Multimodal Neuroimaging of Suicidal Thoughts and Behaviors in a U.S. Population-Based Sample of School-Age Children
Abstract
Objective:
Suicide deaths and suicidal thoughts and behaviors are considered a public health emergency, yet their underpinnings in the brain remain elusive. The authors examined the classification accuracy of individual, environmental, and clinical characteristics, as well as multimodal brain imaging correlates, of suicidal thoughts and behaviors in a U.S. population-based sample of school-age children.
Methods:
Children ages 9–10 years (N=7,994) from a population-based sample from the Adolescent Brain Cognitive Development study were assessed for lifetime suicidal thoughts and behaviors. After quality control procedures, structural MRI (N=6,238), resting-state functional MRI (N=4,134), and task-based functional MRI (range, N=4,075–4,608) were examined. Differences with Welch’s t test and equivalence tests, with observed effect sizes (Cohen’s d) and their 90% confidence intervals <|0.15|, were examined. Classification accuracy was examined with area under precision-recall curves (AUPRCs).
Results:
Among the 7,994 unrelated children (females, N=3,757, 47.0%), those with lifetime suicidal thoughts and behaviors based on child (N=684, 8.6%), caregiver (N=654, 8.2%), and concordant (N=198, 2.5%) reports had higher levels of social adversity and psychopathology, among themselves and their caregivers, compared with never-suicidal children (N=6,854, 85.7%). Only one imaging test survived statistical correction: caregiver-reported suicidal thoughts and behaviors were associated with a thinner left bank of the superior temporal sulcus. On the basis of the prespecified bounds of |0.15|, approximately 48% of the group mean differences for child-reported suicidal thoughts and behaviors comparisons and approximately 22% for caregiver-reported suicidal thoughts and behaviors comparisons were considered equivalent. All observed effect sizes were relatively small (d≤|0.30|), and both non-imaging and imaging correlates had low classification accuracy (AUPRC ≤0.10).
Conclusions:
Commonly applied neuroimaging measures did not reveal a discrete brain signature related to suicidal thoughts and behaviors in youths. Improved approaches to the neurobiology of suicide are critically needed.
Rates of suicide deaths and suicidal thoughts and behaviors have risen by more than 50% among young people in the past decade (www.who.int/data/gho) (1, 2), making suicide the second leading cause of death among those ages 10–19 years (1, 3). Whereas individual, environmental, and clinical risk factors for suicidal thoughts and behaviors have been well established (4–13), including in the sample analyzed in the present study (14), these factors have demonstrated low predictive validity (15–17). In response, the number of studies examining neurobiological underpinnings of suicidal thoughts and behaviors has grown exponentially in the past two decades (18). Nevertheless, our understanding of the neural mechanisms underlying suicidal thoughts and behaviors and their utility remains poor, especially in young children, for several reasons.
First, it is still unclear whether findings from neuroimaging studies examining suicidal thoughts and behaviors apply to children, because most studies have been conducted using adult samples. Second, results of these studies have been inconsistent. Whereas systematic reviews on the topic suggest that suicidal thoughts and behaviors are associated with abnormalities in regions involved in affective processing and impulsive regulation, the specific regions highlighted in each review differ, and all emphasize the modest sample sizes, heterogeneity, and lack of replicability across studies (18–21). In addition, meta-analyses of structural and functional imaging studies have failed to find differences between suicidal and non-suicidal participants (22–24), and those that found differences either were based on a small number of studies or reported inconsistent findings (24, 25). Third, it is unclear whether the effect sizes of any described neural correlates of suicidal thoughts and behaviors are large enough to have clinical utility. Studies with small sample sizes have limited power to detect differences (19). However, finding no difference does not mean that the difference equals zero; the observed effect size could be considered large enough to be meaningful. On the other hand, studies with large sample sizes are more powered to detect small differences, yet the observed effect size of such differences may be too small for practical purposes (23). To examine whether an observed effect size is large enough to be considered meaningful, one can test for equivalence (26), an approach originally employed in the field of pharmacokinetics (27) with the aim of showing that a new, cheaper drug was practically as effective as an existing one.
In the present study, we employed data from a large population-based sample from the Adolescent Brain Cognitive Development (ABCD) study (https://abcdstudy.org) (28, 29) to examine the correlates of suicidal behaviors using a multi-informant approach. In children ages 9–10 years, we first examined the classification accuracy (i.e., predicted probability of an observation of belonging to a class [e.g., suicide case compared with never-suicidal case]) of individual, environmental, and clinical correlates of suicidal thoughts and behaviors that have been shown to differ in the present sample (14). Next, we sought to identify associations between suicidal thoughts and behaviors and brain morphometry, functional connectivity at rest, and functional measures during three tasks. Specifically, we examined neural correlates of processes that have been implicated in suicidal behavior in clinical and at-risk samples of youths, namely, reward processing (30–33) with the monetary incentive delay task (34), inhibitory control (35, 36) with the stop-signal task (37), and working memory and affective processing (38–41) with an emotional version of the N-back task (42). We tested for differences in these measures using a traditional null hypothesis significance test and complemented our analyses with equivalence testing (26) to examine whether observed effect sizes were large enough to be considered meaningful based on a prespecified benchmark. Finally, we examined the ability of neural correlates to classify suicide cases in our sample and analyzed this in relation to non-imaging correlates of suicidal thoughts and behaviors.
Methods
The ABCD Study
All data used here were accessed from the ABCD Study Curated Annual Release, 2.1 (available on request from the National Institute of Mental Health Data Archive [https://data-archive.nimh.nih.gov/abcd]). The baseline ABCD sample comprises 11,875 children from 22 sites across the United States that match the demographic profile of the American Community Survey (29). The institutional review board at the University of California, San Diego, was responsible for the ethical oversight of the ABCD study. The present study is based on data from 7,994 unrelated ABCD participants for whom complete self-report and caregiver data on childhood suicidal thoughts and behaviors were available. The neuroimaging analyses involved subsamples based on the availability of high-quality MRI data for each modality (see the Methods section and Figure S1 in the online supplement).
Determination of Childhood Suicidal Thoughts and Behaviors
Suicidal thoughts and behaviors in children were assessed using the child and caregiver report of the computerized Kiddie Schedule for Affective Disorders and Schizophrenia for DSM-5–Present and Lifetime Version (K-SADS-PL) (43). A detailed description of the assessment is provided in the Methods section in the online supplement. On the basis of children and caregiver reports, four suicidal thoughts and behaviors groups were computed: child-reported suicidal thoughts and behaviors, caregiver-reported suicidal thoughts and behaviors, concordantly reported suicidal thoughts and behaviors (i.e., both the child and the parent endorse at least one item, the overlap between the first two groups), and never suicidal (i.e., both the child and the parent do not endorse any item). Analyses were conducted on the basis of all three suicidal thoughts and behaviors groups compared with the never-suicidal group.
