Association of GSK-3β Genetic Variation With GSK-3β Expression, Prefrontal Cortical Thickness, Prefrontal Physiology, and Schizophrenia
Abstract
Objective
Glycogen synthase kinase 3β (GSK-3β) is an enzyme implicated in neurodevelopmental processes with a broad range of substrates mediating several canonical signaling pathways in the brain. The authors investigated the association of variation in the GSK-3β gene with a series of progressively more complex phenotypes of relevance to schizophrenia, a neurodevelopmental disorder with strong genetic risk.
Method
Based on computer predictions, the authors investigated in humans the association of GSK-3β functional variation with 1) GSK-3β mRNA expression from postmortem prefrontal cortex, 2) GSK-3β and β-catenin protein expression from peripheral blood mononuclear cells (PBMCs), 3) prefrontal imaging phenotypes, and 4) diagnosis of schizophrenia.
Results
Consistent with predictions, the TT genotype of a single-nucleotide polymorphism in GSK-3β (rs12630592) was associated with reduced GSK-3β mRNA from postmortem prefrontal cortex. Furthermore, this genotype was associated with GSK-3β protein expression and kinase activity, as well as with downstream effects on β-catenin expression in PBMCs. Finally, the TT genotype was associated with attenuated functional MRI prefrontal activity, reduced prefrontal cortical thickness, and diagnosis of schizophrenia.
Conclusions
These results suggest that GSK-3β variation is implicated in multiple phenotypes relevant to schizophrenia.
Glycogen synthase kinase 3β (GSK-3β) is highly expressed in the brain (1), plays a crucial role in modulating signal transduction from the extracellular space to the nucleus, and is implicated in a series of neurodevelopmental processes (2). These diverse effects are also likely mediated by its role in phosphorylation of β-catenin, which regulates gene expression (3). Schizophrenia is a neurodevelopmental disorder (4) whose risk is mainly explained by genetic variation (5) and is characterized by dysfunction in the prefrontal cortex, especially during working memory and attention (6). Previous studies have demonstrated genetic association of GSK-3β with diagnosis of schizophrenia (7, 8). Other investigations have demonstrated reduced levels of GSK-3β (9) and of its phosphorylation (10, 11) in postmortem prefrontal cortex samples from individuals with schizophrenia. Furthermore, several molecular pathways already implicated in schizophrenia, including dopamine, serotonin, DISC1, neuregulin, and Wnt, converge onto GSK-3β (12–16). In particular, previous evidence robustly suggests that a dopamine D2-signaling pathway including the serine/threonine protein kinase AKT1 modulates GSK-3β activity and its regulatory output on β-catenin (3, 16, 17). Consistently, antipsychotic drugs blocking D2 receptors also modulate GSK-3β expression and activity (18).
Studies of genetic variation within GSK-3β have also been conducted. In vitro experiments have suggested association of GSK-3β single-nucleotide polymorphisms (SNPs) with GSK-3β promoter activity (8), transcriptional strength, and splicing (19). Other studies have indicated that SNPs in GSK-3β affect temporal lobe volume in individuals with major depression (20) and schizophrenia (21). Furthermore, association of GSK-3β variants with clinical measures related to mood disorders (22, 23) and with susceptibility to schizophrenia (8) has been reported. However, to our knowledge, no previous study has evaluated the functional molecular effects of GSK-3β polymorphisms in humans together with their direct implications for brain physiology relevant to schizophrenia.
The aim of this study was to explore molecular correlates of GSK-3β genetic variation as well as its relevance to several phenotypes related to schizophrenia. Since genetic variation does not directly cause behavioral phenotypes but rather has molecular effects that influence neural systems-level processing, we investigated the possible effect of GSK-3β polymorphisms on a series of progressively more complex phenotypes potentially linked to risk for schizophrenia. Specifically, after conducting computer predictions of functional SNPs, we tested the association of genetic variation (rs12630592) with GSK-3β mRNA levels in postmortem prefrontal cortex, which was further validated in terms of protein expression and phosphorylation in peripheral lymphocytes in another independent sample. Demonstration of association of this SNP with prefrontal gene expression prompted the question of whether this molecular mechanism underlies more complex phenotypes (i.e., prefrontal activity during cognitive performance and prefrontal cortical thickness in healthy humans). Robust effects of rs12630592 on these phenotypes further suggested investigation of this SNP regarding its relationship with the diagnosis of schizophrenia, the most complex of the phenotypes examined.
