ACE model (A, additive genetic, also known as narrow-sense heritability; C, shared environment; and E, nonshared environment), is reported to be in the range of 35%–50% (1, 4, 8–14).
As an alternative to the analysis of recurrence risk for OCD within pedigrees, heritability can also be estimated from individuals drawn from a population who have no obvious familial relationships, as long as they have been characterized for genetic variation across their genomes. Usually, this genetic characterization employs genotypes of single-nucleotide polymorphisms (SNPs) for which alleles are common in the population. In this approach, which we will call SNP based, the central idea is that the multiplicity of SNP genotypes allows estimation of familial relationships, albeit distant ones, among subjects as well as the covariance of their phenotypes, and these are the key elements for estimating heritability. When the heritability of OCD is computed in this manner, estimates range from 25% to 43% (5, 14–16).
It is useful to compare the heritability results from family-based and SNP-based approaches. Family-based studies, being more direct, typically yield estimates of heritability with lower standard errors, whereas the inaccuracy of estimating distant relationships from genetic data tends to produce fuzzier estimates. Family-based estimates also tend to yield higher estimates of heritability because the familial covariance reflects both rare and common genetic variation, whereas SNP-based estimates mostly arise from covariance due to common genetic variants. Looking at the results summarized above, one might conclude that this is also operating for OCD, that is, that family-based studies are producing higher heritability estimates than SNP-based studies.
However, in an influential study of data from the International Obsessive-Compulsive Disorder Foundation Genetics Collaborative (IOCDF-GC) by Davis et al. (5) (1,061 case subjects, 4,236 control subjects, 373,846 SNPs), there was no evidence for heritability from SNPs with minor allele frequency (MAF) <0.05, and over 60% of total heritability mapped to the most common variants (MAF >0.3). In addition, in a meta-analysis of data from the International Obsessive Compulsive Disorder Foundation Genetics Collaborative (IOCDF-GC) and OCD Collaborative Genetics Association Study (OCGAS) (16), ∼60% of heritability was accounted for by SNPs with MAF >0.4 in both the OCGAS sample alone and in the combined sample. If this observation were true, it could have profound implications for which evolutionary forces shaped this unusual mapping of risk alleles to their population frequency distribution. For example, balancing selection, where multiple alleles are maintained in the gene pool of a population at frequencies larger than expected from genetic drift alone, may play a role in OCD.
At the same time, other studies have implicated rare variants in risk for OCD (17–20). Thus, the contribution of inherited genetic variation across the allelic frequency spectrum to the risk of OCD remains uncertain and worthy of further study, as it affects both our understanding of processes underlying OCD risk architecture and rational study design. Here, using a substantially larger sample compared with previous studies and new genetic data from the Swedish population, we estimate SNP-based heritability for OCD.
Methods
Study Population
Ethical approval was obtained from the Institutional Review Board at the Icahn School of Medicine at Mount Sinai, New York, and the Regional Ethical Review Board in Stockholm. We used Swedish OCD cases collected through two studies: the EGOS cohort (Epidemiology and Genetics of Obsessive-Compulsive Disorder and Chronic Tic Disorders in Sweden) (21) and the NORDiC cohort (Nordic OCD and Related Disorders Consortium) (22).
In the EGOS cohort, individuals who were born between 1954 and 1998 and had at least two clinical diagnoses of OCD in the Swedish National Patient Register were eligible for inclusion (21). At the Swedish site of the NORDiC cohort, individuals with OCD were recruited from specialty OCD and related disorder clinics across Sweden (22). Genotype data on the Global Screening Array (Illumina) were collected for 1,108 individuals from the EGOS cohort and 1,107 individuals from the NORDiC cohort (Table 1).
EGOS (N=1,108) | NORDiC (N=1,107) | |||
---|---|---|---|---|
Characteristic | N | % | N | % |
Female | 692 | 63 | 651 | 59 |
Comorbidities | ||||
Chronic tic disorders (ICD-10 code F95) | 100 | 9 | 43 | 5 |
ADHD (ICD-10 code F90) | 40 | 4 | 83 | 10 |
Bipolar disorder (ICD-10 code F31) | 33 | 3 | 93 | 11 |
Phobic anxiety disorders (ICD-10 code F40) | 19 | 2 | 98 | 12 |
Other anxiety disorders (ICD-10 code F41) | 112 | 10 | 106 | 13 |
Autistic disorder (ICD-10 code F84.0) | 3 | <1 | 8 | 1 |
Asperger’s syndrome (ICD-10 code F84.5) | 40 | 4 | 51 | 6 |
Intellectual disability (ICD-10 codes F71–F73) | 7 | <1 | 2 | <1 |
At least one psychiatric comorbidity | 417 | 37 | 424 | 53 |
TABLE 1. Characteristics of the OCD cohorts before quality controla
A sample of 4,738 control subjects from the LifeGene cohort was available for this study. LifeGene is a prospective population-based cohort of around 50,000 individuals in Sweden (23). The samples were available in four batches: LifeGene-EGOS (N=1,444), LifeGene-NORDiC (N=500), LifeGene-ANGI-Wave-1 (N=1,500), and LifeGene-ANGI-Wave-2 (N=1,500) (ANGI is the Anorexia Nervosa Genetics Initiative). LifeGene-ANGI control subjects were previously used in a study of anorexia nervosa (24); they were mostly females (2,935 females and 65 males), and all individuals with a diagnosis of anorexia nervosa were previously removed from this batch. All control subjects were genotyped using the Global Screening Array.
