Evidence for Dissociable Linkage of Dimensions of Psychopathology to Brain Structure in Youths
Abstract
Objective:
High comorbidity among psychiatric disorders suggests that they may share underlying neurobiological deficits. Abnormalities in cortical thickness and volume have been demonstrated in clinical samples of adults, but less is known when these structural differences emerge in youths. The purpose of this study was to examine the association between dimensions of psychopathology and brain structure.
Methods:
The authors studied 1,394 youths who underwent brain imaging as part of the Philadelphia Neurodevelopmental Cohort. Dimensions of psychopathology were constructed using a bifactor model of symptoms. Cortical thickness and volume were quantified using high-resolution 3-T MRI. Structural covariance networks were derived using nonnegative matrix factorization and analyzed using generalized additive models with penalized splines to capture both linear and nonlinear age-related effects.
Results:
Fear symptoms were associated with reduced cortical thickness in most networks, and overall psychopathology was associated with globally reduced gray matter volume across all networks. Structural covariance networks predicted psychopathology symptoms above and beyond demographic characteristics and cognitive performance.
Conclusions:
The results suggest a dissociable relationship whereby fear is most strongly linked to reduced cortical thickness and overall psychopathology is most strongly linked to global reductions in gray matter volume. Such results have implications for understanding how abnormalities of brain development may be associated with divergent dimensions of psychopathology.
Psychiatric disorders have high rates of comorbidity, with many symptoms being continuous, showing nonspecificity, cutting across disorders, and being hierarchically arranged (1–3). Additionally, there is substantial heterogeneity within psychiatric disorders (1, 3). It is increasingly recognized that such clinical comorbidity is mirrored by neurobiological nonspecificity, with similar abnormalities of brain structure being described in multiple disorders. For example, abnormalities in cortical thickness have been reported in anxiety, depression, psychosis, and behavioral disorders (4–7). Likewise, a meta-analysis by Goodkind et al. (8) reported common gray matter volume loss in the dorsal anterior cingulate and insula across disorders as varied as schizophrenia, bipolar disorder, major depressive disorder, addiction, obsessive-compulsive disorder, and anxiety. Genetic studies suggest that while cortical thickness influences volume measurements of cortical gray matter, these two measures can provide unique information (9).
However, within this broad literature, several current limitations are notable. First, the specific effects of each structural neuroimaging study are quite heterogeneous. Inconsistent results may be due to small sample sizes and selective reporting of regions of interest. Second, most studies are restricted to adults. This is an important caveat, as most psychiatric disorders first manifest during childhood, adolescence, or young adulthood (10, 11), suggesting that large studies of brain structure in youths are needed.
Third and perhaps most important, studies have usually employed a case-control approach with restrictive inclusion criteria, limiting comorbidity and failing to take into account the dimensional nature of psychopathology. Previous factor analytic work has commonly revealed four dimensions of psychiatric symptoms: anxious-misery/distress, psychosis, behavioral/externalizing symptoms, and fear (1, 3, 12). However, dimensions derived from traditional factor analytic models are often highly correlated with each other, suggesting the importance of considering the overall burden of psychopathology in an individual. Akin to the overall “g” intelligence factor in cognition research, the “p” psychopathology factor quantifies the overall level of psychopathology present across clinical domains (2, 3). This p factor can be measured using bifactor models, which yield both the p factor and orthogonal factors for each specific dimension. Previous work has related higher p factor scores with reduced gray matter volume in adults (13) and children (14); further studies are needed to evaluate the relationship between p and diverse measures of brain structure in youths.
In response to these gaps in the field, we investigated associations between brain structure and psychopathology using a sample of 1,394 youths who underwent brain imaging as part of the Philadelphia Neurodevelopmental Cohort (PNC), a large-scale community-based study of brain development (15, 16). We quantified psychopathology dimensions using a bifactor analysis of dimensional clinician ratings that were assessed for every individual. We then delineated structural covariance networks using nonnegative matrix factorization (NMF), a multivariate analysis technique developed in the context of computer vision research that has recently been adapted for neuroimaging data (17). Using these networks, we investigated how abnormalities of two different measures of brain structure (cortical thickness and volume) were associated with each dimension of psychopathology.
