Pharmacogenetics of Psychotropic Drug Response
Abstract
OBJECTIVE: Molecular genetic approaches provide a novel method of dissecting the heterogeneity of psychotropic drug response. These pharmacogenetic strategies offer the prospect of identifying biological predictors of psychotropic drug response and could provide the means of determining the molecular substrates of drug efficacy and drug-induced adverse events. METHOD: The authors discuss methods issues in executing pharmacogenetic studies, review the first generation of pharmacogenetic studies of psychotropic drug response, and consider future directions for this rapidly evolving field. RESULTS: Pharmacogenetics has been most commonly used in studies of antipsychotic drug efficacy, antidepressant drug response, and drug-induced adverse effects. Data from antipsychotic drug studies indicate that polymorphisms within the serotonin 2A and dopamine receptor 2 genes may influence drug efficacy in schizophrenia. Moreover, a growing body of data suggests a relationship between the serotonin transporter gene and clinical effects of the selective serotonin reuptake inhibitors used to treat depression. A significant relationship between genetic variation in the cytochrome P450 system and drug-induced adverse effects may exist for certain medications. Finally, a number of independent studies point to a significant effect of a dopamine D3 receptor polymorphism on susceptibility to tardive dyskinesia. CONCLUSIONS: Initial research into the pharmacogenetics of psychotropic drug response suggests that specific genes may influence phenotypes associated with psychotropic drug administration. These results remain preliminary and will require further replication and validation. New developments in molecular biology, human genomic information, statistical methods, and bioinformatics are ongoing and could pave the way for the next generation of pharmacogenetic studies in psychiatry.
The variation in individual clinical response to psychotropic drug treatment remains a critical problem in the management of the seriously mentally ill patient. Although a minority of patients may experience complete symptom remission, a large proportion of patients continues to experience significant psychiatric symptoms (1), and in addition there is a subset of patients who develop drug-induced adverse events that may range from the troublesome to the life threatening (2). Moreover, psychotropic drug efficacy may not occur until weeks after initiation of drug treatment (3), and thus, the time period before a clinician can determine whether a specific treatment is ineffective and consider alternative pharmacotherapy can be lengthy. During this period, treated patients may experience continuous psychiatric symptoms, employment loss, social dysfunction, medical morbidity, and—in a significant proportion of patients with psychosis and affective disorders—even commit suicide (4).
Early efforts to identify the predictors of psychotropic drug response focused on clinical variables with limited success. More recent efforts with biological variables such as plasma and CSF levels of neurotransmitter metabolites, neurohormone levels, and brain imaging measures have provided some initially promising results (5–9), but consistent data have remained elusive. A major drawback of these approaches is the considerable variation in the biological variable being used as the independent measure, which reduces the power of these measures to predict or correlate with measures of treatment response.
Molecular genetic approaches provide a novel method of dissecting the heterogeneity of psychotropic drug response. This field of inquiry, traditionally termed “pharmacogenetics,” provides a number of distinct advantages in the search for informative correlates of psychotropic drug response (Appendix 1). First, an individual subject’s genotype is essentially invariable, and thus, collection of the independent measure for analysis versus treatment response can be performed at any time during treatment (or thereafter) and remain unaffected by the treatment itself (10). Second, current molecular biological techniques provide an accurate assessment of an individual’s genotype (11), and measurement error plays little or no role in these analyses. Third, the dramatic increase in the amount of publicly available genomic information (12) now provides the necessary data to conduct comprehensive studies of individual genes and, perhaps, investigations of entire genomes. Finally, the ease of accessibility to genotype information by means of peripheral blood samples, coupled with advances in molecular techniques, has increased the feasibility of routine DNA collection and genotyping in large-scale clinical trials samples (13). Therefore, pharmacogenetic approaches provide a new opportunity to identify biological predictors of psychotropic drug response, but perhaps more important, they may provide the means to determine the actual molecular substrates of psychotropic drug efficacy. In this article, we will discuss basic methods issues in executing pharmacogenetic studies, review the first generation of pharmacogenetic studies of psychotropic drug response, and consider future directions for this rapidly evolving field.