Quality Control
All OCD case subjects, LifeGene-EGOS control subjects, and LifeGene-NORDiC control subjects were genotyped in the same laboratory but in different batches. GenomeStudio’s genotyping module was used to recall genotypes on the joint data.
Quality control was first carried out on three batches of samples that may differ in key variables: 1) all case subjects, LifeGene-EGOS control subjects, and LifeGene-NORDiC control subjects, 2) LifeGene-ANGI-Wave-1 control subjects, and 3) LifeGene-ANGI-Wave-2 control subjects. We employed the following quality control steps using PLINK, version 2.0 (see Tables S1–S3 in the online supplement): individuals were removed who had a genotype non-call rate >0.05, were discrepant for nominal versus genetically determined sex, or had either low or high heterozygosity (>±3 SD from the mean); a SNP was removed if its non-call rate for genotypes was >0.05, if its MAF was <0.01, or if it had a Hardy-Weinberg equilibrium p value <0.00125. GEMTools was used to choose individuals with European ancestry where indicated (see Figure S1 in the online supplement).
We next used the tool developed by McCarthy (https://www.well.ox.ac.uk/∼wrayner/tools/) to match the SNPs to 1000 Genomes data, and Genotype Harmonizer (automatic strand alignment software) to align the different cohorts (25). After quality control, we merged the cohorts based on the set of all intersecting SNPs and performed additional quality control procedures as noted in the online supplement. The final data set included 2,090 case subjects and 4,567 control subjects, with 412,813 SNPs (Table 2).
After Quality Control | |||||
---|---|---|---|---|---|
Before Quality Control | Females (%) | ||||
Study | Individuals (N) | SNPs (N) | Individuals (N) | N | % |
EGOS, case subjects | 1,108 | 759,993 | 1,066 | 667 | 63 |
NORDiC, case subjects | 1,107 | 759,993 | 1,024 | 596 | 58 |
LifeGene-EGOS, control subjects | 1,444 | 759,993 | 1,238 | 452 | 36 |
LifeGene-ANGI-Wave-1, control subjects | 1,500 | 688,032 | 1,432 | 1,378 | 96 |
LifeGene-ANGI-Wave-2, control subjects | 1,500 | 688,032 | 1,442 | 1,432 | 99 |
LifeGene-NORDiC, control subjects | 500 | 759,993 | 455 | 228 | 50 |
Total (merged)a | 7,059 | 6,657 | 4,753 | 71 | |
Case subjects | 2,115 | 2,090 | 1,263 | 60 | |
Control subjects | 4,944 | 4,567 | 3,490 | 76 |
TABLE 2. Summary of data before and after quality control
Statistical Analysis
We used the Genome-Wide Complex Trait Analysis (GCTA) program, version 1.26.0, to estimate the genetic relationship matrix between all pairs of individuals from SNPs (26). Next, we used PLINK, version 2.0, to extract the top principal components from the variance-standardized relationship matrix (for more details, see the online supplement). We performed restricted maximum likelihood analysis, implemented in GCTA, to estimate the heritability of OCD attributable to SNP genotypes. Because the OCD diagnosis is dichotomous, we scaled the phenotypic variance to an underlying liability scale using the population prevalence of 1%, similar to our most recent estimate of population prevalence in Sweden using data from the Swedish national registers (1) (for more details, see the online supplement, where we also provide results for 2% prevalence).
To evaluate the sensitivity of estimates of SNP-based heritability to modeling approaches, we assessed the data in multiple ways. The first assessment included all affected and unaffected individuals born in Sweden, of whom most but not all were of Swedish/European genetic ancestry, and used all 405,105 high-quality genotyped SNPs for analysis. The sampling in the first assessment is consistent with our previously published family-based analyses and constitutes our primary analytical approach here. The second assessment pruned SNPs according to linkage disequilibrium to obtain a smaller set of 184,296 largely independent SNPs. The third assessment limited the sample to individuals of European genetic ancestry. The fourth assessment removed all individuals for whom there was also a fifth-degree or greater relative in the sample. The fifth assessment analyzed only pairs of affected and unaffected individuals, matched on two dimensions of genetic ancestry using the function pairmatch in the package optmatch in R (“1-to-1 fullmatch”) (see the online supplement). The sixth assessment was conducted as was done in assessment 5, using only individuals of European ancestry. Pair matching, as done in assessments 5 and 6, is a common epidemiological approach for controlling confounding (here, differences in ancestry in case subjects versus control subjects) and has been shown to be useful for genetic studies (27–29). Note that assessments 3–6 use all high-quality SNPs.
