Basal Ganglia Volume and Shape in Children With Attention Deficit Hyperactivity Disorder
Abstract
Objective: Volumetric abnormalities of basal ganglia have been associated with attention deficit hyperactivity disorder (ADHD), especially in boys. To specify localization of these abnormalities, large deformation diffeomorphic metric mapping (LDDMM) was used to examine the effects of ADHD, sex, and their interaction on basal ganglia shapes. Method: The basal ganglia (caudate, putamen, globus pallidus) were manually delineated on magnetic resonance imaging from 66 typically developing children (35 boys) and 47 children (27 boys) with ADHD. LDDMM mappings from 35 typically developing children were used to generate basal ganglia templates. Shape variations of each structure relative to the template were modeled for each subject as a random field using Laplace-Beltrami basis functions in the template coordinates. Linear regression was used to examine group differences in volumes and shapes of the basal ganglia. Results: Boys with ADHD showed significantly smaller basal ganglia volumes compared with typically developing boys, and LDDMM revealed the groups remarkably differed in basal ganglia shapes. Volume compression was seen bilaterally in the caudate head and body and anterior putamen as well as in the left anterior globus pallidus and right ventral putamen. Volume expansion was most pronounced in the posterior putamen. No volume or shape differences were revealed in girls with ADHD. Conclusions: The shape compression pattern of basal ganglia in boys with ADHD suggests that ADHD-associated deviations from typical brain development involve multiple frontal-subcortical control loops, including circuits with premotor, oculomotor, and prefrontal cortices. Further investigations employing brain-behavior analyses will help to discern the task-dependent contributions of these circuits to impaired response control that is characteristic of ADHD.
Attention deficit hyperactivity disorder (ADHD) is a prevalent neuropsychiatric syndrome characterized by excessive difficulty with inattentive and/or hyperactive-impulsive behavior. Before the era of neuroimaging, neurologists reported colocalizing motor impairments and cognitive anomalies that implicated frontal lobe subdivisions (and/or interconnected subcortical regions “in circuit” with the frontal lobe) as candidate sources of developmental delay/deviation (1) . Among these subcortical regions, the basal ganglia structures have been particularly emphasized because they are important for selecting appropriate goal-directed behavior (2) . Anomalous basal ganglia structure and function may thereby contribute to difficulty with response control that is characteristic of ADHD (3 , 4) .
With the advent of magnetic resonance imaging (MRI), investigators began using MR-based volumetric techniques to study basal ganglia structures in ADHD (5 , 6) . These studies, yielding volumetric measurements of the caudate, putamen, and globus pallidus, have produced conflicting results. Decreased right caudate volume has been reported in prior studies (7 – 9) and in a meta-analysis (10) . Reversed caudate asymmetry, i.e., not the typical right-left caudate relationship, was reported in four other publications (11 – 14) . Decreased volume of the putamen was reported once (15) , and reversed putamen asymmetry was reported once (16) . Decreased volume of the globus pallidus has been reported twice on the left (7 , 17 , 18) and twice on the right (14 , 15) . These discrepancies may reflect the underlying biological heterogeneity in ADHD, including sex-specific effects. Discrepancies may also be a consequence of limitations in anatomic imaging methods.
Investigations of basal ganglia structures have thus far been limited to examination of volumetric analysis and are prone to missing localized abnormalities. Large deformation diffeomorphic metric mapping (LDDMM) (19) is a powerful computational tool that provides detailed analysis of the morphological shape of cortical and subcortical brain regions, thereby allowing for precise examination of these structures, well beyond what has been examined in prior MRI studies of ADHD. Thus far, LDDMM has widely been used to study neurodegenerative diseases and neuropsychiatric disorders, such as Alzheimer’s disease and schizophrenia; it has revealed specific regional shape changes associated with stages of diseases (20 , 21) .
In ADHD, LDDMM offers a critical opportunity to precisely localize differences within the basal ganglia. Furthermore, it can be used to determine the nature of those differences, both in terms of direction (inward or outward deformation) and degree. In doing so, it is possible to both 1) further specify ADHD-associated differences in brain structures observed using volumetric techniques and, more important, 2) detect localized differences within brain structures that have thus far gone unrecognized (because localized effects were “washed out” in examination of total volume).
In this study, we further specify volumetric differences and use LDDMM to identify local shape differences in the basal ganglia in children with ADHD. Furthermore, we sought to examine the interaction between ADHD and sex on differences in basal ganglia volume and shape. Based on the prior findings from our study revealing multiple frontal circuit involvement at the cortical level, with reductions in both premotor and prefrontal cortical volumes (22) , we hypothesized that children with ADHD, particularly boys, would show mild reductions in basal ganglia volume, with remarkable nonuniform shape differences distributed over the basal ganglia. To achieve this, LDDMM (19 , 23 – 25) was used to map basal ganglia shapes in populations of typically developing children and children with ADHD. The outcome of LDDMM, a surface deformation map indexed over a template coordinate system of the basal ganglia, encodes variations of local shape differences of each individual subject relative to the template, which facilitates statistical inference to investigate shape differences across groups. Thus, in this article, we first report the template generation for the basal ganglia structures using a subset of our typically developing subjects. The templates were then used as references to characterize local shape variation of each individual subject using LDDMM for statistical testing on shape differences between the groups of typically developing children and children with ADHD.