Methods
Participants
A total of 1,601 participants completed multimodal neuroimaging as part of the PNC (15, 16). The institutional review boards of the University of Pennsylvania and the Children’s Hospital of Philadelphia approved the study procedures. Participants over age 18 provided written informed consent after receiving a complete description of the study, and we obtained assent and parental consent for minors. The final sample consisted of 1,394 youths; the demographic characteristics of the sample are summarized in Table 1 (see the online supplement for details). In this final sample, 155 participants (11%) were taking psychotropic medications at the time of imaging and were evaluated in sensitivity analyses, as described below.
Measure | ||
---|---|---|
Mean | SD | |
Age (years) | 14.98 | 3.64 |
N | % | |
Male | 663 | 48 |
Caucasian | 618 | 44 |
Maternal education level | ||
≤12 years | 509 | 37 |
>12 years | 868 | 62 |
Missing data | 17 | 1 |
Lifetime prevalencea | ||
Typically developing | 428 | 31 |
ADHD | 230 | 16 |
Agoraphobia | 81 | 6 |
Anorexia nervosa | 16 | 1 |
Bulimia nervosa | 5 | <1 |
Conduct disorder | 121 | 9 |
Generalized anxiety disorder | 27 | 2 |
Major depressive disorder | 193 | 14 |
Mania | 16 | 1 |
Obsessive-compulsive disorder | 43 | 3 |
Oppositional defiant disorder | 458 | 33 |
Panic | 14 | 1 |
Psychosis spectrum disorder | 399 | 29 |
Posttraumatic stress disorder | 172 | 12 |
Separation anxiety | 63 | 5 |
Social anxiety | 328 | 24 |
Specific phobia | 426 | 31 |
TABLE 1. Summary of demographic and clinical data in a study of psychopathology and brain structure in youths (N=1,394)
Clinical and Cognitive Assessment
As described previously (15, 16), psychiatric symptoms were assessed using a structured screening interview (GOASSESS) based on a modified version of the Kiddie Schedule for Affective Disorders and Schizophrenia (see the online supplement). The lifetime prevalence of each disorder is summarized in Table 1. An exploratory factor analysis of 112 item-level symptoms (see the online supplement) identified four correlated dimensions of psychopathology: anxious-misery, psychosis, behavioral, and fear (Figure 1A), which show a high degree of overlap across dimensions and diagnostic screening categories. To increase specificity, we then used a confirmatory item bifactor analysis, which yielded five orthogonal dimensions of psychopathology: anxious-misery, psychosis, behavioral/externalizing, fear, and overall psychopathology (symptom burden across all psychiatric disorders; see Figure 1B and 1C). Additionally, three cognitive factors (executive function/complex reasoning, social cognition, and episodic memory) derived from factor analysis (see the online supplement) were included as predictors in the multivariate analyses described below.
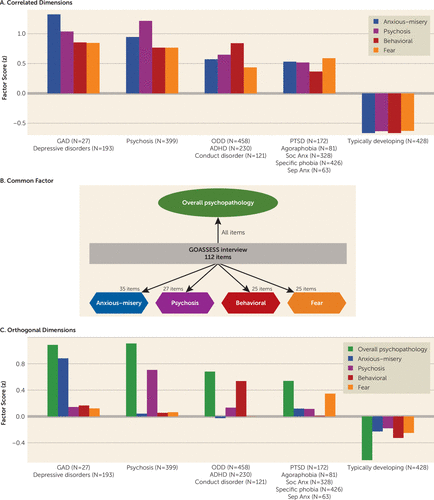
FIGURE 1. High degree of overlapping symptoms shown in correlated dimensions of psychopathology in a study of psychopathology and brain structure in youthsa
a In panel A, an exploratory factor analysis of 112 psychiatric symptoms identified four correlated dimensions of psychopathology: anxious-misery, psychosis, behavioral, and fear, which show a high degree of overlap across dimensions and diagnostic screening categories. The graphs show the mean factor scores for each of the four dimensions in the related screening diagnoses. Panel B illustrates a confirmatory bifactor analysis in which we constrained the dimensions of psychopathology (anxious-misery, psychosis, behavioral, and fear) to be orthogonal and extracted a common factor (overall psychopathology). In panel C, the orthogonal factors derived from the bifactor analysis load more specifically onto the relevant disorders. GAD=generalized anxiety disorder; ODD=oppositional defiant disorder; ADHD=attention deficit hyperactivity disorder; PTSD=posttraumatic stress disorder; Soc Anx=social anxiety disorder; Sep Anx=separation anxiety disorder.
