Feedback-Driven Trial-by-Trial Learning in Autism Spectrum Disorders
Abstract
Objective:
Impairments in learning are central to autism spectrum disorders. The authors investigated the cognitive and neural basis of these deficits in young adults with autism spectrum disorders using a well-characterized probabilistic reinforcement learning paradigm.
Method:
The probabilistic selection task was implemented among matched participants with autism spectrum disorders (N=22) and with typical development (N=25), aged 18–40 years, using rapid event-related functional MRI. Participants were trained to choose the correct stimulus in high-probability (AB), medium-probability (CD), and low-probability (EF) pairs, presented with valid feedback 80%, 70%, and 60% of the time, respectively. Whole-brain voxel-wise and parametric modulator analyses examined early and late learning during the stimulus and feedback epochs of the task.
Results:
The groups exhibited comparable performance on medium- and low-probability pairs. Typically developing persons showed higher accuracy on the high-probability pair, better win-stay performance (selection of the previously rewarded stimulus on the next trial of that type), and more robust recruitment of the anterior and medial prefrontal cortex during the stimulus epoch, suggesting development of an intact reward-based working memory for recent stimulus values. Throughout the feedback epoch, individuals with autism spectrum disorders exhibited greater recruitment of the anterior cingulate and orbito-frontal cortices compared with individuals with typical development, indicating continuing trial-by-trial activity related to feedback processing.
Conclusions:
Individuals with autism spectrum disorders exhibit learning deficits reflecting impaired ability to develop an effective reward-based working memory to guide stimulus selection. Instead, they continue to rely on trial-by-trial feedback processing to support learning dependent upon engagement of the anterior cingulate and orbito-frontal cortices.
Individuals with autism spectrum disorders display a unique, pervasive pattern of learning abilities and disabilities. Their learning of simple associations, facts, details, and habits is intact (1) as demonstrated by their responsiveness to operant conditioning-based interventions, ability to memorize extensive information about special interests, and insistence on sameness (2). However, they are impaired in goal-directed learning (3), rapid processing of ambiguous stimulus feedback contingencies (4, 5), and generalization of learning to new contexts (6). This impairment greatly interferes with everyday social, language, and classroom learning, which typically is goal-oriented and involves quick, subtle, and inconsistent stimulus/reward sequences (7–9). A mechanistic explanation for this pattern has remained elusive.
Animal, computational modeling, and human neuroimaging studies suggest that learning simple associations and facts typically recruits the hippocampus, whereas the processing of the quick, inconsistent stimuli encountered in everyday life recruits the striatum (10). The striatum receives projections from brainstem nuclei of the dopamine system and generates prediction error signals (dopamine bursting and dips) that train the organism about the reward value of different actions (11). Over time, this process leads to the development of reward-based working memory mediated by the orbitofrontal cortex (12).
As learning becomes more goal-directed and driven by unpredictable feedback, it requires increasing recruitment of cognitive control-related brain regions, including lateral (for information maintenance and manipulation) and anterior (for higher-order rule representation) regions of the prefrontal cortex. This prefrontal involvement permits the online maintenance of the task-related working memories (13) that make possible more complex forms of goal and unpredictable feedback-driven learning. In this more complex learning, prefrontal dysfunction results in a paradoxical pattern of performance. It is relatively harder to learn more predictable pairings of information where using reward-based working memory would be helpful and relatively easier to learn less predictably reinforced pairings of information where the use of reward-based working memory is actually misleading (5).
During learning, organisms must predict future rewards, evaluate errors in those predictions, respond to volatility in signals about the probability with which rewards are received, and recruit cognitive systems when additional effort is required. These processes engage the anterior cingulate cortex, which then signals the orbitofrontal cortex and other prefrontal regions to increase available working memory-related resources (14). To the extent the prefrontal cortex cannot be brought online “proactively” to sustain task- or reward-based working memories, the learner may engage in a less efficient, slower strategy whereby rules and task memories are retrieved from the hippocampus and, to the extent possible, the prefrontal cortex “reactively” on a trial-by-trial basis (15).
In the present study, we investigated the neural mechanisms of learning in young adults with an autism spectrum disorder and with typical development using a probabilistic reinforcement task with both a stimulus and a feedback epoch. Given their impairments in prefrontal functioning (16, 17), we predicted that the autism spectrum disorders group would be less able to accurately encode task- and reward-based working memories to guide responding as demonstrated by greater error rates on high-probability AB trials early in learning, reduced error rates on low-probability EF trials, and reduced sustained recruitment of cognitive control-related regions of the prefrontal cortex during all trials. Second, as found previously (5), we hypothesized that the autism spectrum disorders group would show attenuated reward anticipation during stimulus presentation as reflected in poorer win-stay performance (the selection of the previously rewarded stimulus on the next trial of that type). Finally, given their impairments in reward-based working memory and dysregulated positive feedback processing, we predicted that the autism spectrum disorders group, compared with the typically developing group, would exhibit a more reactive pattern of task performance involving less proactive cognitive control and neural recruitment during the stimulus epoch of the task and greater reactive recruitment of neural circuits of the anterior cingulate cortex during feedback processing, a pattern demonstrated recently in adolescents with autism spectrum disorders (18).
