Methamphetamine-Induced Disruption of Frontostriatal Reward Learning Signals: Relation to Psychotic Symptoms
Abstract
Objective
Frontostriatal circuitry is critical to learning processes, and its disruption may underlie maladaptive decision making and the generation of psychotic symptoms in schizophrenia. However, there is a paucity of evidence directly examining the role of modulatory neurotransmitters on frontostriatal function in humans. In order to probe the effects of modulation on frontostriatal circuitry during learning and to test whether disruptions in learning processes may be related to the pathogenesis of psychosis, the authors explored the brain representations of reward prediction error and incentive value, two key reinforcement learning parameters, before and after methamphetamine challenge.
Method
Healthy volunteers (N=18) underwent functional MRI (fMRI) scanning while performing a reward learning task on three occasions: after placebo, after methamphetamine infusion (0.3 mg/kg body weight), and after pretreatment with 400 mg of amisulpride and then methamphetamine infusion. Brain fMRI representations of learning signals, calculated using a reinforcement Q-learning algorithm, were compared across drug conditions.
Results
In the placebo condition, reward prediction error was coded in the ventral striatum bilaterally and incentive value in the ventromedial prefrontal cortex bilaterally. Reward prediction error and incentive value signals were disrupted by methamphetamine in the left nucleus accumbens and left ventromedial prefrontal cortex, respectively. Psychotic symptoms were significantly correlated with incentive value disruption in the ventromedial prefrontal and posterior cingulate cortex. Amisulpride pretreatment did not significantly alter methamphetamine-induced effects.
Conclusions
The results demonstrate that methamphetamine impairs brain representations of computational parameters that underpin learning. They also demonstrate a significant link between psychosis and abnormal monoamine-regulated learning signals in the prefrontal and cingulate cortices.
The processes of making a prediction, acting on the prediction, registering any mismatch between expectation and outcome, and then updating future predictions in the light of any mismatch are critical factors underpinning learning and decision making. Considerable evidence demonstrates that the striatum is a key structure involved in registering mismatches between expectation and outcome (prediction errors) (1). Representations of the value of stimuli and actions (incentive values) are encoded in ventral and medial parts of the frontal cortex (2). Available evidence, mainly from experimental animal research, suggests that the monoamine neuromodulators norepinephrine, serotonin, and especially dopamine play an important role in neural computations of incentive value and reward prediction error (3–6). These findings from basic neuroscience have considerable implications for our understanding of psychiatric illness, given that many psychiatric disorders have been shown to involve frontal or striatal pathology, monoamine neurochemical imbalances, and abnormalities in learning and decision making (7).
It has been suggested that in schizophrenia, abnormal learning of associations and inappropriate attribution of incentive salience and value could be key processes underpinning the development of psychotic symptoms (8, 9). Functional MRI (fMRI) studies in unmedicated patients and in patients with active psychotic symptoms have shown abnormal frontal, striatal, and limbic markers of prediction error or stimulus value during or after associative learning, with some evidence of a correlation between abnormal brain learning indices and symptom severity (10–15). In this study, we sought to explore further the link between prediction error-based learning, brain mechanisms of valuation, and psychotic symptom formation by examining the ability of methamphetamine to induce transient psychotic symptoms in healthy volunteers and relating this to the drug’s effect on neural learning signals.
Amphetamines can induce psychotic-like symptoms even after a single administration, especially at high doses (16–18). Experimental administration of amphetamines and related stimulants, whether in humans or animals, has proved a long-standing useful preclinical model of aspects of the pathophysiology of schizophrenia. The mechanisms through which amphetamines cause psychotic symptoms are unknown, but through their effects on release of dopamine (and/or other monoamines) they may induce a disruption of frontostriatal reinforcement value and prediction error learning signals, which in turn may contribute to the generation of symptoms.
With this in mind, we adopted an approach characterizing 1) the impact on fMRI measures of administration of an amphetamine (methamphetamine) on neural computations of incentive value and prediction error during learning in healthy volunteers, and 2) the degree to which methamphetamine-induced disruption of these neural computations is related to drug-induced changes in mental state. In a third session, participants received pretreatment with the second-generation antipsychotic amisulpride, a potent dopamine D2 receptor antagonist, before methamphetamine was administered. We included amisulpride in the study in the hope of gaining insight into the mechanism of action of antipsychotic medication and clarifying the neurochemistry of any changes in mental state or brain learning signals induced by methamphetamine.