Method
Subjects
Forty-seven children (27 boys, 20 girls; mean age=10.4 years) with ADHD, ages 8–13 years, and 66 age-matched, typically developing children (35 boys, 31 girls; mean age=10.5 years) were included in this study.
The diagnosis of ADHD was confirmed based on the structured parent interview, Diagnostic Interview for Children and Adolescents (DICA-IV) (26) , and an ADHD-specific broad behavior rating scale (Conners’ Parent and Teacher Rating Scales-Revised—CPRS-R, CTRS-R, long form) (27) . The CPRS-R and DICA-IV were also used to evaluate the ADHD subtype. Twenty-seven children with ADHD met criteria for ADHD combined subtype, and 20 met criteria for ADHD predominantly inattentive subtype.
Intellectual ability was assessed using the WISC-III (28) or WISC-IV (29) . Children with Full Scale IQ scores below 80 were excluded from participation.
The DICA-IV was additionally used to examine for the presence of other psychiatric disorders in all children. Children who met criteria for conduct disorder, mood disorders, generalized anxiety disorder, separation anxiety disorder, or obsessive-compulsive disorder were excluded from this study. Children with ADHD and comorbid oppositional defiant disorder (ODD) or specific phobia were included; 12 subjects with ADHD met criteria for ODD, and five children with ADHD met criteria for specific phobia (including two of the children with ODD). No subject had a history of other neurological disorders, including Tourette’s syndrome. All children were also administered the basic reading subtest from the Wechsler Individual Achievement Test (WIAT) (30) or the Word Reading subtest from the WIAT-II (31) in order to rule out a learning disability in reading. Children were excluded from participation if they demonstrated a statistically significant discrepancy between Full Scale IQ and WIAT/WIAT-II score or a basic/word reading subtest score below 85. Of the 47 children with ADHD, 28 were being treated with stimulant medication at the time of the study. For those children, medication was withheld 48 hours preceding testing. Children with ADHD taking longer acting medications were excluded.
Children were included in the typically developing comparison group only if they did not meet ADHD diagnostic criteria on any of the administered rating scales and questionnaires. All but four (meeting for specific phobia) of the typically developing children were free of criteria for psychiatric disorders on the DICA-IV. None of the typically developing children were taking psychoactive medications.
MRI Acquisition
High-resolution T 1 -weighted magnetization prepared rapid gradient recalled echo (MPRAGE) (32) images were acquired on a 1.5T Philips Gyroscan NT system (MPRAGE parameters: TR=8 msec; TE=3.76 msec; flip angle=8°; matrix=256×256; 155 partitions; field of view=260; voxel size=1×1×1.2 mm 3 ). The MRI scanner is located at the F.M. Kirby Research Center for Functional Brain Imaging at the Kennedy-Krieger Institute, Baltimore, Md.
Data Processing
Manual structural delineation
The basal ganglia structures were delineated from MR images using the MIPAV (Medical Image Processing and Visualization) program (33) . The protocols used for manual tracing of the caudate (nucleus accumbens included), putamen, and globus pallidus were adapted from previously published methods (34) . Caudate delineation was based on a protocol used in prior studies from the laboratory (17 , 35) ; putamen and globus pallidus delineation was based on a protocol developed at the University of North Carolina (http://www.psychiatry.unc.edu/).
Manual delineations used in this study were performed by two raters, both of whom were blind to diagnosis. Intraclass correlation (ICC) statistics revealed high rates of intra- and interrater reliability for the caudate (intra=0.96, inter=0.92), putamen (intra=0.98, inter=0.93) and globus pallidus (intra=0.96, inter=0.90).
Basal ganglia template generation
One of the fundamental limitations of choosing the anatomy of a single subject as a template in template-based brain mappings is the introduction of a statistical bias based on the arbitrary choice of the template anatomy. To avoid this issue in our study, we created a representative shape for each structure in the basal ganglia from a randomly selected sample of typically developing children (total 35, 18 males and 17 females) using a LDDMM template generation technique (36) . This technique involves taking the binary image masks of the structure and iteratively seeking the average shape among the population. Figure 1 illustrates the medial view of the basal ganglia template.

a The caudate (C), putamen (P), and globus pallidus (G) are respectively represented in blue, yellow, and green.
Shape Analysis via Large Deformation Diffeomorphic Metric Surface Mapping (LDDMM-Surface)
The LDDMM-surface (23 , 25) is one of the LDDMM algorithms that takes triangulated surfaces as objects and provides a diffeomorphic transformation (one-to-one, reversible smooth transformations with the property of topology preservation). It allows for the reconstitution of the variations by encoding precise variations of the boundary of anatomies relative to the template. The resultant template-based representation can be interpreted as a change of coordinates, representing anatomies in local coordinates centered at the template.