Method
Participants
We studied young adulthood, which has been defined by developmental theorists as spanning ages 18–40 years (19), given its importance for adult outcomes and the fact that it has been understudied in persons with autism spectrum disorders. Given recent concerns about the integrity of small neuroscience studies (20), we conducted a power analysis before initiating this study. Twenty-five subjects per group were selected as the final target sample size to enable us to find a moderate effect size in behavioral and functional MRI (fMRI) analyses. We used our recent fMRI study of another domain of higher cognition (cognitive control), where we examined differences in percent signal change in our main contrast of interest and found an effect size (Cohen’s d) of 0.75 (17). Assuming that a similar effect size would be present in the current study, we determined that a sample of 25 subjects per group would enable us to achieve 80% power at a p value <0.05. We then recruited 30 individuals with autism spectrum disorders, versus 25, to allow for 20% data loss due to task difficulty, poor toleration of the scanner, and motion. Additional confidence in power estimates was provided by a simulation analysis described by Desmond and Glover (21), in which power curves were derived with an alpha of 0.002 (two-tailed) for a fixed signal change of 50% and fixed inter- and intrasubject variability showing that 24 subjects per group in a two-group study were required to achieve 80% power. Twenty-two of those with autism spectrum disorders were able to complete the task with AB and CD pair accuracy above chance (screening criteria described with the task description), leaving a final sample size of 22 adults with autism spectrum disorders (mean age=22.95 years [SD=5.11]; range: 18–35 years) and 25 adults with typical development (mean age=23.36 years [SD=4.15]; range=18–34 years), who were matched on age, gender, and IQ. Demographic characteristics of the study participants are presented in Table 1. Based on the male-to-female gender ratio of approximately 4:1 in the population (22), four women were enrolled in each group. Recruitment was conducted through psychiatrists, speech and language pathologists, advocacy groups, psychologists, state-funded centers for persons with developmental disabilities, and the MIND (Medical Investigation of Neurodevelopmental Disorders) Institute Subject Tracking System database. All participants had a full-scale IQ >70 on the Wechsler Abbreviated Scale of Intelligence (23). We enrolled no individuals who could read or speak Japanese. Of the 22 enrolled participants with autism spectrum disorders, five were diagnosed with high-functioning autism, 16 were diagnosed with Asperger’s syndrome, and one with pervasive developmental disorder, not otherwise specified, according to DSM-IV-TR criteria and the Autism Diagnostic Observation Schedule-Generic (24). Exclusion criteria for those with autism spectrum disorders included diagnoses of autism with known genetic etiologies and current diagnoses of depression, anxiety disorders, or psychosis. Individuals taking antipsychotic medications, known to interact with the dopamine system, were also excluded. Individuals taking psychostimulants (two in the autism spectrum disorders group) were asked to discontinue these medications for 48 hours before the start of the study. Five remaining participants in the autism group were taking selective serotonin reuptake inhibitors, and one was taking atomoxetine.
Autism Spectrum Disorders Group (N=22) | Typically Developing Group (N=25) | |||||
---|---|---|---|---|---|---|
Variable | Mean | SD | Range | Mean | SD | Range |
Age (years) | 22.95 | 5.11 | 18–35 | 23.36 | 4.15 | 18–34 |
Gender ratio (male:female) | 4.5:1 | – | – | 4:1 | – | – |
Full-scale IQ | 112.64 | 12.44 | 94–140 | 114.17 | 11.51 | 91–132 |
Verbal IQ | 110.82 | 13.07 | 91–137 | 112.54 | 12.32 | 86–129 |
Performance IQ | 112.18 | 12.94 | 84–134 | 112.04 | 11.53 | 86–131 |
Autism Diagnostic Observation Schedule-Generic rating | ||||||
Communication | 3.55 | 1.63 | 2–7 | – | – | – |
Social interaction | 7.45 | 1.68 | 4–12 | – | – | – |
Total | 11.00 | 2.79 | 7–19 | – | – | – |
Stereotyped behavior and restricted interests | 1.50 | 1.22 | 0–4 | – | – | – |
Task accuracy | ||||||
High-probability paira | 0.76 | 0.16 | 0.51–0.98 | 0.88 | 0.11 | 0.52–1.00 |
Medium-probability pair | 0.77 | 0.16 | 0.52–1.00 | 0.82 | 0.12 | 0.60–1.00 |
Low-probability pair | 0.65 | 0.19 | 0.22–0.98 | 0.64 | 0.21 | 0.09–0.97 |
Win-stay performanceb | 0.73 | 0.16 | 0.36–1.00 | 0.82 | 0.11 | 0.59–0.97 |
Lose-shift analysis | 0.35 | 0.16 | 0.04–0.64 | 0.33 | 0.13 | 0.11–0.57 |
TABLE 1. Demographic Characteristics and Univariate Analyses of Task Performance
After receiving complete description of the study, all individuals gave written consent to participate. The study was approved by the institutional review board of the University of California, Davis.