Image Acquisition, Quality Assurance, and Processing
Image acquisition and processing have been reported in detail elsewhere (15). Briefly, imaging data were acquired on the same MRI scanner using the same imaging sequences for all participants (see the online supplement). Three highly trained image analysts independently assessed structural image quality control using manually derived ratings (18) (see the online supplement), and the average quality rating across the three raters was included as a covariate in all models to control for the confounding influence of variation in image quality.
Structural image processing utilized Advanced Normalization Tools (ANTs; see the online supplement). Previous large-scale evaluation studies have shown that this procedure is highly accurate and more sensitive to individual differences over the lifespan than comparable techniques. To avoid registration bias and maximize sensitivity, a custom adolescent template and tissue priors were used. This procedure yielded two maps for each subject: a cortical thickness image and a volume image (log transformed determinant of the Jacobian of the deformation field).
Nonnegative Matrix Factorization
We used nonnegative matrix factorization (NMF) to identify networks where brain structure covaried consistently across participants (17). Details regarding the implementation of NMF have been presented elsewhere (17, 19) and in the online supplement (see Figure S1 for a schematic of the NMF procedure). NMF yields networks that are highly interpretable and have improved statistical power compared with standard mass univariate analyses (see the online supplement for details).
NMF networks were calculated from cortical thickness maps; to allow for correspondence across image types, the loadings for these networks were then applied to the volume maps, resulting in only cortical regions being analyzed. Results were similar when networks were calculated directly from volume maps. NMF networks were visualized on the inflated Population-Average, Landmark-, and Surface-Based (PALS) cortical surfaces using the Caret software package.
Group-Level Statistical Analyses
After identifying networks, we conducted analyses to identify associations between brain structure and dimensions of psychopathology. Given that structural maturation is a nonlinear process, we modeled both linear and nonlinear age effects using penalized splines within generalized additive models using the R package voxel, which relies on the mgcv package in R (20). Generalized additive models assess a penalty on nonlinearity to avoid overfitting and to capture both linear and nonlinear effects in a data-driven fashion. All models used the restricted maximum likelihood framework, which produces estimates of variance and covariance parameters. Based on previous work documenting sex differences in cortical thickness and volume (21), we included sex in the model. In addition, we added mean image quality ratings (described above) as an additional model covariate to ensure that image quality did not drive the observed associations (18). For each network, we examined associations between each dimension of psychopathology and cortical thickness or volume, using the following formula: NMF structural network = spline(age) + sex + image quality rating + anxious-misery + psychosis + behavioral + fear + overall psychopathology.
Interactions between fear and age, fear and sex, and age and sex were evaluated and found to be nonsignificant for cortical thickness. The same interactions for overall psychopathology and anxious-misery were nonsignificant for volume; significant age-by-sex interactions are reported below. To control for multiple testing across networks, we controlled the false discovery rate (q<0.05).
Sensitivity Analyses
We conducted sensitivity analyses to ensure that our results were robust to methodological choices and were not influenced by confounding variables. First, we repeated the analyses described above using standard anatomical regions. Anatomical regions were delineated using a top-performing, highly accurate multi-atlas labeling tool with joint label fusion implemented in ANTs (see the online supplement). Second, we included maternal education level as an additional covariate and excluded the participants who were taking psychotropic medications at the time of imaging (N=155, 11%). Third, we evaluated whether global differences in total gray matter volume or average cortical thickness were driving the observed effects. Fourth, we used a traditional case-control approach to illustrate the usefulness of the bifactor model in increasing the specificity of the results by taking into account the overlapping variance between disorders.
NMF Networks as Predictors of Psychopathology
In addition to univariate associations between each structural covariance network and each dimension of psychopathology, we also conducted a multivariate analysis to examine whether structural networks predicted psychopathology above and beyond demographic characteristics and cognitive performance. To do this, we compared a reduced model including age, sex, and three cognitive performance factors to a full model predicting fear with age, sex, cognitive factors, and all 18 cortical thickness networks using an F test. Similarly, we also performed the same analyses with the volume networks as predictors of overall psychopathology and anxious-misery. Adjusted R2 is reported for the models.
Data and Code Availability
For the code and a companion wiki detailing the analytic procedures used in this study, see https://github.com/PennBBL/KaczkurkinPark_BifactorStructure/wiki/ KaczkurkinPark_BifactorStructure. Data from the PNC can be accessed at https://www.ncbi.nlm.nih.gov/projects/gap/cgi-bin/study.cgi?study_id=phs000607.v3.p2. The NMF code can be found at https://github.com/asotiras/brainlets.