We also estimated heritability partitioned by chromosomes and MAF bins and compared the results with those from the study of the IOCDF-GC sample by Davis et al. (5). We created six MAF bins: 0.01–0.05, 0.05–0.1, 0.1–0.2, 0.2–0.3, 0.3–0.4, and 0.4–0.5. For each bin, we computed a genetic relationship matrix, and then the additive genetic variance attributed to each subset was jointly estimated with multiple genetic relationship matrices (using −mgrm in GCTA). This allows for the effects of linkage disequilibrium to be partitioned by the restricted maximum likelihood analysis.
Results
Our study population included 2,090 case subjects and 4,567 control subjects after quality control was completed. Sixty percent of the case subjects were female, and 76% of the control subjects were female (Table 1). Based on principal component analysis (see Figure S2 in the online supplement), we used the first six principal components as covariates to adjust for variation in ancestry in all heritability analyses. As a check for compatibility of cohorts, we first estimated heritability by treating EGOS and NORDiC control subjects as cases and LifeGene-ANGI control subjects as controls. Heritability was estimated at 0.0001% (SE=5%). These results show that the control cohorts were homogeneous. Next, we estimated heritability of OCD for the full sample, contrasting OCD cases to controls and yielding an estimate of 29% (SE=4%), for a population prevalence of 1%.
Technically, heritability is first estimated on the observed scale, namely, dichotomous OCD diagnosis; however, heritability on the continuous liability scale is more interpretable and so is usually reported. Heritability can be transformed from the observed to the continuous liability scales because they are functions of prevalence (30). To determine how sensitive our heritability estimate was to prevalence, we varied it between 0.5% and 3% and found heritability to vary between 25% and 38% (see Table S4 in the online supplement).
We next performed a set of sensitivity analyses by different treatments of the data, as described in the Methods section, and found the estimates to be quite robust (Table 3). Notably, although analyses suggested that EGOS and NORDiC case subjects had slightly different ancestry distributions, the results in Table 3 show that our adjustments for ancestry were sufficient to compensate for these differences (see Figures S4–S9 and Table S5 in the online supplement). In addition, we did not observe a significant difference in heritability between the EGOS and NORDiC case subjects (see Table S5).
Heritability | ||||
---|---|---|---|---|
Data | Case Subjects (N) | Control Subjects (N) | % | SE |
All case and control subjects | 2,090 | 1,263 | 29 | 4 |
All case and control subjects (based on 184,296 SNPs after pruning according to linkage disequilibrium) | 2,090 | 1,263 | 28 | 4 |
Individuals with European ancestry | 1,831 | 4,065 | 28 | 5 |
All third cousins or closer relatives removed | 1,822 | 3,954 | 30 | 5 |
Ancestry matched (1-to-1 fullmatch) | 2,090 | 2,090 | 26 | 6 |
Ancestry and sex matched (1-to-1 fullmatch) | 2,090 | 2,090 | 29 | 6 |
Ancestry matched (1-to-1 fullmatch), European ancestry | 1,831 | 1,831 | 23 | 7 |
TABLE 3. Estimates of heritability of OCD under various treatments of the data
Heritability Analysis Partitioned by MAF Bin
Having established that a substantial portion of OCD traces to common variation, we next addressed an important issue about its nature. In the study of the IOCDF-GC sample (5), it was reported that alleles with MAF <0.05 did not contribute meaningfully to the heritability of OCD (0.0001% of total heritability). To compare our results with those of Davis et al. (5), we estimated the portion of total heritability for groups of autosomal SNPs with distinct allele frequencies, grouping the SNPs into six bins based on their MAF (Figure 1; see also Table S9 in the online supplement): 0.01–0.05, 0.05–0.1, 0.1–0.2, 0.2–0.3, 0.3–0.4, and 0.4–0.5. For all the bins, we included the first six principal components as covariates and set population prevalence to 0.01. Estimates of the portion of total heritability for the bins were distributed differently between these two studies (Figure 1; see also Table S9). Curiously, although the total heritability of the first two bins (MAF <0.1) was similar across studies—that is, 2.6% in our study, compared with 4% in the study of the IOCDF-GC sample (5)—estimates for specific bins were not similar. In the Davis et al. study (5), the 0.01–0.05 MAF bin accounted for essentially no heritability (0.0001%), whereas our estimate was much larger (2.6%) (Figure 1; see also Table S9). A portion of the difference could be due to the number and nature of the SNPs falling in this bin: approximately 10 times more genotyped SNPs fell into this bin in the present study compared with the study of the IOCDF-GC sample (5) (see Table S9); however, Davis et al. also imputed genotypes for over 2 million SNPs for this bin, and those genotypes did not alter their heritability estimate in that bin (see their Table 2, in reference 5).
