Proof-of-Principle Study on ECT Illustrates Challenges and Possible Merits of Using Polygenic Risk Scores to Predict Treatment Response in Psychiatry
Major depressive episodes occur frequently in the context of major depressive disorder and bipolar disorder, with over 300 million people currently living with depression worldwide (1). Although a range of treatment options are available, approximately one-third of patients do not respond to the most common treatment strategies (2). Electroconvulsive therapy (ECT) is one of the most effective medical interventions in clinical psychiatry, with remission rates in the range of 50%–80%, even for people with difficult-to-treat depression (3, 4). Despite these good response rates overall, individual responses to ECT may vary greatly. Recent meta-analyses have indicated that various clinical factors, including psychotic features, older age, shorter duration of the depressive episode, and absence of medication resistance, are associated with a more favorable response to ECT (5, 6). There is currently no established multifactorial algorithm that can reliably predict an individual’s response to ECT. This is unfortunate, as personalized prediction of treatment response would be of great relevance for clinical decision making, reducing the number of treatments needed to achieve remission.
While the precise mechanisms underlying the effects of ECT are not yet known, it is clear that the elicited seizures can induce biological processes, including neuroplasticity and immune system activation (see Jiang et al. [7] for a review). One can speculate that genetic variation underlying these biological processes would moderate responsivity to ECT. Genetic data may therefore be informative for personalized prediction of treatment response, which could potentially inform personalized treatment selection (8). An estimate of an individual’s genetic liability to a trait or diagnosis can be computed with a polygenic risk score (PRS) based on genome-wide association studies (GWASs). PRSs have been shown to have predictive value for some psychiatric disorders (8), although the field of psychiatric genetics is still in its developing stage (at least in terms of translation to clinical practice; for an excellent tutorial review, see Murray et al. [9]), and current evidence indicates that the variance in the phenotype explained by cumulative genetic risk scores is low, and much lower than the variance explained by cumulative environmental risk scores (10). Given that most psychiatric phenotypes have a complex origin and polygenic nature, the field of psychiatric genetics has learned that such discovery studies require very large sample sizes, which are not yet at hand for treatment studies in psychiatry. However, PRSs for a range of traits and diagnoses can now be calculated relatively simply for individuals participating in clinical studies. To date, two studies have examined whether the clinical effects of ECT in patients with major depressive episodes were associated with higher PRSs for psychiatric diagnoses (11, 12). Both studies had relatively small samples sizes (one study had 51 subjects, and the other 266 subjects), and neither study revealed a statistically significant association between response to ECT and PRS for major depression. Interestingly, the latter study (12) did report that response to ECT was better in patients with major depressive episodes who had a higher PRS for schizophrenia.
In this issue of the Journal, Sigström et al. (13) report that clinical response to ECT was associated with cumulative genetic risk scores for major depression (PRS-MDD), bipolar disorder (PRS-BP), and schizophrenia (PRS-SCZ). Their primary analysis focused on the Clinical Global Impressions improvement score (CGI-I) documented by the treating clinician after the last ECT session. This primary clinical measure formed a practical cornerstone of clinical outcome monitoring and was collected for 2,320 patients throughout Sweden, as organized via the Swedish National Quality Register for ECT, which by 2014 covered almost 90% of all ECT series in Sweden. Because the genetic liability to be diagnosed with a certain psychiatric diagnosis is probably not the same as the genetic liability to the response to a certain treatment—here, ECT—the study was conceptually limited by the use of PRSs derived from case-control GWAS analyses (i.e., individuals with a diagnosis of major depressive disorder, bipolar disorder, or schizophrenia vs. control subjects), although it forms an interesting first exploration. As summarized in Figure 1, the findings of this study on genetic prediction of ECT response were quite striking. Where (as expected) patients with major depression as well as patients with bipolar disorder were good responders to ECT, the CGI-I score after ECT was negatively associated with PRS-MDD, and positively with PRS-BD. Thus, people with a higher cumulative genetic risk for major depression showed less clinical improvement after ECT than people with a higher cumulative genetic risk for bipolar disorder. Similar findings were obtained when the study authors investigated response and remission using depression ratings with the self-report Montgomery-Åsberg Depression Rating Scale. With respect to the PRS-SCZ, the authors did not find a significant association with CGI-I score after ECT, although it was associated with posttreatment remission (similar to findings in the study cited above [12]).