Results
NMF Identifies Structural Covariance Networks
Structural covariance networks were delineated using NMF at multiple resolutions. The final 18-network solution was chosen on the basis of two considerations. First, we evaluated the gradient of reconstruction error (see Figure S2 in the online supplement), which shows only nominal decrements in error beyond 14 networks. Second, we checked the split-half reliability at this resolution, which revealed an adjusted Rand index of 0.93 for the 18-network solution, suggesting that this solution is highly reproducible. This resolution is also consistent with previous reports (19). Accordingly, the 18-network solution was used for all subsequent analyses. As in previous work using NMF (17, 19), the structural covariance networks identified were highly symmetric bilaterally (Figure 2).

FIGURE 2. Structural covariance networks delineated by nonnegative matrix factorization in a study of psychopathology and brain structure in youthsa
a Structural covariance networks are shown for the 18-network solution, with the spatial distribution of each network indicated by loadings at each voxel in arbitrary units (shown with the color bar, where warmer colors correspond to higher values). High symmetry can be seen between the left (L) and right (R) hemispheres. The anatomical coverage of each structural covariance network was as follows: 1) cingulate cortex; 2) medial temporal cortex; 3) temporal pole; 4) dorsolateral prefrontal cortex; 5) posterior cingulate cortex; 6) superior parietal cortex; 7) superior temporal cortex; 8) dorsal prefrontal cortex; 9) insular cortex; 10) fusiform cortex; 11) inferior temporal cortex; 12) right lateral occipital cortex; 13) subgenual cingulate, anterior cingulate, and anterior insula; 14) inferior prefrontal cortex; 15) inferior parietal cortex; 16a) precuneus and 16b) temporoparietal junction; 17) lingual gyrus; and 18) medial occipital cortex.
Psychopathology Dimensions Are Associated With Structural Differences in Multiple Networks
Having identified 18 cortical thickness covariance networks using NMF, we next examined associations with the dimensions of psychopathology summarized by the bifactor analysis. Results revealed that increased fear was associated with reduced cortical thickness in 13 networks after false discovery rate correction (Figure 3; see also Table S1 in the online supplement), with the relationship between fear and cortical thickness showing small effect sizes (partial r values ≤−0.12). Regions affected included the posterior cingulate and temporal-parietal junction (network 16, see Figure 3A), the anterior and subgenual cingulate cortex, and the anterior insula (network 13, see Figure 3C). Furthermore, there were relatively widespread associations across the temporal, orbitofrontal, and occipital cortex. Notably, the association between fear and cortical thickness was specific, and cortical thickness was only weakly associated with other dimensions of psychopathology. The anxious-misery and behavioral dimensions were not associated with cortical thickness in any network, whereas psychosis and overall psychopathology were associated with diminished thickness in only a single network (networks 5 and 16, respectively). However, the results for psychosis and overall psychopathology did not remain significant during sensitivity analyses (described below). Interactions between fear and age, fear and sex, and age and sex were nonsignificant for cortical thickness.
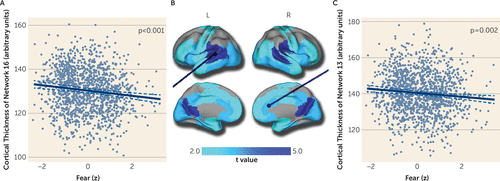
FIGURE 3. Association of fear with reduced cortical thickness in multiple structural covariance networks in a study of psychopathology and brain structure in youthsa
a Mass univariate analyses using generalized additive models that controlled for linear and nonlinear age, sex, and image quality revealed that fear symptoms were associated with reduced cortical thickness in multiple networks. This association was maximal in networks such as the temporal-parietal junction and posterior cingulate cortex (panel A; network 16). Significant associations were also present in networks that included the anterior cingulate, anterior insula, and subgenual cingulate cortex (panel C; network 13). Composite network boundaries were obtained by assigning each voxel to one of the 18 networks with the highest loading for that voxel. Multiple comparisons were accounted for using the false discovery rate (q<0.05). Dotted lines on scatterplots represent the 95% confidence interval.
