Reduced Neural Tracking of Prediction Error in Substance-Dependent Individuals
Abstract
Objective
Substance-dependent individuals make poor decisions on the Iowa Gambling Task, a reward-related decision-making task that involves risk and uncertainty. Task performance depends on several factors, including how sensitive individuals are to feedback and how well they learn based on such feedback. A physiological signal that guides decision making based on feedback is prediction error. The authors investigated whether disruptions in the neural systems underlying prediction error processing in substance-dependent individuals could account for decision-making performance on a modified Iowa Gambling Task.
Methods
Thirty-two substance-dependent individuals and 30 healthy comparison subjects played a modified version of the Iowa Gambling Task during MR scanning. Trial-to-trial behavior and functional MRI (fMRI) blood-oxygen-level-dependent (BOLD) signal were analyzed using a computational model of prediction error based on internal expectancies. The authors investigated how well BOLD signal tracked prediction error in the striatum and the orbitofrontal cortex as well as over the whole brain in patients relative to comparison subjects.
Results
Compared with healthy subjects, substance-dependent patients were less sensitive to loss compared with gain, made less consistent choices, and performed worse on the modified Iowa Gambling Task. The ventral striatum and medial orbitofrontal cortex did not track prediction error as strongly in patients as in healthy subjects.
Conclusions
Weaker tracking of prediction error in substance-dependent relative to healthy individuals suggests that altered frontal-striatal error learning signals may underlie decision-making impairments in drug abusers. Computational fMRI may help bridge the knowledge gap between physiology and behavior to inform research aimed at substance abuse treatment.
Drug addiction is characterized by poor decision making associated with urges to seek and take drugs despite knowledge of harmful consequences.
Few model-based fMRI studies of prediction error learning have been conducted in drug users. One study in smokers (8) and another in alcohol-dependent men (9) did not find evidence of altered prediction error learning signals in the striatum. While nicotine and alcohol share mechanisms of drug addiction, most of the research suggesting maladaptive predictive learning involves stimulants. Yet, no study to date has investigated prediction error learning signals in stimulant-dependent individuals using a clinically valid measure of decision making.
The Iowa Gambling Task is a reward-related decision-making task involving risk and uncertainty. It is sensitive to decision-making deficits in patients with ventral medial frontal lesions and in clinical populations that include substance users (10–13). The goal of the task is to earn as much money as possible by selecting from four decks of cards. Two of the decks are associated with large gains and occasional large losses that, over time, yield a net loss; the other two decks are associated with small gains and small losses that, over time, yield a net gain. In order to succeed, subjects must learn to forgo the “risky decks” with immediate large gains in favor of “safe decks” with small gains. Task performance is influenced by sensitivity to reward and risk, temporal foresight, mental flexibility, and emotion. Cognitive models have been useful for separating some of these processes and have shown, for example, that compared with healthy subjects, cocaine users’ performance on the task reflects lower sensitivity to loss and lower response consistency (14).
Our goal in this study was to determine whether the tracking of prediction error is altered in stimulant-dependent individuals. We used a computational model of prediction error and a modified version of the Iowa Gambling Task to test the hypothesis that substance-dependent individuals would have reduced tracking of prediction error in the striatum and orbitofrontal cortex relative to comparison subjects. Such a finding could suggest a mechanism for poor decision making in substance users and inform future research aimed at therapy.
Method
Subjects
Forty-two substance-dependent patients and 36 healthy comparison subjects were recruited. Ten of the substance-dependent patients and six comparison subjects were excluded (three were excluded because of diagnostic exclusions, four because of scanner failure, and nine because of head motion exceeding 3 mm). Data are reported on 32 substance-dependent patients (19 of them male; age range, 22–50 years, with a mean of 36.6 years [SD=9.0]) and 30 comparison subjects (15 of them male; age range, 20–49 years, with a mean of 34.5 years [SD=7.5]), on whom some behavioral data have been published previously (24). The two groups had a similar mean IQ (comparison group: mean=103.3, SD=12.7, range=82–126; patient group: mean=102.2, SD=10.3, range=83–125).