Method
Bioinformatic Modeling of Molecular Effects of GSK-3β Variants
We used bioinformatic tools (rVISTA 2.0, SpliceAid 2, NNSPLICE, rBLOSUM64, SIFT, PolyPhen, and miRBase) to predict GSK-3β SNPs with potential effects on molecular regulatory mechanisms possibly affecting GSK-3β expression (see the data supplement that accompanies the online edition of this article). Based on these bioinformatic analyses, we further investigated variants associated with predicted functional effects. In order to focus our analysis on genetic variation with possible common biological relevance in humans, we selected SNPs with a known minor allele frequency >0.03, which allows investigation of a large enough number of individuals with the minor allele to detect genotype effects in postmortem and in vivo studies (24). Thus, a publicly available database of postmortem brains was interrogated to study association of the remaining SNPs with GSK-3β mRNA expression in the prefrontal cortex.
Association of GSK-3β Variation With Postmortem Prefrontal Expression of GSK-3β mRNA
Data from 215 brains of nonpsychiatric individuals (males, N=152; mean age, 33.1 years [SD=21.0]; brain pH=6.5 [SD=0.3]; postmortem interval, 30.6 hours [SD=15.4]), with available information on age, sex, race, postmortem interval, pH, and smoking status, were analyzed. These data were obtained from the largest postmortem collection publicly available (http://braincloud.jhmi.edu [25]) (see the online data supplement). We focused on association of prefrontal mRNA expression with GSK-3β SNPs that had been predicted by bioinformatic tools and that had a minor allele frequency >0.03. Based on these selection criteria, data related to two SNPs (rs12630592 and rs6797689) were available from the postmortem collection.
For GSK-3β rs12630592, the postmortem sample included Caucasian and African American subjects with GG (N=44), GT (N=83), and TT (N=88) genotypes. For GSK-3β rs6797689, the sample included subjects with CC (N=170), CT (N=43), and TT genotypes (N=2). For both SNPs, genotype groups did not differ in terms of age, sex, smoking status, postmortem interval, and pH, even after collapsing the GSK-3β rs6797689 TT and CT groups into a single T carrier group (all p values >0.05).
Analysis of variance (ANOVA) was used to explore association of rs12630592 and rs6797689 with prefrontal mRNA expression. Given that rs6797689 did not demonstrate any statistically significant association with prefrontal mRNA expression, we focused further in vivo analyses on rs12630592.
Healthy Human Subjects
DNA was extracted from whole blood from a sample of 424 healthy humans (see the online data supplement) using standard procedures. GSK-3β rs12630592 was determined by real-time polymerase chain reaction (see the data supplement). Participants underwent one or more of the procedures described below.
Association of rs12630592 With GSK-3β and β-Catenin Expression in Peripheral Blood Mononuclear Cells (PBMCs)
Blood samples were drawn from 31 healthy individuals (female, N=17; mean age, 27.5 years [SD=5.9]; GG genotype, N=9; GT genotype, N=10; TT genotype, N=12). PBMCs were isolated (26), and aliquots (2 μl) of samples were used for the protein determination (see the data supplement). Immunoblot was performed using selective antibodies against GSK-3β, P-Ser9-GSK-3β, β-catenin, and α-tubulin (see the data supplement). Optical density values of GSK-3β and β-catenin were normalized to α-tubulin for variation in loading and transfer. The levels of P-Ser9-GSK-3β were normalized to total GSK-3β. ANOVA was used for statistical analyses of averaged and normalized protein values.
Association of rs12630592 With Prefrontal Physiology During Cognition
A total of 209 healthy subjects underwent functional MRI (fMRI) during cognitive performance. Of these, 108 individuals (female, N=50; mean age, 29.8 years [SD=7.2]; IQ=110.1 [SD=12.5]) performed the one-, two-, and three-back versions of the n-back working memory task (27) (GG genotype, N=32; GT genotype, N=59; TT genotype, N=17). We used a three-run block design in which each block consisted of eight alternating zero- and one-, two-, or three-back tasks. Presentation of the runs was counterbalanced. Genotype groups were matched for age, IQ (WAIS), and handedness (Edinburgh Handedness Inventory [28]) (all p values >0.4) but not for sex (χ2=14.3, p<0.01).
A total of 151 subjects (female, N=85; mean age, 26.3 years [SD=5.6]; IQ=110.7 [12.0]) performed the variable attentional control task, eliciting parametric increase of attentional control (29) (GG genotype, N=46; GT genotype, N=86; TT genotype, N=19). The variable attentional control task is an event-related design (17, 29–31) that allows for investigation of brain activity during three levels of attentional control (low, intermediate, and high), which are obtained by manipulating both the relative directions of arrows with different sizes and the related cue words (see the data supplement).
