The Relationship Between White Matter Microstructure and General Cognitive Ability in Patients With Schizophrenia and Healthy Participants in the ENIGMA Consortium
Abstract
Objective:
Schizophrenia has recently been associated with widespread white matter microstructural abnormalities, but the functional effects of these abnormalities remain unclear. Widespread heterogeneity of results from studies published to date preclude any definitive characterization of the relationship between white matter and cognitive performance in schizophrenia. Given the relevance of deficits in cognitive function to predicting social and functional outcomes in schizophrenia, the authors carried out a meta-analysis of available data through the ENIGMA Consortium, using a common analysis pipeline, to elucidate the relationship between white matter microstructure and a measure of general cognitive performance, IQ, in patients with schizophrenia and healthy participants.
Methods:
The meta-analysis included 760 patients with schizophrenia and 957 healthy participants from 11 participating ENIGMA Consortium sites. For each site, principal component analysis was used to calculate both a global fractional anisotropy component (gFA) and a fractional anisotropy component for six long association tracts (LA-gFA) previously associated with cognition.
Results:
Meta-analyses of regression results indicated that gFA accounted for a significant amount of variation in cognition in the full sample (effect size [Hedges’ g]=0.27, CI=0.17–0.36), with similar effects sizes observed for both the patient (effect size=0.20, CI=0.05–0.35) and healthy participant groups (effect size=0.32, CI=0.18–0.45). Comparable patterns of association were also observed between LA-gFA and cognition for the full sample (effect size=0.28, CI=0.18–0.37), the patient group (effect size=0.23, CI=0.09–0.38), and the healthy participant group (effect size=0.31, CI=0.18–0.44).
Conclusions:
This study provides robust evidence that cognitive ability is associated with global structural connectivity, with higher fractional anisotropy associated with higher IQ. This association was independent of diagnosis; while schizophrenia patients tended to have lower fractional anisotropy and lower IQ than healthy participants, the comparable size of effect in each group suggested a more general, rather than disease-specific, pattern of association.
Schizophrenia is a leading cause of disability worldwide (1). Although this disability is typically characterized by clinical symptom severity, the cognitive deficits associated with the disorder strongly predict social and functional outcomes (2–4). These deficits are observed across multiple cognitive domains (5), suggesting that broad, rather than regionally specific, changes in brain function are likely to underpin these deficits.
At a neural systems level, robust evidence of widespread differences in both white and gray matter has been demonstrated in large samples of patients with schizophrenia compared with healthy participants (6, 7). Compared with healthy participants, people with schizophrenia show widespread thinning of cortical gray matter and reduced cortical surface area, particularly in frontal and temporal lobe regions (7). Analysis of subcortical gray matter volumes has similarly shown evidence of widespread differences, including bilateral volume abnormalities of the hippocampus, amygdala, and thalamus (8). Recently, in the largest diffusion tensor imaging (DTI) study of white matter abnormalities undertaken to date, widespread reductions in fractional anisotropy (FA) were observed for a majority (19/25) of tracts, with the largest effects observed for global white matter FA, and more locally, in large tracts including the anterior corona radiata and the corpus callosum (6).
Taken collectively, these widespread abnormalities indicate a disease pathology reflective of generalized changes to the brain’s structural network and functions. This is consistent with the disconnectivity hypothesis of schizophrenia (9, 10), which suggests that functional impairments and disability result from abnormal and inefficient communication among distributed networks of brain regions (11, 12). This hypothesis would be further supported if these indices of disconnectivity could be directly related to variation in cognitive performance and functional outcomes, but well-powered studies in this area are lacking.
To address this gap, we aimed in this study to examine the relationship between brain structure and cognitive function on a large, global scale. To do this, we carried out a meta-analysis of available data through the ENIGMA Consortium, using a common analysis pipeline, to elucidate the relationship between white matter microstructure and a measure of general cognitive performance, IQ, in patients with schizophrenia and healthy participants. We hypothesized that 1) a significant positive correlation would be observed between white matter microstructure and IQ across samples, and 2) this association would be moderated by diagnosis.
Methods
Meta-Analysis
Study sample.
Data for this study were collected via the ENIGMA Schizophrenia DTI Working Group and consisted of a subsample of participating sites in a previously published study (6). Inclusion criteria for the present study were based on the availability of data processed using the ENIGMA DTI protocol and measures of estimated IQ for each participant in a given data set. The final sample consisted of 11 sites with data on both DTI and IQ, totaling 957 healthy control participants and 760 patients. Each study sample had been assessed with all participants’ written informed consent and approved by the relevant local institutional review boards. Individuals whose diffusion images were of poor quality were excluded from the analysis.
Measurement of IQ.
The Wechsler Scale of Adult Intelligence (WAIS) was used to estimate IQ in all 11 studies. Ten sites used the English version of the test (WAIS, 3rd edition); at the Madrid site, the Spanish version of the WAIS was used. The WAIS consists of a battery of verbal and nonverbal subtests, scores of which are combined to derive scores for verbal IQ, performance IQ, and full-scale (total) IQ. Because not all sites had both verbal and performance scores, our analyses were based exclusively on full-scale IQ scores. The number of subtests used to determine this full-scale score also varied among sites. Following previous large-scale multisite analyses of IQ in schizophrenia by other consortia (e.g., COGENT [13]), IQ scores calculated for all sites were based on prorated subtest scores. For nine of the 11 sites, full-scale IQ was based on three or more subtests, and at two sites, it was calculated on the basis of only the two subtests that were available for those samples (the Australian Schizophrenia Research Bank [ASRB] and the Maryland Psychiatric Research Center [MPRC]).