Measures
Autism Diagnostic Observation Schedule-Generic (24).
We used module 4 of the Autism Diagnostic Observation Schedule-Generic, which is a semistructured interactive session and interview protocol. Participants are rated based on their responses to standardized social “presses.” An algorithm score that combines ratings for communication and reciprocal social interaction is the basis for diagnostic classification.
Probabilistic selection task (5, 10).
Three stimulus pairs, each consisting of two Japanese characters and referred to as AB, CD, and EF (or the high-, medium-, and low-probability pairs), were presented in randomized order. The Japanese characters representing the three pairs also were randomized. Participants were instructed to select the character they thought had the greatest likelihood of being correct as quickly and accurately as possible. Feedback consisted of either “Correct!” (printed in blue), “Incorrect” (printed in red), or “No response detected.” For high-probability AB trials, a choice of stimulus A led to valid positive feedback 80% of the time, while invalid feedback (i.e., B is correct and A is incorrect) was given 20% of the time. For CD and EF trials, valid feedback was given 70% and 60% of the time, respectively. Reinforcement contingencies were tied to stimulus type versus the side it was presented on. There were four runs of 72 trials. Given past findings that early and late learning can be discriminated in this task because reward-based working memory takes time to develop (5, 25), we delineated runs 1 and 2 as the early learning stage and runs 3 and 4 as the later learning stage of the task. Twelve “catch trials” (a fixation cross alone) were included per run. Trials consisted of a stimulus epoch (1.5 seconds); a jittered interstimulus interval, with a mean duration of 3 seconds and a range of 2–4 seconds; a feedback epoch in which participants were presented with the words “correct” or “incorrect” (0.5 seconds); and a jittered intertrial interval, with a mean duration of 2.8 seconds and a range of 2–7 seconds (Figure 1). Only participants scoring above 50% on high-probability AB trials and medium-probability CD trials were retained.
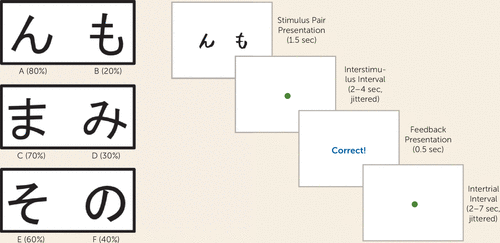
FIGURE 1. The Probabilistic Selection Taska
a Stimulus pairs and valid and invalid probabilities are presented (left), as well as a schematic of the probabilistic selection task, including the stimulus epoch, interstimulus interval, feedback epoch, and intertrial interval (right). Sec=seconds.
Data Analysis
Behavioral data.
Univariate t tests and Bayesian state-space analyses were used to examine learning. For additional methodological information about the state-space model, see the data supplement accompanying the online version of this article. Trial-to-trial behavior as a function of feedback was investigated in a “win-stay” analysis (exploitation of rewarded stimuli, defined as the percentage of trials following positive feedback in which the participants chose the same stimulus on the next presentation of that stimulus) and a “lose-shift” analysis (shifting to the alternative choice when stimuli are not rewarded, defined as the percentage of trials following negative feedback in which participants shifted to the other stimulus) using t tests completed in SPSS Version 21.0 (IBM, Armonk, N.Y.).