Genotype groups were matched for age, sex, IQ, and handedness (all p values >0.1). Fifty-two individuals performed both tasks used in this study.
fMRI Data Acquisition and Analysis
fMRI data were acquired using a 3-T General Electric scanner (General Electric, Fairfield, Conn.). The analysis was completed using Statistical Parametric Mapping 8.0 (http://www.fil.ion.ucl.ac.uk/spm). After preprocessing (see the data supplement), the individual contrast images relative to the n-back (one-, two-, and three-back tasks compared with zero-back tasks) and variable attentional control (low, intermediate, and high levels of attentional control compared with baseline) tasks were used in random-effects models for group-level analysis. In particular, ANOVAs were performed on these contrasts, with genotype as the predictor and working memory or attentional control load as repeated-measures factors. Sex was used as a covariate of no interest in the n-back ANOVA to account for nonhomogeneous distribution of this variable. We used a statistical threshold with a p value <0.001 (minimum cluster size [k]=5), with further family-wise error small-volume correction at a p value <0.05, using as volume of interest the Wake Forest University PickAtlas (http://fmri.wfubmc.edu/cms/software#PickAtlas) Brodmann’s areas (BAs), in which significant clusters were located (i.e., BA 10 for the variable attentional control task and BA 46 for the n-back task). These regions of interest were chosen a priori based on our investigation of prefrontal phenotypes and on the well-established association between these tasks and activity in the dorsolateral prefrontal cortex (BA 9/10/46) (27, 30, 32). Fisher’s post hoc test was performed on blood-oxygen-level-dependent (BOLD) responses extracted from significant clusters using MarsBar (http://marsbar.sourceforge.net/) (see the data supplement).
Pearson’s test was used to investigate brain activity-behavior correlations. In particular, we reasoned that the repeated-measures design measuring brain activity and behavior at different cognitive loads within a single cognitive domain allows for maximization and better characterization of the relationship between brain and behavior if differences between the different cognitive loads are considered. Therefore, we correlated the delta of BOLD responses between loads with the delta of percent-correct responses between loads as follows: n-back task: three-back minus two-back activity compared with two-back minus three-back accuracy (a similar procedure was used for deltas between two-back and one-back tasks); variable attentional control task: high- minus intermediate-loads activity compared with intermediate- minus high-loads accuracy (the same method was used for deltas between intermediate and low loads).
ANOVA was used for analysis of behavioral data.
Association of rs12630592 With Prefrontal Cortical Thickness
A total of 166 healthy subjects (female, N=103; mean age, 26.11 years [SD=6.69]; IQ=107.43 [SD=12.66) underwent structural MRI (GG genotype, N=48; GT genotype, N=94; TT genotype, N=24). Genotype groups were matched for age, sex, IQ, and handedness (all p values >0.5). After acquisition of three-dimensional images (see the online data supplement), MRI-based quantification of cortical thickness was performed using Freesurfer 5.0.0 (http://surfer.nmr.mgh.harvard.edu/) (see the data supplement). For each hemisphere, differences in prefrontal cortical thickness between genotype groups were tested by computing a general linear model of the effects of genotype (GG compared with TT, GG compared with GT, and GT compared TT) on prefrontal cortical thickness at each vertex. Age and sex were used as covariates of no interest. A statistical threshold was set at a p value <0.001. To control for the effects of multiple testing, permutation analysis (105 permutations) was performed on the mean cortical thickness values extracted from the prefrontal clusters associated with a main effect of genotype using the Resampling software program (http://core.ecu.edu/psyc/wuenschk/StatHelp/Resampling.htm), which adopts a classical “randomization without replacement” algorithm.
Association of rs12630592 With Diagnosis of Schizophrenia
A case-control study was performed in a sample of Caucasian individuals (N=1,057), including 459 patients with schizophrenia (female, N=116; mean age, 36.65 years [SD=11.11]) and 598 healthy subjects (female, N=313; mean age, 26.86 years [SD=7.53]) recruited from the Apulia region. The Structured Clinical Interview for DSM-IV Axis I Disorders (33) was used to diagnose schizophrenia and to exclude any psychiatric disorders in comparison subjects. Personal and family histories of psychiatric disorders were ruled out for all comparison subjects. Genotype groups deviated from Hardy-Weinberg equilibrium in both the patient and comparison groups (p<0.01). A quality-control check was also performed (see the data supplement). Logistic regression was performed on GSK-3β rs12630592 genotype data using HAPSTAT (http://www.bios.unc.edu/~lin/hapstat/). Results were corrected for multiple comparisons considering the number of models applied (i.e., dominant, recessive, and additive).