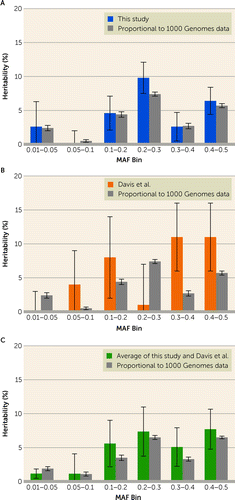
FIGURE 1. Estimates of OCD heritability partitioned by MAF bins from the results in this study and the study of the IOCDF-GC sample, and weighted averagesa
aWeighted averages (weights proportional to the inverse of variance) are of this study and the study of the IOCDF-GC sample (5). Each panel also shows the estimate of heritability for each bin from 1000 Genomes data, presented as the mean of heritability for that bin for 10 samples of 180,000 SNPs, where sampling from each bin was proportional to the percentage of SNPs in that bin from 1000 Genomes data. Note that the standard error for this latter analysis is the standard error of the sample mean for the 10 samples and is not directly comparable to the SNP-based standard error. Correlations with 1000 Genomes data were 0.99 (p<0.001) for panel A, 0.04 (p=0.94) for panel B, and 0.94 (p=0.005) for panel C.
To investigate these differences, we estimated what the expected portion of total heritability in these bins should be. First, we observed that the percentages of the total SNPs in each bin were distributed differently in comparison to 1000 Genomes data (for SNPs with MAF >0.01) (see Tables S6 and S7 in the online supplement), which we would expect is more representative of variation in the general population. For example, 45.2% of the SNPs in our study had MAF between 0.01 and 0.05, compared with 29.5% of SNPs in 1000 Genomes data. Under the standard quantitative genetic “infinitesimal model” (also referred to as the “polygenic model”) (31), it is reasonable to assume that the effect of all risk SNPs is equal. With this assumption, we then explored various models to predict the expected heritability in each MAF bin (Figure 1; see also Tables S6–S8 and Figures S10 and S11 in the online supplement).
The model that best fit the data was one in which risk alleles were sampled in proportion to their occurrence in 1000 Genomes data, with a goodness-of-fit-adjusted R2 of 0.49. Notably, and in contrast to what was observed in the study of the IOCDF-GC sample (5), the largest proportion of expected heritability was not explained by SNPs in the higher-frequency allele bins (0.3–0.4 and 0.4–0.5). In addition, we observed that SNPs with low MAF (0.01–0.05) are expected to account for 10.4% of the heritability under this model, similar to the 10% that we observed and in contrast to the IOCDF-GC sample, where low-MAF SNPs accounted for almost no heritability. These discrepancies and the smaller ones observed in our study track with sample size. For example, the size of the IOCDF-GC sample (1,061 case subjects and 4,236 control subjects) was smaller than in our study, and variance of estimates is a direct function of sample size. Combining results from both studies demonstrated strong concordance with expectation (Figure 1C). In addition, previous studies (5, 16) are likely more ancestrally heterogeneous than our Swedish sample, which can lead to increased variance.
Heritability Analysis Partitioned by Chromosomes
Under the infinitesimal model, SNPs affecting heritability of OCD (or any trait) should be scattered randomly across chromosomes, so that heritability per chromosome should track with chromosome length. This is observed in our study (Figure 2), and there is a significant correlation between heritability per chromosome and length (r=0.55, p=0.008). Chromosome 13 had the lowest heritability, significantly lower than what would be expected under the uniform distribution model.
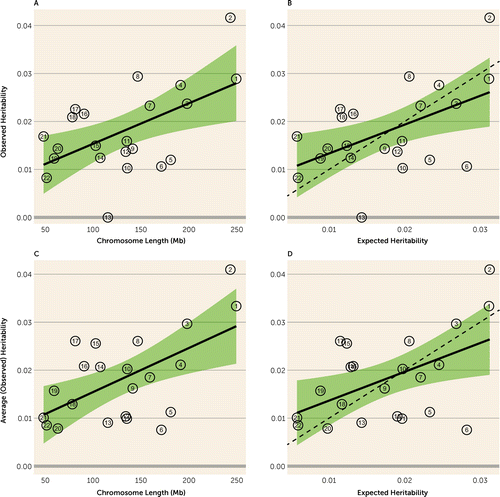
FIGURE 2. Estimates of OCD heritability partitioned by chromosomea
aPanel A presents the observed heritability by chromosome length and the 95% confidence interval (shaded area) for the regressed line (adjusted R2=0.27, p=0.008). Panel B presents the observed heritability by expected heritability and the 95% confidence interval for the regressed line (adjusted R2=0.23, p=0.014). Panel C presents the weighted average observed heritability by chromosome length and the 95% confidence interval for the regressed line (average over this study and the study of the IOCDF-GC sample [5]) (adjusted R2=0.31, p=0.004); the results for chromosome 21 and 22 are overlapping. Panel D presents the weighted average heritability by expected heritability and the 95% confidence interval for the regressed line (adjusted R2=0.22, p=0.0161). The dashed lines in panels B and D have slope of 1 and an intercept of zero (observed=expected).