Imaging Data Acquisition
Structural and functional images were acquired on a Siemens 3T TIM Trio (Siemens Medical Solutions, Erlangen, Germany) MRI scanner, with an eight-channel phased array head coil. Cushions and pretraining in a mock scanner were used to minimize head motion. Earplugs and headphones were used to dampen scanner noise and to enable communication. Structural and functional images were acquired at each scan session. Thirty-six interleaved whole-brain axial slices (thickness=4.0 mm) were acquired in a plane parallel to the anterior commissure-posterior commissure line using a single-shot T2*-weighted echo-planar sequence (repetition time=2,000 ms; echo time=24 ms; flip angle=90°; field of view=22 cm; 64×64 voxels). The first two acquisitions of each run were discarded to allow for T1 equilibration. High-resolution T1-weighted images were acquired using a magnetization prepared rapid acquisition gradient-echo (MPRAGE) pulse sequence (repetition time=2,100 ms; echo time=2.56 ms; flip angle=8°; field of view=256 mm; 256×224 voxels; isotropic voxel size of 1 mm) to obtain structural images in the same plane as the functional images. The task was presented on a desktop computer interfaced with a response box and color liquid-crystal display projector using the E-Prime 1.0 software package (Psychology Software Tools, Sharpsburg, Pa.). Stimuli were projected onto a screen viewed through a mirror attached to the head coil.
Data Preprocessing and Analysis
Imaging data were preprocessed and then analyzed using SPM8 (Wellcome Trust Centre for Neuroimaging, University College London). Images underwent slice time acquisition correction, realignment to correct for motion, spatial normalization to the Montreal Neurological Institute template, and then smoothing with a three-dimensional Gaussian kernel (8 mm full width at half maximum). They were then visually checked for quality. After eliminating participants with performance that was not better than chance, no additional participants were excluded for excess motion (>3 mm translational or 3 degrees rotational movement across the session) or as outliers in percent signal change (as calculated by the art_groupoutlier function in the ArtRepair toolbox [http://cibsr.stanford.edu/tools/human-brain-project/artrepair-software.html] for SPM8). Comparisons of six movement parameters across the age and diagnostic groups showed no significant group differences in means (all p values >0.18) or in root mean square value of the frame displacement derivative (26) calculated from realignment parameters (t=1.88, df=45, p=0.07). Estimations were made using the ordinary least squares method, SPM’s canonical haemodynamic response function, a high-pass filter of 100s, and SPM’s first-order autoregressive model. Motion estimates were included as nuisance regressors. Regressors were included to indicate participants who were and were not taking medications. The stimulus and feedback epochs of the task were modeled separately. At the first level, regressors were included for each run and each trial type for both the early (runs 1 and 2) and late (runs 3 and 4) phases of the task. In the second-level analysis, we conducted t tests on the contrast images for each group for both the stimulus and feedback epochs for both early and late phases. Bayesian state-space learning curves for each trial type for each individual participant were used as a parametric modulator in the general linear model during the stimulus epoch of the task to assess brain regions associated with the probability of having learned the pair. Analyses of both the stimulus and feedback epochs included only correct trials with valid feedback. There were no group differences in the numbers of correct trials included in the analyses. We thresholded random-effects analyses at a voxel-wise height threshold of p<0.01 (27) and report clusters that are family-wise error-corrected at a p value <0.05 across the whole brain.
Results
Hypothesis 1: The Autism Spectrum Disorders Group Exhibits Deficits in Prefrontal Function
Behavior.
As shown in Table 1, univariate analyses demonstrated that the autism spectrum disorders group was significantly less accurate on the high-probability AB pair than the typically developing group early in learning. These were based on a 2×3 group-by-trial type analysis of variance. There was a main effect of group (F=5.07, df=1, 140, p=0.02), a main effect of trial type (F=13.28, df=2, 140, p=0.001), and a group-by-trial type interaction (F=3.22, df=2, 140, p=0.04) during early learning. Follow-up tests showed that the interaction was driven by significantly worse performance by the autism group on the AB pair (t=2.8, df=45, p=0.007).
As described more extensively in the online data supplement, state-space learning curves also were computed to use in fMRI analyses of learning. The median state-space learning curves and their 90% confidence intervals for the typically developing and autism groups for the three pairs (AB, CD, and EF) are presented in Figure 2. From these curves, it is possible to compute, by sampling, the trial-to-trial probability of the groups being different using certainty plots. For example, there were significant differences (typically developing > autism) for the AB pair on trials 2, 3, 6, 51–62, 75–77, and 85–96. In addition to the trial-by-trial measures, across the task, the probability of the typically developing participants outperforming the autism group was significant at a p value <0.001 for the AB and CD pairs and nonsignificant for the EF pair (p=0.83). An analysis of average learning trial (the trial by which the probability of having learned exceeded 99% for the sample) showed that learning was achieved by trial 40, which occurred close to the end of block 2, validating blocks 1 and 2 as representative of early learning.