The experimental protocol was approved by the local institutional review boards, and participants provided written informed consent after receiving complete description of the study.
Results
Bioinformatic Modeling of Molecular Effects of GSK-3β Variants
Computer predictions indicated that 50 SNPs within GSK-3β have molecular effects on gene function (see the online data supplement). Eight of these SNPs had a known minor allele frequency >0.03 and thus were considered and investigated in the subsequent step of our study. Among these eight SNPs, data related to rs12630592 and rs6797689 were available for postmortem biological validation of the predictions and therefore were of particular interest in our hierarchical strategy. In particular, the NNSPLICE prediction algorithm and SpliceAid2 database indicated that the intronic variant rs12630592 creates a strong 3′-splice site (score=0.95 on a scale from 0 to 1) and a binding site for the SRp20 exonic splicing enhancer. Furthermore, rs12630592 destroys a binding site for MBNL1, a splicing regulatory factor that can act as a silencer or enhancer, depending on the context. These effects may modify splicing patterns with possible regulatory outcomes on the amount of the total GSK-3β transcript because of mechanisms of mRNA surveillance.
Computer analysis of rs6797689 predicted that this deep intronic variation destroys a binding site for the exonic splicing enhancer SRp30c and creates a binding site for the exonic splicing silencers hnRNP A1 and hnRNP A2/B1. Generally, these events do not imply splicing alterations.
Association of GSK-3β Variation With Postmortem Prefrontal Expression of GSK-3β mRNA
Postmortem data were available for two of the eight selected SNPs, rs12630592 and rs6797689. ANOVA indicated a main effect of rs12630592 on GSK-3β mRNA expression (F=7.18, df=2, 222, p=0.0009). Fisher’s post hoc analysis revealed reduced mRNA expression in subjects with the TT genotype compared with those with the GG genotype (p<0.0002) but no statistical difference between subjects with the TT genotype and those with the GT genotype. Furthermore, GG subjects exhibited greater mRNA expression than GT subjects (p=0.008) (Figure 1). There was no race effect on the association between genotype and GSK-3β mRNA expression (see the data supplement).
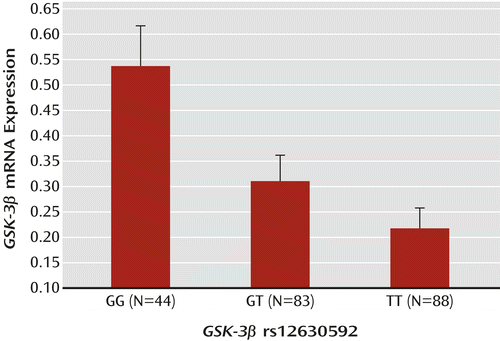
a Graph depicts normalized log2 ratios (sample/reference). Data from http://braincloud.jhmi.edu/. Error bars indicate standard error.
There was no effect of rs6797689 genotype on GSK-3β mRNA expression, even after combining T carriers into one group. Based on these results, we restricted all subsequent in vivo analyses to rs12630592.
Association of rs12630592 With GSK-3β and β-Catenin Expression in PBMCs
ANOVA indicated a main effect of rs12630592 on GSK-3β protein expression (F=11.0, df=2, 28, p=0.0003) and phosphorylation of the Ser9 residue (F=3.8, df=2, 28, p=0.03) in PBMCs. Fisher’s post hoc test revealed that TT subjects have attenuated immunoreactivity of GSK-3β protein and of its phosphorylation compared with GG subjects (expression, p=0.0001; phosphorylation, p=0.01) and GT subjects (expression, p=0.002; phosphorylation, p=0.04) (Figure 2). Furthermore, there was a main effect of rs12630592 on β-catenin protein expression (F=5.1, df=2, 28, p=0.01), with TT subjects having reduced measures compared with GG (p=0.004) and GT (p=0.03) subjects (Figure 2). No statistical difference was found between GG and GT subjects in these analyses.
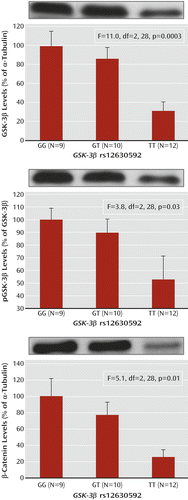
a PBMCs=peripheral blood mononuclear cells; pGSK-3β=phosphorylated GSK-3β. Error bars indicate standard error.