As noted above, the noisy nature of these results can likely be attributed to the relatively small sample size for this type of analysis. We conjectured that if this were the case, and assuming that both study samples were homogeneous, combining the heritability estimates from the IOCDF-GC sample (5) and our heritability estimates, per chromosome, would produce a somewhat better fit between heritability per chromosome and length. This result is confirmed in Figure 2C,D; the fit of the regression for this weighted average heritability (weights proportional to the inverse of variance), an adjusted R2 of 0.31, is better than the fit for our sample alone, with an adjusted R2 of 0.27. Furthermore, note that chromosome 6, which had very low heritability in the Davis et al. study, shows reasonable heritability in both our analyses and in the combined data, again suggesting that small sample sizes are driving some of the results.
Discussion
Common genetic variation—variants shared among many individuals in a population, which are most frequently SNPs—has been found to play a role in liability for most psychiatric disorders, including OCD. Open questions remain about the impact on risk due to common variation, including how much of the heritability of OCD common variation accounts for and how it is partitioned across the frequency spectrum of alleles. These are important questions for a variety of reasons. For example, both schizophrenia and autism spectrum disorder demonstrate high heritability (32, 33), and much of the heritability traces to common genetic variation. Yet, rare variation with a damaging impact on gene function, especially de novo variation, plays a larger role in overall autism spectrum disorder risk than in overall schizophrenia risk (32, 34, 35); for example, Singh et al. (35) found, when they evaluated evolutionarily constrained genes, that de novo protein-truncating variants were four times more common among individuals with autism than among individuals with schizophrenia. This difference is critical for clinical genetics, genetic counseling, and possibly treatment. It also could be relevant for disentangling evolutionary processes underlying different psychiatric disorders, consistent with stronger natural selection on autism than schizophrenia. Finally, it would have an impact on study design (if, for example, rare variants contribute little to OCD heritability).
Here, we evaluate whether a substantial portion of the heritability of OCD traces to common variation, as it does for autism and schizophrenia, and we characterize its frequency spectrum, which is directly relevant to evolutionary processes. For example, in an early study estimating the heritability of OCD from common variation, results from the IOCDF-GC sample (5) suggested that alleles with the highest frequencies—that is, those with MAF >0.3—account for the bulk of SNP-based heritability of OCD. Similar findings were reported using meta-analysis of data from IOCDF-GC/OCGAS (16). Such a strong pattern would suggest that OCD was under strong balancing selection.
By sampling individuals with OCD from the Swedish population, as well as a larger sample of unaffected (control) individuals, we were able to address these questions. Our analyses of over 2,000 individuals diagnosed with OCD and twice as many unaffected individuals, each genotyped across their genome via >400,000 SNPs, yielded an OCD heritability estimate of 29% (SE=4%), a robust estimate (Table 3).
Moreover, when we assumed that SNPs contributed equally to risk for OCD, regardless of MAF, we obtained good fit between estimated OCD heritabilities from MAF bins of our sample and what was expected based on the distribution of MAF in 1000 Genomes data (Figure 1). SNPs affecting risk appear to be distributed at random over chromosomes, because size was a good predictor of a chromosome’s contribution to total heritability (Figure 2). Chromosome 13 showed the poorest fit to this model, which may be partially explained by its having one of the lowest gene densities (6.5 genes per Mb) among human chromosomes. All of these results fit expectations of the infinitesimal quantitative genetics model.
In terms of estimated heritability from common variation, our results compare favorably with previous studies of OCD. Published estimates of SNP-based heritability, based on different samples from different populations, range from 25% to 43% (5, 15, 16). Thus, all studies have converged on a substantial contribution of common variation to the heritability of OCD, showing notable consistency. There are some differences, however. Notably, recent studies (5, 16) suggest that SNPs with substantial frequency (MAF >0.05) contribute disproportionately to this heritability, and the contribution tends to increase with increasing MAF.
In light of our findings, we found their results intriguing: An increasing heritability associated with MAF is appealing because the contribution to heritability of any SNP of frequency p is 2p(1−p)a2, where the SNP’s effect a can be assumed to be roughly equal over all SNPs under the infinitesimal model; on the other hand, it seems unlikely that low-MAF SNPs have no contribution to heritability because there are so many of them in the human genome (see Table S9 in the online supplement). Our results argue that these low-MAF SNPs do contribute to OCD heritability, that their contribution is roughly in proportion to the frequency spectrum of alleles, and that they can be assumed to be of similar effect (i.e., a) across the frequency spectrum. Thus, our results show that future studies of less common and even rare alleles will also be informative on OCD etiology, with the caveat that effects of risk alleles of very low frequency can be difficult to detect by case-control methods.
