Genetic Associations Between Stress-Related Disorders and Autoimmune Disease
Abstract
Objective:
Emerging evidence supports a bidirectional phenotypic association between stress-related disorders and autoimmune disease. However, the biological underpinnings remain unclear. Here, the authors examined whether and how shared genetics contribute to the observed phenotypic associations.
Methods:
Based on data from 4,123,631 individuals identified from Swedish nationwide registers, familial coaggregation of stress-related disorders (any disorder or posttraumatic stress disorder [PTSD]) and autoimmune disease were initially estimated in seven cohorts with different degrees of kinship. Polygenic risk score (PRS) analyses were then performed with individual-level genotyping data from 376,871 participants in the UK Biobank study. Finally, genetic correlation analyses and enrichment analyses were performed with genome-wide association study (GWAS) summary statistics.
Results:
Familial coaggregation analyses revealed decreasing odds of concurrence of stress-related disorders and autoimmune disease with descending kinship or genetic relatedness between pairs of relatives; adjusted odds ratios were 1.51 (95% CI=1.09–2.07), 1.28 (95% CI=0.97–1.68), 1.16 (95% CI=1.14–1.18), and 1.01 (95% CI=0.98–1.03) for monozygotic twins, dizygotic twins, full siblings, and half cousins, respectively. Statistically significant positive associations were observed between PRSs of stress-related disorders and autoimmune disease, as well as between PRSs of autoimmune disease and stress-related disorders. GWAS summary statistics revealed a genetic correlation of 0.26 (95% CI=0.14–0.38) between these two phenotypes and identified 10 common genes and five shared functional modules, including one module related to G-protein–coupled receptor pathways. Similar analyses performed for PTSD and specific autoimmune diseases (e.g., autoimmune thyroid disease) largely recapitulated the results of the main analyses.
Conclusions:
This study demonstrated familial coaggregation, genetic correlation, and common biological pathways between stress-related disorders and autoimmune disease.
The evidence for a bidirectional phenotypic association between stress-related disorders, including posttraumatic stress disorder (PTSD), and autoimmune disease has accumulated over recent decades (1–3). Epidemiological studies based on large-scale prospective cohorts suggest that individuals suffering severe stress reactions, such as those who have experienced trauma and patients with a diagnosis of a stress-related disorder (3–5), might have an increased subsequent risk of developing various autoimmune diseases, such as Addison’s disease, Guillain-Barré syndrome, and IgA nephropathy (1, 2, 6, 7). Likewise, individuals with autoimmune disease show a higher susceptibility to psychological stress (8) and psychiatric disorders such as depression and PTSD than those without such conditions (9–11). This psychological distress can in turn lead to disease exacerbation, demonstrated as, for instance, delayed lesion clearance in patients with psoriasis (12). Therefore, understanding the role of shared risk factors contributing to the bidirectional phenotypic associations between stress-related disorders and autoimmune disease is important for understanding the etiologies of both diseases and for developing novel interventions to improve outcomes among these patient groups.
Stress-related disorders are associated with disruption of the hypothalamic-pituitary-adrenal axis (13) that might be accompanied by immune dysfunction, such as amplified proinflammatory cytokine production (3). The exact temporal relationships are, however, unknown due to the complex interactions among these events. In addition, abnormal inflammatory responses have been observed in many autoimmune diseases, such as rheumatoid arthritis and systemic lupus erythematosus (14, 15), which might be induced by chronic activation of innate and adaptive immunity (14). These findings suggest that stress-related disorders and autoimmune disease might involve common biological pathways. Although scarce, the existing literature provides suggestive data for a role of genetic factors for psychiatric disorders in the development of autoimmune disease. Genome-wide association studies (GWASs) have found evidence of genetic pleiotropy between PTSD and rheumatoid arthritis and psoriasis (16). In addition, at the level of gene expression, schizophrenia (a psychiatric disorder with high genetic overlap with PTSD [17]) was found to cluster with immunological diseases using enrichment correlation analyses (18). There remains, however, a clear need to advance current understanding of the mechanisms linking stress-related disorders and autoimmune disease.
Leveraging population-based family data from Sweden, individual genotyping data from the UK Biobank study, and GWAS summary statistics, we aimed to perform, for the first time, a comprehensive assessment of genetic associations of stress-related disorders with autoimmune disease to identify specific pathways linking these two phenotypes. Specifically, we examined associations with stress-related disorders as a single category of disorders related to trauma and stressful life events and examined associations with PTSD—the most severe and clearly defined phenotype among stress-related disorders—separately.