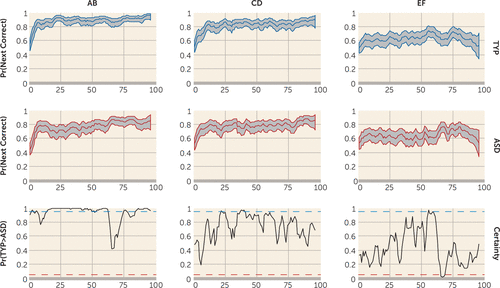
FIGURE 2. State-Space Learning Curves and Certainty Plots for the Autism Spectrum Disorders and Typically Developing Groupsa
a The bottom row consists of certainty plots depicting trials for which the probability of typical development > autism spectrum disorders exceeds 95% certainty and becomes significant (blue dotted line) or for which the probability of autism spectrum disorders > typical development exceeds 95% certainly (red dotted line). There were significant differences in typical development > autism for the AB pair on trials 2, 3, 6, 51–62, 75–77, and 85–96. For CD trials, there were significant differences in typical development > autism spectrum disorders on trials 22 and 37–40. For EF trials, there were significant differences in typical development > autism spectrum disorders on trial 56 and in autism spectrum disorders > typical development on trials 66–69. ASD=autism spectrum disorders; Pr=probability; TYP=typical development.
Imaging.
To investigate brain regions associated with the probability of having learned during the stimulus epoch of the task, we used a multiple regression analysis with the Bayesian state-space learning curves for each trial type for each individual participant as a parametric modulator in the general linear model. Whole-brain voxel-wise one-tailed t tests were conducted on the analysis results, revealing two results of interest. First, as shown in Figure 3A, early in the task, for the typically developing group, recruitment of the anterior prefrontal cortex (Brodmann’s area [BA] 10; Talairach coordinates [x, y, z]: 12, 47, 14) and the rostral anterior cingulate cortex (BA 32; Talairach coordinates [x, y, z]: 0, 47, 9) was associated with having learned the AB pair significantly more than recruitment of these regions in the autism group (t=2.07, df=43, p=0.04, family-wise error-corrected). Second, as shown in Figure 3B, during the stimulus epoch through both stages of the task, the canonical model revealed that there was significantly greater prefrontal activation in the typically developing group than in the autism group (t=3.87, df=45, p<0.001, family-wise error-corrected) in the superior (BA 8; Talairach coordinates [x, y, z]: 36, 25, 40) and anterior (BA 10; Talairach coordinates [x, y, z]: 30, 56, 17) prefrontal cortex.
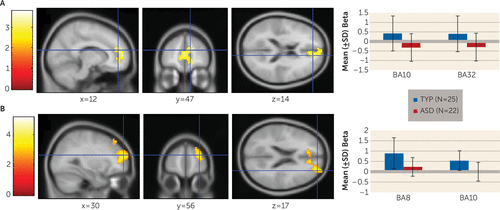
FIGURE 3. Group Differences in Neural Recruitment During the Stimulus Epocha
a The top panel (A) represents the learning curve analysis. Neural recruitment on AB trials in the early phase of the task was significantly greater in the typically developing group compared with the autism spectrum disorders group in the anterior and rostral prefrontal cortex at a family-wise error-corrected p value <0.05. The bottom panel (B) shows results of the canonical model. The typically developing group exhibited greater neural recruitment on all trials through both phases of the task in the superior and anterior regions of the prefrontal cortex at a family-wise error-corrected p value <0.05. Error bars represent standard deviations. ASD=autism spectrum disorders; BA=Brodmann’s area; TYP=typical development.
Hypothesis 2: The Autism Spectrum Disorders Group Exhibits Dysregulated Feedback Processing
Behavior.
Two-tailed t tests demonstrated that individuals with autism spectrum disorders performed significantly worse than typically developing individuals at winning and staying on all trial types throughout the task (t=2.31, df=45, p=0.03; Cohen’s d=0.68), although they did not differ from the typically developing group on losing and shifting (p=0.64) (see Table 1)
Hypothesis 3: The Autism Spectrum Disorders Group Exhibits Feedback-Driven Learning
Imaging.
Whole-brain voxel-wise one-tailed t tests demonstrated that the autism spectrum disorders group exhibited significantly greater recruitment of rostral areas of the anterior cingulate cortex (BA 24; Talairach coordinates [x, y, z]: –6, 26, –3 and BA 32; Talairach coordinates [x, y, z]: 0, 35, –7) and the orbito-frontal cortex (BA 11; Talairach coordinates [x, y, z]: –9, 22, –19) during both the early- and late-feedback stages of the task compared with the typically developing group (t=2.28, df=45, p=0.03, family-wise error-corrected), suggesting that they continued to use these regions throughout the task (see Figure 4).