In addition, on the basis of previous reports suggesting that some suicidal thoughts and behaviors phenotypes are more likely to be associated with neural correlates (22, 44), we also performed secondary analyses of three other groups of participants: those with lifetime suicide attempts, those with current suicidal thoughts and behaviors (as opposed to lifetime), and those with lifetime suicidal thoughts and behaviors and family history of suicide attempt or death by suicide in parents. Given the small number of participants meeting these criteria in the present sample, we computed these groups by combining child and caregiver reports.
Individual and Environmental Characteristics
We examined the classification accuracy of individual and environmental characteristics, as well as clinical factors of the child and caregivers that have been associated with suicidal thoughts and behaviors in previous studies (4–6, 8–10, 12) and in the present sample (14). Detailed descriptions of these factors and the instruments employed to assess these variables are presented in Table S1 in the online supplement.
Neuroimaging
High-resolution T1-weighted images, as well as resting-state and task-based fMRI data, were obtained at each ABCD study site using 3-T MRI systems. In this study, we examined cortical thickness (68 parcellations), subcortical volumes (18 parcellations), functional connectivity at rest (306 connectivity indices), and neural activations (167 parcellations) evoked by three tasks: a modified monetary incentive delay task (34), the stop-signal task (37), and the emotional N-back task (42, 45). To preserve statistical power, we analyzed each modality separately rather than selecting only those children who had high-quality data across all three of the imaging modalities (see Figure S1 in the online supplement). Detailed descriptions of the acquisition protocols, quality-control procedures, imaging processing, and analyses of the ABCD study have been published previously (28, 46) and are summarized in the Methods section in the online supplement.
Statistical Analysis
All analyses compared never-suicidal children with those with endorsed suicidal thoughts and behaviors. A detailed rationale and description of the tests is presented in the Methods section in the online supplement.
Analysis of individual and environmental characteristics.
Group differences in psychosocial factors were examined with Welch’s t tests (47), to allow for unequal number of observations, and with chi-square tests. Results were considered significant at a p value <0.05 with false discovery rate (FDR) correction for multiple comparisons. We further estimated effect sizes (Cohen’s d) of mean and frequency differences and examined the classification accuracy of the most robust correlates calculating the area under precision-recall curve (AUPRC) (for further details, see the Methods section in the online supplement).
Analysis of differences and equivalence of neuroimaging data.
We examined differences in neuroimaging measures between groups with Welch’s t tests to account for unequal number of observations. We examined equivalence of mean differences (i.e., whether observed effect sizes of mean differences were meaningful effects) with equivalence tests (which also included Welch’s t tests to account for unequal number of observations). In equivalence testing, the observed data are statistically compared against an equivalence interval specified a priori (δ), defined by upper (ΔU) and lower (−ΔL) equivalence bounds. The aim of equivalence testing is to reject the null hypothesis that the observed effect size (Cohen’s d) is at least as extreme as a prespecified smallest effect size of interest. We used the two one-sided tests (TOST) procedure (26, 48), implemented with the TOSTtwo function from the library TOSTER in R. Given the present sample size and previous results from a large sample (23), the upper (ΔU) and lower (−ΔL) equivalence bounds were specified as conservative d values of −0.15 and 0.15 (i.e., smallest effect size of interest=|0.15|), which correspond to traditional notions of a small effect size (49). That is, effect sizes with 90% confidence intervals within [−0.15, 0.15] were considered statistically equivalent (i.e., not meaningful effects). The threshold for statistical significance for both tests was set at a p value <0.05 after applying FDR correction for multiple comparisons.
Analysis of classification accuracy.
Classification accuracy of the most robust differences in both non-imaging and imaging data was estimated with the area under precision-recall curve (AUPRC), which provides more accurate information on the performance of a prediction model than the widely used receiver operating characteristic curve in cases where there is an imbalance in the observations between the two classes (50). Precision, or positive predictive value, can be defined as how good a model is at predicting true positive cases. Recall, or sensitivity, can be defined as how good a model is at predicting all the true positive cases. A perfect model would have an AUPRC of 1, as in a receiver operating characteristic curve. However, whereas in a receiver operating characteristic curve a random classifier would have an area under the curve close to 0.50, in precision recall curves that value would be close to the prevalence of positive cases in the population, calculated as y=P/(P+N) (e.g., AUPRC=0.10, if the prevalence is 10%) (for further details, see the Methods section in the online supplement).
Results
Prevalence of Suicidal Thoughts and Behaviors in the Sample
The four groups based on suicidal thoughts and behaviors were categorized as follows: child-reported suicidal thoughts and behaviors (N=684, 8.6%), caregiver-reported suicidal thoughts and behaviors (N=654, 8.2%), concordantly reported suicidal thoughts and behaviors (N=198, 2.5%), and never suicidal (N=6,854, 85.7%). Among participants with endorsed suicidal thoughts and behaviors, either by the child or the caregiver (N=1,140, 14.3%), there was an agreement of 17.4% (N=198).
Lifetime suicidal ideation without attempts (including passive, active, and plans) by the child was endorsed by 577 children (7.2% of the sample, 84.4% of cases) and 615 caregivers (7.7% of the sample, 94% of cases). Lifetime suicide attempts by the child were endorsed by 107 children (1.3% of the sample, 15.6% of cases) and 39 caregivers (0.5% of the sample, 6% of cases). Current suicidal thoughts and behaviors in the child were endorsed by 183 children (2.3% of the sample, 27% of the cases) and 119 caregivers (1.5% of the sample, 18.2% of cases). The number of case subjects with lifetime suicidal thoughts and behaviors and family history of suicide attempt or death by suicide was 68 based on child reports (0.9% of the sample, 9.9% of cases) and 90 based on caregiver reports (1.1% of the sample, 13.8% of cases). The rate of suicidal behaviors reported by either the children or the caregivers and the rate of positive agreement for each item are presented in Table S2 in the online supplement.