We hypothesized that administering methamphetamine would impair reinforcement learning and disrupt frontal and striatal learning signals. We hypothesized furthermore that individual differences in the degree of frontal and striatal reinforcement learning signal disruption would be associated with individual differences in the degree to which the drug induced psychotic symptoms. We reasoned that if these hypotheses were to be confirmed, it would strengthen the evidence supporting a disruption in frontostriatal learning processes in the generation of psychotic symptoms in schizophrenia and other psychiatric disorders. A third hypothesis, investigating the mechanism of action of antipsychotic medication and the precise neurochemical basis of methamphetamine-induced changes, was that pretreatment with amisulpride prior to methamphetamine administration would mitigate any abnormalities induced by methamphetamine.
Method
Participants and Pharmacological Conditions
The study was approved by the Cambridgeshire 2 National Health Service research ethics committee. Eighteen healthy volunteers (11 of them men; mean age, 25.3 years [SD=4.9]) without psychiatric or neurological disorders or contraindications for MRI gave written informed consent and were included in the study. Participants attended on three visits, separated by at least 1 week. In one visit, they received an infusion over 10 minutes with a methamphetamine solution (0.3 mg/kg of body weight), approximately 1 hour before the scan, and a placebo tablet. In another visit, participants received the intravenous methamphetamine as described above, and they were given an amisulpride tablet (400 mg) approximately 1 hour before the infusion. In the third visit, they received a saline infusion and a placebo tablet. The order of the visits was pseudorandomized for each participant in a counterbalanced manner. Participants, researchers who administered fMRI, and psychiatrists who measured mental state were all blind to the pharmacological condition of the visit. One of the male participants was excluded because of an error during drug administration.
Reinforcement Learning Task
During the fMRI scan, participants carried out an instrumental discrimination learning task with probabilistic feedback that required making choices to maximize wins and minimize losses (Figure 1; see also the data supplement that accompanies the online edition of this article). In each trial, one of three possible pairs of abstract pictures was randomly presented: rewarding, punishing, or neutral. There were 90 trials per visit in total. Selection of one of the pictures (by button press) would lead to a particular outcome (a picture of a £1 coin in rewarding trials, a red cross over a £1 coin in punishing trials, and a purple circle the same size of the coin in neutral trials) with a 70% probability, whereas selection of the other picture led to the outcome with 30% probability.
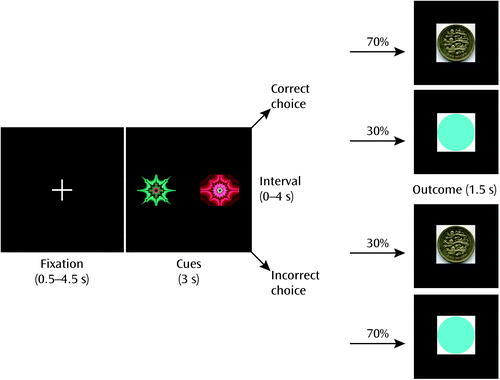
a After a variable intertrial interval, participants were presented one of three pairs of stimuli, each corresponding to a gain, loss, or neutral trial (only gain trial displayed). The high-probability cue led to the main outcome (in this case, a £1 win) seven out of 10 times, whereas the low-probability cue provided the main outcome only 30% of the time.
Rating Scales and Behavioral Analyses
Immediately after the fMRI scan, participants were interviewed by an experienced psychiatrist who had passed the membership examination of the Royal College of Psychiatrists to measure the severity of any mild (prodromal) psychotic symptoms (Comprehensive Assessment of At-Risk Mental States, subscales 1.1, 1.2, and 1.3) (19).