Methods Issues
Phenotype Definition
A critical issue for genetic studies is the reliability and validity of the phenotype under investigation. Disease susceptibility studies use structured diagnostic interviews and family informants, when available, but diagnostic heterogeneity remains a major potential confounder for these studies. In psychiatric pharmacogenetics, the most common phenotype has been short-term drug response, in which efficacy is assessed by changes in standardized rating scales such as the Brief Psychiatric Rating Scale (BPRS) or the Hamilton Depression Rating Scale over periods that range from 3 weeks up to a few months. Little data has been collected on remission rates or from longer-term relapse prevention studies.
Few pharmacogenetic studies include placebo arms in their design. In part, this is because of the ethical considerations in conducting placebo-controlled studies in disorders for which effective treatments are known to exist, but economic considerations may also play a role. This may be a particular problem in antidepressant studies that use clinical response as a phenotype since the placebo response rate in many of these studies often approaches 30%–40% compared to an active treatment response rate of 70% (14). These placebo response rates suggest that half of the patients characterized as “active” drug responders may be placebo responders, and thus, these subjects could represent false positive observations in a pharmacogenetic analysis. Placebo “run-ins” may diminish the frequency of this but are difficult to accomplish in the large-scale studies that are needed to provide adequate power for pharmacogenetics. New study designs may need to be developed to better address this phenotypic issue.
Similarly, the phenotype of drug-induced side effects may be complex. For example, patients may be taking multiple medications or have comorbid illnesses that predispose them to the development of an adverse event. Pharmacogenetic studies of antipsychotic drug-induced weight gain are often conducted in patient populations with chronic schizophrenia in which previous antipsychotic drug treatment may have resulted in weight gain (15). This may complicate the analysis of subsequent weight gain when they take a new antipsychotic. Likewise, in some cases, the underlying illness may contribute to the development of an adverse event, and thus, the drug may not be specifically responsible for the observed event. These issues require careful consideration and should be critically evaluated during consideration of the design of pharmacogenetic studies, particularly those conducted in chronically ill patient groups.
Heritability of Psychotropic Drug Response
Gene discovery studies may be informed by the assessment of the contribution of genetic factors, or heritability estimates, to the trait of interest. Although family, twin, and epidemiological studies suggest that the major psychiatric disorders have significant genetic components (16), there is a paucity of heritability data regarding psychotropic drug response. Heritability studies traditionally involve ascertainment of twin pairs that are discordant for zygosity or adoption studies of siblings separated at birth (17), but these approaches are better suited to the study of traditional illness classifications rather than drug response. Moreover, pedigree studies of several generations cannot provide informative data because of the continual development of new psychotropic drugs and the subsequent alteration in prescription patterns.
The majority of heritability data on psychotropic drugs is from studies of antidepressant drug response. Angst (18) examined 41 first-degree relative pairs that were both treated with the tricyclic antidepressant imipramine and reported that 38 pairs were concordant for response. Pare et al. (19) studied first-degree relatives of 170 depressed patients who had participated in antidepressant drug clinical trials and assessed concordance rates in the relative pairs that underwent similar treatment. Of the 12 cases of concordant treatments, both members of each relative pair had equivalent responses, with an overall rate of response of 42%. A follow-up study of a new cohort (20) found that 10 of 12 patients with concordant antidepressant treatment were concordant for clinical response. These data are consistent with a retrospective analysis of two generations of a family with multiple ill relatives with major depression in which all four family members who underwent treatment with the monoamine oxidase inhibitor tranylcypromine responded, despite nonresponse to conventional treatment (21). Finally, Franchini et al. (22) studied 45 first-degree relative pairs who were treated with the serotonin reuptake inhibitor fluvoxamine for at least 6 weeks. A total of 67% of the relative pairs were concordant for response, compared to the 50% that would be expected by chance. These studies suggest that genetic factors may play a role in antidepressant drug response; however, it is possible that shared environmental factors may bias relative pairs toward similar response patterns. Studies designed to specifically assess the heritability of antidepressant drug response may still be required.