Individual and Environmental Characteristics
A full report of suicidal thoughts and behavior correlates in the study sample has been published elsewhere (14). A summary of the descriptive statistics and differences of individual, environmental, and clinical characteristics between the suicidal thoughts and behaviors groups is presented in Table S3 in the online supplement. We further examined current and lifetime mental health problems among caregivers. Several variables differed at pFDR<0.05 between the three groups with suicidal thoughts and behaviors and the never-suicidal group. For these variables, we estimated effect sizes and classification accuracy with the AUPRC (Table 1). Absolute effect sizes (Cohen’s d) of non-imaging correlates of suicidal thoughts and behaviors ranged from 0.14 to 0.87 for child report, from 0.29 to 1.42 for caregiver report, and from 0.33 to 1.47 for concordantly reported suicidal thoughts and behaviors. Overall, the smallest effect sizes of significant correlates were for sex of the child, economic problems in the past year, and use of mental health services by the father. The largest effect sizes of significant correlates were for child-reported family conflict, child psychopathology (14), and caregiver current and lifetime mental health problems, especially in the mother. General psychopathological symptoms, behavioral disorders (i.e., oppositional defiant disorder and conduct disorder), and posttraumatic stress disorder (PTSD) had the largest effect.
Child-Reported (N=684, 8.6%) | Caregiver-Reported (N=654, 8.2%) | Concordantly Reported (N=198, 2.5%) | ||||
---|---|---|---|---|---|---|
Characteristics | |d| | AUPRC | |d| | AUPRC | |d| | AUPRC |
Individual | ||||||
Sex (% male) | 0.17 | 0.04 | 0.29 | 0.03 | 0.33 | 0.01 |
Exposure to stressful events (%) | 0.18 | 0.06 | 0.36 | 0.05 | 0.35 | 0.02 |
Environmental, family, and school | ||||||
Economic problems in the past year (%) | 0.22 | 0.07 | 0.31 | 0.07 | 0.33 | 0.02 |
Family conflict, caregiver report (mean±SD) | 0.18 | 0.11 | 0.49 | 0.14 | 0.44 | 0.05 |
Family conflict, child report (mean±SD) | 0.52 | 0.15 | 0.40 | 0.13 | 0.64 | 0.06 |
Positive school environment (mean±SD) | 0.44 | 0.07 | 0.36 | 0.07 | 0.61 | 0.02 |
Individual clinical | ||||||
General psychopathology, T-scores (CBCL) (mean±SD) | 0.62 | 0.18 | 1.16 | 0.30 | 1.33 | 0.15 |
Current psychiatric disorders, caregiver report (%) | ||||||
Any disorder | 0.37 | 0.07 | 0.78 | 0.06 | 0.87 | 0.02 |
Any depressive disorder | 0.38 | 0.12 | 0.60 | 0.13 | 0.72 | 0.05 |
Any anxiety disorder | 0.35 | 0.10 | 0.62 | 0.10 | 0.77 | 0.04 |
ADHD | 0.37 | 0.10 | 0.71 | 0.11 | 0.77 | 0.04 |
Oppositional defiant disorder | 0.52 | 0.13 | 0.91 | 0.16 | 0.97 | 0.06 |
Conduct disorder | 0.59 | 0.15 | 1.07 | 0.20 | 1.05 | 0.08 |
PTSD | 0.87 | 0.20 | 1.42 | 0.30 | 1.47 | 0.15 |
Family clinical | ||||||
General psychopathology T-scores (ASR) (mean±SD) | 0.35 | 0.13 | 0.66 | 0.17 | 0.74 | 0.06 |
Maternal mental health service use (%) | 0.25 | 0.06 | 0.51 | 0.05 | 0.64 | 0.02 |
Paternal mental health service use (%) | 0.14 | 0.07 | 0.31 | 0.07 | 0.37 | 0.02 |
Maternal mental health hospitalization (%) | 0.40 | 0.12 | 0.63 | 0.13 | 0.77 | 0.05 |
Paternal mental health hospitalization (%) | 0.31 | 0.11 | 0.44 | 0.11 | 0.57 | 0.04 |
Maternal history of depression (%) | 0.27 | 0.07 | 0.53 | 0.07 | 0.67 | 0.02 |
Paternal history of depression (%) | 0.30 | 0.09 | 0.45 | 0.09 | 0.58 | 0.03 |
Maternal history of suicide attempt or death (%) | 0.50 | 0.13 | 0.73 | 0.15 | 0.81 | 0.06 |
Paternal history of suicide attempt or death (%) | 0.48 | 0.13 | 0.58 | 0.14 | 0.83 | 0.06 |
Maternal alcohol or substance use during pregnancy (%) | 0.29 | 0.10 | 0.31 | 0.10 | 0.34 | 0.03 |
TABLE 1. Effect sizes and predictive value of the most consistent differences in individual, environmental, and clinical characteristics between suicidal thoughts and behaviors groups, by informanta
However, in terms of classification accuracy, this was either very poor or not better than what would be expected by chance. Specifically, the AUPRC ranged from 0.04 to 0.20 for child-reported suicidal thoughts and behaviors, from 0.03 to 0.30 for caregiver-reported suicidal thoughts and behaviors, and from 0.01 to 0.15 for concordantly reported suicidal thoughts and behaviors.
Differences and Equivalence of Neuroimaging Data
The sample sizes of the groups for each imaging modality analyzed are presented in Figure S1 in the online supplement. For each modality, we report the combined results of applying traditional null hypothesis Welch’s t tests and equivalence tests, after applying FDR correction for multiple comparisons. The distribution of results is shown in Figure 1.
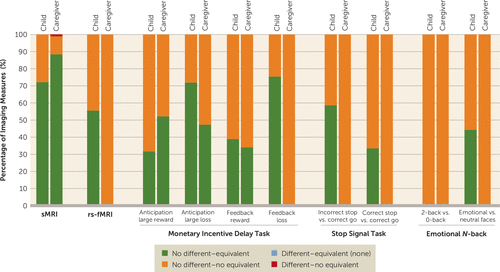
FIGURE 1. Percentage of outcomes of Welch’s t test and equivalence test for each imaging modality by suicidal thoughts and behaviors group comparisona
a For each informant, structural MRI (sMRI) was used to examine 86 regions, resting-state functional MRI (rs-fMRI) was used to examine 306 connectivity indices, and task-based fMRI was used to examine activations in 167 regions. Both no evidence of difference (Welch’s t test, false discovery rate corrected p (pFDR) ≥0.05, 95% CI includes zero) and evidence of difference (Welch’s t test, pFDR <0.05, 95% CI does not include zero) were observed. Both evidence of equivalence (equivalence test, pFDR <0.05, 90% CI does not overlap with bounds) and no evidence of equivalence (equivalence test, pFDR ≥0.05, 90% CI overlaps with bound[s]) were observed.