In this study, each basal ganglia structure is associated with a homogeneous binary image mask whose shape representation was reduced to a representation of scalar fields. Such fields were concentrated at the boundary of the binary image mask. We thus constructed triangulated surfaces at the boundary of each structure’s binary mask and then applied the LDDMM-surface for mapping the template to each subject’s structure. The Jacobian determinant of the deformation was computed at every location of the template coordinates for each subject and used to examine group differences (e.g., ADHD versus typically developing) in shape. A logarithmic scale of the Jacobian determinant was used to quantify surface deformation with the map encoding the local volume ratio of subject’s structure to the template. For each location (coordinate) a value of 0 indicated no deformation between the subject and template surfaces; greater or less than 0 indicated expansion or compression in the subject relative to the template. We shall call the Jacobian determinant of the deformation in the logarithmic scale a surface deformation map throughout the article.
Statistical Analysis on Shapes
A two-level hierarchical statistical test on the surface deformation maps was used to detect anatomical abnormalities of the basal ganglia in ADHD, which are described in detail in data supplement (available at http://ajp.psychiatryonline.org) material (37) . The deformation map of each individual structure was first modeled a random field in the form of

where F i(j)(x) is the deformation map of structure i in the j th subject. is the k th basis function of the Laplace-Beltrami (LB) operator on the template surface, . is deterministic for a specific structure and only dependent on the geometry of (38 , 39) . A finite number of random variables, , k =1,2, …, N i , are used to represent the deformation map. N i is determined based on the goodness of fit at a certain discrepancy level. Principal component analysis was then used to study the correlation of the shapes in the three basal ganglia structures through , k =1,2,…, N i , i ={caudate, putamen, globus pallidus}. The principal component scores were modeled using a linear regression with diagnosis and sex as independent variables after covarying for total cerebral volume and Full Scale IQ. The interaction of diagnosis and sex was also included. Diagnostic effects on the basal ganglia shapes within separate groups of boys and girls were also examined in the post hoc analysis.
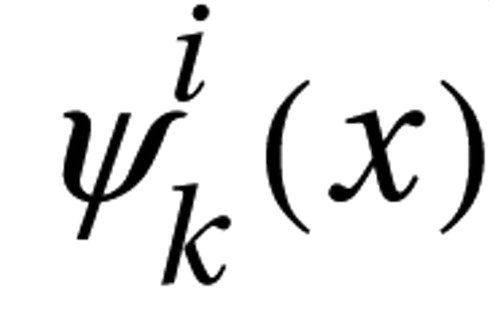


Results
Demographic Information
Demographic information for the sample is provided in Table 1 . Participants were 75% Caucasian, 18% African American, 2% Hispanic, 2% Native American, and 3% unspecified. ADHD and comparison groups did not differ significantly in sex distribution (χ 2 =0.70, df=1, p=0.64), handedness (χ 2 =0.36, df=1, p=0.32), racial distribution (χ 2 =0.49, df=4, p=0.42), or age (F=0.05, df=1, 111, p=0.82).
There was a significant group difference in Full-Scale IQ, with ADHD children having lower Full-Scale IQ (mean=109.1, SD=15.1) than children in the comparison group (mean=115.2, SD=10.6) (F=6.32, df=1, 110, p=0.01). Full-Scale IQ scores include measures of working memory and processing speed. These skills are often impaired in individuals with ADHD, selectively lowering Full-Scale IQ scores in children with ADHD (40) . For most children in this study (N=101), Full-Scale IQ was assessed using the WISC-IV, which provides for Verbal Comprehension Index (VCI) and Perceptual Reasoning Index (PRI) scores that are distinct from indices of working memory and processing speed. Thus, WISC-IV VCI and PRI scores were examined as more valid measures of intellectual reasoning in children with ADHD. There were no statistically significant differences between groups for either PRI or VCI score (PRI: F=1.11, df=1, 110, p=0.29; VCI: F=2.31, df=1, 110, p=0.13). Verbal and nonverbal intellectual reasoning were therefore felt to be equivalent in both groups. Nevertheless, to ensure the validity of our findings, Full-Scale IQ was entered as a covariate in group comparisons of the basal ganglia structures.
Total cerebral volume (TCV) for each subject was assessed using Freesurfer (41) . Consistent with prior studies (7 , 12 , 22 , 42) , the ADHD group showed decreased TCV compared to typically developing comparison subjects (ADHD, 1108 cm 3 ; typical, 1154 cm 3 , F=5.922, p<0.07 in our study). TCV was also entered as a covariate in group comparisons of the basal ganglia structures.