Methods
Study Design
The study contained two main parts (Figure 1). First, to answer the question of whether stress-related disorders (any disorder or PTSD) share genetic liability with autoimmune disease, we performed a familial coaggregation analysis, using family data from Swedish national registers; we examined how the odds of having the two diseases in a pair of relatives varied with familial relatedness. Then, using individual-level genotyping data from approximately 0.5 million UK Biobank study participants, we measured the genetic associations between stress-related disorders and autoimmune disease (any disease and the six most common subtypes) by polygenic risk score (PRS) analysis; we tested whether genetic predisposition to stress-related disorders was associated with the risk of autoimmune disease and vice versa. Second, we aimed to identify common genes or pathways potentially underlying the susceptibility to both stress-related disorders (any disorder or PTSD) and autoimmune disease. We used gene mapping, annotation, and enrichment analyses based on GWAS summary statistics for these two phenotypes. Summary statistics for stress-related disorders as a whole and for PTSD specifically were released by the Lundbeck Foundation Initiative for Integrative Psychiatric Research (iPSYCH) (19) and the Psychiatric Genomics Consortium (20), respectively (see Table S1 in the online supplement). However, because no GWAS has considered all autoimmune diseases as a single phenotypic category, we used genotyping data from the UK Biobank study to generate the summary statistics. We validated the UK Biobank–based analysis by using the publicly available GWAS summary statistics for several specific autoimmune diseases (see Table S1 in the online supplement). Detailed information about the data sources is presented in the Supplementary Methods section in the online supplement. This study was approved by the regional ethics review board in Stockholm (Dnr 2013/862-31/5), the NHS National Research Ethics Service (16/NW/0274), and the biomedical research ethics committee of West China Hospital (2019-1171).

FIGURE 1. Components of the study designa
aThe study includes two components: using data from registers to determine shared genetic liability between stress-related disorders and autoimmune disease risk and using genome-wide association study (GWAS) summary statistics to identify common genes or pathways.
Statistical Analysis
Familial coaggregation analyses using family data from Swedish registers.
We included all individuals born in Sweden between 1953 and 1993 according to Swedish Population and Housing censuses. Individual follow-up data through December 31, 2013, were obtained through cross-linkage to the Swedish National Patient Register, the Causes of Death Register, and the Migration Register. Among 4,423,174 Swedes, we excluded individuals who died or emigrated before the age of 20 and those whose biological parents were unidentifiable, leaving 4,123,631 eligible individuals; we compiled seven kinship cohorts according to relatedness among these individuals, namely, monozygotic twins, dizygotic twins, normal full siblings, maternal half siblings, paternal half siblings, cousins, and half cousins, according to the Swedish Multi-Generation Register and the Twin Register (see Figure S1 in the online supplement).
We defined stress-related disorders by clinical diagnoses of PTSD, acute stress disorder, adjustment disorder, and other stress reactions, according to the Swedish editions of ICD-9 and ICD-10 (codes 308 and 309 in ICD-9 and code F43 in ICD-10) (see Table S2 in the online supplement); these data were obtained from the National Patient Register, which includes data on inpatient (since 1987) and specialized outpatient (since 2001) hospital visits in Sweden. The data on autoimmune diseases were also from the National Patient Register; we considered 36 specific autoimmune diseases (21) by using their corresponding ICD codes (see Table S2 in the online supplement), as in our previous study (6). Sex and birth year, obtained from the Multi-Generation Register, were used as covariates in these analyses.
We used logistic regression models to measure associations between stress-related disorders (any disorder or PTSD) and autoimmune disease in the individual-level and within-relative-pair analyses, adjusting for sex and birth year. In the individual-level analyses, we compared the odds of having an autoimmune disease among individuals with any stress-related disorders or PTSD compared with the odds among individuals without such a condition. The within-relative-pair analyses were performed for the seven types of kinships mentioned above; we estimated odds ratios (with 95% confidence intervals) of having an autoimmune disease by comparing individuals who had a relative with any stress-related disorder or PTSD with individuals who did not have a relative with any stress-related disorder or PTSD. The genetic relatedness of the relatives was considered in descending order: monozygotic twins are genetically identical, dizygotic twins and full siblings share on average 50% of their segregating alleles, and half siblings, cousins, and half cousins share on average 25%, 12.5%, and 6.25% of their segregating alleles, respectively.
Phenotypic association analyses with the UK Biobank data.
After exclusion of participants who had withdrawn from the UK Biobank (N=19), 502,488 eligible individuals were included in the analyses to estimate phenotypic associations between stress-related disorders and autoimmune disease (see Figure S2 in the online supplement).
We identified stress-related disorders (any disorder or PTSD) by diagnoses in the UK Biobank inpatient hospital data (using ICD codes) and primary care data (using Read v2/v3 codes) (see Table S3 in the online supplement); the presence of autoimmune disease was based on the UK Biobank inpatient hospital data (using ICD codes). We studied 36 specific autoimmune diseases, as in the above familial coaggregation analyses (see Table S2 in the online supplement). Covariates, including sex, birth year, sociodemographic factors (race/ethnicity, Townsend deprivation index, educational attainment, and annual household income), and lifestyle factors (body mass index, drinking status, and smoking status), were collected at baseline through questionnaires.
Logistic regression models were used to estimate phenotypic associations between any stress-related disorder or PTSD and autoimmune disease (any disease, as well as the six most common types, including autoimmune thyroid disease, rheumatoid arthritis, ulcerative colitis, psoriasis, insulin-dependent diabetes mellitus, and giant cell arteritis), adjusting for the covariates mentioned above. The analysis was repeated with data obtained from White British UK Biobank participants alone (N=409,601).
PRS analyses with the UK Biobank data.
Before polygenic risk profiling, we first performed a standard GWAS quality control procedure on genotyping data from 408,885 White British participants (22), leaving 376,871 participants for further analyses (see the Supplementary Methods section in the online supplement).