Next, we repeated these analyses, but instead quantified volume (rather than cortical thickness) within each structural covariance network. These analyses revealed that overall psychopathology was associated with reduced volume in all networks, suggesting a global association with gray matter volume (partial r values ≤−0.14; see Table S2 in the online supplement). As illustrated in Figure 4A and 4B, total gray matter volume was negatively associated with overall psychopathology symptoms. In contrast, anxious-misery symptoms were associated with increased volume in most networks (partial r values ≤−0.12; see Table S2). Specifically, total gray matter volume (Figure 4C) was positively associated with anxious-misery symptoms, as were all networks except one (network 7; Figure 4D). Furthermore, fear symptoms were associated with reduced volume in multiple regions (see Table S2), and the behavioral dimension showed reduced volume in the superior parietal cortex (network 6) and the fusiform cortex (network 10). However, associations with the fear and behavioral dimensions did not remain significant during sensitivity analyses (see below). Psychopathology-by-age and psychopathology-by-sex interactions were nonsignificant for volume. Significant age-by-sex interactions were apparent in all volume networks (false discovery rate–corrected p values ≥0.027) except networks 4 and 8.
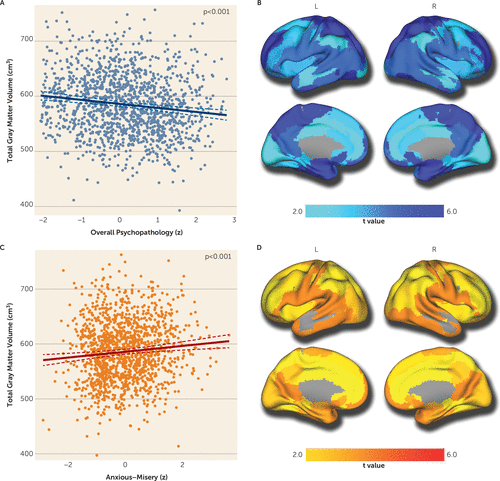
FIGURE 4. Association of overall psychopathology with reduced volume globally and of anxious-misery with greater volume in multiple structural covariance networks in a study of psychopathology and brain structure in youthsa
a Mass univariate analyses using generalized additive models that controlled for linear and nonlinear age, sex, and image quality revealed that overall psychopathology was associated with reduced volume across the brain (panels A and B). Conversely, anxious-misery symptoms were associated with increased volume in most networks (panels C and D). Composite network boundaries were obtained by assigning each voxel to one of the 18 networks with the highest loading for that voxel. Multiple comparisons were accounted for using the false discovery rate (q<0.05). Dotted lines on scatterplots represent the 95% confidence interval.
Sensitivity Analyses Provide Convergent Results
To ensure that our results were not specific to NMF networks, we evaluated associations using a highly accurate anatomical brain parcellation. Parcellation results aligned with analyses using NMF networks for all dimensions including fear, overall psychopathology, and anxious-misery (see Figure S3 in the online supplement). In addition, we conducted sensitivity analyses to evaluate potentially confounding variables. Nearly all associations between cortical thickness and fear and between volume and overall psychopathology or anxious-misery remained significant after excluding participants taking psychotropic medications (11%) and including maternal education as an additional covariate (see Table S3 in the online supplement). However, the associations between network thickness and fear were no longer significant when average cortical thickness was included as an additional covariate (false discovery rate–corrected p values ≥0.485), suggesting a distributed effect. Similarly, the associations between network volume and either overall psychopathology or anxious-misery were no longer significant when total gray matter volume was added as a covariate, consistent with a global effect (false discovery rate–corrected p values ≥0.229). Finally, using a traditional case-control approach, we found that cortical thickness and volume were reduced in most diagnostic categories (see Table S4 in the online supplement), illustrating the lack of specificity when using this approach.
Structural Covariance Networks Predict Dimensions of Psychopathology
Next, we tested whether structural covariance networks provided information about psychopathology above and beyond demographic characteristics and cognitive performance. We found a significant difference between a reduced model with only age, sex, and the three cognitive factors and a full model in which fear was predicted by age, sex, cognitive factors, and the 18 cortical thickness networks (F=2.32, df=1367, 1385, p=0.001). The correlation (r) between the actual fear scores and the predicted fear scores in the full model (N=1,389) was 0.28 (p<0.001) (see Figure S4A in the online supplement). However, while the proportion of variance in fear explained by the predictors improved in the full model, it was still relatively modest (adjusted R2=0.06). For volume networks, there was a significant difference between the reduced and full models for overall psychopathology (F=1.78, df=1367, 1385, p=0.023) but not anxious-misery (p=0.218). The correlation between the actual and predicted overall psychopathology scores in the full model (N=1,389) was 0.35 (p<0.001) (see Figure S4B in the online supplement). Compared with the reduced model, the proportion of variance in overall psychopathology explained by the predictors also showed a small improvement in the full model (adjusted R2=0.11).