Patients with DSM-IV stimulant dependence were recruited from a residential drug treatment program at the University of Colorado Denver Addiction Research and Treatment Service. Other dependence diagnoses and the duration of dependence and abstinence are listed in Table 1. Average abstinence was 1.9 years.
Patients (N=32) | Comparison Subjects (N=30) | |||||||||||
---|---|---|---|---|---|---|---|---|---|---|---|---|
Duration of Dependence (Years) | Duration of Abstinence (Years) | Duration of Dependence (Years) | Duration of Abstinence (Years) | |||||||||
Drug | N | % | Mean | Range | Mean | Range | N | % | Mean | Range | Mean | Range |
Stimulants | 32 | 100 | 14.4 | 2–34 | 1.7 | 0.3–6 | 0 | |||||
Nicotine | 26 | 81 | 21.6 | 5–37 | 0.3 | 0–2 | 5 | 17 | 22.0 | 10–32 | 4.8 | 0–14 |
Alcohol | 15 | 47 | 19.5 | 5–31 | 1.3 | 0.1–3 | 0 | |||||
Opioids | 5 | 16 | 16.4 | 5–28 | 1.8 | 0.4–6 | 0 | |||||
Cannabis | 4 | 13 | 19.8 | 11–31 | 0.9 | 0.4–2 | 0 | |||||
Othera | 7 | 22 | 8.3 | 2–13 | 7.5 | 3–8 | 0 |
Community comparison subjects were recruited using advertisements, flyers, and a marketing company. Volunteers were excluded if they met DSM-IV criteria for drug or alcohol dependence.
Exclusion criteria included head trauma with loss of consciousness exceeding 15 minutes, neurological disease, schizophrenia, bipolar disorder, and current major depression. All participants provided written informed consent as approved by the Colorado Multiple Institutional Review Board and completed diagnostic and structured interviews, behavioral tasks, and MRI scanning.
Interviews and Tasks
We used the Composite International Diagnostic Interview–Substance Abuse Module (25), a computerized structured interview, to characterize the DSM-IV dependence diagnoses for amphetamine, cocaine, marijuana, alcohol, tobacco, hallucinogens, opioids, inhalants, sedatives, club drugs, and phencyclidine. Specific modules of the Diagnostic Interview Schedule–Version IV were administered to exclude schizophrenia, bipolar disorder, current major depression (<2 months) and to assess for antisocial personality disorder, which was not exclusionary. IQ was assessed with the Wechsler Abbreviated Scale of Intelligence (WASI). The WASI Two-Subtest Form was used to estimate general intelligence (Psychological Corporation, 1999). We also administered the Barratt Impulsiveness Scale, a 30-item self-report questionnaire that provides a measure of impulsivity (26).
We used a modified version of the Iowa Gambling Task (27). Subjects were shown four decks of cards and instructed to try to earn as much money as possible by choosing to either play or pass on a given deck. “Play” resulted in a single positive or negative monetary value, along with the running total. “Pass” resulted in no change. Decks differed in the magnitude and frequency of wins and losses. Two of the four decks resulted in a net gain; the other two decks resulted in a net loss. To perform well, subjects had to learn to “play” good decks and “pass” bad decks over time. Task details have been described elsewhere (24); a diagram of the task is provided in the data supplement that accompanies the online edition of this article. The decision period was 2 seconds, and the outcome period was 4 seconds. Each deck was presented 50 times (for a total of 200 trials) in pseudorandom order, interspersed with 65 6-second fixation trials. Total scan time was 26.4 minutes, divided into three runs of 8.8 minutes each.
Expectancy Valence Model
Prediction error is the difference between expected and received outcome. For each subject and for each trial, prediction error was computed as the difference between the individual’s internally generated expected outcome (termed “valence”) and actual outcome. The expectancy valence model, adapted from Stout et al. (28), deconstructs decision making on the Iowa Gambling Task into three processes: sensitivity to loss (ω), recency or updating (α), and response consistency (c). Model details are provided in the online data supplement.