To generate PRSs for stress-related disorders, we restricted the analysis to single-nucleotide polymorphisms (SNPs) available in both the base data set (i.e., GWAS summary statistics of stress-related disorders from iPSYCH [19]) and the target data set (i.e., UK Biobank genotyping data that passed the quality control assessment) (see Figure S3 in the online supplement). We further excluded variants with ambiguous strands and those meeting a linkage disequilibrium (LD) threshold of r2>0.1 in the 250-kb window, leaving 196,130 SNPs with relative independence for the PRS calculation. PRSs were computed as the sum of SNP dosages weighted by the allele effect across all SNPs in the target data set under p thresholds of 5×10–8, 1×10–6, 1×10–4, 1×10–3, 0.01, 0.05, 0.1, 0.2, 0.3, 0.4, and 0.5. PLINK (version 1.9) was used for the polygenic risk profiling. In a validation step, we observed statistically significant associations between standardized PRSs for stress-related disorders and clinical diagnoses of stress-related disorders in the UK Biobank population using logistic regression models (see Table S4 in the online supplement), adjusting for sex, birth year, genotyping array, and the first five principal components. The calculation of PRSs for PTSD was not feasible because there were no publicly available data from a well-powered GWAS for PTSD using independent European-ancestry samples (i.e., without UK Biobank participants) (20).
To perform a GWAS and generate PRSs for autoimmune disease as a single group (i.e., any autoimmune disease), the available genotyping data from the UK Biobank study were randomly split into a base data set (N=184,028) and a target data set (N=192,843) (see Figure S3 in the online supplement). To avoid the influence of the phenotypic association (i.e., the association between stress-related disorders and autoimmune disease) on the identification of genetic profiles related to autoimmune disease, individuals with stress-related disorders were excluded in the generation of GWAS summary statistics for autoimmune disease. The PRS calculation was based on 293,355 SNPs with relative independence and was performed following steps similar to those described above (see Table S5 in the online supplement).
We then tested the associations between the calculated PRSs for stress-related disorders and risk of autoimmune disease and vice versa by using logistic regression models in R (version 3.4.3), adjusting for sex, birth year, genotyping array, and the first five principal components. The estimates derived from the model with the highest R-squared value (i.e., a measurement of the largest variance explained by another trait) are reported (see Figure S4 in the online supplement).
We repeated the same steps (i.e., GWAS and PRS analyses) for the six most common autoimmune diseases (see Figures S5–S7 and Tables S6–S11 in the online supplement). Satisfactory agreement was shown between our GWAS results (based on the UK Biobank data) and publicly available GWAS summary statistics for these diseases (e.g., autoimmune thyroid disease [23], rheumatoid arthritis [24], ulcerative colitis [25], and insulin-dependent diabetes mellitus [26]) (see Table S1 in the online supplement) based on LD score regression (27) (see details in the Supplementary Methods section in the online supplement) and based on a comparison of the top SNPs (see Figure S8 in the online supplement), with genetic correlations (rg) ranging from 0.52 to 1.11, and overlap in the top SNPs, which ranged from 10.00% to 60.34% (see Tables S12–S15 in the online supplement).
Gene mapping and enrichment analyses using GWAS summary statistics.
First, LD score regression was applied to verify the existence of genetic correlations between stress-related disorders (any disorder or PTSD) and autoimmune disease based on aggregate-level genetic data (i.e., summary statistics). By using the same GWAS summary statistics as those mentioned above, we identified lead SNPs and mapped risk genes for stress-related disorders (p thresholds <1×10–5, LD r2<0.1), PTSD (p thresholds <1×10–5, LD r2<0.1), and autoimmune disease (p thresholds <5×10–8, LD r2<0.1) separately, using FUMA (http://fuma.ctglab.nl/) (28). The strategies for gene mapping included positional mapping, expression quantitative trait loci mapping based on the GTEx v8 Project (29), and chromatin interaction mapping (30, 31). The common risk genes for any stress-related disorder and autoimmune disease and those for PTSD and autoimmune disease were identified and annotated with a short description of functional relevance, based on the Gene Ontology database. Statistical significance of the number of shared genes was tested using a hypergeometric test. The genetic overlap of these two phenotypes at the level of pathways was subsequently explored using the protein-protein interaction (PPI) network analysis in Metascape, a web-based bioinformatics resource for comprehensive gene annotation and analysis (https://metascape.org/) (32). In addition to using GWAS summary statistics based on UK Biobank for any autoimmune disease, we performed similar analyses using published GWAS summary statistics for autoimmune thyroid disease (the most common type of autoimmune disease in the UK Biobank) (20, 23).
Results
Analyses Using Swedish Family Data
Individuals with any stress-related disorder were at higher risk of autoimmune disease compared with individuals without a stress-related disorder (odds ratio=1.66, 95% CI=1.63–1.69) (Figure 2). In the familial coaggregation analysis, the odds of having both diseases in a pair of relatives decreased with descending kinship or genetic relatedness of the pair (Figure 2). The highest odds ratio, 1.51 (95% CI=1.09–2.07) was found for monozygotic twins, followed by 1.28 (95% CI=0.97–1.68) for dizygotic twins and 1.16 (95% CI=1.14–1.18) for full siblings. There was no excess risk for half cousins (odds ratio=1.01, 95% CI=0.98–1.03). Although there was a smaller number of PTSD patients (i.e., those with PTSD accounted for 6.2% of all individuals with a stress-related disorder based on the Swedish National Patient Register) (see Table S16 in the online supplement) and thereby limited statistical power, we obtained an identical pattern of results in the analyses for PTSD and autoimmune disease (Figure 2).