Discussion
Leveraging a large sample of youths and multivariate analysis techniques, we provide novel evidence that dimensions of psychopathology that cross clinical diagnostic categories are dissociably linked to abnormalities in brain structure. The fear dimension was associated with diminished cortical thickness in the majority of networks. Furthermore, higher levels of overall psychopathology were associated with global reductions in gray matter volume, while anxious-misery symptoms were associated with increased volume in most networks. Results were highly convergent when accounting for a range of covariates and when different image analysis methods were used. Finally, structural networks predicted psychopathology symptoms above and beyond demographic characteristics and cognitive performance.
Advantages of a Dimensional Approach to Fear
We found that cortical thickness was reduced in association with fear symptoms in most networks. This result is consistent with case-control studies in adults showing cortical thinning in posttraumatic stress disorder, specific phobia, and social anxiety disorder (4, 22, 23). Our results are also broadly convergent with fear and anxiety networks identified using task-based functional MRI including the salience and ventral attention networks, which are critical for processing of emotionally salient information and attention bias to threat (24). These networks include regions that were affected in this study, including the anterior insula, anterior cingulate cortex, temporo-parietal junction, and ventrolateral prefrontal cortex. The anterior cingulate has been associated with self-regulation, including emotional processing (25), salience processing (26), and attention (27), and has demonstrated functional connectivity with the insula (25, 26), which is implicated in fear. However, our results also extend beyond these salience and emotion regulation networks, suggesting a more widespread effect. While our results are generally consistent with previous case-control research, they build on this literature by showing that global reduced cortical thickness is associated with the spectrum of fear symptoms across disorders in a community-based developmental sample with substantial comorbidity.
Overall Psychopathology Across Disorders Is Associated With Reduced Gray Matter Volume
It is increasingly recognized that psychopathology exists on a continuum. In a dimensional framework, individuals may be characterized by a profile of symptoms that span categorical boundaries (1, 3). Critically, the bifactor model captures comorbidity and individual variation in the overall level of psychopathology through the general p factor, which may contribute to the nonspecificity of biomarkers found across disorders (2). Recent research has demonstrated that p is linked to individual variation in cognition (28), executive function (29), cerebral blood flow (30), and genomics (31). In this study, we add to this growing literature by demonstrating that higher levels of p were associated with global reductions in gray matter volume in youths. This finding is consistent with work by Goodkind et al. reporting common gray matter volume loss across multiple psychiatric disorders in adults (8) and with previous work showing reduced volume associated with p (13, 14). In contrast to the reduction of gray matter volume seen with higher levels of overall psychopathology, higher levels of anxious-misery symptoms were associated with significant increases in global gray matter volume. Although the magnitudes of these effects were small, they are consistent with effect sizes reported for other variables associated with brain structure, such as IQ (32), and for other forms of psychopathology (5).
Interpreting Specificity Within the Bifactor Model
Associations with specific model dimensions, such as fear or anxious-misery, must be understood within the context of the bifactor model. Notably, scores from the bifactor model are uncorrelated and represent the burden of a specific dimension while accounting for the overall burden of general psychopathology. In contrast, dimensions derived from traditional factor-analytic models can be highly correlated with each other, which reduces the specificity of observed associations (1, 3). As in any factor model, an individual receives a score from each dimension of the bifactor model, and groups can be compared on these scores. For example, while patients with depression, on average, have high scores on the anxious-misery dimension, because of prominent comorbidity, they also tend to have high levels of overall psychopathology. Accordingly, even though the specific anxious-misery subfactor is associated with greater gray matter volume, this effect may be overwhelmed by the countervailing impact of the overall psychopathology dimension. Indeed, such an example illustrates the advantages of a hierarchical dimensional framework for parsing heterogeneous categorical clinical diagnoses. In contrast, case-control analyses in each diagnostic category yielded nonspecific results, further illustrating the advantage of this approach. Given that traditional diagnostic categories do not take into account the substantial heterogeneity within and comorbidity among disorders, reliance on categorical diagnoses may impede the development of clinically useful neurobiological markers (33–35).