MRI Acquisition and Preprocessing
Acquisition.
Images were acquired on a 3.0-T scanner using a quadrature head coil. Functional images were acquired with a gradient-echo T2* blood-oxygen-level-dependent (BOLD) contrast technique, TR=2,000 ms, TE=30 ms, field-of-view 220 mm2, 64×64 matrix, 30 slices, 4 mm thick, no gap, angled parallel to the planum sphenoidale. To reduce inferior frontal susceptibility artifact, we used a volume-selective z-shimmed image acquisition (29). MR-compatible goggles were used for visual stimuli, and responses were recorded with a two-button response device.
Preprocessing.
The first four image volumes from each run were excluded for saturation effects. Data were processed and analyzed using SPM8 (http://www.fil.ion.ucl.ac.uk/spm/software/spm8/). Functional data were realigned to the first volume. Data were excluded for head motion exceeding 3 mm. The realigned images were normalized to Montreal Neurological Institute space. Data were smoothed with a 6-mm full width at half maximum Gaussian kernel. The final smoothness of the data after preprocessing was 8.2×8.4×7.9 mm3 and was used in the Monte Carlo simulations to determine cluster-wise corrected threshold levels.
fMRI Data Analysis
First-level model.
fMRI time-series data were analyzed using the general linear model after filtering low-frequency noise, correcting for temporal autocorrelation, and convolving the stimulus function with a canonical hemodynamic response function. The regressor of interest was the trial-to-trial prediction error. Regressors of no interest were deck, run, and play. The play regressor controls for the mean effect of a play response during the stimulus phase. By including this regressor, the variability in signal captured by the prediction error regressor is more likely to capture the processes associated with prediction error computed at the time of the outcome.
Second-level model.
Contrast maps for the prediction error regressor were brought to the second level and analyzed using whole-brain and region-of-interest analyses.
Whole brain.
Whole-brain group comparison was conducted using a two-sample t test with education as a covariate, as this variable differed between groups. The statistical threshold was set at a voxel level of p<0.005, uncorrected, and p<0.01 whole-brain cluster-corrected, corresponding to an extent threshold of 39 voxels based on 10,000 Monte Carlo simulations using AlphaSim in the AFNI program (http://afni.nimh.nih.gov/afni/).
Regions of interest.
Based on the literature, four regions of interest were defined: the ventral striatum, the dorsal striatum, the medial orbitofrontal cortex, and the lateral orbitofrontal cortex. The medial orbitofrontal cortex consisted of Brodmann’s area (BA) 25, BA 32, and medial BA 11. The lateral orbitofrontal cortex consisted of BA 47, BA 12, and BA 10. The boundaries of the ventral and dorsal striatum were manually drawn (30). Regions of interest were created using the Wake Forest University PickAtlas (see the online data supplement). Time-series data were extracted for each region of interest using the MarsBaR toolbox in SPM8.
Statistical Analysis
Demographic and behavioral data were tested for normality and analyzed with two-tailed t tests or chi-square tests, for continuous and categorical variables, respectively.
Modified Iowa Gambling Task performance and valence model parameters.
Performance was analyzed with a 2×2 (group-by-time) repeated-measures analysis of variance on net score. Net score is the number of plays on good decks minus the number of plays on bad decks. Since there were 50 trials for each deck, net score was calculated for the first half and for the second half, consisting of 25 trials each.
The three parameters (ω, α, c) from the expectancy valence model of the Iowa Gambling Task were analyzed with a bootstrapping procedure because the parameters were highly skewed. Parameters were compared across the group by bootstrapping the sampling distribution of the mean difference between groups (5,000 iterations).
Regions of interest.