There is less data on the heritability of antipsychotic drug response. One line of evidence that suggests that genetic factors play a role is derived from studies of treatment response across different ethnic groups. A recent study incorporating data from randomized clinical trials found that black patients displayed greater acute response (in 6 weeks) to treatment with atypical and typical antipsychotic agents than white patients of European descent (23). In this study, however, there were significant differences in baseline symptoms between groups and no control for potential differences in nutritional status and body mass. Moreover, underlying cultural bias in the treatment and assessment of disparate ethnic groups may play a role in apparent treatment response variation (24). Other data on the heritability of antipsychotic drug response is limited to a study of 28 schizophrenia sibling pairs that found no more concordance for the response to typical antipsychotic agents than that predicted by chance (25) and case reports (26, 27) of monozygotic twin pairs with schizophrenia who were concordant for atypical antipsychotic response despite prior nonresponse to typical antipsychotic agents.
Pharmacogenetics of Antipsychotic Drugs
In psychiatry, pharmacogenetic studies have focused on three major phenotypes: clinical efficacy of antipsychotic drugs, efficacy of antidepressant medications, and development of adverse effects associated with psychotropic drug treatment.
Pharmacogenetic studies of antipsychotic drug response have focused on the atypical antipsychotic drug clozapine, perhaps because of the ease of access to blood samples from clozapine-treated patients from which to extract DNA or because clozapine’s superior efficacy in treatment-resistant populations (28) suggests that an understanding of the genetic contributions to its effects could provide novel data on the molecular basis of antipsychotic efficacy. The initial clozapine studies were conducted either in the context of clinical trials of clozapine (29, 30) or were based upon retrospective analyses of clozapine-treated patients (31–34) in ethnically heterogeneous study groups derived from Western countries, including the United Kingdom, Germany, and the United States.
Pharmacogenetic studies of clozapine have primarily used a candidate gene approach (Figure 1), with genetic loci in the dopamine and serotonin receptor systems—obvious candidates because of the high affinity of clozapine for these receptor subtypes. Arranz and colleagues (31) initially attracted interest in the serotonin 5-HT2A T102C polymorphism with a report of a significant (p=0.02) association between the 102C allele and failure to respond to clozapine in a cohort of 149 patients with chronic schizophrenia who were retrospectively assessed with the Global Assessment Scale. These data were not replicated in a series of smaller clozapine studies from independent laboratories (29, 35–37), as well as in a study that included typical antipsychotic agents (38) (Table 1). 5-HT2A T102C could be considered a relatively weak candidate polymorphism because it does not result in an amino acid substitution at the protein level and there is little evidence that it produces significant functional effects on 5-HT2A receptor function (40). A less common polymorphism within the 5-HT2A gene, His452Tyr, which was not found to be in significant linkage disequilibrium (nonrandom population association) with T102C (29), does appear to produce functional effects in vitro; however, it has not consistently been found to be associated with clozapine response (29, 35).
Nevertheless, more recent data suggest that the positive association between 5-HT2A T102C and clozapine response may not have been a false positive result. First, this variant is in strong linkage disequilibrium with a putatively functional polymorphism, –1438 G/A, in the promoter region of the gene (41). Second, a meta-analysis of clozapine pharmacogenetic studies of 5-HT2A T102C revealed an excess of the 102C allele in clozapine nonresponders in each data set, with a significant effect of this variant on clozapine response in the combined sample (42). Since the majority of studies included in this meta-analysis were published as “negative” studies, publication bias is unlikely to have confounded these results, and the smaller studies may have provided insufficient power to detect the modest effects of this gene on a complex clinical phenotype such as clozapine response. Finally, a recent study of 5-HT2A T102C and antipsychotic response to the atypical agent risperidone in 100 Han Chinese schizophrenia patients (39) also identified an association, but in this instance, the association was in the opposite direction than previously observed in primarily Caucasian study groups. Definitive studies with larger group sizes, prospective clinical data, and comprehensive examination of the gene will be needed to further address the role of this gene in antipsychotic drug response.