FIGURE 2. Phenotypic associations between stress-related disorders (any disorder or posttraumatic stress disorder) and autoimmune disease, based on family data in Swedena
aOdds ratios and 95% confidence intervals were obtained from the individual-level analysis performed among all eligible participants and from the within-relative-pair analysis performed in the cohorts of different kinships, adjusted for birth year and sex. The numbers of observations for each cohort are as follows: 4,123,631 in the within-individual analysis; 12,718 in the analysis of monozygotic twins; 20,374 in the analysis of dizygotic twins; 5,246,854 in the analysis of full siblings; 700,488 in the analysis of maternal half siblings; 879,724 in the analysis of paternal half siblings; 17,371,852 in the analysis of cousins; and 1,811,878 in the analysis of half cousins.
Analyses Using the UK Biobank Data
We found odds ratios of 1.28 (95% CI=1.22–1.35) and 2.13 (95% CI=1.85–2.45) for autoimmune disease among individuals with a diagnosis of any stress-related disorder (Figure 3) and with an inpatient diagnosis of any stress-related disorder (see Figure S9 in the online supplement). The corresponding odds ratios for individuals with any PTSD and inpatient PTSD were 1.79 (95% CI=1.52–2.11) (Figure 3) and 2.35 (95% CI=1.90–2.91) (see Figure S9 in the online supplement), respectively. We obtained comparable estimates across autoimmune diseases, and these estimates remained largely identical when the analysis was restricted to White British participants (Figure 3; see Figure S9 in the online supplement).
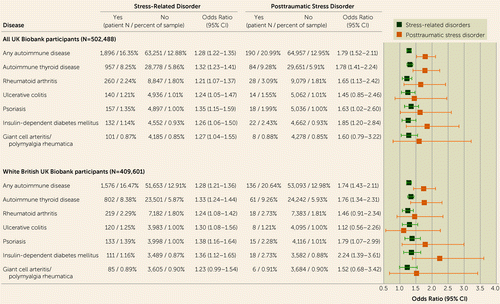
FIGURE 3. Associations between stress-related disorders (any disorder or posttraumatic stress disorder) and autoimmune diseasea
aOdds ratios and 95% confidence intervals were estimated by logistic regression model, adjusting for sex, birth year, sociodemographic factors (race/ethnicity, Townsend deprivation index, educational attainment, and annual household income), and lifestyle factors (body mass index, drinking status, and smoking status). N=number of individuals (patients and those without a stress-related disorder) with the corresponding autoimmune disease.
The PRS analyses demonstrated a contribution of genetic components to the observed phenotypic association: there was an association between the PRS for stress-related disorders and the risk of autoimmune disease (at pthreshold<0.3, odds ratio=1.05, 95% CI=1.04–1.06, p=3.23×10–24), as well as associations between the PRS for autoimmune disease and the risk of a diagnosis of any stress-related disorder (at pthreshold<0.3, odds ratio=1.06, 95% CI=1.04–1.08, p=5.36×10–8) and an inpatient diagnosis of any stress-related disorder (odds ratio=1.13, 95% CI=1.05–1.21, p=2.88×10–3) (Table 1). In the analyses of specific autoimmune diseases, although we obtained consistently statistically significant odds ratios for the associations between PRSs for stress-related disorders and risk of autoimmune disease (ranging from 1.03 [95% CI=1.00–1.07] to 1.08 [95% CI=1.04–1.12]) (Table 1), the associations between PRSs for specific autoimmune diseases and the risk of stress-related disorders were somewhat attenuated. We obtained similar patterns of results when the PRSs of specific autoimmune diseases were calculated based on publicly available GWAS summary statistics (see Table S17 in the online supplement).