Computational Model
We estimated reward prediction error and incentive value parameters for each trial by following a basic Q-learning algorithm (20), as described elsewhere (11, 21; see also the online Figure 1; see also the data supplement).
fMRI Data Acquisition and Analysis
Brain imaging data were collected using a 3-T Siemens TIM Trio system and analyzed in the FMRIB Software Library (FSL; http://fsl.fmrib.ox.ac.uk/fsl/fslwiki/). (Details of data acquisition and preprocessing are provided in the online Figure 1; see also the data supplement.) Ten explanatory variables were defined in our statistical model: 1) incentive value of the chosen cue during reward trials as estimated by our computational model; 2) incentive value of the chosen cue during punishment trials; 3) incentive value of the chosen cue during neutral trials; 4) onset of reward cue; 5) onset of punishment cue; 6) onset of neutral cue (no parametric modulator was used for these three onset variables); 7) reward prediction error during valenced outcomes (reward or punishment trials); 8) prediction error during neutral outcomes; 9) outcomes on reward or punishment trials; and 10) outcomes on neutral trials. We decided to include both valences in the same reward prediction error regressor because of evidence that the mesostriatal reward prediction error signal is coded in the same way in reward and punishment trials (22). Incentive values were coded separately for reward and punishment trials because of previous evidence that expected values are coded in different frontal regions according to valence (23). All these regressors were modeled as 2-second events and were convolved with a canonical double gamma function. Temporal derivatives of the events were added to the model. We focused on examining drug effects on brain representations of the reward prediction error signal (i.e., significant parameter estimate for explanatory variable 7 with respect to the residuals) or of the incentive value of the selected action in reward trials (significant parameter estimate for explanatory variable 1 with respect to the residuals). We also focused our analyses on two brain regions of interest: the ventral striatum and the ventromedial prefrontal cortex (sometimes referred to as the ventromedial prefrontal cortex/orbitofrontal cortex; see the online Figure 1; see also the data supplement for details on the regions of interest).
For group analyses, we used the “randomise” tool from FSL (www.fmrib.ox.ac.uk/fsl/randomise), a permutation-based method (24). We performed 5,000 permutations and smoothed each voxel variance (3 mm), as recommended for experiments with few degrees of freedom (24). All results were thresholded at p<0.05, family-wise error corrected, after threshold-free cluster enhancement (25), except as otherwise specified. Although our focus was on our hypothesized regions of interest, we performed additional (secondary) whole-brain analyses (corrected for multiple comparisons).
Results
Behavioral Results
Psychotic symptoms.
Methamphetamine induced mild psychotic symptoms (as rated by the Comprehensive Assessment of At-Risk Mental States scale), even when it was administered together with amisulpride (Figure 2) (placebo condition: score, 0.18 [SD=0.39]; methamphetamine condition: score, 1.94 [SD=2.44]; methamphetamine plus amisulpride condition: score, 3.12 [SD=3.28]; placebo compared with methamphetamine, t=−2.87, df=16, p=0.011; methamphetamine compared with methamphetamine plus amisulpride, t=−1.57, df=16, p=0.138).
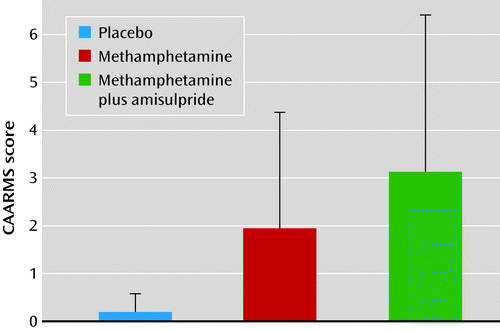
a Methamphetamine significantly induced psychotic symptoms in volunteers (p=0.011), even with amisulpride pretreatment (p=0.003). Error bars indicate standard deviation. CAARMS=Comprehensive Assessment of At-Risk Mental States.
Task performance.
There was no effect of drug on the accuracy of performance. The number of correct choices (“high-likelihood” in reward, “low-likelihood” in punishment) when decisions on reward and punishment trials were summed was similar in all visits (placebo condition: total=43.8 [SD=8.9]; methamphetamine condition: total=45.3 [SD=6.1]; methamphetamine plus amisulpride condition: total=41.4 [SD=9.1]). Participants made a higher number of correct decisions in win trials than in loss trials, as demonstrated by the effect of valence in the 3×2 (drug-by-valence) repeated-measures analysis of variance (ANOVA) (F=7.9, df=1, 16, p=0.013). No drug or drug-by-valence interaction effects were observed.
Learning parameters to calculate reward prediction error and incentive values.