Other serotonin-related genes that have been examined in pharmacogenetic studies of clozapine include the 5-HT2C(30, 32, 37, 43), the 5-HT6 (44, 45), the 5-HT7(45), as well as the serotonin transporter (SLC6A4) gene (46, 47). Although there have been some positive reports of association, there is little current evidence to suggest that variation within these genes significantly influences clozapine’s efficacy.
In addition to significant affinities for serotonin receptor subtypes, clozapine is also a dopamine receptor antagonist. Initial pharmacogenetic studies focused on the relationship between the dopamine D4 receptor gene (DRD4) and clozapine response (48, 49). DRD4 was an attractive candidate gene because of clozapine’s affinity for the D4 receptor (50) and the identification of a common variable number of tandem repeat polymorphisms within the putative third cytoplasmic loop of the receptor, associated with significant effects on the binding affinity of the receptor for clozapine (51). Most groups, however, have been unable to detect a significant association between this variant and clozapine response (48, 49, 52, 53). Ozdemir and colleagues (54) have reported an association between a repeat polymorphism within the first intron of DRD4 in a preliminary study of 50 patients; however, correction for multiple testing could alter the significance of these results. Clinical trials demonstrating that dopamine D4 receptor antagonists are ineffective in the treatment of schizophrenia (55) have also diminished enthusiasm for this receptor in antipsychotic drug efficacy.
Another obvious candidate for pharmacogenetic studies of antipsychotic drug response is the D2 receptor gene (DRD2). To date, all known antipsychotic drugs have potent affinities for the D2 receptor (56, 57), and functional brain imaging studies have suggested that D2 receptor binding by antipsychotic agents may be “necessary and sufficient” for antipsychotic efficacy (58). However, there are few common polymorphisms within the coding regions of DRD2(59), and thus, fewer studies of DRD2 and antipsychotic drug response have been conducted, compared with the 5-HT system.
Two preliminary studies have included evidence that genetic variation within DRD2 is associated with antipsychotic response (60, 61). Moreover, Shäfer and colleagues (62) examined the relationship between haloperidol, an antipsychotic drug with greater D2 affinity than clozapine, and the DRD2 Taq1A polymorphism and found that subjects with the A2/A2 genotype displayed poorer clinical response than heterozygous patients (no A1/A1 homozygotes were obtained). The distinction between genotypic groups was evident after 2 weeks of treatment, with 63% of the heterozygous subjects meeting response criteria versus 29% of the subjects with the A2/A2 genotype. Consistent with these results, recent preliminary data have suggested a relationship between the Taq1 A2/A2 genotype and failure to respond to risperidone (63), another agent with greater D2 affinity than clozapine. Finally, Suzuki and colleagues (64) report preliminary evidence for a relationship between DRD2 and response to the typical antipsychotic agents bromperidol and nemonapride. Therefore, many, although not all (65), studies suggest that variation within DRD2 may significantly influence response to antipsychotic drugs, particularly with agents with higher affinities for the D2 receptor. It should be noted, however, that these studies used two different polymorphisms—the Taq1A variant and the promoter region variant –141C Ins/Del—that are located more than 250 kilobases (kb) apart from each other. Therefore, more comprehensive examination of DRD2 and antipsychotic drug response may be required.
Finally, it is increasingly recognized that any individual gene’s effect on antipsychotic drug response will be modest in most populations. Therefore, examination of multiple genes and multiple single nucleotide polymorphisms (SNPs) in order to identify sensitive and specific “predictor profiles” is currently under way. A retrospective analysis of 19 candidate polymorphisms identified a grouping of six variants that provided 76.7% success in predicting clozapine response (66). Since these data have not yet been replicated (67), it will be critical to conduct prospective studies of this profile before these data can be considered to provide potential clinical use. Nevertheless, studies that begin to dissect the complex interactions between genetic loci and their effect on clinical phenotypes are likely to be necessary for a greater understanding of the genetic contribution to antipsychotic drug response.