Image acquisition and processing.
Image data were acquired using site-specific diffusion MRI sequences. Details of study type, scanner, and acquisition parameters for each site are presented in Table S1 in the online supplement. For each site, preprocessing, including eddy current correction, echo-planar imaging-induced distortion correction, and tensor fitting, was carried out locally based on local protocols and procedures, and was further informed by quality control pipelines available at the ENIGMA DTI web site (http://enigma.ini.usc.edu/protocols/dti-protocols) and the Neuroimaging Informatics Tools and Resources Clearinghouse site. To correct for motion during image acquisition, preprocessing included the alignment of diffusion-weighted images to the b=0 using linear image registration. Individual subject data with excessive motion were not included in this analysis. As per Kelly et al. (6), harmonization of preprocessing schemes was not enforced across sites, to allow individual sites to use existing pipelines that may be more appropriate for their data acquisition. After preprocessing, harmonized image analysis of DTI measures of FA was then conducted at each site in exactly the same manner using the ENIGMA DTI protocol. The ENIGMA DTI protocol using tract-based spatial statistics (TBSS) (14) outputs averaged FA across all white matter tracts (listed in Table S2 in the online supplement). The TBSS output includes FA values for both right and left white matter tracts and an average FA value based on the average FA from both hemispheres. Average FA values were used in this analysis to minimize multiple comparisons and any potential issues of site-based left/right flipping, which would limit interpretations of a lateralized analyses.
Statistical Analysis
Per-site analysis.
Calculation of FA is based on specific acquisition protocols, including scanner make and model, diffusion sequence parameters, methods of tensor estimation models, and processing pipelines (15, 16). To overcome this systematic limitation, preliminary analysis was carried out individually at each site to assess the association between white matter tract microstructure and estimates of IQ. Only then were summary statistics compared, thus removing the issue of variances across sites due to scanner or acquisition parameters.
Per-site latent fractional anisotropy factor analysis.
To reduce the burden of multiple testing, we undertook principal component analysis of white matter tracts indexed by diffusion tensor imaging to index white matter. For each site separately, principal component analysis, implemented in SPSS, was used to derive an unrotated first principal component, representing global white matter, termed gFA. In addition, six long association tracts, which have previously been associated with variation in IQ (see Table S2 in the online supplement), were also subjected to a principal component analysis, for which the first unrotated principal component was again derived, termed LA-gFA. Calculation of these components followed an approach similar to that used in analyses carried out by Cox et al. (17) and Penke et al. (18). These studies reported that a latent factor explained a substantial portion of the variance in FA across all white matter tracts, whereby at an individual level, higher FA in a single tract predicted higher FA in all tracts. Generation of a single principal component for our analyses was designed to minimize the need to control for multiple comparisons across all white matter tracts. For each principal component analysis, we examined scree plots and the extraction values to determine whether tract FA values could be represented by a single latent factor. Comparable scree plots were observed for data across all sites for gFA (Figure 1A). The loadings of each white matter tract on the first principal component are presented in Supplement 2 (Excel file) in the online supplement. FA variance explained by the first unrotated component ranged from 44% to 70%, with a median of 56% (see Table S3 in the online supplement). Our second principal component analysis included FA of six long association tracts (LA-gFA) based on white matter tracts previously associated with IQ in the literature (19–26). The six tracts were the arcuate fasciculus, the anterior limb of the internal capsule, the superior longitudinal fasciculus, the uncinate fasciculus, the inferior fronto-occipital fasciculus, and the cingulate bundle. As with gFA, comparable scree plots were observed for data across all sites for LA-gFA (Figure 1B).
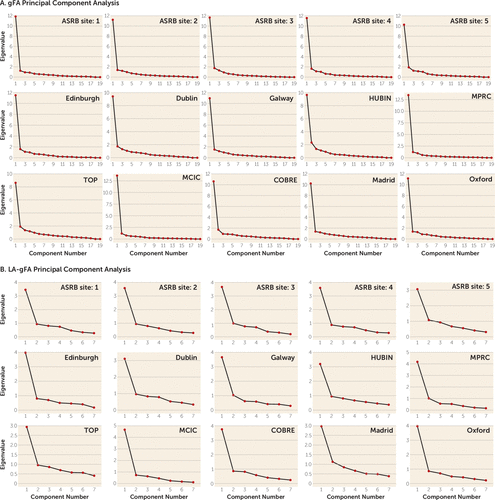
FIGURE 1. Scree plots for gFA and LA-gFA principal component analyses in a study of white matter microstructure and cognitive ability in schizophreniaa
a gFA=global fractional anisotropy component; LA-gFA=fractional anisotropy component for six long association tracts; ASRB=Australian Schizophrenia Research Bank; COBRE=Center for Biomedical Research Excellence; HUBIN=Human Brain Informatics; MCIC=MIND Clinical Imaging Consortium; MPRC=Maryland Psychiatric Research Center; TOP=Thematically Organized Psychosis (TOP) NORMENT Research Study.