Another interesting contrast is the evidence for heritability across chromosomes. Davis et al. (5) observed essentially no heritability for OCD on chromosome 6, which encodes both the HLA and histone gene clusters, and extremely high heritability on chromosome 15. In discussing these results, the authors suggest that chromosome 15 has an outsized contribution to OCD risk and that the HLA locus is effectively excluded from OCD risk. Given the contrasting results in our study, and in our analyses combining results from both studies, we again conclude that the data are consistent with the infinitesimal model and that smaller sample sizes may account for results that diverge from expectation.
The Davis et al. study (5) involved about 50% fewer OCD cases, and the variance in any estimate is a direct function of sample size. It is also possible that the IOCDF-GC sample had a different distribution of distantly related individuals than our relatively homogeneous sample from Sweden. The accuracy of SNP-based heritability diminishes as the fraction of very distantly related pairs, relative to all relative pairs, increases. Consistent with estimates from both studies being noisy, when we combined our results with results from the IOCDF-GC sample (5) to obtain new estimates of average heritability per allele bin and heritability per chromosome, the average fit expectation was better than in either study alone.
Prior to the advent of dense genotyping, the heritability of a trait was typically estimated from its distribution within pedigrees. These kinds of studies continue to this day, in large part because they capture heritability due to both common and rare inherited genetic variation. It is thus interesting to compare our SNP-based heritability estimate from common variation, 29%, to that from Swedish families, 35%–50% (1, 4). This comparison suggests that while the majority of inherited liability for OCD traces to common genetic variation, rare variation contributes to OCD liability as well, but to a lesser degree, consistent with the findings to date regarding rare variation and risk for OCD (17–20).
The present study had strengths and limitations. We used OCD cases from the EGOS and NORDiC cohorts, the two largest OCD studies in Sweden, to examine the role of genetic and environmental factors. The EGOS cohort utilized the Swedish National Patient Register for its sampling frame, and thus it is an epidemiological cohort, minimizing selection biases, while NORDiC recruited through specialty OCD clinics across Sweden, a sampling frame more typical of case-control studies. This difference in sampling frames could introduce heterogeneity into our study. Nonetheless, when we evaluated this possibility by estimating the heritability induced by contrasting OCD case subjects from EGOS to OCD cases from NORDiC, and doing the same for control subjects, both estimated heritabilities were not significantly different from zero. Hence, while there could be subtle heterogeneity between the cohorts, it must be small. Furthermore, for both cohorts, reliance on inclusion as a result of individuals seeking care at mental health hospitals and clinics can inadvertently exclude those with milder forms of the disorder who may seek treatment from primary care providers and those who do not present to clinical services at all. If such individuals were included, and if their genetic architecture were different from that of our current OCD case sample, it would affect the estimated heritability. By restricting case subjects to individuals in Sweden, we had a genetically homogeneous sample, which minimized the risk of confounding due to population stratification and facilitated the combining of the cohorts. Nonetheless, it does limit the generalizability of our results. However, after combining our results with those of the IOCDF-GC sample (5), we observe results that fit expectation, thus suggesting that the results are likely to be relevant for most populations.
Our results provide new insights into the genomics architecture of OCD, affecting research design for genomic discovery and the ultimate clinical impact of such studies. While there is no doubt that rare and common genetic variation contributes to risk for OCD, the balance of their contributions has remained uncertain. The results from the IOCDF-GC sample and the meta-analysis of IOCDF-GC and OCGAS samples (5, 16) implied an unexpectedly large role for very common variation in OCD risk and no evidence for heritability related to rarer variation (MAF <0.05). This would be quite distinct from what is known about other psychiatric disorders, and it would be consistent with some form of evolutionary selection, such as balancing selection. Our results differ substantially from those of the earlier studies; specifically, we observed that the contribution to risk from common SNP variation follows expectations. Hence, our results do not support a role for unusual evolutionary forces playing a role in OCD risk and do support a role for rare variation in risk.
Assessing the contribution of rare variants in OCD has the additional benefit of uncovering variation of major effect, which can lead to direct insight into OCD biology and potentially pave the way for family counseling. In addition, these high-effect genes represent tools to create animal models of OCD to study pathobiology and also may represent targets for developing novel therapeutics.
As data sets become larger, risk prediction will improve, as will our ability to characterize the distribution and effects of common and rare risk variation. We conjecture that the liability arising from common and rare risk variation likely combines additively to determine risk for individuals diagnosed with OCD, similar to the risk patterns for ASD (36, 37). This knowledge can be translated into a deeper etiological understanding of OCD subtypes and their treatment and, in the future, into better predictors of OCD risk. OCD is a clinically and etiologically heterogeneous condition (38) with a complex symptom structure (39). Studies suggest that the burden of common risk alleles of OCD may differ by OCD symptom type. For example, although not yet replicated, in one study (40), compulsive symptoms rather than obsessive symptoms showed higher SNP heritability and genetic correlations with OCD. However, it is still unclear to what extent rare genetic variation, and the joint effect with common variation, may 1) differ between the subtypes of OCD, 2) interact with sex, 3) influence age at onset, and 4) affect the risk of comorbid conditions. Further research in this area could accelerate discovery of biomarkers and novel treatments, eventually helping clinicians offer patients optimal prognosis and treatment.