PRS for Stress-Related Disorder and Risk of Autoimmune Disease | PRS for Autoimmune Disease and Risk of Any Stress-Related Disorder | PRS for Autoimmune Disease and Risk of Inpatient Stress-Related Disorder | |||||||
---|---|---|---|---|---|---|---|---|---|
Disease | Odds Ratio | 95% CI | p | Odds Ratio | 95% CI | p | Odds Ratio | 95% CI | p |
Autoimmune disease | 1.05 | 1.04–1.06 | 3.23×10–24 | 1.06 | 1.04–1.08 | 5.36×10–8 | 1.13 | 1.05–1.21 | 2.88×10–3 |
Specific disease types | |||||||||
Autoimmune thyroid disease | 1.05 | 1.03–1.06 | 1.37×10–11 | 1.04 | 1.02–1.07 | 9.94×10–5 | 1.1 | 1.02–1.17 | 9.11×10–3 |
Rheumatoid arthritis | 1.06 | 1.03–1.09 | 2.07×10–6 | 1.04 | 1.02–1.06 | 8.71×10–4 | 0.98 | 0.91–1.04 | 0.471 |
Ulcerative colitis | 1.03 | 1.00–1.07 | 0.051 | 0.98 | 0.96–1.00 | 0.108 | 0.94 | 0.88–1.01 | 0.097 |
Psoriasis | 1.06 | 1.02–1.09 | 1.07×10–3 | 1.04 | 1.02–1.06 | 3.02×10–4 | 1.02 | 0.96–1.10 | 0.49 |
Insulin-dependent diabetes mellitus | 1.08 | 1.04–1.12 | 1.52×10–5 | 0.98 | 0.96–1.00 | 0.127 | 0.98 | 0.91–1.05 | 0.529 |
Giant cell arteritis | 1.05 | 1.02–1.09 | 2.75×10–3 | 1.02 | 1.00–1.05 | 0.038 | 0.97 | 0.90–1.04 | 0.352 |
TABLE 1. Genetic associations between stress-related disorders and autoimmune diseasea
Identification of Shared Genes and Pathways
The degree of pleiotropy or genetic overlap between stress-related disorders and autoimmune disease was estimated using cross-trait LD score regression, and a coefficient (rg) of 0.26 (95% CI=0.14–0.38, p=1.30×10–5) corroborated a positive genetic correlation between the two phenotypes (Figure 4A). We merged 635 FUMA-annotated risk genes of stress-related disorders and 989 risk genes of autoimmune disease and identified 10 common genes (hypergeometric test: p=4.51×10–6) (Figure 4B) that mainly included genes relevant to the regulation of a variety of cytokine signaling–related biological processes and immune-related disease. The PPI network analysis detected five densely connected regions, that is, molecular complex detection (MCODE) components, that were assembled by both genes associated with stress-related disorders and those associated with autoimmune disease (Figure 4C). The five MCODE components were marked as components relevant to pathways of “signaling by G proteins/G protein coupled receptors (GPCRs),” “cilium assembly,” “membrane trafficking,” “eukaryotic translation initiation,” and “cell cycle,” according to the putative biological roles annotated by the functional enrichment analysis applied to each complex (the top three enriched pathways of each complex are shown in Table S18 in the online supplement).
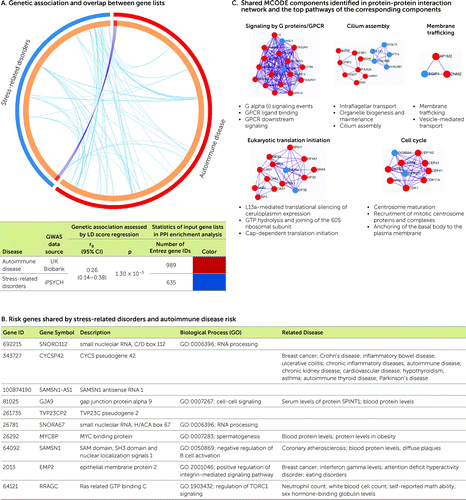
FIGURE 4. Identification of genes and pathways shared by stress-related disorders and autoimmune diseasea
aPanel A shows the genetic associations and overlap between gene lists. Each candidate gene is assigned to one spot on the arc of the corresponding gene lists. Dark orange represents the genes that appear in both lists and light orange represents genes that are unique to that gene list. Purple curves link identical genes, and blue curves link genes that have different identities but share an enriched pathway or process (i.e., they represent the functional overlaps between gene lists). Panel B shows the risk genes shared by stress-related disorders and autoimmune disease. The short description for identical genes were annotated using the Metascape tool, and the related diseases are reported based on the GWAS Catalog. Panel C shows the shared MCODE components identified in protein-protein interaction network and the top pathways of the corresponding components. GWAS=genome-wide association study; MCODE=Molecular Complex Detection algorithm; rg=genetic correlation.
The gene mapping and PPI network analyses for autoimmune thyroid disease recapitulated the above findings; these analyses showed a genetic correlation between stress-related disorders and autoimmune thyroid disease (rg=0.14, 95% CI=0.03–0.25, p=0.01) (see Figure S10 in the online supplement) and identified three of the aforementioned shared MCODE components, including those relevant to “signaling by G proteins/GPCRs,” “cilium assembly,” and “membrane trafficking” (see Figure S10 and Table S19 in the online supplement).
The analyses for PTSD, using the summary statistics from the largest GWAS of PTSD (23,212 PTSD cases and 151,447 controls) (20), also recapitulated the above results; the analyses demonstrated a similar genetic correlation in LD regression (autoimmune disease: rg=0.39, 95% CI=0.24–0.54, p=2.79×10–7; autoimmune thyroid disease: rg=0.22, 95% CI=0.10–0.35, p=5.00×10–5) (see Figures S11–S12 in the online supplement) and identified several identical shared genes and pathways, including the “signaling by G proteins/GPCRs” pathway, in gene mapping and PPI network analyses (see Figures S11–S12 and Tables S20–S23 in the online supplement).