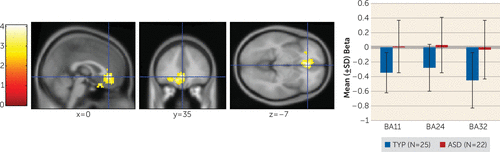
FIGURE 4. Group Differences in Neural Recruitment During the Feedback Epocha
a The autism spectrum disorders group exhibited greater neural recruitment than the typically developing group in the rostral anterior cingulate and the orbito-frontal cortex on all trials through the early- and late-feedback epochs of the task at a family-wise error-corrected p value <0.05. Error bars represent standard deviations. ASD=autism spectrum disorders; BA=Brodmann’s area; TYP=typical development.
One 26-year-old male participant in the autism spectrum disorders group, who was a successful cashier in a grocery store at the time of the study and had been promoted to working on the store’s pricing integrity and internal marketing when we contacted him again, reported on the experience of being in the study. He found the functional MRI task to be highly challenging and remembered needing to maintain a memory for associations for one event after another. He told us that the task made him feel as though he had studied for a test but had difficulty remembering the correct answers. He also reported being surprised at how many times he actually gave the right responses. He believes his success at his job is related to strengths he demonstrated while completing our task. He reports being better at learning when there is adequate repetition. He feels that learning in this way helps him to form associations between concepts, which he uses when writing advertisements that are played on the store’s public address system. These advertisements involve sales pitches that are cleverly combined with the items to be sold, for example, “rustling up bargains on steak.”
Discussion
We examined performance of young adults with autism spectrum disorders on a task simulating real-life feedback processing. Individuals with autism spectrum disorders showed learning deficits related to impairment in the ability to build a reward-based working memory representation. They exhibited poorer performance on the simplest AB pair. During the stimulus epoch, they showed reduced medial prefrontal activation associated with the probability of having learned the AB pair and less superior and anterior prefrontal cortex recruitment early and late in the task for all trial types. Additionally, they exhibited dysregulated feedback processing and made fewer previously rewarded choices. This is likely the cause of their poor performance on the AB pairs, which involved receiving the most positive feedback. In contrast to the typically developing group who developed a reward-based working memory, during the feedback phase of the task, the autism group persisted in recruiting brain regions associated with feedback-based trial-by-trial learning.
To our knowledge, this study is the first fMRI investigation of reinforcement learning with probabilistic feedback in young adults with autism spectrum disorders. Most other studies of learning and reward have examined aspects of the social motivation theory (28), which proposes that individuals with autism spectrum disorders exhibit early impairments in learning from social rewards provided by caregivers, leading to a cascade of atypical cognitive, social, and language development. However, the social motivation theory does not 1) provide a model for the full range of autism-related learning and reward-processing impairments, 2) address when problems in the learning process occur, or 3) explain learning deficits in nonsocial contexts.
Two perspectives from the literature may help interpret our findings. First, we examined the results in light of the widely held neurocognitive view that autism spectrum disorders involve intact simple versus complex information processing (1). Indeed, an fMRI study applying this view to learning suggested that the autism group exhibited reductions in the recruitment of prefrontal brain regions and reduced prefrontal functional connectivity compared with individuals with typical development (3). However, given that in the present study participants with autism spectrum disorders performed significantly worse on the simplest stimulus pair and equivalently on more difficult ones, a deficit in simple versus complex information processing does not provide a satisfactory explanation of our results.
Second, studies of social motivation theory demonstrate that persons with autism anticipate and seek social (29, 30), and even nonsocial (5, 31, 32), rewards less than persons with typical development and show decreased recruitment of striatal brain regions. The first study of reward processing in adults with autism spectrum disorders provides further support for the idea of dysregulated feedback processing. In that study, the autism group exhibited increased ventromedial prefrontal and anterior cingulate recruitment (33) in response to feedback compared with typically developing adults, suggesting that they may have experienced greater arousal, conflict, and signaling for the increased allocation of attention when evaluated (34). Results of our present study are consistent with both these points of view.
Findings provide several insights that may inform intervention. First, psychosocial and neural retraining, as well as psychopharmacological interventions that enhance dopaminergic or noradrenergic function in the prefrontal cortex and improve the ability to maintain information online, may secondarily improve learning. Second, the ability to experience positive feedback and/or rewards and to orient behavior toward them may be compromised in individuals with autism spectrum disorders (35). Consequently, it may be especially important to make positive feedback explicit and salient in academic and intervention settings. Indeed, this strategy already is used in autism treatments for young children, including in early intensive-behavioral intervention, in which praise is delivered using exaggerated positive affect (e.g., reference 36), in social skills groups for high-functioning school-age and adolescent children, which use positive behavioral supports in an enjoyable setting (37), and in coaching models that teach parents to deliver feedback clearly and positively (38). Future studies can help refine our understanding of the mechanisms by which positive feedback is effective.