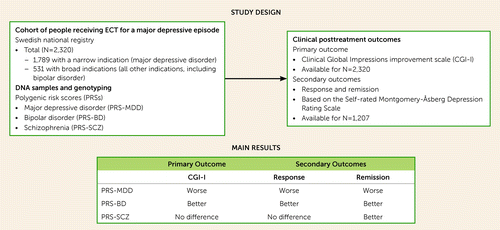
FIGURE 1. Summary of the design and main findings of the study by Sigström et al. (13)
As compared to most other studies on psychiatric genetics, the major strengths of the Sigström et al. study lie in 1) its focus on longitudinal changes (with baseline, pre-exposure measurements) and treatment response to ECT, and 2) its embedding in regular clinical routines through the use of registry-based clinical cohorts and the use of the CGI-I as the main outcome measure, strengthening clinical translation and value.
Interpretation of the Findings
The finding of dissociative effects of PRS-MDD and PRS-BP (and PRS-SCZ) suggests that genetic variation underlying bipolar disorder (and schizophrenia) is more strongly connected to the potency to activate or reactivate certain biological processes in response to ECT (and thereby the clinical response) than the genetic variation underlying major depressive disorder. While indeed the genetic correlation of bipolar disorder with schizophrenia is strong (rg=0.68), and moderate with major depressive disorder (rg=0.44), recent work has modeled the genetic correlations of a range of psychiatric diagnoses (including major depressive disorder, bipolar disorder, and schizophrenia), identifying four transdiagnostic factors: “neurodevelopmental, compulsive, psychotic, and internalizing factors” (14). It would be interesting in future analyses to investigate whether the genetic variants underlying ECT response (as observed in the Sigström et al. study) may indeed be enriched in the genetic variants underlying the “psychotic factor” (in line with the clinical observation that people with major depressive disorder with psychotic features respond better to ECT) and the “internalizing factor” (in line with the clinical observation that people with chronic and treatment-resistant depression respond less well to ECT) (5, 6).
In fact, the field of psychiatric genetics has been advancing from cross-sectional analyses on categorical diagnoses toward longitudinal analyses of trans-syndromal and specific phenotypes as well as on the differential impact of treatments and other environmental exposures (early-life risk factors, etc.) on such trajectories (e.g., 15). Given 1) the strong developments in the fundamental insights of genetic background linked to broad and specific phenotypes, 2) the relatively low cost of a genome-wide analysis, and 3) the current culture and accepted value of collaboration in large-scale networks within the international scientific and clinical communities (such as in the existing infrastructure of the Swedish registry and the Psychiatric Genomics Consortium), we foresee that the next phase of research will allow for putting to the test the predictive value of PRSs of treatment response in clinical psychiatry through appropriately designed and adequately powered studies.
Predictive Power of Current Polygenic Scores
It should be noted that the predictive power of the PRSs to explain clinical outcome after ECT was very small in this study; they explained less than 1% of the variance in the ECT-related clinical improvement, while in another recent study (12), PRS-SCZ explained 5%–7% of clinical response to ECT. Technical and conceptual optimization of the computation of PRSs (larger samples sizes, advanced statistical models, more phenotypes and traits) is currently ongoing and may lead to incremental improvements in explained variance in the near future. Nevertheless, the predictive value of measurable genetic background is expected to remain minor to modest, and other factors are expected to have higher predictive value than genetic background (by itself), particularly when combined into multifactorial prediction models.
Next Steps
Replication of the present analysis in ECT studies as well as in genetic studies on other treatment modalities (e.g., pharmacotherapy, repetitive transcranial magnetic stimulation, psychotherapies) through large-scale scientific and clinical collaborative efforts is now needed to establish the possible value of genetic prediction of treatment response in psychiatry.
Setups of standardized longitudinal clinical monitoring (exemplified in the Sigström et al. study) also facilitate other scientific endeavors with potential impact in the near future. For example, longitudinal follow-up studies enable the study of other biological factors, such as the immune system and epigenetic regulation of gene expression of neurotrophins (e.g., 16, 17) in relation to response to ECT. Such studies can complement studies at the genetic level, thereby forming a strong basis for identification of actionable biological targets and the establishment of markers and biomarkers predicting and monitoring response to ECT.