For each region, fMRI signal (beta estimates) for prediction error tracking was first analyzed in all subjects using a one-sample t test and then compared across groups using a two-sample t test after adjusting for education.
Correlations among fMRI signal, behavioral measures, and drug characteristics.
fMRI signal, duration of abstinence, duration of drug use, impulsivity score, and decision-making parameters were correlated within group using Pearson’s r and Spearman’s rho for normal and nonnormal data, respectively.
Results
Demographic Characteristics and Behavior
The patient and comparison groups were not significantly different in mean age (36.6 years [SD=9.0] and 34.5 years [SD=7.5], respectively), gender distribution (19 men and 13 women compared with 15 men and 15 women), and mean IQ (102.2 [SD=10.3] compared with 103.3 [SD=12.7]). Comparison subjects had more years of education on average than did patients (14.3 years [SD=1.9] compared with 12.0 years [SD=2.3], p<0.001), so education was entered as a covariate in all group comparisons. Patients were more impulsive on average than comparison subjects (mean Barratt Impulsiveness Scale score, 70.1 [SD=9.5] compared with 59.7 [SD=7.0], p<0.001). Thirty-two patients and 11 comparison subjects met criteria for antisocial personality disorder.
Drug Characteristics
Table 1 lists dependence diagnoses and duration of dependence and abstinence.
Behavioral Performance on the Modified Iowa Gambling Task
As shown in Figure 1, a significant group-by-time interaction was observed on net score, which improved over time in the comparison group but not in the patient group (F=4.09, df=1, 58, p=0.05; in the comparison group, the mean net score was 4.62 [SD=1.3] for the first 25 trials and 6.97 [SD=1.7] for the next 25 trials; in the patient group, these values were 4.18 [SD=1.3] and 4.05 [SD=1.62], respectively).
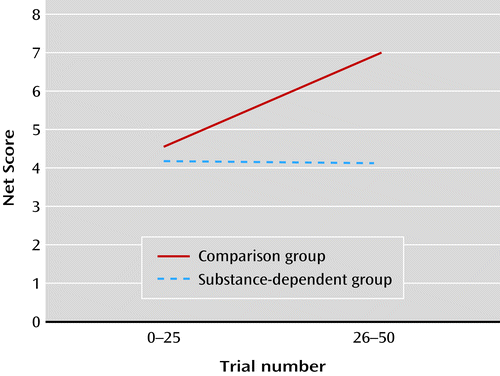
a Net score is the number of plays on good decks minus the number of plays on bad decks; it was calculated for the first half and for the second half of the task, consisting of 25 trials each. The figure shows improvement over time in net score in the comparison group but not in the substance-dependent group.
Expectancy valence model parameters (ω, α, c).
Patients’ decisions were significantly less consistent over time than those of the comparison subjects (c=−0.46 and c=−0.01, respectively; p=0.02). Patients also showed a lower sensitivity to loss, although this difference did not reach statistical significance (ω=0.16 and ω=0.26, respectively; p=0.06). There was no group difference in recency (α) (see Table S4 in the online data supplement).
Imaging
Whole-brain analysis.
Figure 2 shows prediction error-related signal in the ventral striatum and medial orbitofrontal cortex in the comparison group and the patient group, as well as the group difference. Patients had weaker tracking of prediction error in the medial orbitofrontal cortex. Table 2 lists significant clusters of lower prediction error-related activity in patients relative to comparison subjects in the left insula, thalamus, and left superior temporal gyrus. There were no regions of higher prediction error-related fMRI signal in patients relative to comparison subjects.
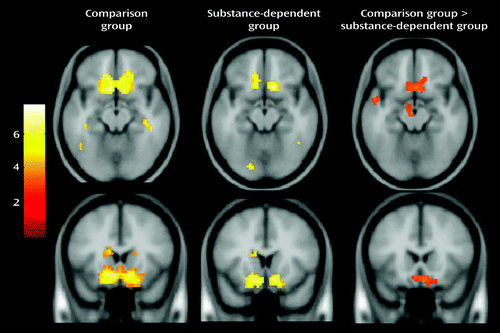
a Difference maps show higher activity in the medial orbitofrontal cortex in the comparison group relative to the substance-dependent group. All maps are thresholded at p<0.01, corrected for multiple comparisons using cluster correction, voxel-level p<0.005.