Pharmacogenetics of Antidepressant Drugs
Pharmacogenetic studies of antidepressants can be classified as addressing either pharmacokinetic or pharmacodynamic effects. Genetic variants affecting the metabolism of antidepressants may change pharmacokinetic factors, such as plasma drug concentration and half-life. Polymorphisms that affect the expression or function of receptors and signal transduction molecules in the brain may alter pharmacodynamics. Both pharmacokinetic and pharmacodynamic changes can affect the efficacy and side effects of antidepressants.
Pharmacokinetic pharmacogenetic studies have focused on polymorphisms in liver cytochrome P450 isoenzymes that metabolize many antidepressant medications. The most intensively investigated gene is CYP2D6, which encodes debrisoquine hydroxylase. Many antidepressants, including tricyclics, selective serotonin reuptake inhibitors (SSRIs), venlafaxine, and others, are metabolized primarily by debrisoquine hydroxylase (68). The CYP2D6 gene is highly polymorphic, with over 70 known variants (for a current classification, view the CYP P450 allele nomenclature at http://www.imm.ki.se/CYPalleles/). Homozygosity for null alleles gives rise to the poor metabolizer phenotype for debrisoquine hydroxylase characterized by no enzyme activity. Null allele heterozygosity or homozygosity for intermediate metabolic alleles gives rise to an intermediate debrisoquine hydroxylase metabolic phenotype characterized by impaired—but not absent—enzyme activity (69–71). CYP2D6 gene duplications give rise to the ultrametabolic activity of debrisoquine hydroxylase (72).
In highly controlled studies of drug metabolism performed with healthy volunteers in pharmacokinetics laboratories, the CYP2D6 genotype has been shown to predict tricyclic and SSRI plasma concentrations (73–76). However, there are few studies performed in clinical settings. In a group of inpatient and outpatient geriatric patients suffering from major depression, the CYP2D6 genotype predicted plasma nortriptyline levels at a steady state (77). The genotype-drug concentration correlation was evident, despite the fact that, on average, the patients took 9.8 concurrent medications in addition to nortriptyline. Hence, CYP2D6 genotyping could be useful in identifying patients likely to experience high plasma levels when treated with nortriptyline. In a retrospective study of 100 patients treated with tricyclic antidepressants and other psychotropics in a state hospital, adverse events were associated with CYP2D6 genotypes encoding debrisoquine hydroxylase with poor metabolism (78). These results suggest that CYP2D6 genotypes could be useful in identifying patients likely to experience antidepressant side effects.
However, in a prospective, double-blind study of paroxetine treatment of 122 patients with geriatric major depression, Murphy et al. (79) found that the CYP2D6 genotype had no effect on medication-related adverse events (determined by clinician ratings), treatment discontinuations due to adverse events, and measures of treatment efficacy, such as change in Hamilton depression scale ratings over time. A number of studies have shown a relationship between the CYP2D6 genotype (and/or the debrisoquine hydroxylase phenotype) and paroxetine levels during short- or long-term dosing (74, 80–84), although another as yet unidentified cytochrome is also involved (84, 85). The safety margin of SSRI agents such as paroxetine may be sufficient that impaired clearance in patients with intermediate or poor debrisoquine hydroxylase activity does not result in more adverse events. This may also be the case with other modern antidepressants that are metabolized largely by debrisoquine hydroxylase. Recommendations for dose adjustments based on CYP2D6 genotype have been published (86). However, until more prospective pharmacogenetic data are available, these recommendations may be premature.