The same principal component analysis method was used to derive both global and long association latent factors for sites that included secondary diffusion parameters. Secondary parameters included mean diffusivity, radial diffusivity, and axial diffusivity.
Per-site assessment of the variance in IQ explained by white matter microstructure.
To calculate the variance in IQ explained by white matter microstructure, a hierarchical regression analysis was carried out on a site-by-site basis (using SPSS, version 24). After controlling for age and gender, the change in r2 was used to estimate the variance in IQ explained by either gFA or LA-gFA. These regression analyses were carried out for both the full sample and for patients and control participants separately to allow determination of the effects of diagnosis.
Meta-Analysis
The Comprehensive Meta-Analysis software program (https://www.meta-analysis.com/) (statistical consultancy was not provided) was used locally to analyze summary data from all 11 contributing sites. The meta-analysis consisted of a two-level model comprising 1) a random-effects model estimating the average effect size by combining the observed effect sizes across all studies in the sample, and 2) a mixed-effects model incorporating diagnosis as a moderator variable to estimate the between-group variation and determine the effect of diagnosis on the observed association between white matter microstructure and IQ. A secondary analysis was carried out to determine the moderating effects of gender on the association between white matter tracts and IQ.
Results
Meta-Analysis
Demographic data (gender, age, and IQ) for the total ENIGMA samples of 957 healthy control participants and 760 patients with schizophrenia are provided in Table S4 in the online supplement. The mean age for the patient and control samples across all sites was 36 years (SD=9.1 and SD=10.1, respectively). With adolescent sites removed, the mean age for the patient and control samples was 39 years (SD=5.55 and SD=5.98, respectively). The patient group was 70% male (535 males, 225 females), and the control group was 56% male (539 males, 418 females) (χ2=4.2, p=0.04). The mean IQ was 97 (SD=16.47) across patient samples and 113 (SD=13.14) for the control participants (see Table S4). These values are somewhat higher than might be expected, especially for the patient group; a review of Table S4 suggests that this difference is due to the IQ of patients in the ASRB data set, which was the only data set to have a mean patient IQ >100.
Analysis of means and standard deviations for IQ across sites (see Table S4) shows that on average, patients had significantly lower IQ compared with the control participants (control group: mean=113, SD=5.82; patient group: mean=97, SD=8.24; t=5.94, df=27, p<0.001). The variance in IQ across sites was less for the control participants compared with the patients (variance for control group: mean=13.14, SD=2.85; for patients: mean=16.53, SD=3.31; t=−2.94, df=27, p<0.01). The differences in IQ and variance in IQ remained significant with exclusion of the ASRB data (IQ for control participants: mean=110, SD=6.02; for patients: mean=93, SD=6.89; t=5.85, df=27, p<0.001; variance in IQ for control group: mean=14.33, SD=2.65; for patients: mean=17.50, SD=3.41; t=−2.24, df=27, p<0.05).
DTI and IQ
The white matter tracts included in the gFA and LA-gFA principal component analyses are outlined in Table S2 in the online supplement. The scree plots from the principal component analysis for each site provided evidence for a strong single latent factor for both global FA (gFA) and the six long association tracts (LA-gFA) in each case (Figure 1). To determine the variances in IQ explained by global and long association white matter tracts, a regression analysis was carried out for gFA and LA-gFA separately, controlling for both age and gender, in patients and healthy control participants, on a site-by-site basis.
gFA Analyses
Meta-analytical results from the regression analysis for gFA showed that global white matter accounted for a significant amount of variance in IQ in the overall sample (3% variance, effect size [Hedges’ g]=0.27, 95% CI=0.17–0.36, p<0.001) (Figure 2; see also Table S5 in the online supplement).
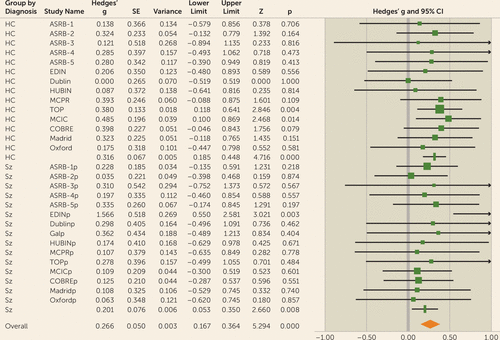
FIGURE 2. Forest plot for gFA meta-analysis in a study of white matter microstructure and cognitive ability in schizophreniaa
a There was no significant difference between the observed effect size in patients compared with control participants (χ2=1.3, p=0.26). Effect values indicated with a vertical line are Hedges’ g group summaries for patients and control groups separately; the diamond represents summary statistics for the full sample. gFA=global fractional anisotropy component; ASRB=Australian Schizophrenia Research Bank; COBRE=Center for Biomedical Research Excellence; EDIN=Edinburgh; HUBIN=Human Brain Informatics; MCIC=MIND Clinical Imaging Consortium; MPRC=Maryland Psychiatric Research Center; TOP=Thematically Organized Psychosis (TOP) NORMENT Research Study. In the lower half of the figure, the “p” appended to site names indicates the values relating to the patient cohort for each sample.