MNI Coordinatesa | |||||
---|---|---|---|---|---|
Contrast and Region | x | y | z | t | Extent (df=61) |
Comparison subjects > patients | |||||
Left medial orbitofrontal cortex | –15 | 48 | –18 | 4.13 | 214 |
Right medial orbitofrontal cortex | 15 | 33 | –18 | 4.07 | |
5 | 9 | –29 | 3.50 | ||
Thalamus | 0 | –15 | 0 | 4.10 | 154 |
18 | –18 | 12 | 3.47 | ||
15 | –9 | 6 | 3.42 | ||
Left insula | –39 | 30 | 24 | 4.02 | 94 |
–33 | –30 | 24 | 3.63 | ||
–30 | –18 | 12 | 3.59 | ||
Left superior temporal gyrus | –54 | –3 | –12 | 3.51 | 45 |
–51 | –18 | –5 | 3.00 | ||
Patients > comparison subjects | |||||
No regions |
Region-of-interest analysis.
Table 3 presents the results of one-sample t tests across all subjects and two-sample t tests of prediction error fMRI signal. Across all subjects, there was tracking of prediction error in the ventral and dorsal striatum and the medial orbitofrontal cortex. Relative to comparison subjects, patients did not track prediction error as strongly in the medial orbitofrontal cortex (t=2.3, df=61, p=0.01) and the ventral striatum (t=1.9, df=61, p=0.03). There were no group differences in the dorsal striatum or the lateral orbitofrontal cortex.
Region | All | Comparison Subjects > Patients | Patients > Comparison Subjects |
---|---|---|---|
Dorsal striatum | <0.001 | 0.12 | 0.88 |
Ventral striatum | <0.001 | 0.03 | 0.97 |
Medial orbitofrontal cortex | 0.01 | 0.01 | 0.93 |
Lateral orbitofrontal cortex | 0.10 | 0.07 | 0.99 |
Correlations.
In the comparison group, decision-making consistency correlated with fMRI signal in the lateral (r=0.39, p<0.05) and medial orbitofrontal cortex (r=0.43, p<0.05). Recency correlated negatively with fMRI signal in the lateral orbitofrontal cortex (r=−0.37, p<0.05). Impulsivity correlated negatively with response consistency (r=−0.38, p<0.05). In contrast, in the patient group, fMRI signal did not correlate with any behavioral measures.
Discussion
Effect of Substance Dependence on Striatal Prediction Error
Effect of Substance Dependence on Orbitofrontal Cortex Expectancies
Cue-selective firing during prediction learning is not unique to the striatum but is also found in prefrontal cortex neurons (33, 34). Stalnaker et al. (35) showed that cocaine-treated rats fail to develop cue-selective firing in orbitofrontal cortex neurons using the odor discrimination task described above. Furthermore, when the odor-outcome pairings were reversed, these neurons failed to demonstrate normal reversal of cue-selective firing in cocaine-treated compared with saline-treated rats, suggesting that drugs disrupt learning signals processed in the orbitofrontal cortex. Our finding of weaker tracking of prediction error in the medial orbitofrontal cortex in patients relative to comparison subjects is consistent with these studies. Drugs may disrupt outcome expectancies encoded in the orbitofrontal cortex and prevent the striatum from accurately updating prediction error (34). This is consistent with reduced frontal-striatal connectivity reported in alcohol-dependent patients and suggests a disruption in the transfer of prediction error signals from the striatum to the orbitofrontal cortex (9). Another hypothesis is that dopaminergic striatal activity serves as a gating signal to the prefrontal cortex and that drug exposure dampens the ability of natural rewards to open the gating so that the orbitofrontal cortex can influence decision making (5).