Basal Ganglia Volumes
Linear regressions were used to explore diagnostic and sex effects on the volume of each structure. After control was added for TCV and Full Scale IQ, analyses for effects of diagnosis (displayed in Figure 2 ) revealed smaller left basal ganglia volumes in children with ADHD compared to typically developing children in the caudate (p=0.03), putamen (p=0.03), and globus pallidus (p=0.004). For the right basal ganglia, significant differences were limited to the putamen (p<0.05) and globus pallidus (p=0.002). Significant sex effects were seen in the putamen bilaterally (both left and right, p<0.001) and globus pallidus bilaterally (both left and right, p<0.001). No significant interaction between diagnosis and sex were observed for any of the right or left basal ganglia structures.

a Asterisks and circles denote the volume measurement from the typically developing (TYP) children (35 boys and 30 girls) and children with ADHD (27 boys and 20 girls), respectively. Bars represent the mean location within each group.
To further investigate the diagnostic effects, we repeated the previous statistical testing separately for boys and girls. A significant diagnostic effect was seen for volumes of the left caudate, putamen, and globus pallidus (p<0.04, 0.004, 0.003, respectively) in boys but not girls after controlling TCV and Full-Scale IQ. In the right hemisphere, significant diagnostic effects were observed for volumes of the putamen (p=0.008) and globus pallidus (p=0.003) in boys but not girls after control was added for TCV and Full-Scale IQ.
Analyses examining differences based on ADHD subtype (controlling TCV, sex, and Full-Scale IQ) revealed smaller left basal ganglia volumes (caudate: p=0.03; putamen: p<0.02; globus pallidus: p=0.005) and smaller right putamen (p<0.05) and globus pallidus (p=0.002) in children with combined subtype ADHD compared to typically developing children. Compared with typically developing children, children with inattentive subtype ADHD showed smaller left caudate (p=0.03) and left and right globus pallidus (left: p=0.02, right: p<0.05) volumes. No group differences in the basal ganglia volumes were found in analyses comparing the combined and inattentive subtypes of ADHD.
Basal Ganglia Shapes
The surface deformation maps of the left caudate, putamen, and globus pallidus, respectively, were represented by 20, 18, and 8 Laplace-Beltrami coefficients based on the goodness of fit at a discrepancy level of 0.05. These Laplace-Beltrami coefficients were then projected to 14 principal components with 85% of total variance using PCA (see data supplement material for the variance contribution of the principal components). Linear regressions were used to test each of the principal component scores with diagnosis and sex as main factors controlling for TCV and Full-Scale IQ. Principal components reaching significance (p<0.05) are listed in columns 2–4 of Table 2 (uncorrected p value), which indicate significant effects of diagnosis, sex, and their interaction on the basal ganglia shapes.
Linear regressions further examined diagnosis effects on the basal ganglia shapes in boys and girls separately when controlling for TCV and Full-Scale IQ. Analyses did not reveal significant diagnostic effects on left basal ganglia shapes within girls. Conversely, compared with typically developing boys, boys with ADHD showed significant diagnostic effects that are characterized by the 5th, 10th, 11th, and 12th principal components (the fifth column in Table 2 ). Their visual representation is shown in Figure 3 (A and B), which reveals a multifocal pattern of significant shape differences. Compared with typically developing boys, boys with ADHD showed significant volume loss (compression) in the left medial/lateral caudate body and anterior-lateral caudate head, left dorsomedial as well as in the left anterior/middle lateral putamen and anterior-medial globus pallidus. Boys with ADHD also showed significant expansion in the left medial caudate head, medial/lateral caudate tail, medial/lateral posterior putamen, and posterior-medial globus pallidus.
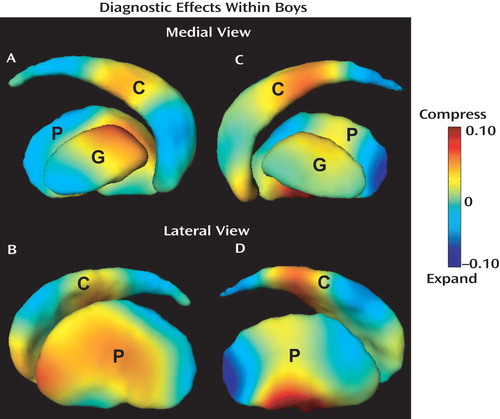
a Panels A and B illustrate the difference map of the left basal ganglia in the medial and lateral views, while panels C and D show the difference map of the right basal ganglia in the medial and lateral views. Color encodes the local volume ratio of the typically developing group to the ADHD group in the logarithmic scale and indicates the strength of the shape alteration in ADHD. Warm color denotes the regions that are compressed in the boys with ADHD when compared with the typically developing boys, while cool color depicts the regions that are expanded in the boys with ADHD relative to the typically developing boys. Key: C=caudate, P=putamen, G=globus pallidus.