Toward Accurate and Clinically Relevant Prediction
Although the potential of prediction models for treatment response in depression is promising, this field is still in its infancy, and the models are not yet ready to inform treatment selection in clinical practice (18, 19). As depression is highly heterogeneous, multiple features from different domains (e.g., sociodemographic, clinical, genetic, and neuroimaging data) have to be considered to accurately predict the complex processes that underlie response to depression treatment. In addition, the modest results from prediction models that include variables from only one domain could support this notion (18). One excellent study that combined both clinical and genetic data (20) developed two models that accurately predicted outcomes of 12-week treatment with escitalopram and nortriptyline in an independent data set (a hold-out data set—i.e., one that was not used for model development). Interestingly, the prediction models were drug specific, meaning that remission was accurately predicted in the hold-out sample treated with the same type of antidepressant medication. These treatment-specific predictions hold promise for treatment selection. Another example of a prediction model based on multifactorial data has been reported by Taliaz et al. (21), who found that combined demographic, clinical, and genetic data accurately predicted response to various types of antidepressant medication in an independent data set. Although both studies (20, 21) tested the prediction accuracy of their models within a hold-out data set, this process of external validation has been done infrequently in other prediction studies, making it difficult to estimate their generalizability to clinical practice. This is especially worrying, as prediction models are often developed from relatively small data sets (an analysis using simulations has suggested that at least 300 patients per treatment arm are needed [22]), leading to overfitting, meaning that the model perfectly fits the data set in which it is developed, but performs poorly in new data. One way to solve this sample size issue is by using naturalistic data for model development (23), although for treatment selection, confounders that guide real-world treatment allocation should be considered carefully. With these considerations, collaborations such as the Swedish national quality registers are key to the future of accurate and clinically relevant prediction (13).
ECT Research Deserves More Scientific Attention and Support
Research on ECT has important advantages over research on other treatment modalities in psychiatry. The strong effect sizes (in a distinct time window), the individual variation in effects of ECT, its relatively good controllability, the relatively homogeneous clinical settings in which ECT is performed, and the good setup for longitudinal outcome monitoring provide important advantages for adequate designs for biomarker studies. The current wave of studies on prediction of response to ECT can therefore be considered proof-of-principle studies for testing the potential value for personalized genetic prediction of treatment response in psychiatry and the elucidation of biological mechanisms underlying treatment-associated changes in mental health status.
ECT as an Opener of Plasticity
One hypothesis on the mechanism of action of ECT is the neuroplasticity hypothesis, which was originally based on preclinical animal studies that reported a dose-dependent neurogenesis following ECT (24) and is partly supported by neuroimaging studies (25). Although this mechanism may apply to other depression treatments as well, the onset and effect of ECT are more pronounced, presumably resulting in a “plasticity window.” As relapse rates following ECT discontinuation are high, cognitive and behavioral interventions to consolidate the plasticity changes may have some (synergistic) promise for sustainable mental health care (26). Recent studies provide evidence that cognitive-behavioral therapy may indeed be an effective intervention for sustaining the effects of a successful course of ECT (e.g., [27]).
In summary, Sigström et al. provide a proof-of-principle study on the predictive value of PRSs for response to ECT. With their large-scale longitudinal design embedded in clinical practice, they found that genetic variation underlying bipolar disorder was associated with successful ECT. Although the predictive value was small, this could be considered a step toward the optimization of (transdiagnostic) PRSs and the development of multifactorial prediction models. ECT research provides a very useful context for biomarker research, and future studies hold promise in providing a better understanding of underlying biological processes, and better prediction of individual response to ECT.