When considered separately, similar effects were observed in both the healthy control group (effect size=0.32, 95% CI=0.18–0.45, p<0.001) and the patient group (effect size=0.20, 95% CI=0.05–0.35, p<0.01) (Figure 3).
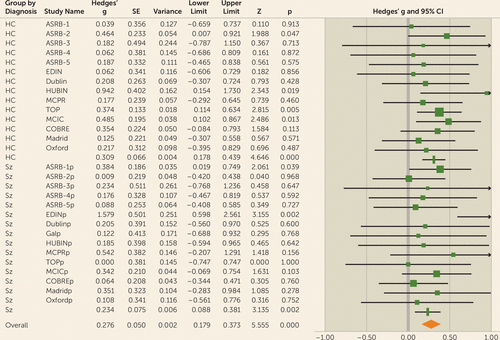
FIGURE 3. Forest plot for LA-gFA meta-analysis in a study of white matter microstructure and cognitive ability in schizophreniaa
a There was no significant difference between the observed effect size in patients compared with control participants (χ2=0.55, p=0.46). Effect values indicated with a vertical line are Hedges’ g group summaries for patients and control groups separately; the diamond represents summary statistics for the full sample. LA-gFA=fractional anisotropy component for six long association tracts; ASRB=Australian Schizophrenia Research Bank; COBRE=Center for Biomedical Research Excellence; EDIN=Edinburgh; HUBIN=Human Brain Informatics; MCIC=MIND Clinical Imaging Consortium; MPRC=Maryland Psychiatric Research Center; TOP=Thematically Organized Psychosis (TOP) NORMENT Research Study. In the lower half of the figure, the “p” appended to site names indicates the values relating to the patient cohort for each sample.
A between-group analysis was undertaken to estimate whether the strength of association between white matter and IQ was different in the patient group compared with the healthy control group. No effect of diagnosis was observed (mixed-model between-groups χ2=1.29, p=0.26), indicating that the amount of variance in IQ explained by gFA was comparable between these groups.
Given the differences in developmental stage of two samples that included adolescent participants (Oxford and Madrid) compared with the rest of the cohort, we reran the analysis excluding these two sites (control participants, N=120; patients, N=84). Removal of these adolescent data sets did not change the results of the meta-analysis, and comparable findings were obtained for the sample overall (effect size [Hedges’ g]=0.27, 95% CI=0.17–0.38, p<0.001), the control group (effect size=0.32, 95% CI=0.18–0.46, p<0.001), and the patient group (effect size=0.21, 95% CI=0.06–0.37, p<0.01). Furthermore, we observed higher IQ in the patient group across the ASRB sites. Again, removing this site from the analysis did not change the observed effect size for the control group (effect size=0.33, 95% CI=0.18–0.48, p<0.001) and the patient group (effect size=0.21, 95% CI=0.006–0.40, p<0.05).
Because of the variance in effect size across sites, a leave-one-out analysis was carried out to determine whether the observed results were driven by single sites. The leave-one-out cross-validation requires multiple iterations of the meta-analysis on all the data except the one site excluded per iteration (n−1). A meta-analysis was then carried out on the mean and standard deviation of the observed effect size for each iteration, with one study omitted for the analysis. The results of gFA leave-one-out analysis remained significant for each iteration, with a mean effect size of 0.25 (range=0.18–0.34) for the full sample, a mean effect size of 0.29 (range=0.26–0.34) for the control participants, and a mean effect size of 0.20 (range=0.18–0.22) for the patients (Figure 4A; see also Table S8 in the online supplement).
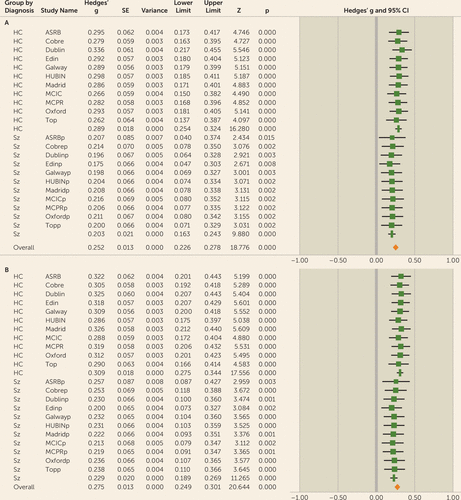
FIGURE 4. Leave-one-out meta-analysis for gFA and LA-gFA in a study of white matter microstructure and cognitive ability in schizophreniaa
a For both gFA and LA-gFA, 11 separate meta-analyses were carried out with n−1 site. The mean Hedges’ g effect size was taken for each meta-analysis with one site omitted for each iteration. The study name corresponds to the results when this site was omitted from the analysis. The association between both gFA and LA-gFA with IQ remained significant for each iteration, indicating that the results are not driven by a specific site. gFA=global fractional anisotropy component; LA-gFA=fractional anisotropy component for six long association tracts; ASRB=Australian Schizophrenia Research Bank; COBRE=Center for Biomedical Research Excellence; EDIN=Edinburgh; HUBIN=Human Brain Informatics; MCIC=MIND Clinical Imaging Consortium; MPRC=Maryland Psychiatric Research Center; TOP=Thematically Organized Psychosis (TOP) NORMENT Research Study. In the lower half of each panel, the “p” appended to site names indicates the values relating to the patient cohort for each sample.