Results at the corrected level and uncorrected level for all modalities are summarized in Tables S4 and S5 in the online supplement, along with brain measures, if any, that were statistically different and not statistically equivalent across two or more group comparisons.
Brain structural imaging.
Among the 86 regions examined, only the left bank of the superior temporal sulcus was found to be significantly thinner in the caregiver-reported suicidal thoughts and behaviors group than in the never-suicidal group after applying FDR correction (d=−0.17, 95% CI=−0.26, −0.08, pFDR=0.019) (Figures 1 and 2; see also Tables S6–S8 in the online supplement). In addition, on the basis of our prespecified bounds of ±0.15, this effect was large enough to be considered meaningful. Thickness in the superior temporal sulcus was not associated with having a parent with a history of suicide or attempt (t=−0.59, df=5969, p=0.557). However, it was associated with level of income (r=0.05, df=5679, p<0.001), having economic problems in the past year (t=3.11, df=6727, p=0.002, d=0.10), caregiver’s highest level of education achieved (r=0.05, df=6227, p<0.001), history of mental health hospitalizations in the mother (t=2.36, df=6016, p=0.018, d=0.14), and history of depression in either the mother or the father (t=2.13, df=5976, p=0.033, d=0.06). Nevertheless, in a multivariate analysis including caregiver-reported suicidal thoughts and behaviors as a predictor, only suicidal thoughts and behaviors remained significantly associated with lower thickness in the superior temporal sulcus (β=−0.04, t=−2.93, df=5040, p=0.003).
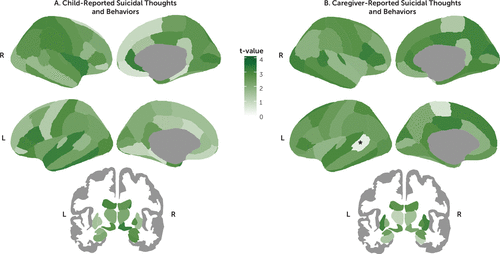
FIGURE 2. Equivalence testing for mean differences in brain cortical thickness and subcortical volumes relating to suicidal thoughts and behaviorsa
a Panel A shows the distribution of t-values from equivalence tests comparing the regional means between the never-suicidal group (N=5,381) and the child-reported suicidal thoughts and behaviors group (N=525), and panel B shows the caregiver-reported suicidal thoughts and behaviors group (N=482). Higher t-values (i.e., darker green) suggest equivalence between groups. As indicated by the asterisk, only the left bank of the temporal sulcus in the caregiver-reported suicidal thoughts and behaviors analysis was statistically different and not statistically equivalent after false discovery rate correction.
All the remaining regions were not statistically different (all pFDR values >0.05). Of these, most regions were statistically equivalent (i.e., effect sizes were practically zero) for the child-reported suicidal thoughts and behaviors comparison (62 regions [72.1%], d range, −0.07, 0.07) and for the caregiver-reported suicidal thoughts and behaviors comparison (76 regions [88.4%], d range=−0.06, 0.07). In contrast, for the concordantly reported suicidal thoughts and behaviors comparisons, all regions were found not to be statistically equivalent (i.e., 90% confidence intervals for Cohen’s d included zero and overlapped with at least one of the |0.15| bounds), with d ranging from −0.23 to 0.23 (Figure 1, see also Figures S2–S7 in the online supplement).
Resting-state functional imaging.
Among the 306 functional connectivity measures, none were statistically different after applying FDR correction (all pFDR values >0.05) (Figure 1; see also Tables S9–S11). In addition, most functional connectivity measures were statistically equivalent (i.e., effect sizes were practically zero) for the child-reported suicidal thoughts and behaviors comparison (170 [55.6%], d range, −0.04, 0.04). In contrast, for the caregiver-reported and concordantly reported suicidal thoughts and behaviors comparisons, all functional connectivity measures were found not to be statistically equivalent (i.e., 90% confidence intervals for Cohen’s d included zero and overlapped with at least one of the |0.15| bounds), with d ranging from −0.18 to 0.20 and −0.34 to 0.28, respectively (see Figures S8–S10 in the online supplement).
Task-based functional imaging.
Results of Welch’s t test and equivalence tests for each of the tasks and contrasts examined are shown in Figures 1 and 3 (see also Tables S12–S35 and Figures S11–S60 in the online supplement). Briefly, among the 167 region-of-interest mean activations examined for each of the three tasks and eight contrasts, none were statistically different after applying FDR correction (all pFDR values >0.05).
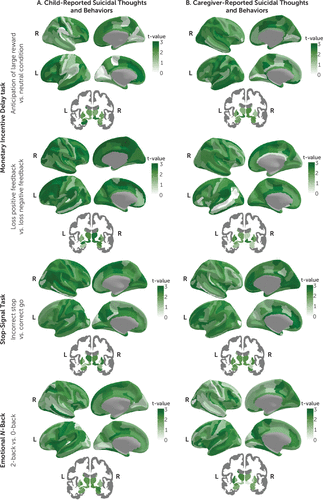
FIGURE 3. Equivalence testing for mean differences in functional activation during the monetary incentive delay task, the stop-signal task, and emotional N-back task relating to suicidal thoughts and behaviorsa
a Panel A shows distribution of absolute t-values from equivalence tests comparing the regional means between the never-suicidal group and the child-reported suicidal thoughts and behaviors group, and panel B shows the caregiver-reported suicidal thoughts and behaviors group. Higher t-values (i.e., darker green) suggest equivalence between groups. No statistical differences were found after applying false discovery rate correction for multiple comparisons. The remaining contrasts are presented in Figure S60 in the online supplement.
Of the region-of-interest mean activations, the number of statistically equivalent measures ranged between 0 and 126 (0%−75.4%, d range, −0.05, 0.05) for the child report comparison, 0 and 116 (0%−69.5%, d range, −0.04, 0.04) for the caregiver report comparison, and none for the concordantly reported suicidal thoughts and behaviors comparison (Figures 1 and 3; see also Figure S60 in the online supplement). No evidence of equivalence was found for 41–167 (24.6%−100%, d range, −0.17, 0.16), 51–167 (30.5%−100%, d range, −0.17, 0.20), and 167 (100%, d range, −0.34, 0.25) of region-of-interest mean activations for child-reported, caregiver-reported, and concordantly reported suicidal thoughts and behaviors comparisons, respectively. The monetary incentive delay task showed the higher rates of region-of-interest activations that were statistically equivalent, followed by the stop-signal task and the emotional N-back (Figures 1 and 3; see also Figure S60 in the online supplement).