Association of rs12630592 With Prefrontal Physiology During Cognition
n-Back task.
No main effect of genotype or genotype-by-load interaction was present in behavioral data for this sample (all p values >0.2). Thus, the effect of the rs12630592 genotype on brain responses during working memory processing in this sample reflects how the brain processed working memory and not how individuals scored on the test. Statistical Parametric Mapping ANOVA of imaging data indicated a main effect of rs12630592 on a cluster in the left dorsolateral prefrontal cortex (Montreal Neurological Institute [MNI] coordinates: x=–40, y=56, z=6; BA 10; k=7; family-wise error-corrected, p=0.04) (Figure 3). Analysis of parameter estimates extracted from this cluster revealed that TT subjects have attenuated dorsolateral prefrontal cortex activity compared with GT (p=0.005) and GG (p=0.002) subjects. No significant difference was present between GG and GT subjects (Figure 3). No genotype-by-load interaction was found on brain activity.
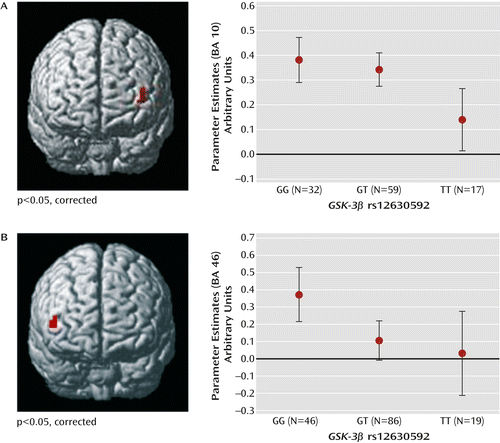
a Data from functional MRI scans and graphs depict main effect on prefrontal activity during the n-back task in panel A and the variable attentional control task in panel B. Error bars indicate standard error.
Pearson’s test also indicated a relationship between activity in the cluster showing a main effect of genotype and indices of behavioral accuracy. In particular, in GG subjects, there was a significant negative correlation between the difference in prefrontal BOLD responses (three-back minus two-back task) and the difference in accuracy (two-back minus three-back task) at high cognitive loads (r=–0.36; p=0.04). In contrast, a positive correlation was present in TT subjects (r=0.40; p=0.1) (Figure 4). A difference test indicated that the slope of these correlations was statistically different (p=0.01). Results for GT subjects did not demonstrate a significant correlation. No significant correlations were present when investigating deltas between two-back and one-back task data.
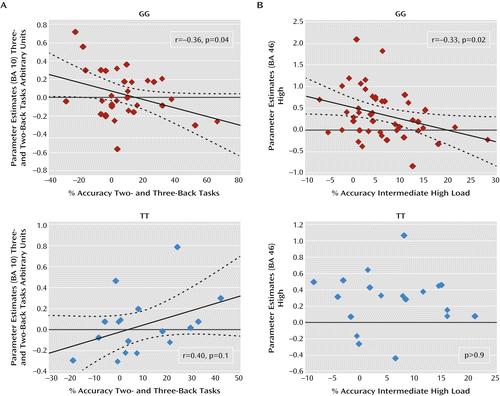
a BOLD=blood-oxygen-level-dependent; BA=Brodmann’s area. Panel A shows a negative correlation in GG subjects between the difference in prefrontal BOLD responses (three- minus two-back task) and the difference in accuracy (two- minus three-back task) at high cognitive loads during working memory. Panel B shows a similar correlation between deltas of BOLD responses (high minus intermediate) and deltas of accuracy (intermediate minus high) at high cognitive loads during attentional control in GG subjects. In TT subjects, a positive correlation during the n-back task fell short of statistical significance, and no relationship was observed during the variable attentional control task.
Variable attentional control task.
Again, no main effect of genotype or genotype-by-load interaction was present in behavioral fMRI data. Imaging data again revealed a main effect of rs12630592 on a region in the right dorsolateral prefrontal cortex similar to that found in the n-back task analysis (MNI coordinates: x=50, y=45, z=7; BA=46; k=5; corrected p=0.04) (Figure 3). Parameter estimates extracted from this cluster indicated that TT and GT subjects had attenuated responses compared with GG subjects (p=0.02 and p=0.007, respectively). No significant difference was present between TT and GT subjects (Figure 3).