Understanding the Interplay Between Psychopathology and Brain Development
Whereas the majority of translational psychiatric imaging studies have considered adults, in this study we document associations with dimensions of psychopathology in a community-based sample of youths. The PNC is a quasi-epidemiological sample; however, the clinical screening diagnoses were globally consistent with population rates (36). It is important to interpret our results in the context of normative cortical maturation, where reductions in cortical thickness and volume due to both myelination and pruning follow distinct trajectories throughout development (21, 37). Reduced volume or thickness associated with psychopathology is consistent with at least three potential developmental aberrations. First, our findings could represent a structural abnormality that is present from early life (or even in utero) and fixed throughout development. Such a deficit could potentially be linked to maternal infections during pregnancy or obstetric complications at birth (38). Second, these results could alternatively stem from a flattened trajectory of cortical expansion in early childhood, with reduced peak cortical volume and thickness. Finally, these results may be consistent with an accelerated course (or earlier onset) of the normative process of cortical thinning and gray matter volume loss. Intriguingly, recent evidence from multiple lines of research, including epigenetics and translational neuroimaging, suggests that childhood adversity may accelerate the process of cortical development (39). To disambiguate these possibilities, it will be necessary to follow large samples of youths longitudinally from early in life and to acquire detailed data regarding pregnancy and the childhood environment.
Conclusions
This study provides novel evidence that transdiagnostic fear symptoms are associated with reduced cortical thickness during development, while reduction in gray matter volume scales with the overall level of psychopathology present. Longitudinal designs may allow researchers to determine whether these changes precede and predict the onset or worsening of psychopathology over time. Additionally, the use of bidirectional translational models that integrate multiple modalities will allow us to better probe for causal relationships (40).
1 : A hierarchical taxonomy of psychopathology can transform mental health research. Perspect Psychol Sci 2019;
2 : All for one and one for all: mental disorders in one dimension. Am J Psychiatry 2018; 175:831–844Link, Google Scholar
3 : Implications of the hierarchical structure of psychopathology for psychiatric neuroimaging. Biol Psychiatry Cogn Neurosci Neuroimaging 2017; 2:310–317Crossref, Medline, Google Scholar
4 : Reduced cortical thickness with increased lifetime burden of PTSD in OEF/OIF veterans and the impact of comorbid TBI. Neuroimage Clin 2013; 2:601–611Crossref, Medline, Google Scholar
5 : Cortical abnormalities in adults and adolescents with major depression based on brain scans from 20 cohorts worldwide in the ENIGMA Major Depressive Disorder Working Group. Mol Psychiatry 2017; 22:900–909Crossref, Medline, Google Scholar
6 : Progressive reduction in cortical thickness as psychosis develops: a multisite longitudinal neuroimaging study of youth at elevated clinical risk. Biol Psychiatry 2015; 77:147–157Crossref, Medline, Google Scholar
7 : Cortical thinning of the attention and executive function networks in adults with attention-deficit/hyperactivity disorder. Cereb Cortex 2007; 17:1364–1375Crossref, Medline, Google Scholar
8 : Identification of a common neurobiological substrate for mental illness. JAMA Psychiatry 2015; 72:305–315Crossref, Medline, Google Scholar
9 : Cortical thickness or grey matter volume? The importance of selecting the phenotype for imaging genetics studies. Neuroimage 2010; 53:1135–1146Crossref, Medline, Google Scholar
10 : Age of onset of mental disorders: a review of recent literature. Curr Opin Psychiatry 2007; 20:359–364Crossref, Medline, Google Scholar
11 : Twelve-month and lifetime prevalence and lifetime morbid risk of anxiety and mood disorders in the United States. Int J Methods Psychiatr Res 2012; 21:169–184Crossref, Medline, Google Scholar
12 : Is there a general factor of prevalent psychopathology during adulthood? J Abnorm Psychol 2012; 121:971–977Crossref, Medline, Google Scholar
13 : Structural alterations within cerebellar circuitry are associated with general liability for common mental disorders. Mol Psychiatry 2018; 23:1084–1090Crossref, Medline, Google Scholar