Pharmacogenetic studies of OCD have focused on the role of common genetic variants in treatment response (41). However, to date, no replicated significant GWAS variant has been reported for OCD—likely because of the small sample sizes—and therefore it has been challenging to contextualize the results of OCD pharmacogenetic studies. Future pharmacogenetic studies examining predictors of treatment response will likely shift focus from selected common genetic variants to genome-wide studies that also estimate how rare and common risk variants jointly affect liability (42).
The heterogeneity of OCD should always be considered in the light of psychiatric comorbidity, an approach that is facilitated in samples such as EGOS and NORDiC that are linked to national health registries. For example, in EGOS, using an epidemiological frame, approximately 40% of individuals with OCD have more than one psychiatric comorbidity, with anxiety disorders and major depressive disorder being most common (43). In addition, the severity of OCD was significantly higher in individuals with at least one additional psychiatric comorbidity compared with individuals with no psychiatric comorbidity: higher symptoms of obsessing and ordering, measured using the Obsessive Compulsive Inventory–Revised, were observed in individuals with OCD and at least one additional psychiatric comorbidity. In future studies, it will be important to investigate how the combinations of rare and common genetic variants differ in their relationship with the comorbid conditions.
In summary, our results demonstrate that the majority of inherited liability for OCD traces to common genetic variation, and the distribution of risk as a function of allele frequency is consistent with expectations of the infinitesimal quantitative genetics model. These results indicate that balancing selection or other, more complex evolutionary forces are not strongly at play in OCD. Furthermore, since the infinitesimal model assumes that genetic loci across the entire frequency spectrum influence the risk of OCD, future studies that examine the contribution of rare variation, both inherited and de novo, together with common variation, will allow a more comprehensive understanding of OCD genetic risk.
1 : Maternal effects as causes of risk for obsessive-compulsive disorder. Biol Psychiatry 2020; 87:1045–1051Crossref, Medline, Google Scholar
2 : The epidemiology of obsessive-compulsive disorder in the National Comorbidity Survey Replication. Mol Psychiatry 2010; 15:53–63Crossref, Medline, Google Scholar
3 : Overview of genetics and obsessive-compulsive disorder. Psychiatry Res 2009; 170:7–14Crossref, Medline, Google Scholar
4 : Population-based, multigenerational family clustering study of obsessive-compulsive disorder. JAMA Psychiatry 2013; 70:709–717Crossref, Medline, Google Scholar
5 : Partitioning the heritability of Tourette syndrome and obsessive compulsive disorder reveals differences in genetic architecture. PLoS Genet 2013; 9:e1003864Crossref, Medline, Google Scholar
6 : Association of perinatal risk factors with obsessive-compulsive disorder: a population-based birth cohort, sibling control study. JAMA Psychiatry 2016; 73:1135–1144Crossref, Medline, Google Scholar
7 : Systematic review of environmental risk factors for obsessive-compulsive disorder: a proposed roadmap from association to causation. Neurosci Biobehav Rev 2016; 65:36–62Crossref, Medline, Google Scholar
8 : Genetic and environmental influences on obsessional traits and symptoms. Psychol Med 1984; 14:791–800Crossref, Medline, Google Scholar
9 : Obsessive and compulsive symptoms in a general population sample of female twins. Am J Med Genet 2000; 96:791–796Crossref, Medline, Google Scholar
10 : A twin study of anxiety-related behaviours in pre-school children. J Child Psychol Psychiatry 2003; 44:945–960Crossref, Medline, Google Scholar
11 : Genetic and environmental contributions to the Child Behavior Checklist Obsessive-Compulsive Scale: a cross-cultural twin study. Arch Gen Psychiatry 2004; 61:608–616Crossref, Medline, Google Scholar
12 : Etiology of obsessions and compulsions: a meta-analysis and narrative review of twin studies. Clin Psychol Rev 2011; 31:1361–1372Crossref, Medline, Google Scholar
13 : The structure of genetic and environmental risk factors for dimensional representations of DSM-5 obsessive-compulsive spectrum disorders. JAMA Psychiatry 2014; 71:182–189Crossref, Medline, Google Scholar
14 : Genetics of obsessive-compulsive disorder. Psychol Med 2021; 51:2247–2259Crossref, Medline, Google Scholar