Classification Accuracy of Neuroimaging Data
Overall, observed effect sizes were small, especially for child-reported and caregiver-reported suicidal thoughts and behaviors analyses. Maximum effect sizes for child-reported, caregiver-reported, and concordant analyses were |0.17|, |0.20|, and |0.34|, respectively (see Table S36 in the online supplement). On the basis of the lowest and highest 90% confidence interval bounds, all results would have been statistically equivalent if thresholds were |0.29|, |0.33|, and |0.56| for child-reported, caregiver-reported, and concordantly reported suicidal thoughts and behaviors comparisons, respectively.
For child-reported and caregiver-reported suicidal thoughts and behaviors comparisons, only 23 tests (0.67%) resulted in an effect size equal to or greater than our smallest effect size of interest (d≥|0.15|) (Figure 4, see also Table S37 in the online supplement). These included lower thickness of the left bank of the superior temporal sulcus, aberrant connectivity of the default and cingulo-parietal network with the hippocampus and other subcortical areas, and aberrant task-elicited activation of frontal, temporal, and parieto-occipital areas, and the insula. The AUPRC of these observed effect sizes ranged from 0.07 to 0.10. On the basis of the prevalence of suicidal thoughts and behaviors in child and caregiver reports in our sample (approximately 8.5%), these can be considered random classifiers. The AUPRC of the largest effect size, found in the sensorimotor mouth-visual area connectivity in the concordant group analysis (d=0.34, 95% CI=−0.55, −0.12), was 0.02.
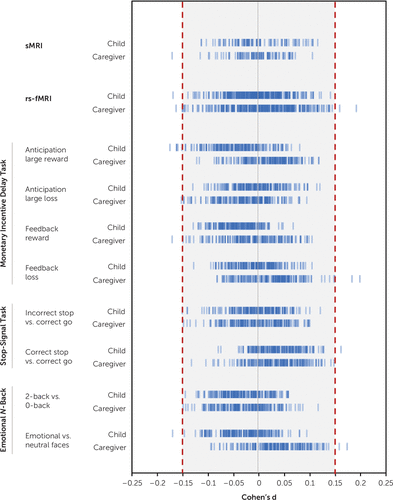
FIGURE 4. Observed effect sizes of mean differences for each imaging modality by suicidal thoughts and behaviors group comparisona
a For each informant, structural MRI (sMRI) was used to examine 86 regions, resting-state functional MRI (rs-fMRI) was used to examine 306 connectivity indices, and task-based fMRI was used to examine activations in 167 regions. Blue individual lines represent the effect size of group mean differences for a region or connectivity index. The shaded area represents the effect size lower than the prespecified smallest effect size of interest of d=|0.15|.
Neuroimaging Correlates of High-Risk Suicidal Thoughts and Behaviors Groups
The sample size of the groups for each imaging modality analyzed is presented in Table S38 in the online supplement, and a summary of the findings in high-risk groups in terms of difference and equivalence of means is presented in Table S39 in the online supplement. For case subjects with current suicidal thoughts and behaviors or lifetime suicidal thoughts and behaviors with a family history of suicide attempt or death, all results were not statistically different and not statistically equivalent after applying FDR correction (all pFDR values >0.05). The same was true for case subjects with lifetime suicide attempt, with two exceptions: during the stop-signal task, children with lifetime suicide attempt showed higher activation in the left pallidum when they were expected to inhibit a response but did not (d=0.47, 95% CI=0.20, 0.74, pFDR=0.028) and lower activation in the right ventral diencephalon when they succeeded in inhibiting a response (d=0.56, 95% CI=0.28, 0.84, pFDR=0.013). However, the classification accuracy of these two findings was not better than what would be expected by chance, with AUPRCs of 0.02 and 0.01, respectively.
Discussion
In a large U.S. population-based sample of school-age children, we found that endorsement of suicidal thoughts and behaviors was strongly associated with higher levels of psychopathology and social adversity, but these factors had poor classification accuracy. In terms of neural correlates, over the 5,000 tests performed to examine differences in structural MRI and resting-state and task-based fMRI, only one survived correction, in which suicidal thoughts and behaviors were associated with thinner left bank of the superior temporal sulcus. Nevertheless, effect sizes of neural correlates were very small, and their ability to classify case subjects with suicidal thoughts and behaviors was not better than what would be expected by chance.
The rate of reported suicidal thoughts and behaviors in our sample was in line with rates found in prepubertal and school-age children (51, 52), which is lower than in community samples of adolescents and young adults (4–7, 53). Child and caregiver reports of suicidal thoughts and behaviors were not consistent, which is a common observation in adolescents and young adults, in whom nondisclosure may involve concerns about stigmatization, difficulties in communication, and unavailability of social and family support (54).
In this sample, suicidal thoughts and behaviors were associated with higher psychosocial adversity and clinical correlates, thus replicating a number of studies (14). The effect sizes of these associations ranged from small to large, with the largest effect sizes being linked to clinical characteristics in the child and caregivers and child-reported family conflict. For example, behavioral disorders such as oppositional defiant disorder and conduct disorder, as well as PTSD, had effect sizes >1. The strongest association of suicidal behaviors with externalizing rather than internalizing behaviors is consistent with reports on prepubertal children (10, 11). However, regardless of the effect sizes, the classification accuracy of non-imaging correlates either was no better than random selection or was extremely poor, which is in line with previous reports (16).
In terms of neuroimaging correlates, at the uncorrected level, we found several regions associated with suicidal thoughts and behaviors not consistently reported in the literature (18), and those regions that we found that had been reported (e.g., aberrant thickness in the medial orbitofrontal gyrus, aberrant connectivity in the default mode and salience networks, and aberrant task-elicited activations in the temporal lobe and insula) differed in directionality or specific regions involved (18, 40, 41, 55–58). Moreover, after false discovery rate correction, we only found a thinner left bank of the superior temporal sulcus in the caregiver-reported suicidal thoughts and behaviors analysis. Similar findings in cortical thickness have been found in adults with schizophrenia (59), and reduced volume but not thickness in this same region has been found in adolescents with a history of suicide attempt (60). The superior temporal region is part of a neural network involved in inhibitory control and emotion processing in social contexts and has been associated with lethality of attempts and impulsivity (61). Secondary analyses in those with lifetime suicide attempts showed altered activation during inhibitory control in the pallidum and the ventral diencephalon. The latter has been associated with suicidal ideation, but not attempts, in adults with depression (62). No associations were found with current suicidal thoughts and behaviors or lifetime suicidal thoughts and behaviors with family history of suicide attempt or death.