It remains unclear whether the elevated cingulate activation constitutes an intervention target. In the absence of a fully functional prefrontal cortex, enhanced cingulate recruitment may be a marker of a successful compensatory strategy. However, sustained anterior cingulate activation has been associated with anxiety disorders (39), poorer emotion regulation (40), and developmental immaturity (41–43). Future investigations of the effectiveness of the anterior cingulate in error monitoring and in signaling the need for increased working memory-related resources in individuals with autism spectrum disorders are needed.
There are several limitations of the present study. First, while the sample size was close to 60 participants, 20% of the recruited autism spectrum disorders group could not perform the task. This limits the generalizability of our findings. Future studies should include simpler tasks. Second, sample size calculations were based on power analysis techniques available at the inception of the study. More sophisticated methods, including region-of-interest-based analyses (44), and correction for multiple comparisons (45) are currently available. This may lead to larger, more replicable studies (20) and to sufficiently large samples to permit subgroup analyses of the heterogeneous autism spectrum disorders phenotype (46). Third, the 18- to 40-year-old period is long. However, it is relevant to point out that we examined age effects in the imaging analyses and found none.
In summary, we provide evidence that learning impairments in young adults with autism spectrum disorders reflect a weakness in the ability to integrate positive reward-related information into working memory and a tendency to rely more heavily on trial-by-trial feedback-based learning. Future studies are needed that use more standardized paradigms and a wider range of both common and idiosyncratic rewards, that examine other forms of learning, and that investigate the effect of learning impairments on daily functioning and mental health.
1 : Neuropsychologic functioning in autism: profile of a complex information processing disorder. J Int Neuropsychol Soc 1997; 3:303–316Crossref, Medline, Google Scholar
2 : Variety is not the spice of life for people with autism spectrum disorders: frequency ratings of central, variable and inappropriate aspects of common real-life events. J Autism Dev Disord 2010; 40:730–742Crossref, Medline, Google Scholar
3 : Distinctive neural processes during learning in autism. Cereb Cortex 2012; 22:937–950Crossref, Medline, Google Scholar
4 : Differential fear conditioning in Asperger’s syndrome: implications for an amygdala theory of autism. Neuropsychologia 2007; 45:2125–2134Crossref, Medline, Google Scholar
5 : Probabilistic reinforcement learning in adults with autism spectrum disorders. Autism Res 2011; 4:109–120Crossref, Medline, Google Scholar
6 : An implicit technology of generalization. J Appl Behav Anal 1977; 10:349–367Crossref, Medline, Google Scholar
7 : Reduced behavioral flexibility in autism spectrum disorders. Neuropsychology 2013; 27:152–160Crossref, Medline, Google Scholar
8 : Learning from social rewards predicts individual differences in self-reported social ability. J Exp Psychol Gen 2013; 143:332–339Crossref, Medline, Google Scholar
9 : Transitive inference in adults with autism spectrum disorders. Cogn Affect Behav Neurosci 2011; 11:437–449Crossref, Medline, Google Scholar
10 : By carrot or by stick: cognitive reinforcement learning in parkinsonism. Science 2004; 306:1940–1943Crossref, Medline, Google Scholar
11 : A neural substrate of prediction and reward. Science 1997; 275:1593–1599Crossref, Medline, Google Scholar
12 : Learning theory: a driving force in understanding orbitofrontal function. Neurobiol Learn Mem 2013; 108:22–27Crossref, Medline, Google Scholar
13 : How much of reinforcement learning is working memory, not reinforcement learning? a behavioral, computational, and neurogenetic analysis. Eur J Neurosci 2012; 35:1024–1035Crossref, Medline, Google Scholar
14 : From conflict management to reward-based decision making: actors and critics in primate medial frontal cortex. Neurosci Biobehav Rev (Epub ahead of print, Nov 15, 2013)Google Scholar
15 : The variable nature of cognitive control: a dual mechanisms framework. Trends Cogn Sci 2012; 16:106–113Crossref, Medline, Google Scholar
16 : Cognitive control in autism spectrum disorders. Int J Dev Neurosci 2008; 26:239–247Crossref, Medline, Google Scholar