Discussion
To our knowledge, this is the first comprehensive assessment of the genetic associations between stress-related disorders and autoimmune disease. The existence of genetic overlap between stress-related disorders and autoimmune disease was shown in both familial coaggregation analyses (i.e., a decreasing concurrence of these diseases with descending kinship or genetic relatedness of pairs of relatives) and PRS analyses based on individual-level genotyping data (i.e., a genetic association between these diseases). Furthermore, using GWAS summary statistics, we identified 10 genes and five functional modules shared between these two phenotypes. Because all of these main findings were confirmed when the analysis was focused on PTSD, which is the most severe and well-defined subtype of stress-related disorders, our results highlight potential biological mechanisms that may partially explain the observed phenotypic associations between stress-related disorders and autoimmune disease.
Epidemiological studies have consistently reported an increased risk of autoimmune disease, any type and specific types, among patients with stress-related disorders, particularly PTSD, using national register data (6) and cohort studies of veterans (7), female nurses (33), and traumatized individuals (34). Moreover, in addition to the documented association with disease onset, the role of stressful life events in the exacerbation or relapse of autoimmune disease, such as ulcerative colitis (35), multiple sclerosis (36), and Graves’ disease (37), has also been described in previous investigations. Nevertheless, there is limited knowledge about the underlying mechanisms of the observed phenotypic associations. Previous studies have described alterations in pathways of the neuroendocrine and immune systems, for example, altered cortisol concentrations and dysregulation of innate and adaptive immunity, in both stress-related disorders (38) and autoimmune disease (14, 15, 39). In addition, based on GWAS summary statistics, significant genetic correlations have been observed between several psychiatric disorders (e.g., schizophrenia and major depression) and immune-related phenotypes (40). Moreover, using whole-transcriptome RNA-seq gene expression data, a study of 188 U.S. Marines found that a discrete group of coregulated genes relevant to PTSD development was enriched for functions of the innate immune response and interferon signaling (41). Adding to the existing literature, our results demonstrate the contribution of genetic factors to phenotypic associations between stress-related disorders and autoimmune disease and identify potential biological pathways underlying such associations.
The five identified shared functional modules (i.e., MCODE components), characterized by their biological roles in pathways as “signaling by G proteins/GPCRs,” “cilium assembly,” “membrane trafficking,” “eukaryotic translation initiation,” and “cell cycle,” represent novel targets for further study. The first three modules were identified in the analyses using both GWAS results generated from UK Biobank (for any autoimmune disease) and publicly available GWAS results for autoimmune thyroid disease, demonstrating the reliability of the results and the significance of these genes and their related pathways for the phenotypic association between stress-related disorders and autoimmune disease in general. Indeed, existing evidence has revealed the crucial role of GPCRs in inflammation and multiple activities related to autoimmune disease (42). Biologically, as transmembrane proteins, GPCRs respond to a wide variety of extracellular signals and transduce such signals to intracellular signaling pathways via coupling to G proteins, which could regulate a wide range of immune responses (43), such as the regulation of T cell migration (44) and cAMP-dependent protein kinases (45). Both GPCR pathways and cAMP-dependent molecules have been associated with the pathogenesis of multiple autoimmune diseases, including rheumatoid arthritis (46, 47), multiple sclerosis (48), systemic lupus erythematosus (49), and autoimmune thyroid disease (50). Likewise, experimental studies have indicated that molecules in the GPCR pathway could mediate stress responses, such as sustained high anxiety- and depressive-like behaviors, after exposure to trauma or severe stress (51). The activation of cGMP- or cAMP-related neuroprotective molecules might also protect mice against PTSD-like stress-induced traumatic injury (52, 53). Supportive data are also available for cilium-related genes and signaling pathways, which have been suggested to have an important role in autoimmune thyroid disease (54) and major psychiatric disorders (55). Data on the other identified functional modules are limited. Nevertheless, these findings might shed light on the potential genetic components responsible for the co-occurrence of stress-related disorders and autoimmune disease.
Notable study strengths include the combined use of multiple data sources—population-based family data, individual genotyping data, and summary GWAS data—which enabled an assessment of the associations between stress-related disorders and autoimmune disease from the phenotypic to the molecular level. The consistent results noted across all analyses, including the familial coaggregation analyses, PRS analyses, and LD score regression analyses, indicate the validity of these findings.
Nevertheless, this study has several limitations. First, due to the lack of GWAS summary statistics for autoimmune disease, the UK Biobank data set was used for the GWAS and subsequent PRS analysis of autoimmune disease. We addressed the influence of dependent samples by randomly splitting the UK Biobank population into base and test data sets, and the exclusion of individuals with stress-related disorders from the autoimmune disease GWAS (56) might have led to a conservative estimation of polygenic risk association. Nevertheless, we still observed significant associations between autoimmune PRSs and stress-related disorders, providing strong support for the existence of their genetic correlation. Second, it was not feasible to conduct separate analyses for all individual autoimmune diseases, particularly in the Swedish sample, where small number of identified cases were found in the kinship cohorts. Thus, we opted to consider all autoimmune diseases together in the main analysis but performed separate analyses for the six most common autoimmune diseases using the UK Biobank data. Further investigation of the genetic association between stress-related disorders and other autoimmune diseases is warranted. Third, because only a few genetic loci were identified for stress-related disorders in previous GWASs (19), our exploration on the shared genetic mechanisms between stress-related disorders and autoimmune disease provides only suggestive evidence. The importance of shared functional modules, including the module characterized by GPCR pathways, as a biological basis for the high co-occurrence of stress-related disorders and autoimmune disease needs to be replicated in independent samples and verified in functional studies. Finally, similar attempts with more powerful GWAS summary data are needed to comprehensively identify the precise biological similarities between these two diseases.