Regardless of differences, and based on our prespecified conservative bound of |0.15|, we showed that around half of the group means for child-reported suicidal thoughts and behaviors comparisons (approximately 48%) and one-fifth for parent-reported suicidal thoughts and behaviors comparisons (approximately 22%) were equivalent (i.e., not a meaningful effect). These would have been nearly 100% equivalent with a prespecified bound of |0.30|, which is still small. In the case of the concordant group and the high-risk groups, all observed effect sizes of mean differences were not statistically equivalent (i.e., meaningful effects). In these cases, where there was no difference but effects were not statistically equivalent, the data were insufficient to draw conclusions. With our conservative smallest effect size of interest of |0.15|, the equivalence bounds became narrower, and the concordant and high-risk groups should have had a larger sample size in order to obtain a sufficiently narrow confidence interval to conclude that the observed effect sizes were statistically equivalent (i.e., not a meaningful effect). While widely employed, the choice of a d=|0.15| for the equivalence tests was, of course, arbitrary and only meant as an indicator of potential clinical meaningfulness. Our power calculations indicated that our sample sizes in this study could be used to detect such differences at a standard statistical significance threshold and power (α=0.05 and 1−β=0.80; see also the online supplement), at least for structural MRI. Obviously, even larger samples would be required to detect such differences after the application of correction for multiple comparisons. This points to the fact that, much like in the field of genetics, power—and therefore sample size—may be a limitation for the discovery of brain networks significantly contributing to clinical phenotypes, such as suicidality. Equally, our findings indicate that, at least for the phenotypes studied here, brain effects are most likely to be small.
Indeed, in this study, observed effect sizes were relatively small for all regions and connectivity indices tested (d<|0.30|), which is in line with studies conducted in large samples (23). This was even true within the concordant and high-risk groups (with the only exceptions being the two significant findings in those with suicide attempt). Small effect sizes can still be clinically relevant if they can predict clinical outcomes or treatment response or point to mechanistic pathways of disease (63). We therefore examined the classification accuracy of the largest effect sizes in our sample, as we did with the non-imaging correlates. We found that these were not better at classifying suicidal cases than what one would get by selecting cases randomly from the population. This is important because, ironically, the shift from studying psychosocial risk factors to studying neurobiological biomarkers of suicidal thoughts and behaviors was partly motivated by the poor sensitivity of the former in predicting suicide (15–17), which we also showed in this study. While the pattern of increasing suicide rates in young people does not show signs of stopping, it is not yet clear whether this change in focus of study is providing us with any benefit, especially given the cost of neuroimaging studies. The aim was to improve identification and prevention of suicidal thoughts and behaviors. However, to date, the evidence is still weak for this purpose as a result of small sample sizes, heterogeneity and inconsistency across studies, and, as further shown in this study, small effect sizes with limited classification accuracy. Non-imaging correlates, although they also have low classification accuracy, at least have moderate to large effect sizes, especially associated with clinical characteristics. Therefore, addressing psychosocial and clinical factors may be our best bet at this point. Nevertheless, there is an urgent need to improve the study of neurobiological biomarkers. In future studies, investigators should aim their efforts at using other methodologies, such as machine learning (64, 65) and building models based on aggregation of multiple variables, including neuroimaging, psychosocial, clinical, and genetic correlates of suicidal behaviors, to increase predictive validity. That said, what our results show is that vulnerability to suicidal thoughts and behaviors does not appear to have a brain signature with a strong enough effect in school-age children. However, this does not imply that suicidal thoughts and behaviors do not have brain correlates; rather, it indicates that such associations, if any, may not be discernible using common neuroimaging measures at this age, for various reasons. First, it is possible that what we call suicidal thoughts and behaviors in children is different from suicidal thoughts and behaviors in adolescents or adults. Historically, it was believed that prepuberal children could not have suicidal thoughts and behaviors because of their concrete operational thinking, which limits their concept of causality, as well as the concepts of death and finality (66, 67). However, current evidence suggests that even preschoolers can show suicidal behaviors with the intent to cause self-injury or death (66, 68), which is agreed to be the essential quality of suicidal thoughts and behaviors, regardless of an understanding of finality or lethality (67). Second, as brain organization evolves during the adolescent years, also a time when more active suicidal behaviors emerge, it is plausible that neural correlates of suicidal thoughts and behaviors become more evident as the brain matures. In that sense, investigation of the longitudinal data from the ABCD study cohort, when such data become available, will likely shed some light on these incongruent findings across samples of different ages. Finally, it is also plausible that we may not find a single correlate of suicidal thoughts and behaviors in those adolescents. Suicide is known to be a complex phenomenon, and based on works from affective neuroscience (69, 70), it is likely that suicidal behaviors are not discrete categories and thus do not have one-to-one brain signatures. Comprehensive reviews on the topic are a good reminder of that heterogeneity (18). Future studies should combine multiple types of correlates and examine interrelated trajectories across factors that might help us identify the shift to more active suicidal behaviors at peak ages, such as late adolescence and early adulthood.
Our study has some limitations. Because participants were drawn from the community, very few had active suicidal thoughts or behaviors—especially the latter—at the time of scanning and therefore were not necessarily comparable to clinical cases. This is important because some studies suggest that suicidal ideation may have distinct clinical, genetic, and imaging correlates compared with suicidal behaviors (18, 71). We attempted to address these limitations by analyzing only those individuals at highest risk, yet this approach reduced considerably the number of participants and limited the power to detect differences. Nevertheless, the majority of effect sizes were small, which suggests that they would be small even with larger sample sizes. Moreover, passive ideation has been shown to be associated with significant psychiatric comorbidity and to be similar to active ideation in terms of risk factors (72), as also shown in this study. Whereas using a community-based sample avoids referral bias and may aid in the identification of suicidal thoughts and behaviors in nonclinical samples, future waves of the ABCD study should capture the age-related increase in prevalence of more active suicidal behaviors.
Conclusions
Vulnerability to suicidal thoughts and behaviors in young children does not appear to have a discrete brain signature when considering commonly used neuroimaging measures. Moreover, observed effect sizes of imaging correlates of suicidal thoughts and behaviors are small, with limited classification accuracy. Improved approaches to the neurobiology of suicide are critically needed.