The surface deformation maps of the right caudate, putamen, and globus pallidus were characterized by 19, 18, and 7 Laplace-Beltrami coefficients. Fourteen principal components were extracted to represent 85% of total variance among these Laplace-Beltrami coefficients using PCA. Linear regressions were used to test each of the principal component scores with diagnosis and sex as main factors controlling for TCV and Full-Scale IQ. Principal components reaching significance (p<0.05) are listed in columns 2–4 of Table 2 , which indicate significant effects of diagnosis, sex, and their interaction on the basal ganglia shapes. Diagnostic effects on right basal ganglia shapes were also examined within boys and girls separately. Again, our analysis did not reveal significant diagnostic effects on right basal ganglia shape in girls; however, significant effects were found in boys. The map of right basal ganglia shape alteration in the boys with ADHD, shown in Figure 3 (C and D), revealed a multifocal pattern of significant shape differences. Similar to findings for the left caudate, boys with ADHD showed compression, compared with typically developing controls, in the right medial caudate head and medial/lateral caudate body. Volume compression in ADHD boys was also seen in the right anterior-medial and medial/lateral ventral putamen. In contrast, expansion was seen in the right dorsolateral caudate body, medial/lateral caudate tail, and posterior putamen. The right globus pallidus showed relatively mild shape differences in the boys with ADHD.
Linear regressions did not reveal shape alterations in the basal ganglia in either combined or inattentive subtype of ADHD when compared with typically developing subjects, potentially due to small sample size.
Discussion
Neuroimaging studies of the basal ganglia in ADHD have previously reported findings based on volumetric analysis; in this study investigation with use of the LDDMM provided the ability to detect localized differences in shape of the basal ganglia. As a starting point, conventional volumetric measurements revealed ADHD-related anomalies in bilateral subdivisions of the basal ganglia in boys, but not girls. LDDMM methodology refined ADHD-associated differences (boys only) to a set of “compressions” (i.e., surface inward deformations) and expansions (i.e., surface outward deformation), such that LDDMM revealed multifocal shape differences of the basal ganglia in boys with ADHD when compared with typically developing boys. The shape analysis sheds light on the growing discrepancy in the literature between studies that have found differences and those that have not (7 – 10) . Findings of expansion and compression distributed over the basal ganglia surface may help to explain findings of no volume differences reported in several prior studies of ADHD (14 , 17 , 18 , 43) .
Identification of local shape differences using LDDMM may help to specify the contributions of frontal-subcortical circuits to the pathophysiology of ADHD. Recent findings generated from nonhuman primate studies using striatal injection of retrogradely transported tracer (44 – 46) , as well as those from diffusion tensor imaging (DTI) studies of humans, have provided increasingly detailed mapping of cortical-striatal projections. While the segregation of functionally relevant motor, premotor and prefrontal circuits is not complete, revealing cascading interactions at the level of the striatum (45 , 46) , the mapping provides some basis for interpreting the LDDMM findings in the context of specific frontal-subcortical circuits.
The locations in the basal ganglia showing surface inward deformation (compression deformation) in boys with ADHD included 1) bilateral anterior putamen, which is principally in circuit with rostral premotor areas (presupplementary motor area [pre-SMA], dorsal premotor area, frontal eye field, and supplementary eye field) and parietal association cortices (44 – 46) ; 2) bilateral caudate head, which is in circuit with dorsolateral prefrontal (Brodmann’s areas 9 and 46) and orbitofrontal cortices (44 – 46) ; 3) bilateral mid-caudate body, which is in circuit with rostral premotor and dorsolateral prefrontal regions (44 – 46) ; 4) right ventral putamen, in circuit with the anterior cingulate (45 , 46) ; and 5) the left anterior globus pallidus, constituting outflow from left caudate and anterior putamen and, therefore, in circuit with prefrontal and premotor projections (47 – 49) . These observations that basal ganglia regions with compression deformation in boys with ADHD fall within premotor, oculomotor, and cognitive (prefrontal) control circuits are in agreement with previous cortical findings (22 , 50) revealing that ADHD-associated structural and functional deviations from typical development are not restricted to any one frontal-striatal control loop.
Compared with typically developing boys, boys with ADHD also showed surface outward deformation (expansion deformation) in bilateral posterior putamen, left posterior globus pallidus, and right caudate tail. While not all of the implications of these ADHD-related bulges are clear, the posterior putamen findings are concordant with frontal-cortical thickness analysis of ADHD showing normal timing of maturation in the motor cortex, i.e., motor cortex “sparing” from delayed maturation, documented in the data on ADHD reported by Shaw and colleagues (50) . Although speculative, it is tempting to wonder whether the early hyperactivity of young boys with ADHD activates (and thus facilitates) the spared maturation of motor cortex and SMA, thus paradoxically outpacing the other, delayed frontal regions that typically rein in motor hyperactivity. In our samples, including boys with and without ADHD, parent ratings on the CPRS-R Hyperactivity Scale were not correlated with the expansion deformation in the left posterior putamen (Spearman’s r=0.066, p=0.69), although there was a modest tendency for a significant correlation with expansion deformation in the right posterior putamen (Spearman’s r=0.227, p=0.09). A similar pattern in the correlation was observed when these analyses were restricted to boys with ADHD (left: Spearman’s r=0.316, p=0.13; right: Spearman’s r=0.363, p=0.08). Larger sample sizes will be needed to further investigate this hypothesis.