After testing 100 different values of the learning rate constant and of the exploration/exploitation constant, the set of learning parameters that best explained participants’ performance was a learning rate constant of 0.43 and an exploration/exploitation constant of 0.3. These constants were used for all participants and pharmacological conditions to calculate reward prediction error and incentive values for the fMRI analysis for reward, punishment, and neutral trials. The model fitted to the behavior was equally good for all three pharmacological conditions (drug effect, F=2.36, df=2, 32, p=0.11; drug-by-valence interaction, F=1.31, df=4, 64, p=0.28) and was significantly better than chance for all conditions (p<0.005 for each condition).
Individual learning parameters.
For the behavioral analysis, we calculated the individual pair of values that best explained the performance of each participant during the task for the reward and punishment conditions.
Learning rate.
A 3×2 (drug-by-valence) repeated-measures ANOVA with the learning rate as the outcome variable revealed no effect of any of the factors, nor of any interaction between them. When considering reward and punishment trials as a single learning condition, we observed no effect of drug. In an analysis examining only reward trials, there was evidence of learning impairment induced by methamphetamine (F=6.90, df=2, 32, p=0.003). Post hoc tests demonstrated that the learning rate was reduced under methamphetamine and restored by amisulpride (see Figure S1 in the online data supplement).
Exploration/exploitation parameter.
There was no effect of drug, valence, or their interaction on the exploration/exploitation parameter.
fMRI Results
Placebo.
The reward prediction error signal was represented bilaterally in clusters encompassing the nucleus accumbens and ventral aspects of the caudate nucleus and putamen (p<0.05, family-wise error corrected, within limbic striatal region of interest) (Figure 3, Table 1). Voxels within the left and right ventromedial prefrontal cortex represented the incentive value of the chosen action (p<0.05, family-wise error corrected, within the ventromedial prefrontal cortex region of interest) (Figure 3, Table 1). No voxels within the ventral striatum represented incentive value, and no voxels within the ventromedial prefrontal cortex represented reward prediction error at our chosen statistical threshold. Secondary analyses at the whole-brain level are reported in the online data supplement (see Figure S3 and Table S1).
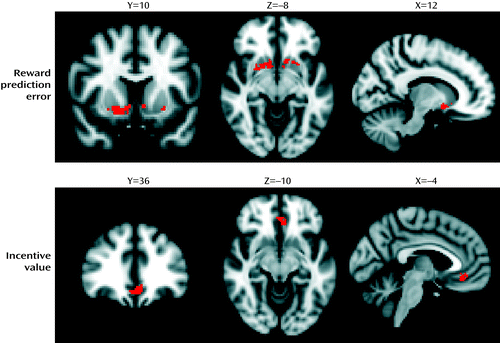
a Thresholded at p<0.05, family-wise error corrected. Left hemisphere is shown in the right side of the image. Coordinates are expressed in standard space, in millimeters.
Contrast and Analysisa | Region | Voxels | Coordinates (x, y, z) (mm) | Peak p |
---|---|---|---|---|
Placebo | ||||
Reward prediction error analysis | Right nucleus accumbens | 121 | 14, 8, –12 | 0.008 |
Left ventral caudate nucleus | 46 | –10, 16, –6 | <0.001 | |
Left ventral putamen | 24 | –26, 6, –4 | 0.015 | |
Incentive value analysis | Left ventromedial prefrontal cortex | 227 | –4, 34, –14 | 0.001 |
Placebo > methamphetamine | ||||
Reward prediction error analysis | Left ventral caudate nucleus | 37 | –10, 16, –6 | 0.007 |
Incentive value analysis | Left ventromedial prefrontal cortex | 9 | –4, 34, –12 | 0.034 |
Placebo compared with methamphetamine.
A cluster located in the left ventral striatum showed a significantly disrupted reward prediction error signal after methamphetamine challenge (placebo > methamphetamine, p<0.05, family-wise error corrected, within the ventral striatal region of interest) (Figure 4, Table 1). In addition, the incentive value signal was attenuated by methamphetamine in the ventromedial prefrontal cortex (placebo > methamphetamine, p<0.05, family-wise error corrected, within the ventromedial prefrontal cortex region of interest) (Figure 4, Table 1). The reverse contrasts (methamphetamine > placebo) showed no significant voxels within our region-of-interest analyses.