14 : Distinct patterns of reduced prefrontal and limbic grey matter volume in childhood general and internalizing psychopathology. Clin Psychol Sci 2017; 5:1001–1013Crossref, Medline, Google Scholar
15 : Neuroimaging of the Philadelphia Neurodevelopmental Cohort. Neuroimage 2014; 86:544–553Crossref, Medline, Google Scholar
16 : The Philadelphia Neurodevelopmental Cohort: a publicly available resource for the study of normal and abnormal brain development in youth. Neuroimage 2016; 124(Pt B):1115–1119Crossref, Medline, Google Scholar
17 : Finding imaging patterns of structural covariance via non-negative matrix factorization. Neuroimage 2015; 108:1–16Crossref, Medline, Google Scholar
18 : Quantitative assessment of structural image quality. Neuroimage 2018; 169:407–418Crossref, Medline, Google Scholar
19 : Patterns of coordinated cortical remodeling during adolescence and their associations with functional specialization and evolutionary expansion. Proc Natl Acad Sci USA 2017; 114:3527–3532Crossref, Medline, Google Scholar
20 : mgcv: GAMs and generalized ridge regression for R. R News 2001; 1:20–25Google Scholar
21 : Sex differences in the developing brain: insights from multimodal neuroimaging. Neuropsychopharmacology 2019; 44:71–85Crossref, Medline, Google Scholar
22 : Cortical thinning of the right anterior cingulate cortex in spider phobia: a magnetic resonance imaging and spectroscopy study. Brain Res 2014; 1576:35–42Crossref, Medline, Google Scholar
23 : Grey matter abnormalities in social anxiety disorder: a pilot study. Metab Brain Dis 2012; 27:299–309Crossref, Medline, Google Scholar
24 : Functional network dysfunction in anxiety and anxiety disorders. Trends Neurosci 2012; 35:527–535Crossref, Medline, Google Scholar
25 : Cognitive and emotional influences in anterior cingulate cortex. Trends Cogn Sci 2000; 4:215–222Crossref, Medline, Google Scholar
26 : Dissociable intrinsic connectivity networks for salience processing and executive control. J Neurosci 2007; 27:2349–2356Crossref, Medline, Google Scholar
27 : The attention system of the human brain: 20 years after. Annu Rev Neurosci 2012; 35:73–89Crossref, Medline, Google Scholar
28 : The structure of psychopathology in adolescence and its common personality and cognitive correlates. J Abnorm Psychol 2016; 125:1039–1052Crossref, Medline, Google Scholar
29 : Common and dissociable mechanisms of executive system dysfunction across psychiatric disorders in youth. Am J Psychiatry 2016; 173:517–526Link, Google Scholar
30 : Common and dissociable regional cerebral blood flow differences associate with dimensions of psychopathology across categorical diagnoses. Mol Psychiatry 2018; 23:1981–1989Crossref, Medline, Google Scholar
31 : A polygenic p factor for major psychiatric disorders. Transl Psychiatry 2018; 8:205Crossref, Medline, Google Scholar
32 : Intellectual ability and cortical development in children and adolescents. Nature 2006; 440:676–679Crossref, Medline, Google Scholar
33 : Getting serious about variation: lessons for clinical neuroscience (a commentary on “The Myth of Optimality in Clinical Neuroscience”). Trends Cogn Sci 2018; 22:368–369Crossref, Medline, Google Scholar
34 : Quantifying heterogeneity attributable to polythetic diagnostic criteria: theoretical framework and empirical application. J Abnorm Psychol 2014; 123:452–462Crossref, Medline, Google Scholar
35 : DSM-5 field trials in the United States and Canada, part II: test-retest reliability of selected categorical diagnoses. Am J Psychiatry 2013; 170:59–70Link, Google Scholar
36 : Comorbidity of physical and mental disorders in the neurodevelopmental genomics cohort study. Pediatrics 2015; 135:e927–e938Crossref, Medline, Google Scholar
37 : Development of the cerebral cortex across adolescence: a multisample study of inter-related longitudinal changes in cortical volume, surface area, and thickness. J Neurosci 2017; 37:3402–3412Crossref, Medline, Google Scholar
38 : The hippocampus in families with schizophrenia in relation to obstetric complications. Schizophr Res 2008; 104:71–78Crossref, Medline, Google Scholar
39 : Annual research review: Parenting and children’s brain development: the end of the beginning. J Child Psychol Psychiatry 2011; 52:409–428Crossref, Medline, Google Scholar
40 : A translational neuroscience approach to understanding the development of social anxiety disorder and its pathophysiology. Am J Psychiatry 2014; 171:1162–1173Link, Google Scholar