15 : Genome-wide association study in obsessive-compulsive disorder: results from the OCGAS. Mol Psychiatry 2015; 20:337–344Crossref, Medline, Google Scholar
16
17 : Rare and de novo variants in obsessive compulsive disorder. Eur Neuropsychopharmacol 2019; 29:S860–S861Crossref, Google Scholar
18 : Genetics of obsessive-compulsive disorder. Indian J Psychiatry 2019; 61(suppl 1):S37–S42Crossref, Medline, Google Scholar
19 : Copy number variation in obsessive-compulsive disorder and Tourette syndrome: a cross-disorder study. J Am Acad Child Adolesc Psychiatry 2014; 53:910–919Crossref, Medline, Google Scholar
20 : Whole-exome sequencing in obsessive-compulsive disorder identifies rare mutations in immunological and neurodevelopmental pathways. Transl Psychiatry 2016; 6:e764Crossref, Medline, Google Scholar
21 : Cohort profile: Epidemiology and Genetics of Obsessive-compulsive disorder and chronic tic disorders in Sweden (EGOS). Soc Psychiatry Psychiatr Epidemiol 2020; 55:1383–1393Crossref, Medline, Google Scholar
22 : Nordic OCD and Related Disorders Consortium: rationale, design, and methods. Am J Med Genet Part B Neuropsychiatr Genet, 2020; 183:38–50Crossref, Medline, Google Scholar
23 : LifeGene: a large prospective population-based study of global relevance. Eur J Epidemiol 2011; 26:67–77Crossref, Medline, Google Scholar
24 : The Anorexia Nervosa Genetics Initiative (ANGI): overview and methods. Contemp Clin Trials 2018; 74:61–69Crossref, Medline, Google Scholar
25 : Genotype harmonizer: automatic strand alignment and format conversion for genotype data integration. BMC Res Notes 2014; 7:901Crossref, Medline, Google Scholar
26 : GCTA: a tool for genome-wide complex trait analysis. Am J Hum Genet 2011; 88:76–82Crossref, Medline, Google Scholar
27 : A method to exploit the structure of genetic ancestry space to enhance case-control studies. Am J Hum Genet 2016; 98:857–868Crossref, Medline, Google Scholar
28 : Using ancestry matching to combine family-based and unrelated samples for genome-wide association studies. Stat Med 2010; 29:2932–2945Crossref, Medline, Google Scholar
29 : On the use of general control samples for genome-wide association studies: genetic matching highlights causal variants. Am J Hum Genet 2008; 82:453–463Crossref, Medline, Google Scholar
30 : Genome-wide complex trait analysis (GCTA): methods, data analyses, and interpretations. Methods Mol Biol 2013; 1019:215–236Crossref, Medline, Google Scholar
31 : The infinitesimal model: definition, derivation, and implications. Theor Popul Biol 2017; 118:50–73Crossref, Medline, Google Scholar
32 : Most genetic risk for autism resides with common variation. Nat Genet 2014; 46:881–885Crossref, Medline, Google Scholar
33 : Estimating the proportion of variation in susceptibility to schizophrenia captured by common SNPs. Nat Genet 2012; 44:247–250Crossref, Medline, Google Scholar
34 : Large-scale exome sequencing study implicates both developmental and functional changes in the neurobiology of autism. Cell 2020; 180:568–584.e23Crossref, Medline, Google Scholar
35 : Exome sequencing identifies rare coding variants in 10 genes which confer substantial risk for schizophrenia. medRxiv, September 18, 2020 (https://doi.org/10.1101/2020.09.18.20192815)Google Scholar
36 : How rare and common risk variation jointly affect liability for autism spectrum disorder. Mol Autism 2021; 12:66Crossref, Medline, Google Scholar
37 : Polygenic transmission disequilibrium confirms that common and rare variation act additively to create risk for autism spectrum disorders. Nat Genet 2017; 49:978–985Crossref, Medline, Google Scholar
38 : Towards a definitive symptom structure of obsessive-compulsive disorder: a factor and network analysis of 87 distinct symptoms in 1366 individuals. Psychol Med 2021; 1–13Crossref, Medline, Google Scholar
39 : Meta-analysis of the symptom structure of obsessive-compulsive disorder. Am J Psychiatry 2008; 165:1532–1542Link, Google Scholar
40 : Genetic meta-analysis of obsessive-compulsive disorder and self-report compulsive symptoms. Am J Med Genet B Neuropsychiatr Genet 2020; 183:208–216Crossref, Medline, Google Scholar
41 : Pharmacogenetics of antidepressant treatment in obsessive-compulsive disorder: an update and implications for clinicians. Pharmacogenomics 2014; 15:1147–1157Crossref, Medline, Google Scholar
42 : Could polygenic risk scores be useful in psychiatry? A review. JAMA Psychiatry 2021; 78:210–219Crossref, Medline, Google Scholar
43 : OCD symptom severity and comorbid psychiatric diagnoses in a Swedish genetic epidemiological obsessive-compulsive disorder cohort. medRxiv, July 1, 2021 (https://doi.org/10.1101/2021.06.28.21259652)Google Scholar