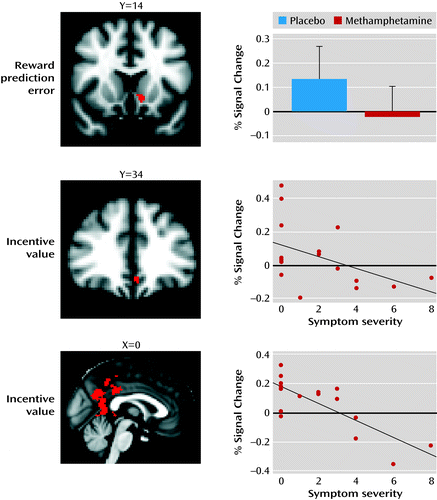
a The top image shows a cluster in the left ventral caudate nucleus indicating a significant difference for reward prediction error signal (p<0.05, family-wise error corrected); the bar graph indicates the drug effect on the signal change extracted for that particular cluster. Error bars indicate standard deviation. The middle image shows a methamphetamine-disrupted incentive value signal in the ventromedial prefrontal cortex (p<0.05, family-wise error corrected); as shown in the graph, the signal change extracted from this cluster in the methamphetamine visit correlated with symptom severity (Spearman’s rank-order correlation [rs]=–0.54, p=0.025). In the bottom image and graph, a large significant cluster was found in the posterior cingulate when the incentive value signal in the methamphetamine condition was correlated with symptom severity at a whole brain level (p<0.05, family-wise error corrected) (rs=–0.73, p=0.001).
Amisulpride plus methamphetamine compared with methamphetamine alone.
Amisulpride did not protect against the effects of methamphetamine. No significant clusters were observed when comparing reward prediction error and incentive value signal in the methamphetamine condition relative to the methamphetamine plus amisulpride condition.
Correlation with psychotic symptoms.
In order to test whether reward prediction error and incentive value signaling were associated with psychotic symptoms induced by methamphetamine, we extracted the mean parameter estimate of both learning signals within the two clusters found in the placebo versus methamphetamine analysis (in the ventral striatum and ventromedial prefrontal cortex) and correlated these with psychotic symptoms as measured in the methamphetamine condition. No correlation was found between the striatal reward prediction error signal in methamphetamine and psychotic symptoms (Spearman’s rank-order correlation [rs]=0.22, p=0.39). However, the incentive value extracted from the ventromedial prefrontal cortex cluster showed a negative correlation with symptom severity (rs=−0.54, p=0.025), demonstrating that participants with a poorer incentive value signal in the ventromedial prefrontal cortex experienced more severe psychotic symptoms (Figure 4). Amisulpride significantly reduced the strength of the correlation between the ventromedial prefrontal cortex incentive value signal and psychotic symptoms (Steiger’s z-test=2.1, p=0.04).
Our region-of-interest analyses were supplemented with a regression analysis at the whole-brain level (correcting for multiple comparisons across all brain voxels) to examine whether any additional regions showed associations between drug-induced psychotic symptoms and learning signals. This analysis revealed a cluster centered in the posterior cingulate cortex in which the incentive value parameter estimates were negatively associated with the severity of psychotic symptoms (p<0.05, family-wise error corrected) (Figure 4). Amisulpride did not significantly alter the strength of this association (Steiger’s z-test=1.41). Reward prediction error correlations with symptom severity were statistically nonsignificant at the selected threshold. There were no correlations with manic symptoms within either region of interest or at the whole-brain level, suggesting a degree of specificity for the relationship between ventromedial prefrontal cortex and posterior cingulate incentive value representation and methamphetamine-induced psychotic experience.
Discussion
Our study yielded the following findings: 1) intravenous methamphetamine induced mild psychotic symptoms in healthy volunteers; 2) methamphetamine significantly attenuated the reward prediction error signal in the limbic striatum and significantly attenuated the incentive value signal in the ventromedial prefrontal cortex; 3) methamphetamine induced behavioral changes in learning, leading to lower learning rates during reward-related reinforcement learning; 4) the degree to which methamphetamine disrupted the encoding of incentive values in the ventromedial prefrontal cortex correlated with the degree to which the drug induced mild psychotic symptoms; 5) the degree to which methamphetamine disrupted the encoding of incentive values in the posterior cingulate correlated with the degree to which the drug induced mild psychotic symptoms; and 6) pretreatment with amisulpride did not alter symptoms or the ventromedial prefrontal cortex incentive value signal, but it did alter the relationship between the ventromedial prefrontal cortex incentive value signal and mild psychotic symptoms.