Consistent with the n-back task results, Pearson’s test revealed in GG subjects a significant negative correlation between the difference in prefrontal BOLD response (high minus intermediate) and the difference in accuracy (intermediate minus high) at high cognitive loads (r=–0.33; p=0.02) (Figure 4). No significant correlation between these variables was present in TT or GT subjects. Furthermore, no significant correlation was found when analyzing deltas between intermediate and low levels of attentional control processing.
Association of rs12630592 With Prefrontal Cortical Thickness
ANOVA indicated a main effect of rs12630592 on cortical thickness in prefrontal clusters, with greater values in GG relative to GT and TT subjects, as well as in GT relative to TT subjects (Figure 5, also see Tables S1 and S2 in the online data supplement). No significant effects were present in the inverse comparisons.
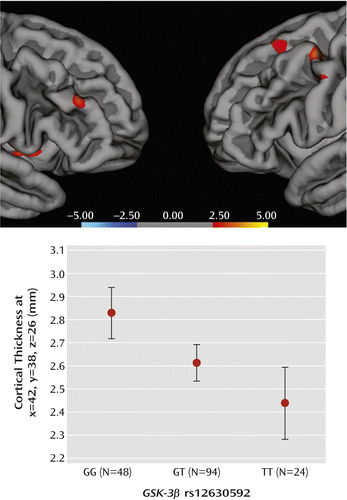
a Color bar represents logarithm10 of p values, showing association between GSK-3β rs12630592 and prefrontal cortical thickness (GG > TT) in healthy subjects, and the graph depicts values of cortical thickness extracted from a representative prefrontal cluster (Montreal Neurological Institute coordinates: x=42, y=38, z=26). Error bars indicate standard error.
Association of rs12630592 With Diagnosis of Schizophrenia
Logistic regression revealed association of the rs12630592 T allele with diagnosis of schizophrenia under a recessive model (p=0.002). The effect size of this association was an odds ratio of 1.13, with a 95% confidence interval of 0.95–1.34 (minor allele T frequency: patients=0.45; comparison subjects=0.41). These results were still significant after correction for the number of models tested (p=0.006). Dominant and additive models were not statistically significant.
Discussion
We used a translational genetics approach to investigate the relevance of GSK-3β to aspects of human brain function and behavior related to schizophrenia. The first level of this strategy entailed computer predictions. Results indicated that the intronic SNP rs12630592 may regulate splicing, possibly triggering mechanisms of mRNA surveillance, thus affecting the abundance of the total transcript (34, 35). In fact, the second level of this strategy addressed the functional relevance of this SNP to the abundance of the transcript in postmortem human prefrontal cortex. Consistent with the bioinformatic prediction, nonpsychiatric TT subjects exhibited reduced GSK-3β mRNA in the prefrontal cortex compared with GG subjects, as measured with a probe positioned in the gene to detect the main transcript variant. In line with the postmortem results, in vivo findings revealed reduced GSK-3β protein expression and reduced Ser-9 phosphorylation in PBMCs from healthy TT subjects. Interestingly, similar reductions in GSK-3β mRNA, protein expression, and its phosphorylation have also been observed in lymphocytes and postmortem prefrontal cortex of individuals with schizophrenia (9–11). A crucial downstream effect of the active Ser-9 dephosphorylated form of GSK-3β is the degradation and thus inactivation of β-catenin (3). In PBMCs, we found association of the TT genotype with reduced phosphorylation of GSK-3β and with reduced protein expression of β-catenin. Regardless of the specific mechanism, the more parsimonious interpretation of these data is that rs12630592 is associated with consistent effects on GSK-3β expression and phosphorylation, which also affect downstream signaling of gene expression with possible effect on the neuronal function of relevance to schizophrenia (11) and antipsychotic treatment (18).
We next validated these molecular effects on more complex phenotypes. Earlier studies have demonstrated that selective deletion of GSK-3β in neural progenitors results in overexpansion of the neural progenitor cells and thinner cortex (36). Our results demonstrate that the T allele predicts reduced prefrontal cortical thickness in healthy humans, suggesting that genetic variation in GSK-3β may confer risk for altered dynamics of neurodevelopment (18). Furthermore, the T allele of rs12630592 was associated with reduced prefrontal activity during working memory and attentional control, regardless of cognitive load. Additionally, rs12630592 genotype differentially predicted the load-dependent relationship between brain activity and cognitive behavior. At greater working memory and attentional control demands, greater load-mediated increase in prefrontal activity predicted lower decrease in behavioral accuracy in GG subjects. In other words, greater dorsolateral prefrontal cortex activity in GG subjects appeared to reflect greater behavioral accuracy at high cognitive loads. This relationship was not present in GT subjects, while in TT subjects, a nonsignificant association in the opposite direction was observed. An interpretation of the main effect of genotype and of the correlations with behavior is that greater dorsolateral prefrontal cortex activity in GG subjects is functional to better behavioral performance at high cognitive loads, while this is not the case in individuals carrying at least one T allele. More generally, these data suggest that rs12630592 is associated with prefrontal physiology during cognition altering the load-dependent architecture of its relationship with behavior. Abnormal prefrontal response (31, 37) during working memory and attentional processing are considered key features of schizophrenia.