In addition to metabolizing many antidepressants, debrisoquine hydroxylase can also be inhibited by these medications (87, 88). Thus, an individual with an extensive or intermediate metabolic phenotype can be converted to a poor metabolic phenotype when treated with an SSRI such as paroxetine (89). This could result in toxicity if the patient were concurrently taking, for example, an antihypertensive or antiarrhythmic substrate with a narrow margin of safety. Case reports have been published of fatalities after an inhibitor of debrisoquine hydroxylase was coadministered with a debrisoquine hydroxylase substrate (90). However, despite extensive discussion in the literature on the consequences of debrisoquine hydroxylase inhibition (76, 91), clinical data are surprisingly sparse, aside from case reports. There may be considerable interindividual variation in the degree of debrisoquine hydroxylase inhibition by agents such as SSRIs (82, 83). Large-scale prospective studies are needed in which patients with known genotypes initiate antidepressant therapy and the effects on serum levels and side effects of concurrent medications are monitored longitudinally. In the study of Murphy et al. (79), 26 elderly depressed subjects who were treated with paroxetine were also taking a medication classified as a debrisoquine hydroxylase substrate. These individuals had no greater severity of adverse events than other paroxetine-treated subjects, and there was no interaction between CYP2D6 genotype, concurrent substrate medications, and adverse events. It is important to note that different results might have been obtained had the paroxetine-treated subjects concurrently taken tricyclic antidepressants, potentially toxic debrisoquine hydroxylase substrates that were not permitted in the study by Murphy et al. (79). However, in a 4-year postmarketing survey, few clinically significant interactions between paroxetine and other medications were detected (92). Further data are needed on this important topic, and clinicians should continue to use appropriate caution when multiple medications are prescribed.
The CYP3A4 liver enzyme is also involved in the metabolism of many psychotropics, including some antidepressants (93–95). A number of allelic variants at the gene encoding CYP3A4 have been identified (92). The effects of these variants on antidepressant metabolism in clinical settings have yet to be tested.
If and when clinically useful pharmacogenetic markers for antidepressant pharmacokinetics are identified, it remains to be seen how rapidly these will be integrated into clinical practice. For example, although a strong case can be made for therapeutic monitoring of drug concentrations, there is limited clinical use of this currently available technique in making treatment decisions (96).
A number of studies have also reported on pharmacogenetic markers that affect antidepressant pharmacodynamics. In one of the first studies involving an SSRI, Smeraldi and colleagues (97) showed that the “short” form of a deletion/insertion polymorphism in the promoter region of the serotonin transporter gene (SLC6A4) impaired the efficacy of fluvoxamine in a group of 53 patients with major depression (Table 2). In some cell biology studies, the long and the short versions of the SLC6A4 promoter polymorphism have been found to differentially affect the expression of the 5-HT transporter protein (103, 104). SSRIs are thought to act through inhibition of the 5-HT transporter, so a genetic variant that affects the expression of this protein could affect treatment response even though the protein structure is unchanged. The same effect of the SLC6A4 long/short promoter polymorphism was reported by Pollock and colleagues (98) in a study of 51 geriatric patients with major depression who were treated with paroxetine. Subsequently, the Zanardi group confirmed this finding in a group of 60 paroxetine-treated depressed patients (99). Minov and colleagues (101) found no effect of the SLC6A4 promoter polymorphism on response in 104 patients receiving a variety of antidepressant treatment regimens. This study is difficult to interpret because of heterogeneity in treatment regimen. However, Kim et al. (100) studied 120 Korean patients treated with fluoxetine or paroxetine and found that the short variant of the SLC6A4 promoter was associated with a better treatment response. A similar result was obtained by Yoshida et al. (102) in a study with Japanese patients. One explanation for these results may be that the effect of the SLC6A4 promoter is dependent on ethnic background. In the studies by Kim et al. (100) and Yoshida et al. (102), all patients were Asian, whereas in other studies, the patients have been predominantly non-Asian. In summary, the effect of the SLC6A4 promoter variant on SSRI treatment is the most robust antidepressant pharmacogenetic finding to date. However, contrary results from a study of Asian patients indicate that replication of this finding in other populations is necessary.