17 : The neural substrates of cognitive control deficits in autism spectrum disorders. Neuropsychologia 2009; 47:2515–2526Crossref, Medline, Google Scholar
18 : The development of the neural substrates of cognitive control in adolescents with autism spectrum disorders. Biol Psychiatry (Epub ahead of print, Oct 24, 2013)Google Scholar
19 : Identity: Youth and Crisis, 1st ed. New York, WW Norton, 1968, p 336Google Scholar
20 : Power failure: why small sample size undermines the reliability of neuroscience. Nat Rev Neurosci 2013; 14:365–376Crossref, Medline, Google Scholar
21 : Estimating sample size in functional MRI (fMRI) neuroimaging studies: statistical power analyses. J Neurosci Methods 2002; 118:115–128Crossref, Medline, Google Scholar
22 : Autism spectrum and attention-deficit disorders in girls: some neuropsychological aspects. Eur Child Adolesc Psychiatry 2000; 9:180–185Crossref, Medline, Google Scholar
23 : Wechsler Abbreviated Scale of Intelligence (WASI). San Antonio, Tex, Harcourt Assessment, 1999Google Scholar
24 : The Autism Diagnostic Observation Schedule-Generic: a standard measure of social and communication deficits associated with the spectrum of autism. J Autism Dev Disord 2000; 30:205–223Crossref, Medline, Google Scholar
25 : Selective reinforcement learning deficits in schizophrenia support predictions from computational models of striatal-cortical dysfunction. Biol Psychiatry 2007; 62:756–764Crossref, Medline, Google Scholar
26 : Spurious but systematic correlations in functional connectivity MRI networks arise from subject motion. Neuroimage 2012; 59:2142–2154Crossref, Medline, Google Scholar
27 : Detecting activations in PET and fMRI: levels of inference and power. Neuroimage 1996; 4:223–235Crossref, Medline, Google Scholar
28 : The social motivation theory of autism. Trends Cogn Sci 2012; 16:231–239Crossref, Medline, Google Scholar
29 : Reward circuitry function in autism during face anticipation and outcomes. J Autism Dev Disord 2012; 42:147–160Crossref, Medline, Google Scholar
30 : Reward circuitry function in autism spectrum disorders. Soc Cogn Affect Neurosci 2012; 7:160–172Crossref, Medline, Google Scholar
31 : Reward system dysfunction in autism spectrum disorders. Soc Cogn Affect Neurosci 2013; 8:565–572Crossref, Medline, Google Scholar
32 : Reward processing in autism. Autism Res 2010; 3:53–67Medline, Google Scholar
33 : Neural correlates of reward in autism. Br J Psychiatry 2008; 192:19–24Crossref, Medline, Google Scholar
34 : Temporal dynamics reveal atypical brain response to social exclusion in autism. Dev Cogn Neurosci 2011; 1:271–279Crossref, Medline, Google Scholar
35 : Enhanced decision making and risk avoidance in high-functioning autism spectrum disorder. Neuropsychology 2013; 28:222–228Crossref, Medline, Google Scholar
36 : Randomized, controlled trial of an intervention for toddlers with autism: the Early Start Denver Model. Pediatrics 2010; 125:e17–e23Crossref, Medline, Google Scholar
37 : A social adjustment enhancement intervention for high functioning autism, Asperger’s syndrome, and pervasive developmental disorder NOS. J Autism Dev Disord 2004; 34:649–668Crossref, Medline, Google Scholar
38 : The effectiveness of parent-child interaction therapy for families of children on the autism spectrum. J Autism Dev Disord 2008; 38:1767–1776Crossref, Medline, Google Scholar
39 : Emotional processing in anterior cingulate and medial prefrontal cortex. Trends Cogn Sci 2011; 15:85–93Crossref, Medline, Google Scholar
40 : The role of emotion regulation in autism spectrum disorder. J Am Acad Child Adolesc Psychiatry 2013; 52:679–688Crossref, Medline, Google Scholar
41 : The influence of personality on neural mechanisms of observational fear and reward learning. Neuropsychologia 2008; 46:2709–2724Crossref, Medline, Google Scholar
42 : Aversive learning in adolescents: modulation by amygdala-prefrontal and amygdala-hippocampal connectivity and neuroticism. Neuropsychopharmacology 2013;39:875–884Crossref, Medline, Google Scholar
43 : Neural correlates of developmental differences in risk estimation and feedback processing. Neuropsychologia 2006; 44:2158–2170Crossref, Medline, Google Scholar
44 : Power calculation for group fMRI studies accounting for arbitrary design and temporal autocorrelation. Neuroimage 2008; 39:261–268Crossref, Medline, Google Scholar
45 : Development of PowerMap: a software package for statistical power calculation in neuroimaging studies. Neuroinformatics 2012; 10:351–365Crossref, Medline, Google Scholar
46 : Autism: many genes, common pathways? Cell 2008; 135:391–395Crossref, Medline, Google Scholar