LA-gFA Analyses
To test specifically for a relationship between cognition and long association fiber tracts previously hypothesized in the literature to be involved in cognitive performance, a single latent FA factor was generated for the six long association tracts identified above (LA-gFA). Similarly to global white matter microstructure, LA-gFA accounted for a significant amount of variance in IQ in the full sample (3.5% variance, effect size=0.28, 95% CI=0.18–0.37, p<0.001) (Figure 3). This significant effect for LA-gFA was also observed separately in the control group (effect size=0.31, 95% CI=0.18–0.44, p<0.001) and the patient group (effect size=0.23, 95% CI=0.09–0.38, p<0.01). The meta-analytic results for LA-gFA are outlined in Table S6 in the online supplement. The between-sample LA-gFA meta-analysis results again indicate that there was no significant difference in the observed effect size between the control and patient groups (χ2=0.55, p=0.46). As with gFA, these results did not change after removing adolescent populations (overall sample: effect size [Hedges’ g]=0.29, 95% CI=0.18–0.40; control group: effect size=0.33, 95% CI=0.19–0.47; patient group, effect size=0.23, 95% CI=0.08–0.39), with no observed effect of diagnosis (χ2=0.85, p=0.36). Similarly, removal of the ASRB data did not significantly change the effect size for both the control group (effect size=0.32, 95% CI=0.18–0.47, p<0.001) and the patient group (effect size=0.27, 95% CI=0.07–0.47, p<0.01), with no significant effect of diagnosis (χ2=0.18, p=0.68). The comparable findings between gFA and LA-gFA are perhaps not surprising given the strong positive correlation between these components (see Table S7 in the online supplement). Given this, to determine whether the effects observed for gFA were driven by long association tracts, we recalculated the gFA component to exclude the six long association tracts on which the LA-gFA was based. The results obtained were largely unchanged, both for the whole group analysis (3% variance, effect size=0.29, 95% CI=0.19–0.39, p<0.001) and separately for the control group (effect size=0.30, 95% CI=0.17–0.44, p<0.001) and the patient group (effect size=0.27, 95% CI=0.13–0.42, p<0.001). Finally, as in the gFA analyses, a leave-one-out analysis was also undertaken. Here again, the results remained unchanged (full sample: mean effect size=0.27, range=0.21–0.33; control group: effect size=0.31, range=0.29–0.33; patient group: effect size=0.23, range=0.21–0.27) (Figure 4B; see also Table S9 in the online supplement).
Association Between gFA/LA-gFA and IQ in Males and Females
To determine the effects of gender on the relationship between white matter and IQ, a further meta-analysis was carried out. Similar results were observed between males and females in gFA (males: effect size [Hedges’ g]=0.36, 95% CI=0.23–0.48, p<0.001; females: effect size=0.39, 95% CI=0.22–0.55, p<0.001), with no significant difference (χ2=0.088, p=0.77) (see Figure S1A and Table S10 in the online supplement). For gFA, female patients had the largest observed effect size, although this was not significantly different compared with male patients (female patients: effect size=0.45, 95% CI=0.25–0.65, p<0.001; male control participants: effect size=0.25, 95% CI=0.07–0.43, p<0.01; χ2=2.14, p=0.14). Similarly, there was no effect of gender in the healthy control sample (female control participants: effect size=0.27, 95% CI=0.06–0.48, p<0.05; male control participants: effect size=0.39, 95% CI=0.20–0.47, p<0.001; χ2=0.68, p=0.14).
Likewise for LA-gFA, there was no significant difference in the observed effect sizes for males and females (males: effect size=0.33, 95% CI=0.21–0.46, p<0.001; females: effect size=0.37, 95% CI=0.20–0.53, p<0.001) (see Figure S1B and Table S11 in the online supplement). Female patients had the largest observed effect size for LA-gFA, although this was not significantly different compared with male patients (female patients: effect size=0.39, 95% CI=0.10–0.68, p<0.01; male healthy participants: effect size=0.19, 95% CI=0.01–0.37, p<0.01; χ2=1.35, p=0.26). Similarly, there was no effect of gender in the healthy control sample (female healthy participants: effect size=0.31, 95% CI=0.10–0.52, p<0.01; male healthy participants: effect size=0.38, 95% CI=0.20–0.57, p<0.001; χ2=0.27, p=0.60).
Association Between Diffusion MRI Secondary Parameters and IQ
Secondary diffusion MRI parameters were available for a subset of sites (ASRB, Edinburgh, Dublin, Human Brain Informatics, MPRC, Galway) that included 397 healthy control participants and 467 patients with schizophrenia. Meta-analysis, reported in Table S12 in the online supplement, shows that radial diffusivity (RD) had the largest effect size for both global (gRD) and long association tracts (LA-gRD) across the full sample (gRD: effect size=0.33, 95% CI=0.13–0.52, p=0.001; LA-gRD: effect size=0.34, 95% CI=0.08–0.0.52, p=0.01). Standardized beta coefficients from this analysis are reported in Table S13 in the online supplement.