According to an influential account of psychotic symptom formation, a disturbance in the ways that affected individuals evaluate stimuli and learn associations leads to mistaken evaluation of irrelevant phenomena as motivationally salient and to faulty association of unconnected ideas and events, ultimately leading to the emergence of characteristic alterations in perceptions and beliefs (8, 9, 26). In this study, we show that a drug intervention that induces psychotic symptoms is also associated with disruption of frontal and striatal neural learning signals. Moreover, the degree to which methamphetamine disrupted representations of incentive value in the ventromedial prefrontal cortex was correlated with the degree to which it induced psychotic symptoms, shedding light on the mechanisms of how amphetamines cause psychosis and increasing support for the argument that brain mechanisms of learning about incentive value and motivational importance are involved in the pathogenesis of psychotic symptoms in schizophrenia.
Considerable evidence has implicated frontal lobe function as being critical in the pathophysiology of schizophrenia (27). Previous research has extensively demonstrated the importance of the ventromedial prefrontal cortex in the representation of action value in rewarding events (23, 28, 29). Here, we suggest an implication of neurochemical disruption of prefrontal value computation: the generation of psychotic symptoms. This is in keeping with previous evidence demonstrating an association between medial frontal lobe function during learning and psychotic symptoms in schizophrenia, although as far as we are aware, a specific disruption of cortical incentive value signaling has yet to be described in schizophrenia (30). An additional novel finding of our study is to implicate a link between a disruption of incentive value signaling in the posterior cingulate with the psychotogenic effects of methamphetamine. Although we did not have a specific hypothesis about the effects of methamphetamine in this region, we note that this region has previously been shown to encode information about reward value (31) and that fMRI studies of memory and learning have documented posterior cingulate dysfunction in actively psychotic patients and ketamine-induced psychotic states (11, 32).
Behavioral analyses confirmed that methamphetamine mildly disrupted learning. While the number of correct decisions was not affected by methamphetamine, we found a deleterious effect of methamphetamine on learning rates in reward trials. We speculate that methamphetamine-induced disruption of monoamine signaling led to increasing levels of uncertainty and a consequent deleterious effect on learning. Impairments in the precision of the brain computations underlying learning introduce uncertainty into environmental appraisal, which, according to Bayesian accounts of belief updating, may lead to small prediction errors being given undue weight and consequent false inference (26, 33). Abnormal decision making under uncertainty is an important factor predisposing individuals to delusion formation in psychotic illness (34). Recent accounts of dopamine function in learning emphasize that dopamine neuron firing encodes not only information about expected value and prediction error but also information about their precision (35), such as the variance.
Our study shows that methamphetamine, a drug known to increase synaptic dopamine levels, affects both behavioral and brain representations of learning parameters along with a link to mental state changes that are typical of the early stages of psychosis. Thus, our results are consistent with the theory that a hyperdopaminergic state leads to psychotic phenomenology because of disruption in dopamine’s role in evaluation and learning of associations of stimuli. However, as methamphetamine affects not only dopamine but also norepinephrine and, to a lesser extent, serotonin, our study allows us to draw direct inferences about how amphetamines may impair decision making and induce psychotic symptoms via a “hypermonoaminergic” state, but not about a hyperdopaminergic state specifically. Pretreatment with 400 mg amisulpride before administration of methamphetamine had no effect on symptoms or brain response, and thus we cannot conclusively demonstrate that methamphetamine’s effects on brain reward learning signals were mediated by stimulation of dopamine D2 receptors. Indeed, the lack of effect of amisulpride may suggest an alternative explanation, that methamphetamine’s effects on symptoms and brain learning signals may have been mediated by nondopaminergic mechanisms, such as its effects on norepinephrine release, or possibly through dopaminergic effects on dopamine D1 receptors, which would not have been blocked by amisulpride. Amisulpride pretreatment did modulate the relationship between methamphetamine-induced alterations in prefrontal function and psychotic symptoms. This suggests that although amisulpride does not normalize methamphetamine’s deleterious effects on ventromedial prefrontal incentive value signaling, it may reduce the tendency of these ventromedial prefrontal disruptions to manifest in psychotic symptoms. The subtle effects of amisulpride in this study make it hard to draw conclusive interpretations concerning the relative contributions of norepinephrine and dopamine to the results. If we had used a higher dose of amisulpride, it might have resulted in clearer results, though at the risk of possibly inducing Parkinsonian side effects in the volunteers.