From the above discussions, the structural shape abnormalities of the basal ganglia in boys with ADHD suggest structural and functional deviations from typical development associated with ADHD are not restricted to any one cortical-subcortical control loop. This establishes precedence for future investigations examining differing preponderances between (and within level of) parallel loops as potential neural underpinnings of the clinical (and/or etiologic) heterogeneity of children receiving the ADHD diagnosis. Findings from functional imaging indicate that task-dependent contributions of individual premotor and prefrontal circuits to response control, impairments which appear central to ADHD, depend on conditions necessary for guiding goal-directed behavior (51 – 53) . By examining correlations of brain shape abnormalities with indices of response control, specific regions and circuits contributing to the pathophysiology of ADHD can be better defined and understood.
No findings of volume and shape differences in the basal ganglia were revealed in girls with ADHD when compared with typically developing girls. This may suggest different underlying neuropathophysiologic processes in boys and girls with ADHD. Alternatively, the relative paucity of findings among girls with ADHD in cortical and subcortical structure (18) may indicate that the core neuroanatomic abnormalities lie elsewhere in girls with ADHD. There is increasing evidence that reciprocal frontal-posterior cortical projections are critical to control of intentional movement (54) and higher order executive processes (55 , 56) . It may be that abnormalities in white matter central to the pathophysiology of ADHD with downstream changes in cortical/subcortical structure are modified by X-linked or sex chromosome effects. Investigations using DTI would help to examine this hypothesis.
1. Denckla MB, Rudel RG: Anomalies of motor development in hyperactive boys. Ann Neurol 1978; 3:231–233Google Scholar
2. Shadmehr R, Krakauer JW: A computational neuroanatomy for motor control. Exp Brain Res 2008; 185:359–381Google Scholar
3. Heilman KM: A possible pathophysiologic substrate of attention deficit hyperactivity disorder. J Child Neurol 1991: (S1):S74–S79Google Scholar
4. Halperin JM, Schulz KP: Revisiting the role of the prefrontal cortex in the pathophysiology of attention-deficit/hyperactivity disorder. Psychol Bull 2006; 132:560–581Google Scholar
5. Hynd GW, Semrud-Clikeman M, Lorys A, Novey ES, Eliopulos D, Lyytinen H: Corpus callosum morphology in attention-deficit hyperactivity disorder (ADHD): morphometric analysis of MRI. J Learn Disabil 1991; 24:141–146Google Scholar
6. Singer HS: Neurobiological issues in Tourette syndrome. Brain Dev 1994; 16:353–364Google Scholar
7. Castellanos FX, Lee PP, Sharp W, Jeffries NO, Greenstein DK, Clasen LS, Blumenthal JD, James RS, Ebens CL, Walter JM, Zijdenbos A, Evans A, Giedd JN, Rapoport JL: Developmental trajectories of brain volume abnormalities in children and adolescents with attention-deficit/hyperactivity disorder. JAMA 2002; 288:1740–1748Google Scholar
8. Castellanos FX, Sharp WS, Gottesman RF, Greenstein DK, Giedd JN, Rapoport JL: Anatomic brain abnormalities in monozygotic twins discordant for attention deficit hyperactivity disorder. Am J Psychiatry 2003; 160:1693–1696Google Scholar
9. Semrud-Clikeman M, Pliszka SR, Lancaster J, Liotti M: Volumetric MRI differences in treatment-naive vs chronically treated children with ADHD. Neurology 2006; 67:1023–1027Google Scholar
10. Makris N, Biederman J, Valera EM, Bush G, Kaiser J, Kennedy DN, Caviness VS, Faraone SV, Seidman LJ: Cortical thinning of the attention and executive function networks in adults with attention-deficit/hyperactivity disorder. Cereb Cortex 2007; 17:1364–1375Google Scholar
11. Frick PJ, Lahey BB, Applegate B, Kerdyck L, Ollendick T, Hynd GW, Garfinkel B, Greenhill L, Biederman J, Barkley RA, et al: DSM-IV field trials for the disruptive behavior disorders: symptom utility estimates. J Am Acad Child Adolesc Psychiatry 1994; 33:529–539Google Scholar
12. Filipek PA, Semrud-Clikeman M, Steingard RJ, Renshaw PF, Kennedy DN, Biederman J: Volumetric MRI analysis comparing subjects having attention-deficit hyperactivity disorder with normal controls. Neurology 1997; 48:589–601Google Scholar