A handful of other pharmacogenetic studies aimed at antidepressant pharmacodynamics have been performed. Tryptophan hydroxylase is important in the synthesis of serotonin. Serretti and colleagues (105, 106) studied the A218C polymorphism (located in a noncoding region of the tryptophan hydroxylase gene) and found that the A allele was associated with poor treatment response in 217 depressed patients treated with fluvoxamine and in 121 patients treated with paroxetine. Because the A218C variant does not affect the expression or structure of the tryptophan hydroxylase protein, its pharmacogenetic effect may be related to another functional polymorphism in close proximity. The 5-HT2A receptor is located on postsynaptic neurons and may be important in antidepressant side effects (107). Cusin and coworkers (108) found that the C allele of the 5-HT2A T102C SNP associated with failure to respond to clozapine was also marginally associated with poor response to SSRIs.
Most pharmacogenetic studies aimed at antidepressant pharmacodynamics have hypothesized the effects on antidepressant efficacy due to functional variation in neurotransmitter receptors and transporter proteins located within the CNS. However, many antidepressant side effects, such as those associated with the vasculature or the gastrointestinal tract, could be mediated by interactions between antidepressants and peripheral neurons and synapses. Future pharmacogenetic studies should target receptors expressed by neurons of the autonomic nervous system and on end organs as predictors of antidepressant side effects and treatment discontinuation (109).
Although variations in genes encoding neurotransmitter receptors are a prime targets for pharmacogenetic studies, antidepressant effects on neurons involve a myriad of downstream effector molecules. Most aminergic receptors transduce signals through guanine nucleotide binding proteins (G proteins). Functional changes in these proteins could alter drug action. Zill and colleagues (110) examined the C825T functional polymorphism in the G-protein β3 subunit gene in 76 depressed patients receiving a variety of antidepressants. TT homozygotes showed greater treatment response than did other patients, although this finding is difficult to interpret because of treatment heterogeneity. Serretti et al. (111) found no association between D2 and D4 receptor polymorphisms and response to fluvoxamine or paroxetine. However, recent evidence shows that among SSRIs, only fluoxetine shows a significant effect on dopamine release (112).
Pharmacogenetics of Mood Stabilizers
There are few pharmacogenetic studies of mood stabilizers. Selecting candidate genes for pharmacogenetic investigation is difficult because the exact mechanism of action of established mood stabilizers such as lithium remains uncertain (113, 114). Lithium inhibits the activity of a number of enzymes, including those involved in the phosphatidylinositol cycle and phospholipase C signal transduction, although it has not been demonstrated that this action is responsible for mood stabilization. Steen and co-workers (115) tested for associations between polymorphisms in the inositol polyphosphate 1-phosphatase gene and lithium response in two small groups of bipolar patients. In a group of 23 patients, an association between the inositol polyphosphate 1-phosphatase C973A variant was found, but in the second group of 54 patients, there was no association. Other enzymes related to inositol phosphate metabolism may be suitable targets for studies of lithium’s action, as well as unrelated enzymes that are inhibited by lithium, such as glycogen synthase kinase-3 (114).
Serretti and Smeraldi and colleagues have extensively analyzed a group of approximately 125 bipolar and depressed patients treated with lithium prophylaxis. They found no association between lithium’s efficacy and polymorphisms at the following loci: the D2 receptor, the D3 receptor, the D4 receptor, the γ-aminobutyric acid (GABA) type A receptor α-1 subunit, and the 5-HT2A and 5-HT2C receptors (116–118). They found a less-than-significant association of the tryptophan hydroxylase A/A genotype with lithium response. In an expanded group of approximately 200 bipolar and depressed patients, they found no association between lithium efficacy and polymorphisms at the catechol O-methyltransferase, the monoamine oxidase (MAO)-A, and the G-protein β3 subunit loci, but they did find a positive association with the SLC6A4 promoter polymorphism (119), which they previously found predicted SSRI response (97). Finally, in a further expanded group of 443 bipolar and depressed patients, they found no association between 5-HT2A and MAO-A polymorphisms and lithium response (108). Interpretation of these results is difficult because of diagnostic heterogeneity in the patient groups. Furthermore, most of the candidate genes studied by these researchers were chosen for their putative association with mood disorders, rather than for a specific role in the mechanism of action of lithium.