To our knowledge, only one previous study has examined the effect of a prodopaminergic drug on brain representations of reward learning parameters in healthy humans; that study found that levodopa improved striatal representations of reward prediction error during learning (21). We now show that an amphetamine-induced hypermonoaminergic state can be associated with impairments in both frontal and striatal representations of incentive value and reward prediction error. The divergence between our results and the previous study can be resolved when considering that levodopa, being a dopamine precursor, facilitates stimulus-locked dopamine release. Methamphetamine, however, can cause stimulus-independent release of monoamines through its actions on the dopamine, norepinephrine, and serotonin transporters and the vesicular monoamine transporter-2, changing the balance of phasic and tonic release of monoamines, thus reducing the signal-to-noise ratio during learning and potentially reducing frontostriatal transmission via stimulation of presynaptic dopamine D2 receptors (36–38). This finding is highly relevant in understanding both how stimulant intoxication may lead to maladaptive learning and induce psychiatric symptoms (16) and how a hyperdopaminergic state in psychotic illness could be accompanied by impaired associative learning (39, 40).
In summary, our study provides new evidence of the role of monoaminergic frontostriatal function in underpinning feedback-based learning in humans. We show, for the first time, that a drug-induced state can disrupt neural representations of computational parameters necessary for reinforcement learning, while causing a reduced learning rate; these findings have implications for understanding decision-making impairments seen in amphetamine intoxication. The finding that the degree to which methamphetamine induces psychotic symptoms is related to the degree to which the drug affects the encoding of incentive value in the frontal and cingulate cortices is consistent with theories linking abnormal mechanisms of learning and incentive valuation in the pathogenesis of psychosis.
1 : Reward representations and reward-related learning in the human brain: insights from neuroimaging. Curr Opin Neurobiol 2004; 14:769–776Crossref, Medline, Google Scholar
2 : The root of all value: a neural common currency for choice. Curr Opin Neurobiol 2012; 22:1027–1038Crossref, Medline, Google Scholar
3 : Dopamine signals for reward value and risk: basic and recent data. Behav Brain Funct 2010; 6:24Crossref, Medline, Google Scholar
4 : Neuropharmacology of performance monitoring. Neurosci Biobehav Rev 2009; 33:48–60Crossref, Medline, Google Scholar
5 : New insights on the subcortical representation of reward. Curr Opin Neurobiol 2008; 18:203–208Crossref, Medline, Google Scholar
6 : Acute tryptophan depletion in healthy volunteers enhances punishment prediction but does not affect reward prediction. Neuropsychopharmacology 2008; 33:2291–2299Crossref, Medline, Google Scholar
7 : Functions of frontostriatal systems in cognition: comparative neuropsychopharmacological studies in rats, monkeys, and humans. Biol Psychol 2006; 73:19–38Crossref, Medline, Google Scholar
8 : Schizophrenic psychology, associative learning, and the role of forebrain dopamine. Med Hypotheses 1976; 2:203–211Crossref, Medline, Google Scholar
9 : Psychosis as a state of aberrant salience: a framework linking biology, phenomenology, and pharmacology in schizophrenia. Am J Psychiatry 2003; 160:13–23Link, Google Scholar
10 : From prediction error to psychosis: ketamine as a pharmacological model of delusions. J Psychopharmacol 2007; 21:238–252Crossref, Medline, Google Scholar
11 : Substantia nigra/ventral tegmental reward prediction error disruption in psychosis. Mol Psychiatry 2008; 13:267–276Crossref, Google Scholar
12 : Expected value and prediction error abnormalities in depression and schizophrenia. Brain 2011; 134:1751–1764Crossref, Medline, Google Scholar
13 : Disambiguating ventral striatum fMRI-related bold signal during reward prediction in schizophrenia. Mol Psychiatry 2012; 17:280–289Crossref, Google Scholar