13. Semrud-Clikeman M, Steingard RJ, Filipek P, Biederman J, Bekken K, Renshaw PF: Using MRI to examine brain-behavior relationships in males with attention deficit disorder with hyperactivity. J Am Acad Child Adolesc Psychiatry 2000; 39:477–484Google Scholar
14. Castellanos FX, Geidd JN, Marsh WL, Hamburger SD, Vaituzis AC, Dickstein DP, Sarfatti SE, Vauss YC, Snell JW, Lange N, Kaysen D, Krain AL, Ritchie GF, Rajapakse JC, Rapoport JL: Quantitative brain magnetic resonance imaging in attention-deficit hyperactivity disorder. Arch Gen Psychiatry 1996; 53:607–616Google Scholar
15. Overmeyer S, Bullmore ET, Suckling J, Simmons A, Williams SC, Santosh PJ, Taylor E: Distributed grey and white matter deficits in hyperkinetic disorder: MRI evidence for anatomical abnormality in an attentional network. Psychol Med 2001; 31:1425–1435Google Scholar
16. Wellington TM, Semrud-Clikeman M, Gregory AL, Murphy JM, Lancaster JL: Magnetic resonance imaging volumetric analysis of the putamen in children with ADHD: combined type versus control. J Attention Disord 2006; 10:171–180Google Scholar
17. Aylward EH, Reiss AL, Reader MJ, Singer HS, Brown JE, Denckla MB: Basal ganglia volumes in children with attention-deficit hyperactivity disorder. J Child Neurol 1996; 11:112–115Google Scholar
18. Castellanos FX, Giedd JN, Berquin PC, Walter JM, Sharp W, Tran T, Vaituzis AC, Blumenthal JD, Nelson J, Bastain TM, Zijdenbos A, Evans AC, Rapoport JL: Quantitative brain magnetic resonance imaging in girls with attention-deficit/hyperactivity disorder. Arch Gen Psychiatry 2001; 58:289–295Google Scholar
19. Beg MF, Miller MI, Trouve A, Younes L: Computing large deformation metric mapping via geodesic flows of diffeomorphisms. Int J Computer Vision 2005; 61:139–157Google Scholar
20. Qiu A, Younes L, Miller MI, Csernansky JG: Parallel transport in diffeomorphisms distinguishes the time-dependent pattern of hippocampal surface deformation due to healthy aging and the dementia of the Alzheimer’s type. Neuroimage 2008; 40:68–76Google Scholar
21. Qiu A, Younes L, Wang L, Ratnanather JT, Gillepsie SK, Kaplan G, Csernansky J, Miller MI: Combining anatomical manifold information via diffeomorphic metric mappings for studying cortical thinning of the cingulate gyrus in schizophrenia. Neuroimage 2007; 37:821–833Google Scholar
22. Mostofsky SH, Cooper KL, Kates WR, Denckla MB, Kaufmann WE: Smaller prefrontal and premotor volumes in boys with ADHD. Biol Psychiatry 2002; 52:785–794Google Scholar
23. Vaillant M, Glaunes J: Surface matching via currents. Inf Process Med Imaging 2005; 19:381–392Google Scholar
24. Qiu A, Miller MI: Cortical hemisphere registration via large deformation diffeomorphic metric curve mapping. Med Image Comput Assist Interv Int Conf 2007; 10(Pt 1):186–193Google Scholar
25. Vaillant M, Qiu A, Glaunes J, Miller MI: Diffeomorphic metric surface mapping in subregion of the superior temporal gyrus. Neuroimage 2007; 34:1149–1159Google Scholar
26. Reich W: Diagnostic Interview for Children and Adolescents (DICA). J Am Acad Child Adolesc Psychiatry 2000; 39:59–66Google Scholar
27. Conners CK: Conners’ Rating Scales—Revised. North Tonawanda, NY, Multi-Health Systems Inc, 1997Google Scholar
28. Wechsler D: Wechsler Intelligence Scale for Children-III. San Antonio, Tex, Psychological Corp, 1991Google Scholar
29. Wechsler DL: Wechsler Intelligence Scale for Children, 4th ed. San Antonio, Tex, Psychological Corp, 2003Google Scholar
30. Wechsler DL: Wechsler Individual Achievement Test. San Antonio, Tex, Psychological Corp, 1992Google Scholar
31. Wechsler DL: Wechsler Individual Achievement Test-II. San Antonio, Tex, Psychological Corp, 2002Google Scholar
32. Mugler JP III, Brookeman JR: Three-dimensional magnetization-prepared rapid gradient-echo imaging (3D MP RAGE). Magn Reson Med 1990; 15:152–157Google Scholar
33. McAuliffe M, Lalonde E, McGarry D, Gandler W, Csaky K, Trus B: Medical image processing, analysis and visualization in clinical research. Proceedings of the 14th IEEE symposium on computer-based medical systems (CBMS2001) 2001:381–386Google Scholar
34. Sears LL, Vest C, Mohamed S, Bailey J, Ranson BJ, Piven J: An MRI study of the basal ganglia in autism. Prog Neuropsychopharmacol Biol Psychiatry 1999; 23:613–624Google Scholar