In line with these results, the TT genotype was associated with schizophrenia in a recessive model, suggesting that the presence of the T allele on both chromosomes is necessary to increase risk. With an odds ratio of 1.13, our results of the association with diagnosis are consistent with earlier evidence indicating a highly polygenic model as best suited to explain risk for schizophrenia (38), such that genetically determined individual differences across domains of brain development and function may form a diathesis for this brain disorder (13). The association with diagnosis is also consistent with previous studies that have evaluated other GSK-3β polymorphisms (7, 8). Of note, SNPs associated with diagnosis of schizophrenia in these studies (i.e., rs3755557 [8], rs7624540, rs4072520, and rs6779828 [7]) are in high linkage disequilibrium with rs12630592 (http://hapmap.ncbi.nlm.nih.gov/). The association of rs12630592 with diagnosis of schizophrenia would not survive the statistical criteria for genome-wide significance, and it requires further study. However, the stringent logic of our hierarchical approach and the consistency of our results from molecular to more complex in vivo phenotypes provide support for this association not being spurious.
A limitation of this study is that the multiple associations that we have demonstrated between rs12630592 and different phenotypes do not imply reciprocal biological relationships because each analysis was performed using data sets from different samples. However, the fact that these associations have been found in samples that do not completely overlap may suggest the functional relevance of this polymorphism to the biology of CNS in the general population. Another important point is that it cannot be concluded that our prefrontal findings are specific to schizophrenia because prefrontal anomalies have been associated with other brain disorders (39, 40). However, the relationship between schizophrenia and prefrontal function is well established, such that the association that we found between rs12630592 and prefrontal phenotypes is relevant to this brain disorder but not exclusively.
1 : Expression of glycogen synthase kinase-3 isoforms in mouse tissues and their transcription in the brain. J Chem Neuroanat 2002; 23:291–297Crossref, Medline, Google Scholar
2 : GSK3 signalling in neural development. Nat Rev Neurosci 2010; 11:539–551Crossref, Medline, Google Scholar
3 : Roles of the Akt/GSK-3 and Wnt signaling pathways in schizophrenia and antipsychotic drug action. Am J Psychiatry 2010; 167:388–396Link, Google Scholar
4 : Implications of normal brain development for the pathogenesis of schizophrenia. Arch Gen Psychiatry 1987; 44:660–669Crossref, Medline, Google Scholar
5 : Genomics and behavior. Toward behavioral genomics. Science 2001; 291:1232–1249Crossref, Medline, Google Scholar
6 : Cognitive impairments in patients with schizophrenia displaying preserved and compromised intellect. Arch Gen Psychiatry 2000; 57:907–913Crossref, Medline, Google Scholar
7 : Association study of GSK3 gene polymorphisms with schizophrenia and clozapine response. Psychopharmacology (Berl) 2008; 200:177–186Crossref, Medline, Google Scholar
8 : Genetic association and identification of a functional SNP at GSK3β for schizophrenia susceptibility. Schizophr Res 2011; 133:165–171Crossref, Medline, Google Scholar
9 : Low GSK-3beta immunoreactivity in postmortem frontal cortex of schizophrenic patients. Am J Psychiatry 2000; 157:831–833Link, Google Scholar
10 : Possible involvement of post-dopamine D2 receptor signalling components in the pathophysiology of schizophrenia. Int J Neuropsychopharmacol 2008; 11:197–205Crossref, Medline, Google Scholar
11 : Convergent evidence for impaired AKT1-GSK3beta signaling in schizophrenia. Nat Genet 2004; 36:131–137Crossref, Medline, Google Scholar
12 : Judging a protein by more than its name: GSK-3. Sci STKE 2001; 2001:re12Medline, Google Scholar
13 : Schizophrenia genes, gene expression, and neuropathology: on the matter of their convergence. Mol Psychiatry 2005;10:40–68Google Scholar
14 : GSK-3β activity and hyperdopamine-dependent behaviors. Neurosci Biobehav Rev 2011; 35:645–654Crossref, Medline, Google Scholar