14 : Disrupted prediction-error signal in psychosis: evidence for an associative account of delusions. Brain 2007; 130:2387–2400Crossref, Medline, Google Scholar
15 : Frontal responses during learning predict vulnerability to the psychotogenic effects of ketamine: linking cognition, brain activity, and psychosis. Arch Gen Psychiatry 2006; 63:611–621Crossref, Medline, Google Scholar
16 : Amphetamine Psychosis. London, Maudsley Monograph, Chapman and Hill, 1958Google Scholar
17 : The experimental reproduction of amphetamine psychosis. Arch Gen Psychiatry 1973; 29:35–40Crossref, Medline, Google Scholar
18 : Amphetamine psychosis: behavioral and biochemical aspects. J Psychiatr Res 1974; 11:13–23Crossref, Medline, Google Scholar
19 : Mapping the onset of psychosis: the Comprehensive Assessment of At-Risk Mental States. Aust N Z J Psychiatry 2005; 39:964–971Crossref, Medline, Google Scholar
20 : Reinforcement Learning. Cambridge, Mass, MIT Press, 1998Google Scholar
21 : Dopamine-dependent prediction errors underpin reward-seeking behaviour in humans. Nature 2006; 442:1042–1045Crossref, Medline, Google Scholar
22 : BOLD responses reflecting dopaminergic signals in the human ventral tegmental area. Science 2008; 319:1264–1267Crossref, Medline, Google Scholar
23 : Choosing the lesser of two evils, the better of two goods: specifying the roles of ventromedial prefrontal cortex and dorsal anterior cingulate in object choice. J Neurosci 2006; 26:11379–11386Crossref, Medline, Google Scholar
24 : Nonparametric permutation tests for functional neuroimaging: a primer with examples. Hum Brain Mapp 2002; 15:1–25Crossref, Medline, Google Scholar
25 : Threshold-free cluster enhancement: addressing problems of smoothing, threshold dependence, and localisation in cluster inference. Neuroimage 2009; 44:83–98Crossref, Medline, Google Scholar
26 : Perceiving is believing: a Bayesian approach to explaining the positive symptoms of schizophrenia. Nat Rev Neurosci 2009; 10:48–58Crossref, Medline, Google Scholar
27 : Dysfunctional and compensatory prefrontal cortical systems, genes, and the pathogenesis of schizophrenia. Cereb Cortex 2007; 17(suppl 1):i171–i181Crossref, Medline, Google Scholar
28 : Dissociating the role of the orbitofrontal cortex and the striatum in the computation of goal values and prediction errors. J Neurosci 2008; 28:5623–5630Crossref, Medline, Google Scholar
29 : Determining a role for ventromedial prefrontal cortex in encoding action-based value signals during reward-related decision making. Cereb Cortex 2009; 19:483–495Crossref, Medline, Google Scholar
30 : Reward feedback alterations in unmedicated schizophrenia patients: relevance for delusions. Biol Psychiatry 2009; 65:1032–1039Crossref, Medline, Google Scholar
31 : Neural mechanisms of foraging. Science 2012; 336:95–98Crossref, Medline, Google Scholar
32 : NMDA hypofunction in the posterior cingulate as a model for schizophrenia: an exploratory ketamine administration study in fMRI. Schizophr Res 2005; 72:235–248Crossref, Medline, Google Scholar
33 : A Bayesian foundation for individual learning under uncertainty. Front Hum Neurosci 2011; 5:39Crossref, Medline, Google Scholar
34 : Plausibility judgment in schizophrenic patients: evidence for a liberal acceptance bias. Ger J Psychiatry 2004; 7:66–74Google Scholar
35 : Adaptive coding of reward value by dopamine neurons. Science 2005; 307:1642–1645Crossref, Medline, Google Scholar
36 : Amphetamine modulates human incentive processing. Neuron 2004; 43:261–269Crossref, Medline, Google Scholar
37 : A review of the clinical pharmacology of methamphetamine. Addiction 2009; 104:1085–1099Crossref, Medline, Google Scholar
38 : Dysfunctions in multiple interrelated systems as the neurobiological bases of schizophrenic symptom clusters. Schizophr Bull 1998; 24:267–283Crossref, Medline, Google Scholar
39 : Relationship between reward-enhancing and stereotypical effects of psychomotor stimulant drugs. Nature 1976; 264:57–59Crossref, Medline, Google Scholar
40 : Integrating schizophrenia. Schizophr Bull 1998; 24:249–266Crossref, Medline, Google Scholar