1 Centers for Disease Control and Prevention: Web-based Injury Statistics Query and Reporting System (WISQARS). Atlanta, National Center for Injury Prevention and Control, 2019Google Scholar
2 : Hospitalization for suicide ideation or attempt: 2008–2015. Pediatrics 2018; 141:141Crossref, Google Scholar
3 Curtin SC, Heron M, Minino AM, et al: Recent increases in injury mortality among children and adolescents aged 10–19 years in the United States: 1999–2016: national vital statistics reports from the Centers for Disease Control and Prevention, National Center for Health Statistics. Atlanta, National Vital Statistics System, 2018Google Scholar
4 : Prevalence, correlates, and treatment of lifetime suicidal behavior among adolescents: results from the National Comorbidity Survey Replication Adolescent Supplement. JAMA Psychiatry 2013; 70:300–310Crossref, Medline, Google Scholar
5 : Prevalence and correlates of suicidal ideation and attempts among children and adolescents. Int J Adolesc Med Health 2017; 29:(2) Crossref, Medline, Google Scholar
6 : What distinguishes adolescents with suicidal thoughts from those who have attempted suicide? a population-based birth cohort study. J Child Psychol Psychiatry 2019; 60:91–99Crossref, Medline, Google Scholar
7 : Neurocognitive functioning in community youth with suicidal ideation: gender and pubertal effects. Br J Psychiatry 2019; 215:552–558Crossref, Medline, Google Scholar
8 : Completed suicide in childhood. Psychiatr Clin North Am 2008; 31:271–291Crossref, Medline, Google Scholar
9 : Suicide in early childhood: a brief review. Int J Adolesc Med Health 2005; 17:221–224Crossref, Medline, Google Scholar
10 : Suicidal behavior in children younger than twelve: a diagnostic challenge for emergency department personnel. Acad Emerg Med 2007; 14:810–818Crossref, Medline, Google Scholar
11 : Adolescent suicide and suicidal behavior. J Child Psychol Psychiatry 2006; 47:372–394Crossref, Medline, Google Scholar
12 : Suicide in children: a systematic review. Arch Suicide Res 2015; 19:285–304Crossref, Medline, Google Scholar
13 : Suicide and suicidal behaviour. Lancet 2016; 387:1227–1239Crossref, Medline, Google Scholar
14 : Risk and protective factors for childhood suicidality: a US population-based study. Lancet Psychiatry 2020; 7:317–326Crossref, Medline, Google Scholar
15 : Prediction of suicide in psychiatric patients: report of a prospective study. Arch Gen Psychiatry 1983; 40:249–257Crossref, Medline, Google Scholar
16 : Risk factors for suicidal thoughts and behaviors: a meta-analysis of 50 years of research. Psychol Bull 2017; 143:187–232Crossref, Medline, Google Scholar
17 : Prediction models for suicide attempts and deaths: a Systematic review and simulation. JAMA Psychiatry 2019; 76:642–651Crossref, Medline, Google Scholar
18 : Imaging suicidal thoughts and behaviors: a comprehensive review of 2 decades of neuroimaging studies. Mol Psychiatry 2019; 25:408–427 Crossref, Medline, Google Scholar
19 : Neuroimaging studies of suicidal behavior and non-suicidal self-injury in psychiatric patients: a systematic review. Front Psychiatry 2018; 9:500Crossref, Medline, Google Scholar
20 : Structural and functional neuroimaging studies of the suicidal brain. Prog Neuropsychopharmacol Biol Psychiatry 2011; 35:796–808Crossref, Medline, Google Scholar
21 : Structural and functional alterations of the suicidal brain: an updated review of neuroimaging studies. Psychiatry Res Neuroimaging 2018; 278:77–91Crossref, Medline, Google Scholar
22 : Neuroimaging-informed phenotypes of suicidal behavior: a family history of suicide and the use of a violent suicidal means. Transl Psychiatry 2018; 8:120Crossref, Medline, Google Scholar
23 : Subcortical brain structure and suicidal behaviour in major depressive disorder: a meta-analysis from the ENIGMA-MDD working group. Transl Psychiatry 2017; 7:e1116Crossref, Medline, Google Scholar
24 : Brain abnormalities associated with self-injurious thoughts and behaviors: a meta-analysis of neuroimaging studies. Sci Rep 2020; 10:2404Crossref, Medline, Google Scholar
25 : Is there a neuroanatomical basis of the vulnerability to suicidal behavior? a coordinate-based meta-analysis of structural and functional MRI studies. Front Hum Neurosci 2014; 8:824Crossref, Medline, Google Scholar
26 : Equivalence tests: a practical primer for t tests, correlations, and meta-analyses. Soc Psychol Personal Sci 2017; 8:355–362Crossref, Medline, Google Scholar
27 : A new statistical procedure for testing equivalence in two-group comparative bioavailability trials. J Pharmacokinet Biopharm 1984; 12:83–91Crossref, Medline, Google Scholar
28 : The adolescent brain cognitive development (ABCD) study: imaging acquisition across 21 sites. Dev Cogn Neurosci 2018; 32:43–54Crossref, Medline, Google Scholar
29 : Recruiting the ABCD sample: design considerations and procedures. Dev Cogn Neurosci 2018; 32:16–22Crossref, Medline, Google Scholar
30 : Preserved hippocampal function during learning in the context of risk in adolescent suicide attempt. Psychiatry Res 2013; 211:112–118Crossref, Medline, Google Scholar
31 : Neural responses to gains and losses in children of suicide attempters. J Abnorm Psychol 2017; 126:237–243Crossref, Medline, Google Scholar
32 : Decreased activation of lateral orbitofrontal cortex during risky choices under uncertainty is associated with disadvantageous decision-making and suicidal behavior. Neuroimage 2010; 51:1275–1281Crossref, Medline, Google Scholar
33 : Blunted neural reward responsiveness in children with recent suicidal ideation. Clin Psychol Sci 2019; 7:958–968Crossref, Medline, Google Scholar
34 : fMRI visualization of brain activity during a monetary incentive delay task. Neuroimage 2000; 12:20–27Crossref, Medline, Google Scholar
35 : Dissociable patterns of neural activity during response inhibition in depressed adolescents with and without suicidal behavior. J Am Acad Child Adolesc Psychiatry 2011; 50:602–611.e3Crossref, Medline, Google Scholar
36 : Impulsivity in the self-harm and suicidal behavior of young people: a systematic review and meta-analysis. J Psychiatr Res 2019; 116:51–60Crossref, Medline, Google Scholar
37 : On the ability to inhibit thought and action: a users’ guide to the stop signal paradigm, in Inhibitory Processes in Attention, Memory, and Language. San Diego, Academic Press, 1994, pp 189–239Google Scholar