35. Singer HS, Reiss AL, Brown JE, Aylward EH, Shih B, Chee E, Harris EL, Reader MJ, Chase GA, Bryan RN, Denckla MB: Volumetric MRI changes in basal ganglia of children with Tourette’s syndrome. Neurology 1993; 43:950–956Google Scholar
36. Qiu A, Brown TL, Fischl B, Kolasny A, Ma J, Buckner RL, Miller M: Subcortical structure template generation with its applications in shape analysis. Neuroimage 2008 (in press)Google Scholar
37. Qiu A, Miller MI: Multi-structure network shape analysis via normal surface momentum maps. Neuroimage 2008; 42:1430–1438Google Scholar
38. Qiu A, Bitouk D, Miller MI: Smooth functional and structural maps on the neocortex via orthonormal bases of the Laplace-Beltrami operator. IEEE Trans Med Imaging 2006; 25:1296–1306Google Scholar
39. Qiu A, Younes L, Miller MI: Intrinsic and extrinsic analysis in computational anatomy. Neuroimage 2008; 39:1803–1814Google Scholar
40. Mayes SD, Calhoun SL: WISC-IV and WISC-III profiles in children with ADHD. J Attention Disord 2006; 9:486–493Google Scholar
41. Fischl B, Salat DH, Busa E, Albert M, Dieterich M, Haselgrove C, van der Kouwe A, Killiany R, Kennedy D, Klaveness S, Montillo A, Makris N, Rosen B, Dale AM: Whole brain segmentation: automated labeling of neuroanatomical structures in the human brain. Neuron 2002; 33:341–355Google Scholar
42. Wolosin SM, Richardson ME, Hennessey JG, Denckla MB, Mostofsky SH: Abnormal cerebral cortex structure in children with ADHD. Hum Brain Mapp 2007 (Epub ahead of print)Google Scholar
43. Hill DE, Yeo RA, Campbell RA, Hart B, Vigil J, Brooks W: Magnetic resonance imaging correlates of attention-deficit/hyperactivity disorder in children. Neuropsychology 2003; 17:496–506Google Scholar
44. Akkal D, Dum RP, Strick PL: Supplementary motor area and presupplementary motor area: targets of basal ganglia and cerebellar output. J Neurosci 2007; 27:10659–10673Google Scholar
45. Haber SN: The primate basal ganglia: parallel and integrative networks. J Chem Neuroanat 2003; 26:317–330Google Scholar
46. Calzavara R, Mailly P, Haber SN: Relationship between the corticostriatal terminals from areas 9 and 46, and those from area 8A, dorsal and rostral premotor cortex and area 24c: an anatomical substrate for cognition to action. Eur J Neurosci 2007; 26:2005–2024Google Scholar
47. Middleton FA, Strick PL: A revised neuroanatomy of frontal-subcortical circuits, in Frontal-Subcortical Circuits in Psychiatric and Neurological Disorders. Edited by Lichter DG, Cummings JL. New York, Guilford, 2001, pp 44–58Google Scholar
48. Parent M, Parent A: Single-axon tracing study of corticostriatal projections arising from primary motor cortex in primates. J Comp Neurol 2006; 496:202–213Google Scholar
49. Lehericy S, Ducros M, Krainik A, Francois C, Van de Moortele PF, Ugurbil K, Kim DS: 3-D diffusion tensor axonal tracking shows distinct SMA and pre-SMA projections to the human striatum. Cereb Cortex 2004; 14:1302–1309Google Scholar
50. Shaw P, Eckstrand K, Sharp W, Blumenthal J, Lerch JP, Greenstein D, Clasen L, Evans A, Giedd J, Rapoport JL: Attention-deficit/hyperactivity disorder is characterized by a delay in cortical maturation. Proc Natl Acad Sci U S A 2007 104(49):19649–19654Google Scholar
51. Mostofsky SH, Schafer JG, Abrams MT, Goldberg MC, Flower AA, Boyce A, Courtney SM, Calhoun VD, Kraut MA, Denckla MB, Pekar JJ: FMRI evidence that the neural basis of response inhibition is task-dependent. Brain Res Cogn Brain Res 2003; 17:419–430Google Scholar
52. Simmonds DJ, Fotedar SG, Suskauer SJ, Pekar JJ, Denckla MB, Mostofsky SH: Functional brain correlates of response time variability in children. Neuropsychologia 2007; 45:2147–2157Google Scholar
53. Mostofsky SH, Simmonds DJ: Response inhibition and response selection: two sides of the same coin. J Cogn Neurosci 2008 (in press)Google Scholar
54. Rizzolatti G, Fogassi L, Gallese V: Neurophysiological mechanisms underlying the understanding and imitation of action. Nat Rev Neurosci 2001; 2:661–670Google Scholar
55. Gazzaley A, Cooney JW, McEvoy K, Knight RT, D’Esposito M: Top-down enhancement and suppression of the magnitude and speed of neural activity. J Cogn Neurosci 2005; 17:507–517Google Scholar
56. Schumacher EH, Cole MW, D’Esposito M: Selection and maintenance of stimulus-response rules during preparation and performance of a spatial choice-reaction task. Brain Res 2007; 1136:77–87Google Scholar