Discussion
In this study, we sought to characterize the relationship between white matter microstructure and IQ and to compare this association between patients with schizophrenia and healthy control participants. We carried out a meta-analysis of data sets from participating ENIGMA groups, analyzed according to a common analysis pipeline, to assess the relationship between white matter microstructure and IQ in patients with schizophrenia and healthy participants. Our findings indicated that global white matter microstructure accounted for a significant amount of variation in IQ (effect size=0.27), both in patients with schizophrenia and in healthy participants, with the size of the association observed to be comparable between groups. Comparable results were obtained with either global white matter values (effect size=0.27) or six regional association white matter tracts (effect size=0.28) that connect frontal, parietal, and temporal lobes and have previously been hypothesized to be involved in IQ. These findings were unequivocal, supporting the value of meta-analysis based on harmonized pipelines and large data sets.
The results of this ENIGMA meta-analysis consistently showed a pattern of significant (albeit modest) associations between white matter FA and IQ in both patients and healthy participants. Robust evidence of association between variation in IQ and variation in white matter structural connectivity was found, with similar effect sizes observed in patients and healthy participants. A landmark review carried out by Deary and Caryl (24) suggested that intelligence is regulated by a widely distributed complex neurological network. Our study provides empirical evidence for this claim in the largest IQ study undertaken to date, consistent with previous studies linking white matter microstructure to processing speed (a cognitive variable highly correlated with IQ) (18, 22, 27). Collectively, these results indicate that global white matter provides a neural network to support the functional cortical communication required for general cognitive performance. The similar effect sizes observed in both global and long association fiber–based measures of FA underline the nature of this relationship as a global phenomenon rather than a regionally specific association.
Previous studies focused mainly on individual white matter tracts, including fronto-parietal white matter (22, 28), the cingulate (21, 29, 30), the uncinate fasciculus (20), the fornix (19), and the corpus callosum (31). A significant positive correlation between IQ in fronto-parietal white matter (28), the uncinate fasciculus (20), and the cingulate bundle (21, 30) was frequently observed in schizophrenia, with negative or no significant associations reported in healthy participants. The heterogeneity of results between studies was most likely due to small sample sizes and methodological differences that limited replicability of results (19–22, 28–32). In this study, we have overcome the limitation of small sample sizes by using a harmonized DTI processing pipeline and statistical analysis on over 1,700 participants. We also concluded that our findings were not driven by any single site (based on the leave-one-out analyses for both gFA and LA-gFA) and can therefore be expected to generalize to independent samples. In addition, these findings did not appear to be explained by the higher proportion of males in the total sample, as we have shown that the association between white matter microstructure and IQ is not significantly different between males and females.
The Relationship Between White Matter Microstructure and IQ in Patients and Healthy Participants
Our results indicate that the relationship between structural connectivity and higher cognitive function is broadly comparable between patient and healthy groups, with no differences in effect sizes observed. We previously reported (6) widespread white matter deficits in schizophrenia that spanned 19 white matter tracts and hypothesized that in schizophrenia these may predict variation in cognition more strongly in patients than control subjects, as suggested by previous small-scale studies (20–22, 28, 30). By contrast, however, we observed similar effects between patients and healthy participants. This finding suggests that individual variances of low to high efficiency in transfer of information across white matter tracts is associated with a range of lower to higher cognitive functioning, irrespective of diagnosis. Therefore, patients with schizophrenia occupied the lower quadrant of the correlation matrix between white matter microstructure and IQ. We speculate, based on these results, that a common neurodevelopmental process and cytoarchitecture predicts outcomes in cognitive performance, independent of a clinical diagnosis. However, further studies of the genetic and neurodevelopment associations between schizophrenia, white matter, and measures of cognitive ability will be needed to address these questions.
Patients in the ASRB data set had an average IQ score of 105. Generally, patients with schizophrenia consistently demonstrate a medium-sized impairment in IQ (33), with an 8-point IQ deficit observed in the premorbid stage (33) and a deficit of 14–21 points among those with first-episode and chronic schizophrenia (34–36). Furthermore, lower IQ is associated with increased risk of schizophrenia (37). To determine whether the ASRB patient IQ (above average norms) was confounding any diagnostic effect, the analysis was rerun without these data. The effect size observed for the patient group, for both gFA and LA-gFA, did not significantly change, supporting the hypothesis that the association between white matter and IQ is independent of diagnosis.
Kelly et al. (6) reported that patients with schizophrenia had significantly higher mean diffusivity and radial diffusivity across the majority of white matter tracts. Higher radial diffusivity, which underlies changes in fractional anisotropy, is indicative of microstructural alterations. Specifically, it provides an index of diffusion in an orientation perpendicular to a white matter tract. Our findings indicate both higher FA and, related to this, lower RD are associated with higher cognitive function in both patients and control participants. Similar effect sizes were observed for the association between radial diffusivity and IQ in both patients and control participants. While the precise biological interpretation of changes in radial diffusivity must be done cautiously, previous studies have speculated that radial diffusivity is associated with demyelination (38). Increased radial diffusivity associated with demyelination supports our finding that efficient global structural connectivity facilitates higher cognitive function, independent of diagnosis.