38 : Attenuated prefrontal and temporal neural activity during working memory as a potential biomarker of suicidal ideation in veterans with PTSD. J Affect Disord 2019; 257:607–614Crossref, Medline, Google Scholar
39 : Working memory mediates increased negative affect and suicidal ideation in childhood attention-deficit/hyperactivity disorder. J Psychopathol Behav Assess 2018; 40:180–193Crossref, Medline, Google Scholar
40 : Differential patterns of activity and functional connectivity in emotion processing neural circuitry to angry and happy faces in adolescents with and without suicide attempt. Psychol Med 2013; 43:2129–2142Crossref, Medline, Google Scholar
41 : Multimodal neuroimaging of frontolimbic structure and function associated with suicide attempts in adolescents and young adults with bipolar disorder. Am J Psychiatry 2017; 174:667–675Link, Google Scholar
42 : When is an adolescent an adult? assessing cognitive control in emotional and nonemotional contexts. Psychol Sci 2016; 27:549–562Crossref, Medline, Google Scholar
43 Kobak K, Kratochvil C, Stanger C, et al: Computerized screening of comorbidity in adolescents with substance or psychiatric disorders, in a poster presented at the proceedings of the 33rd Annual Anxiety Disorders and Depression Conference, La Jolla, Calif, 2013Google Scholar
44 : Maternal suicidality and risk of suicidality in offspring: findings from a community study. Am J Psychiatry 2005; 162:1665–1671Link, Google Scholar
45 Cohen AO, Conley MI, Dellarco DV, et al: The impact of emotional cues on short-term and long-term memory during adolescence, presented at the Proceedings of the Society for Neuroscience, San Diego, 2016Google Scholar
46 : Image processing and analysis methods for the Adolescent Brain Cognitive Development study. Neuroimage 2019; 202:116091Crossref, Medline, Google Scholar
47 : The generalisation of student’s problems when several different population variances are involved. Biometrika 1947; 34:28–35Medline, Google Scholar
48 : A comparison of the two one-sided tests procedure and the power approach for assessing the equivalence of average bioavailability. J Pharmacokinet Biopharm 1987; 15:657–680Crossref, Medline, Google Scholar
49 : Statistical Power Analysis for the Behavioral Sciences, 2nd ed. Hillsdale, NJ, Erlbaum, 1988Google Scholar
50 : The precision-recall plot is more informative than the ROC plot when evaluating binary classifiers on imbalanced datasets. PLoS One 2015; 10:e0118432Crossref, Medline, Google Scholar
51 : Suicidal behavior in normal school children: a comparison with child psychiatric inpatients. J Am Acad Child Psychiatry 1984; 23:416–423Crossref, Medline, Google Scholar
52 : Psychopathology associated with suicidal ideation and attempts among children and adolescents. J Am Acad Child Adolesc Psychiatry 1998; 37:915–923Crossref, Medline, Google Scholar
53 : Predictors of future suicide attempt among adolescents with suicidal thoughts or non-suicidal self-harm: a population-based birth cohort study. Lancet Psychiatry 2019; 6:327–337Crossref, Medline, Google Scholar
54 : Psychosocial factors correlated with undisclosed suicide attempts to significant others: findings from the Adolescence SEYLE Study. Suicide Life Threat Behav 2019; 49:759–773Crossref, Medline, Google Scholar
55 : Neural correlates of emotion regulation and adolescent suicidal ideation. Biol Psychiatry Cogn Neurosci Neuroimaging 2018; 3:125–132Crossref, Medline, Google Scholar
56 : Network basis of suicidal ideation in depressed adolescents. J Affect Disord 2018; 226:92–99Crossref, Medline, Google Scholar
57 : Longitudinal decreases in suicidal ideation are associated with increases in salience network coherence in depressed adolescents. J Affect Disord 2019; 245:545–552Crossref, Medline, Google Scholar
58 : Striatal and cortical midline circuits in major depression: implications for suicide and symptom expression. Prog Neuropsychopharmacol Biol Psychiatry 2012; 36:290–299Crossref, Medline, Google Scholar
59 : Pronounced prefronto-temporal cortical thinning in schizophrenia: neuroanatomical correlate of suicidal behavior? Schizophr Res 2016; 176:151–157Crossref, Medline, Google Scholar
60 : Right superior temporal gyrus volume in adolescents with a history of suicide attempt. Br J Psychiatry 2015; 206:339–340Crossref, Medline, Google Scholar
61 : Impulsivity, aggression and brain structure in high and low lethality suicide attempters with borderline personality disorder. Psychiatry Res 2014; 222:131–139Crossref, Medline, Google Scholar
62 : Cognitive inhibition in depression and suicidal behavior: a neuroimaging study. Psychol Med 2016; 46:933–944Crossref, Medline, Google Scholar
63 : Evaluating effect size in psychological research. Sense and Nonsense 2019; 2:156–168Google Scholar
64 : Machine learning for suicide research-can it improve risk factor identification? JAMA Psychiatry 2020; 77:13–14Crossref, Medline, Google Scholar
65 : Machine learning of neural representations of suicide and emotion concepts identifies suicidal youth. Nat Hum Behav 2017; 1:911–919Crossref, Medline, Google Scholar
66 : Suicidal behaviour—does it exist in pre-school aged children? Ir J Psychol Med 1999; 16:72–74Crossref, Google Scholar
67 : Childhood suicidal behavior: a developmental perspective. Psychiatr Clin North Am 1997; 20:551–562Crossref, Medline, Google Scholar
68 : Suicide in elementary school-aged children and early adolescents. Pediatrics 2016; 138:138Crossref, Google Scholar
69 : Variety in emotional life: within-category typicality of emotional experiences is associated with neural activity in large-scale brain networks. Soc Cogn Affect Neurosci 2015; 10:62–71Crossref, Medline, Google Scholar
70 : The brain basis of emotion: a meta-analytic review. Behav Brain Sci 2012; 35:121–143Crossref, Medline, Google Scholar
71 : Genetic aetiology of self-harm ideation and behaviour. Sci Rep 2020; 10:9713Crossref, Medline, Google Scholar
72 : Characterizing the phenomenology of passive suicidal ideation: a systematic review and meta-analysis of its prevalence, psychiatric comorbidity, correlates, and comparisons with active suicidal ideation. Psychol Med 2020; 50:367–383Crossref, Medline, Google Scholar