15 : The principal features and mechanisms of dopamine modulation in the prefrontal cortex. Prog Neurobiol 2004; 74:1–58Crossref, Medline, Google Scholar
16 : The Akt-GSK-3 signaling cascade in the actions of dopamine. Trends Pharmacol Sci 2007; 28:166–172Crossref, Medline, Google Scholar
17 : DRD2/AKT1 interaction on D2 c-AMP independent signaling, attentional processing, and response to olanzapine treatment in schizophrenia. Proc Natl Acad Sci USA 2011; 108:1158–1163Crossref, Medline, Google Scholar
18 : Schizophrenia as a GSK-3 dysregulation disorder. Trends Neurosci 2007; 30:142–149Crossref, Medline, Google Scholar
19 : GSK3B polymorphisms alter transcription and splicing in Parkinson’s disease. Ann Neurol 2005; 58:829–839Crossref, Medline, Google Scholar
20 : Association of GSK3beta polymorphisms with brain structural changes in major depressive disorder. Arch Gen Psychiatry 2009; 66:721–728Crossref, Medline, Google Scholar
21 : Temporal lobe grey matter volume in schizophrenia is associated with a genetic polymorphism influencing glycogen synthase kinase 3-β activity. Genes Brain Behav 2010; 9:365–371Crossref, Medline, Google Scholar
22 : The impact of glycogen synthase kinase 3β gene on psychotic mania in bipolar disorder patients. Prog Neuropsychopharmacol Biol Psychiatry 2011; 35:1303–1308Crossref, Medline, Google Scholar
23 : A haplotype of glycogen synthase kinase 3β is associated with early onset of unipolar major depression. Genes Brain Behav 2010; 9:799–807Crossref, Medline, Google Scholar
24 : Impact of complex genetic variation in COMT on human brain function. Mol Psychiatry 2006; 11:867–877Google Scholar
25 : Temporal dynamics and genetic control of transcription in the human prefrontal cortex. Nature 2011; 478:519–523Crossref, Medline, Google Scholar
26 : Reduced insulin-induced phosphatidylinositol-3-kinase activation in peripheral blood mononuclear leucocytes from patients with Alzheimer’s disease. Eur J Neurosci 2007; 26:2469–2472Crossref, Medline, Google Scholar
27 : Genetically determined measures of striatal D2 signaling predict prefrontal activity during working memory performance. PLoS ONE 2010; 5:e9348Crossref, Medline, Google Scholar
28 : The assessment and analysis of handedness: the Edinburgh inventory. Neuropsychologia 1971; 9:97–113Crossref, Medline, Google Scholar
29 : Effect of catechol-O-methyltransferase val158met genotype on attentional control. J Neurosci 2005; 25:5038–5045Crossref, Medline, Google Scholar
30 : Differentiating allocation of resources and conflict detection within attentional control processing. Eur J Neurosci 2007; 25:594–602Crossref, Medline, Google Scholar
31 : Nonlinear response of the anterior cingulate and prefrontal cortex in schizophrenia as a function of variable attentional control. Cereb Cortex 2010; 20:837–845Crossref, Medline, Google Scholar
32 : Physiological characteristics of capacity constraints in working memory as revealed by functional MRI. Cereb Cortex 1999; 9:20–26Crossref, Medline, Google Scholar
33 : Guide for the Structured Clinical Interview for DSM-IV Axis I Disorders–Research Version. New York, Biometrics Research, 1996Google Scholar
34 : Quality control of eukaryotic mRNA: safeguarding cells from abnormal mRNA function. Genes Dev 2007; 21:1833–1856Crossref, Medline, Google Scholar
35 : Structural implication of splicing stochastics. Nucleic Acids Res 2009; 37:4862–4872Crossref, Medline, Google Scholar
36 : GSK-3 is a master regulator of neural progenitor homeostasis. Nat Neurosci 2009; 12:1390–1397Crossref, Medline, Google Scholar
37 : Prefrontal dysfunction in schizophrenia controlling for COMT Val158Met genotype and working memory performance. Psychiatry Res 2006; 147:221–226Crossref, Medline, Google Scholar
38 ;
39 : Genetic liability for bipolar disorder is characterized by excess frontal activation in response to a working memory task. Biol Psychiatry 2008; 64:513–520Crossref, Medline, Google Scholar
40 : Activation in ventral prefrontal cortex is sensitive to genetic vulnerability for attention-deficit hyperactivity disorder. Biol Psychiatry 2006; 60:1062–1070Crossref, Medline, Google Scholar