Our finding that group differences in prediction error involved the medial more than the lateral orbitofrontal cortex confirms the critical role of the ventromedial prefrontal cortex in performance on the Iowa Gambling Task (27). It also supports the notion that the medial and lateral orbitofrontal cortex play distinct roles in reward-related decision making. The medial orbitofrontal cortex has been more strongly associated with motivation, uncertainty, valuation, and effort. The lateral orbitofrontal cortex has been associated with processing aversive outcomes (36) or suppressing unrewarded responses (37). The lateral orbitofrontal cortex is thought to exert top-down control, planning how to achieve goals and passing the information to the medial orbitofrontal cortex, which evaluates the cost-benefit balance of those plans and estimates and monitors expected value (38). In our study, fMRI signal in the orbitofrontal cortex correlated positively with response consistency and negatively with recency valence estimates in comparison subjects but not in patients, suggesting that the orbitofrontal cortex plays a role in computing expected valence and may be affected in drug users. These correlates were weak, however, and would need to be replicated.
Model-Based fMRI in Drug Users
Although this was not the first study to investigate neural correlates of prediction error in addiction, it is the first to report differences in individuals with stimulant dependence. Park et al. (9) reported no difference in striatal prediction error in alcohol-dependent compared with healthy men performing a probabilistic learning task, while Chiu et al. (8) found no difference in smokers compared with nonsmokers playing a stock market simulation task. Our study differs from these studies in several ways. First, the substance use problems in our population were more severe. Second, we used a non-commercially available z-shim MR pulse sequence that increases signal in the orbitofrontal region (29). Third, the study tasks differed in cognitive demand. Finally, the use of internally generated (i.e., subjective) prediction error signals could be more sensitive than externally computed (i.e., objective) prediction error signals. For example, Stout et al. (39) showed that a cognitive model of internally generated valence predicted behavior on the Iowa Gambling Task better than a linear model based on externally computed valence, consistent with our finding that the valence model described task behavior better than a conventional reinforcement learning model. We argue that brain activity should more closely track internally generated compared with externally computed expectancy.
Cognitive Processes Underlying Decision Making on the Modified Iowa Gambling Task
Relative to the comparison group, the patient group did not show an improvement in decision making over time, consistent with previous studies (12, 40). Performance on the Iowa Gambling Task is influenced by many factors, including reward sensitivity, ability to forgo short-term in favor of long-term gain, impulsiveness, and cognitive flexibility. The expectancy valence model disentangles some of the processes (14) by parameterizing sensitivity to gain and loss (risk), discounting of prior experiences (recency), and consistency between expectancies and choice (28). Despite the modifications to the original Iowa Gambling Task (i.e., subjects were not free to select but rather a deck was selected for them), our results were consistent with previous model studies in drug users (28, 41). The finding that substance users’ decisions were less consistent and less sensitive to loss than comparison subjects’ may reflect impulsiveness and “myopia for the future.”
Most neuroimaging studies of the Iowa Gambling Task model specific trial conditions (i.e., decision versus no decision or high versus low risk). Brain regions implicated in these models include the medial and lateral prefrontal cortex, the cingulate cortex, the striatum, and the insula (16–19). These models, however, fail to account for learning. To approximate this critical aspect of the task, Li et al. (18) compared brain activity from the first and last blocks of the task and found that orbitofrontal cortex activity increased over time. In contrast, Lawrence et al. (19) reported less activity in the orbitofrontal cortex and insula over time. One possibility for these inconsistencies is that individuals learn at different rates. Model-based fMRI accounts for variations in learning and has been applied to the Iowa Gambling Task (18, 23). Christakou et al. (23) found that the right ventromedial prefrontal cortex was sensitive to individuals’ negative expectancies. Although we did not separate positive from negative expectancies, our finding of ventral medial activity related to global expectancy is consistent with these results (23). Li et al. (18) also found that prediction error correlated with activity in the ventral striatum and the middle frontal gyri. Both of these previous studies, however, used externally derived prediction estimates. Our study extends model-based fMRI studies for the first time using internally derived prediction estimates in substance-dependent individuals.