In summary, using large population-based and diverse phenotypic data with rich genetic information, our study demonstrated a genetic overlap between stress-related disorders and autoimmune disease, highlighting the importance of the shared genes and functional modules, including the one related to GPCR pathways, in the noted phenotypic associations between these disorders. These results have implications for an improved understanding of the common biological mechanisms underlying these diseases and might reveal opportunities for refined efforts in disease prevention and intervention.
1. : Stress as a trigger of autoimmune disease. Autoimmun Rev 2008; 7:209–213Crossref, Medline, Google Scholar
2. : Psychological stress and the human immune system: a meta-analytic study of 30 years of inquiry. Psychol Bull 2004; 130:601–630Crossref, Medline, Google Scholar
3. : Stress and health: psychological, behavioral, and biological determinants. Annu Rev Clin Psychol 2005; 1:607–628Crossref, Medline, Google Scholar
4. : Trauma and PTSD in the WHO World Mental Health surveys. Eur J Psychotraumatol 2017; 8:1353383Crossref, Medline, Google Scholar
5. : Trauma exposure and post-traumatic stress disorder in the general population. Acta Psychiatr Scand 2005; 111:291–299Crossref, Medline, Google Scholar
6. : Association of stress-related disorders with subsequent autoimmune disease. JAMA 2018; 319:2388–2400Crossref, Medline, Google Scholar
7. : Elevated risk for autoimmune disorders in Iraq and Afghanistan veterans with posttraumatic stress disorder. Biol Psychiatry 2015; 77:365–374Crossref, Medline, Google Scholar
8. : Psychological stress, intestinal barrier dysfunctions, and autoimmune disorders: an overview. Front Immunol 2020; 11:1823Crossref, Medline, Google Scholar
9. : Co-morbidity of PTSD and immune system dysfunction: opportunities for treatment. Curr Opin Pharmacol 2016; 29:104–110Crossref, Medline, Google Scholar
10. : Autoimmune diseases, bipolar disorder, and non-affective psychosis. Bipolar Disord 2010; 12:638–646Crossref, Medline, Google Scholar
11. : From inflammation to sickness and depression: when the immune system subjugates the brain. Nat Rev Neurosci 2008; 9:46–56Crossref, Medline, Google Scholar
12. : A cognitive-behavioural symptom management programme as an adjunct in psoriasis therapy. Br J Dermatol 2002; 146:458–465Crossref, Medline, Google Scholar
13. : Stress-induced immune dysfunction: implications for health. Nat Rev Immunol 2005; 5:243–251Crossref, Medline, Google Scholar
14. : From T to B and back again: positive feedback in systemic autoimmune disease. Nat Rev Immunol 2001; 1:147–153Crossref, Medline, Google Scholar
15. : Anti-inflammatory and immune-regulatory cytokines in rheumatoid arthritis. Nat Rev Rheumatol 2019; 15:9–17Crossref, Medline, Google Scholar
16. : Genome-wide association studies of posttraumatic stress disorder in 2 cohorts of US army soldiers. JAMA Psychiatry 2016; 73:695–704Crossref, Medline, Google Scholar
17. : Genome-wide association study of post-traumatic stress disorder reexperiencing symptoms in >165,000 US veterans. Nat Neurosci 2019; 22:1394–1401Crossref, Medline, Google Scholar
18. : Heritability enrichment of specifically expressed genes identifies disease-relevant tissues and cell types. Nat Genet 2018; 50:621–629Crossref, Medline, Google Scholar
19. : Genetic variants associated with anxiety and stress-related disorders: a genome-wide association study and mouse-model study. JAMA Psychiatry 2019; 76:924–932Crossref, Medline, Google Scholar
20. : International meta-analysis of PTSD genome-wide association studies identifies sex- and ancestry-specific genetic risk loci. Nat Commun 2019; 10:4558Crossref, Medline, Google Scholar
21. : The autoimmune spectrum of myasthenia gravis: a Swedish population-based study. J Intern Med 2015; 277:594–604Crossref, Medline, Google Scholar
22. : Tutorial: a guide to performing polygenic risk score analyses. Nat Protoc 2020; 15:2759–2772Crossref, Medline, Google Scholar
23. : FLT3 stop mutation increases FLT3 ligand level and risk of autoimmune thyroid disease. Nature 2020; 584:619–623Crossref, Medline, Google Scholar
24. : Genetics of rheumatoid arthritis contributes to biology and drug discovery. Nature 2014; 506:376–381Crossref, Medline, Google Scholar
25. : Genome-wide association study implicates immune activation of multiple integrin genes in inflammatory bowel disease. Nat Genet 2017; 49:256–261Crossref, Medline, Google Scholar
26. : Type 1 diabetes genome-wide association analysis with imputation identifies five new risk regions. bioRxiv 2017:120022 Google Scholar
27. : LD score regression distinguishes confounding from polygenicity in genome-wide association studies. Nat Genet 2015; 47:291–295Crossref, Medline, Google Scholar