Strengths and Limitations
In this study, we adopted a “prospective” meta-analytic approach that analyzed the relationship between IQ and DTI based on a well-validated and harmonized ENIGMA DTI analysis pipeline carried out in a large sample of 1,717 participants. Doing so overcomes many of the significant limitations of previous studies by minimizing sources of heterogeneity and the potential for consequent false positive or negative findings. However, future analyses could also include methods to incorporate harmonized measures of environmental factors, such as educational level, socioeconomic status, general health, and lifestyle, which may have an impact on cognitive outcomes. ENIGMA DTI pipelines incorporate tract-based spatial statistics (14), which is a widely used method for voxel-based analysis of white matter tracts. Although tensor-based limitations have been widely reported—i.e., the model does not capture all information on white matter microstructure, such as myelination, axonal packing density, or neuroinflammation—DTI remains the most consistently used method in diffusion MRI analysis, and pending a general consensus on non-tensor-based processing methods that are devoid of potential artifacts, the analysis carried out here is the most advanced that definitively supports the structural underpinnings of cognitive performance. During image preprocessing, DTI data were corrected for motion-induced artifacts; however, studies have shown that some white matter tracts may be more sensitive to microscopic head movements, which may produce spurious group differences (39). To overcome motion-induced variances, previous single-site studies have included a metric of motion as a covariate in the analysis (39, 40). Further development of these methods would be required for implementation in multisite analyses in large consortium-based data sets.
We used a principal component analysis to derive components for global and long association tract FA values. While this eliminates the need for correction for multiple comparisons, it reduces the ability to detect a possible, albeit unlikely, association between cognition and specific individual white matter tracts. Previous studies used similar principal component analyses to assess the relationship between global neural underpinnings of functional measures (17, 41).
While we have unequivocally shown here a relationship between white matter and cognitive ability, further studies are necessary to determine the associations with gray matter measures. Although this has been more widely studied, study sample sizes have still been limited, and methodological issues make it difficult to summarize the findings. The latest ENIGMA study (Grasby et al., under review) shows a strong overlap between the genetic influences on cortical surface area and educational attainment. Analyses similar to that carried out here may help identify cortical regions associated with cognitive deficits observed in schizophrenia. Finally, further analysis is also required to determine whether the associations reported here generalize to other psychiatric disorders, although the comparability between patients and healthy participants observed here suggests that this is likely.
Conclusions
This study provides robust evidence that cognitive ability is associated with global structural connectivity and that more efficient white matter microstructure is associated with higher IQ. This association was independent of diagnosis: across the distribution of scores on FA and IQ measures, patients tended to have lower FA and lower IQ, healthy participants tended to have higher FA and higher IQ, and the effect sizes of these associations between FA and IQ were comparable between groups. These findings suggest that a general association between lower FA and lower IQ is likely, with white matter microstructure likely to represent a significant component in the neural basis of IQ.
1 : Global, regional, and national incidence, prevalence, and years lived with disability for 328 diseases and injuries for 195 countries, 1990–2016: a systematic analysis for the Global Burden of Disease Study 2016. Lancet 2017; 390:1211–1259Crossref, Medline, Google Scholar
2 : Impact of cognitive and social cognitive impairment on functional outcomes in patients with schizophrenia. J Clin Psychiatry 2016; 77(suppl 2):8–11Crossref, Medline, Google Scholar
3 : Neurocognitive deficits and functional outcome in schizophrenia: are we measuring the “right stuff”? Schizophr Bull 2000; 26:119–136Crossref, Medline, Google Scholar
4 : What are the functional consequences of neurocognitive deficits in schizophrenia? Am J Psychiatry 1996; 153:321–330Link, Google Scholar
5 : Cognitive deficits and functional outcome in schizophrenia. Neuropsychiatr Dis Treat 2006; 2:531–536Crossref, Medline, Google Scholar
6 : Widespread white matter microstructural differences in schizophrenia across 4322 individuals: results from the ENIGMA Schizophrenia DTI Working Group. Mol Psychiatry 2018; 23:1261–1269Crossref, Medline, Google Scholar
7 : Cortical brain abnormalities in 4474 individuals with schizophrenia and 5098 control subjects via the Enhancing Neuro Imaging Genetics Through Meta Analysis (ENIGMA) Consortium. Biol Psychiatry 2018; 84:644–654Crossref, Medline, Google Scholar
8 : Meta-analysis of regional brain volumes in schizophrenia. Am J Psychiatry 2000; 157:16–25Link, Google Scholar