1. Depression and Other Common Mental Disorders: Global Health Estimates. Geneva, World Health Organization, 2017Google Scholar
2. : Acute and longer-term outcomes in depressed outpatients requiring one or several treatment steps: a STAR* D report. Am J Psychiatry 2006; 163:1905–1917Link, Google Scholar
3. : Efficacy of electroconvulsive therapy in bipolar versus unipolar major depression: a meta‐analysis. Bipolar Disord 2012; 14:146–150Crossref, Medline, Google Scholar
4. : Electroconvulsive therapy in depressive illness that has not responded to drug treatment. J Affect Disord 2004; 83:121–126Crossref, Medline, Google Scholar
5. : Prediction of electroconvulsive therapy response and remission in major depression: meta-analysis. Br J Psychiatry 2018; 212:71–80Crossref, Medline, Google Scholar
6. : Response of depression to electroconvulsive therapy: a meta-analysis of clinical predictors. J Clin Psychiatry 2015; 76:1374–1384Crossref, Medline, Google Scholar
7. : Potential mechanisms underlying the therapeutic effects of electroconvulsive therapy. Neurosci Bull 2017; 33:339–347Crossref, Medline, Google Scholar
8. : Polygenic risk scores: from research tools to clinical instruments. Genome Med 2020; 12:44Crossref, Medline, Google Scholar
9. : Could polygenic risk scores be useful in psychiatry? A review. JAMA Psychiatry 2021; 78:210–219Crossref, Medline, Google Scholar
10. : Polygenic risk scores for predicting outcomes and treatment response in psychiatry: hope or hype? Int Rev Psychiatry 2022:1–13Crossref, Medline, Google Scholar
11. : Evidence for increased genetic risk load for major depression in patients assigned to electroconvulsive therapy. Am J Med Genet B Neuropsychiatr Genet 2019; 180:35–45Crossref, Medline, Google Scholar
12. : Interrogating associations between polygenic liabilities and electroconvulsive therapy effectiveness. Biol Psychiatry 2022; 91:531–539Crossref, Medline, Google Scholar
13. : Association between polygenic risk scores and outcome of ECT. Am J Psychiatry 2022; 179:844–852Abstract, Google Scholar
14. : Genetic architecture of 11 major psychiatric disorders at biobehavioral, functional genomic, and molecular genetic levels of analysis. Nat Genet 2022; 54:548–559Crossref, Medline, Google Scholar
15. : Measures of early-life behavior and later psychopathology in the LifeCycle Project–EU Child Cohort Network: a cohort description. J Epidemiol (Online ahead of print, November 13, 2021) Google Scholar
16. : The impact of electroconvulsive therapy on the tryptophan-kynurenine metabolic pathway. Brain Behav Immun 2015; 48:48–52Crossref, Medline, Google Scholar
17. : Longitudinal alterations in mRNA expression of the BDNF neurotrophin signaling cascade in blood correlate with changes in depression scores in patients undergoing electroconvulsive therapy. Eur Neuropsychopharmacol 2022; 63:60–70Crossref, Medline, Google Scholar
18. : Machine learning in the prediction of depression treatment outcomes: a systematic review and meta-analysis. Psychol Med 2021; 51:2742–2751Crossref, Medline, Google Scholar
19. : Applications of machine learning algorithms to predict therapeutic outcomes in depression: a meta-analysis and systematic review. J Affect Disord 2018; 241:519–532Crossref, Medline, Google Scholar
20. : Antidepressant drug–specific prediction of depression treatment outcomes from genetic and clinical variables. Sci Rep 2018; 8:5530–5539Crossref, Medline, Google Scholar
21. : Optimizing prediction of response to antidepressant medications using machine learning and integrated genetic, clinical, and demographic data. Transl Psychiatry 2021; 11:381Crossref, Medline, Google Scholar
22. : Sample size requirements for multivariate models to predict between-patient differences in best treatments of major depressive disorder. Clin Psychol Sci 2019; 7:445–461Crossref, Google Scholar
23. : Machine learning methods for developing precision treatment rules with observational data. Behav Res Ther 2019; 120:103412Crossref, Google Scholar
24. : Increased neurogenesis in a model of electroconvulsive therapy. Biol Psychiatry 2000; 47:1043–1049Crossref, Medline, Google Scholar
25. : The neurobiological effects of electroconvulsive therapy studied through magnetic resonance: what have we learned, and where do we go? Biol Psychiatry 2022; 91:540–549Crossref, Medline, Google Scholar
26. : Leveraging neuroplasticity to enhance adaptive learning: the potential for synergistic somatic-behavioral treatment combinations to improve clinical outcomes in depression. Biol Psychiatry 2019; 85:454–465Crossref, Medline, Google Scholar
27. : Cognitive-behavioral therapy as continuation treatment to sustain response after electroconvulsive therapy in depression: a randomized controlled trial. Biol Psychiatry 2014; 76:194–202Crossref, Medline, Google Scholar