Strengths of this study include the use of a computational model based on internally generated valence, the relatively large sample size of 32 patients, the use of an MR pulse sequence that increases orbitofrontal cortex signal compared with conventional MRI sequences, and a comparison group matched on IQ. Limitations include an inability to directly compare results to the Iowa Gambling Task, an inability to determine whether drug use preceded or antedated neural changes, the abuse of multiple drug classes in our sample, and the lack of control for medications. We cannot exclude the possibility that antisocial personality disorder could account for some results, as this diagnosis was common in both groups.
In summary, decision-making deficits in substance users compared with healthy subjects were related to inconsistent choices and reduced neural tracking of prediction error signals in the striatum and orbitofrontal cortex, suggesting that frontal-striatal pathways involved in learning may be a target for future treatment.
1 : Neuronal coding of prediction errors. Annu Rev Neurosci 2000; 23:473–500Crossref, Medline, Google Scholar
2 : Addiction as a computational process gone awry. Science 2004; 306:1944–1947Crossref, Medline, Google Scholar
3 : Cocaine exposure shifts the balance of associative encoding from ventral to dorsolateral striatum. Front Integr Neurosci 2007; 1:11Crossref, Medline, Google Scholar
4 : A neural substrate of prediction and reward. Science 1997; 275:1593–1599Crossref, Medline, Google Scholar
5 : Addiction: a disease of learning and memory. Am J Psychiatry 2005; 162:1414–1422Link, Google Scholar
6 : Addiction: beyond dopamine reward circuitry. Proc Natl Acad Sci USA 2011; 108:15037–15042Crossref, Medline, Google Scholar
7 : Computational psychiatry. Trends Cogn Sci 2012; 16:72–80Crossref, Medline, Google Scholar
8 : Smokers’ brains compute, but ignore, a fictive error signal in a sequential investment task. Nat Neurosci 2008; 11:514–520Crossref, Medline, Google Scholar
9 : Prefrontal cortex fails to learn from reward prediction errors in alcohol dependence. J Neurosci 2010; 30:7749–7753Crossref, Medline, Google Scholar
10 : Decision-making biases, antisocial personality, and early-onset alcoholism. Alcohol Clin Exp Res 2000; 24:1036–1040Crossref, Medline, Google Scholar
11 : Drug abusers show impaired performance in a laboratory test of decision making. Neuropsychologia 2000; 38:1180–1187Crossref, Medline, Google Scholar
12 : Decision-making deficits, linked to a dysfunctional ventromedial prefrontal cortex, revealed in alcohol and stimulant abusers. Neuropsychologia 2001; 39:376–389Crossref, Medline, Google Scholar
13 : Decision-making and addiction (part II): myopia for the future or hypersensitivity to reward? Neuropsychologia 2002; 40:1690–1705Crossref, Medline, Google Scholar
14 : Using cognitive models to map relations between neuropsychological disorders and human decision-making deficits. Psychol Sci 2005; 16:973–978Crossref, Medline, Google Scholar
15 : Decision-making in a risk-taking task: a PET study. Neuropsychopharmacology 2002; 26:682–691Crossref, Medline, Google Scholar
16 : Functional activity related to risk anticipation during performance of the Iowa Gambling Task. Neuroimage 2005; 24:253–259Crossref, Medline, Google Scholar
17 : Prefrontal cortex activity is reduced in gambling and nongambling substance users during decision-making. Hum Brain Mapp 2007; 28:1276–1286Crossref, Medline, Google Scholar
18 : The Iowa Gambling Task in fMRI images. Hum Brain Mapp 2010; 31:410–423Medline, Google Scholar