28. : Functional mapping and annotation of genetic associations with FUMA. Nat Commun 2017; 8:1826Crossref, Medline, Google Scholar
29. : The GTEx Consortium atlas of genetic regulatory effects across human tissues. Science 2020; 369:1318–1330Crossref, Medline, Google Scholar
30. : Comprehensive functional genomic resource and integrative model for the human brain. Science 2018; 362:eaat8464 Google Scholar
31. : A compendium of chromatin contact maps reveals spatially active regions in the human genome. Cell Rep 2016; 17:2042–2059Crossref, Medline, Google Scholar
32. : Metascape provides a biologist-oriented resource for the analysis of systems-level datasets. Nat Commun 2019; 10:1523Crossref, Medline, Google Scholar
33. : Association of trauma and posttraumatic stress disorder with incident systemic lupus erythematosus in a longitudinal cohort of women. Arthritis Rheumatol 2017; 69:2162–2169Crossref, Medline, Google Scholar
34. : Systemic autoimmune disease among adults exposed to the September 11, 2001 terrorist attack. Arthritis Rheumatol 2020; 72:849–859Crossref, Medline, Google Scholar
35. : Psychosocial determinants of relapse in ulcerative colitis: a longitudinal study. Am J Gastroenterol 2003; 98:2203–2208Crossref, Medline, Google Scholar
36. : Association between stressful life events and exacerbation in multiple sclerosis: a meta-analysis. BMJ 2004; 328:731Crossref, Medline, Google Scholar
37. : Stress triggers the onset and the recurrences of hyperthyroidism in patients with Graves’ disease. Endocrine 2015; 48:254–263Crossref, Medline, Google Scholar
38. : Neurohormonal-cytokine interactions: implications for inflammation, common human diseases and well-being. Neurochem Int 2008; 52:40–51Crossref, Medline, Google Scholar
39. : Circadian rhythms in rheumatology—a glucocorticoid perspective. Arthritis Res Ther 2014; 16:S3Crossref, Medline, Google Scholar
40. : Genetic correlations among psychiatric and immune-related phenotypes based on genome-wide association data. Am J Med Genet B Neuropsychiatr Genet 2018; 177:641–657Crossref, Medline, Google Scholar
41. : Gene networks specific for innate immunity define post-traumatic stress disorder. Mol Psychiatry 2015; 20:1538–1545Crossref, Medline, Google Scholar
42. : GRKs and arrestins: regulators of migration and inflammation. J Leukoc Biol 2006; 80:1214–1221Crossref, Medline, Google Scholar
43. : Evolving concepts in G protein-coupled receptor endocytosis: the role in receptor desensitization and signaling. Pharmacol Rev 2001; 53:1–24Medline, Google Scholar
44. : G-protein-coupled receptor P2Y10 facilitates chemokine-induced CD4 T cell migration through autocrine/paracrine mediators. Nat Commun 2021; 12:6798Crossref, Medline, Google Scholar
45. : Mechanism of Galpha i-mediated inhibition of type V adenylyl cyclase. J Biol Chem 2002; 277:28823–28829Crossref, Medline, Google Scholar
46. : G protein-coupled receptors in rheumatology. Nat Rev Rheumatol 2014; 10:429–436Crossref, Medline, Google Scholar
47. : Impact of VIP and cAMP on the regulation of TNF-alpha and IL-10 production: implications for rheumatoid arthritis. Arthritis Res Ther 2003; 5:R317–R328Crossref, Medline, Google Scholar
48. : GD1a overcomes inhibition of myelination by fibronectin via activation of protein kinase A: implications for multiple sclerosis. J Neurosci 2017; 37:9925–9938Crossref, Medline, Google Scholar
49. : Deficient protein kinase a in systemic lupus erythematosus: a disorder of T lymphocyte signal transduction. Ann N Y Acad Sci 2002; 968:96–105Crossref, Medline, Google Scholar
50. : Transcriptome analysis of the effect of diosgenin on autoimmune thyroiditis in a rat model. Sci Rep 2021; 11:6401Crossref, Medline, Google Scholar
51. : Molecular and cell signaling targets for PTSD pathophysiology and pharmacotherapy. Neuropharmacology 2012; 62:705–714Crossref, Medline, Google Scholar
52. : Phosphodiesterase-2 inhibitor reverses post-traumatic stress induced fear memory deficits and behavioral changes via cAMP/cGMP pathway. Eur J Pharmacol 2021; 891:173768Crossref, Medline, Google Scholar
53. : Transcriptome analysis reveals novel genes and immune networks dysregulated in veterans with PTSD. Brain Behav Immun 2018; 74:133–142Crossref, Medline, Google Scholar
54. : Integrated miRNA and mRNA expression profiling identifies novel targets and pathological mechanisms in autoimmune thyroid diseases. EBioMedicine 2019; 50:329–342Crossref, Medline, Google Scholar
55. : Patterns of cilia gene dysregulations in major psychiatric disorders. Prog Neuropsychopharmacol Biol Psychiatry 2021; 109:110255Crossref, Medline, Google Scholar
56. : Association of asthma and its genetic predisposition with the risk of severe COVID-19. J Allergy Clin Immunol 2020; 146:327–329Crossref, Medline, Google Scholar