9 : Schizophrenia: a disconnection syndrome? Clin Neurosci 1995; 3:89–97Medline, Google Scholar
10 : A connectionist approach to the prefrontal cortex. J Neuropsychiatry Clin Neurosci 1993; 5:241–253Crossref, Medline, Google Scholar
11 : Aberrant frontal and temporal complex network structure in schizophrenia: a graph theoretical analysis. J Neurosci 2010; 30:15915–15926Crossref, Medline, Google Scholar
12 : Disrupted axonal fiber connectivity in schizophrenia. Biol Psychiatry 2011; 69:80–89Crossref, Medline, Google Scholar
13 : Molecular genetic evidence for overlap between general cognitive ability and risk for schizophrenia: a report from the Cognitive Genomics Consortium (COGENT). Mol Psychiatry 2014; 19:168–174Crossref, Medline, Google Scholar
14 : Tract-based spatial statistics: voxelwise analysis of multi-subject diffusion data. Neuroimage 2006; 31:1487–1505Crossref, Medline, Google Scholar
15 : Identical, but not the same: intra-site and inter-site reproducibility of fractional anisotropy measures on two 3.0T scanners. Neuroimage 2010; 51:1384–1394Crossref, Medline, Google Scholar
16 : Twenty-five pitfalls in the analysis of diffusion MRI data. NMR Biomed 2010; 23:803–820Crossref, Medline, Google Scholar
17 : Ageing and brain white matter structure in 3,513 UK Biobank participants. Nat Commun 2016; 7:13629Crossref, Medline, Google Scholar
18 : A general factor of brain white matter integrity predicts information processing speed in healthy older people. J Neurosci 2010; 30:7569–7574Crossref, Medline, Google Scholar
19 : A diffusion tensor imaging family study of the fornix in schizophrenia. Schizophr Res 2014; 159:435–440Crossref, Medline, Google Scholar
20 : Neuropsychological correlates of diffusion tensor imaging in schizophrenia. Neuropsychology 2004; 18:629–637Crossref, Medline, Google Scholar
21 : Comparing prefrontal gray and white matter contributions to intelligence and decision making in schizophrenia and healthy controls. Neuropsychology 2010; 24:121–129Crossref, Medline, Google Scholar
22 : Fronto-parietal white matter microstructural deficits are linked to performance IQ in a first-episode schizophrenia Han Chinese sample. Psychol Med 2013; 43:2047–2056Crossref, Medline, Google Scholar
23 : Brain white matter tract integrity and cognitive abilities in community-dwelling older people: the Lothian Birth Cohort, 1936. Neuropsychology 2013; 27:595–607Crossref, Medline, Google Scholar
24 : Neuroscience and human intelligence differences. Trends Neurosci 1997; 20:365–371Crossref, Medline, Google Scholar
25 : Development of white matter and reading skills. Proc Natl Acad Sci USA 2012; 109:E3045–E3053Crossref, Medline, Google Scholar
26 : Diffusion tensor imaging of the superior longitudinal fasciculus and working memory in recent-onset schizophrenia. Biol Psychiatry 2008; 63:512–518Crossref, Medline, Google Scholar
27 : Brain-wide white matter tract integrity is associated with information processing speed and general intelligence. Mol Psychiatry 2012; 17:955Crossref, Medline, Google Scholar
28 : Cigarette smoking and white matter microstructure in schizophrenia. Psychiatry Res 2012; 201:152–158Crossref, Medline, Google Scholar
29 : Abnormal asymmetry of white matter tracts between ventral posterior cingulate cortex and middle temporal gyrus in recent-onset schizophrenia. Schizophr Res 2018; 192:159–166Crossref, Medline, Google Scholar
30 : Neuropsychological variability, symptoms, and brain imaging in chronic schizophrenia. Brain Imaging Behav 2013; 7:68–76Crossref, Medline, Google Scholar
31 : Copy number deletion burden is associated with cognitive, structural, and resting-state network differences in patients with schizophrenia. Behav Brain Res 2014; 272:324–334Crossref, Medline, Google Scholar
32 : Similar white matter but opposite grey matter changes in schizophrenia and high-functioning autism. Acta Psychiatr Scand 2016; 134:31–39Crossref, Medline, Google Scholar
33 : Premorbid IQ in schizophrenia: a meta-analytic review. Am J Psychiatry 2008; 165:579–587Link, Google Scholar
34 : Neuropsychological impairments in schizophrenia: integration of performance-based and brain imaging findings. Psychol Bull 2007; 133:833–858Crossref, Medline, Google Scholar
35 : Neurocognitive deficit in schizophrenia: a quantitative review of the evidence. Neuropsychology 1998; 12:426–445Crossref, Medline, Google Scholar
36 : Neurocognition in first-episode schizophrenia: a meta-analytic review. Neuropsychology 2009; 23:315–336Crossref, Medline, Google Scholar
37 : A longitudinal study of premorbid IQ score and risk of developing schizophrenia, bipolar disorder, severe depression, and other nonaffective psychoses. Arch Gen Psychiatry 2004; 61:354–360Crossref, Medline, Google Scholar
38 : Dysmyelination revealed through MRI as increased radial (but unchanged axial) diffusion of water. Neuroimage 2002; 17:1429–1436Crossref, Medline, Google Scholar
39 : Spurious group differences due to head motion in a diffusion MRI study. Neuroimage 2014; 88:79–90Crossref, Medline, Google Scholar
40 : The impact of quality assurance assessment on diffusion tensor imaging outcomes in a large-scale population-based cohort. Neuroimage 2016; 125:903–919Crossref, Medline, Google Scholar
41 : Brain white matter tract integrity as a neural foundation for general intelligence. Mol Psychiatry 2012; 17:1026–1030Crossref, Medline, Google Scholar