19 : Distinct roles of prefrontal cortical subregions in the Iowa Gambling Task. Cereb Cortex 2009; 19:1134–1143Crossref, Medline, Google Scholar
20 : Poor decision-making by chronic marijuana users is associated with decreased functional responsiveness to negative consequences. Psychiatry Res 2011; 191:51–59Crossref, Medline, Google Scholar
21 : Model-based fMRI and its application to reward learning and decision making. Ann NY Acad Sci 2007; 1104:35–53Crossref, Medline, Google Scholar
22 : Neural correlates of risk prediction error during reinforcement learning in humans. Neuroimage 2009; 47:1929–1939Crossref, Medline, Google Scholar
23 : Right ventromedial and dorsolateral prefrontal cortices mediate adaptive decisions under ambiguity by integrating choice utility and outcome evaluation. J Neurosci 2009; 29:11020–11028Crossref, Medline, Google Scholar
24 Negative reinforcement learning is affected in substance dependence. Drug Alcohol Depend 2012; 123:84–90Crossref, Medline, Google Scholar
25 : The reliability of the CIDI-SAM: a comprehensive substance abuse interview. Br J Addict 1989; 84:801–814Crossref, Medline, Google Scholar
26 : Factor structure of the Barratt Impulsiveness Scale. J Clin Psychol 1995; 51:768–774Crossref, Medline, Google Scholar
27 : Insensitivity to future consequences following damage to human prefrontal cortex. Cognition 1994; 50:7–15Crossref, Medline, Google Scholar
28 : Cognitive modeling analysis of decision-making processes in cocaine abusers. Psychon Bull Rev 2004; 11:742–747Crossref, Medline, Google Scholar
29 : Reducing susceptibility artifacts in fMRI using volume-selective z-shim compensation. Magn Reson Med 2007; 57:396–404Crossref, Medline, Google Scholar
30 : Imaging human mesolimbic dopamine transmission with positron emission tomography, I: accuracy and precision of D(2) receptor parameter measurements in ventral striatum. J Cereb Blood Flow Metab 2001; 21:1034–1057Crossref, Medline, Google Scholar
31 : Cocaine cues and dopamine in dorsal striatum: mechanism of craving in cocaine addiction. J Neurosci 2006; 26:6583–6588Crossref, Medline, Google Scholar
32 : Silencing the critics: understanding the effects of cocaine sensitization on dorsolateral and ventral striatum in the context of an actor/critic model. Front Neurosci 2008; 2:86–99Crossref, Medline, Google Scholar
33 : The orbitofrontal cortex: neuronal activity in the behaving monkey. Exp Brain Res 1983; 49:93–115Crossref, Medline, Google Scholar
34 : Orbitofrontal cortex, decision-making, and drug addiction. Trends Neurosci 2006; 29:116–124Crossref, Medline, Google Scholar
35 : Abnormal associative encoding in orbitofrontal neurons in cocaine-experienced rats during decision-making. Eur J Neurosci 2006; 24:2643–2653Crossref, Medline, Google Scholar
36 : Opponent appetitive-aversive neural processes underlie predictive learning of pain relief. Nat Neurosci 2005; 8:1234–1240Crossref, Medline, Google Scholar
37 : Dissociable functions in the medial and lateral orbitofrontal cortex: evidence from human neuroimaging studies. Cereb Cortex 2000; 10:308–317Crossref, Medline, Google Scholar
38 : Orbitofrontal cortex and its contribution to decision-making. Annu Rev Neurosci 2007; 30:31–56Crossref, Medline, Google Scholar
39 : Psychological processes underlying risky decisions in drug abusers. Psychol Addict Behav 2005; 19:148–157Crossref, Medline, Google Scholar
40 : Substance abuse, pathological gambling, and impulsiveness. Drug Alcohol Depend 2001; 63:29–38Crossref, Medline, Google Scholar
41 : Cognitive mechanisms underlying risky decision-making in chronic cannabis users. J Math Psychol 2010; 54:28–38Crossref, Medline, Google Scholar