Bipolar disorder is considered to have a high degree of heritability (120), and it has been suggested that bipolar patients with a strong family history may respond better to lithium (121, 122). Turecki and colleagues (123) performed a genome-wide scan to search for markers linked with lithium response in pedigrees with bipolar disease. Linkage was found between lithium response and markers on chromosomes 15 and 7. These interesting findings will require replication in other families.
Adverse Effects Induced by Psychotropic Drugs
There are often adverse effects arising from the use of psychiatric medications (2), in part due to limited data on the mechanism of action of these drugs. It may be that the best opportunity to counteract adverse effects is the use of molecular genetics to dissect the principal systems in the body that process psychiatric medications, including the liver, the blood-brain barrier, and the target receptors in the brain.
Antidepressant Drugs
Although the efficacy of antidepressant drugs has been studied relatively extensively with molecular genetic approaches, adverse events induced by antidepressant drugs, including weight gain, hypotension, sedation, anticholinergic effects, sleep disturbance, and antidepressant-induced mania, have received little attention. This list of adverse effects of antidepressants is by no means complete, but it points to the fact that a great deal more research is needed to fully exploit the power of molecular genetics to find predictors of the patients who will suffer the most from one or more of these adverse effects. An intriguing study has been published on the molecular genetic prediction of antidepressant-induced mania. Mundo et al. (124) screened more than 300 patients suffering from bipolar disorder in order to delineate a group of 29 patients who had been treated with serotonergic antidepressants and had developed a manic episode while taking that medication. As a control group, from the same large group of bipolar patients, 27 patients were selected who had received at least 10 weeks of treatment with serotonergic antidepressants and had not suffered a manic episode. In most cases, in this latter group, the patients had been treated several times with serotonergic antidepressants and had never suffered a manic episode. Mundo and colleagues examined the obvious candidate gene for SSRIs: SLC6A4. Two informative polymorphisms in this gene were examined: the promoter region polymorphism as well as a repeat polymorphism in the second intron. These two markers have a relatively low level of linkage disequilibrium between them, and thus, they reflect separate regions of genetic variation within the 5-HT transporter gene. The promoter polymorphism was shown to have significant association with antidepressant-induced mania, and the short allele was the variant associated with greater risk. In the previously discussed pharmacogenetic studies of antidepressant efficacy, the long allele was most often associated with antidepressant response. Thus, it is not immediately clear regarding the mechanism by which the short allele might increase the risk of manic episodes. The most important next step for the investigation of antidepressant-induced mania is to replicate these findings.
Antipsychotic Drugs
The main untoward effects of antipsychotic medications include tardive dyskinesia, weight gain, sedation, extrapyramidal symptoms, long-QT syndrome, blood lipid abnormalities, and diabetes (2). Pharmacogenetic studies have been conducted primarily on the adverse effects of tardive dyskinesia and weight gain.
Tardive dyskinesia
Because overactivity of dopamine transmission in the basal ganglia and up-regulation of D2-like receptors have been postulated to play a role in the pathophysiology of tardive dyskinesia (125), the genes for these receptors were the highest priority for molecular genetic study. The D2 receptor gene (DRD2) is the obvious candidate because it is the main site of action of typical antipsychotic medications (56, 57). Studies of genetic variation in DRD2 have yielded mostly negative results (126, 127). It should be noted that DRD2 is large relative to the other dopamine receptor genes, and thus, it is difficult for any one investigation to examine all of the polymorphic sites distributed across the more than 250-kb length of the gene. Most studies have focused on the common polymorphisms, including the TaqIA and B sites, as well as the serine to cysteine variant at amino acid position 311. Although further work with DRD2 may be helpful, we can summarize that the dopamine receptor genes other than the D3 gene have not shown consistent results in the prediction of tardive dyskinesia.
In the case of the D3 receptor gene (DRD3), several groups have independently shown that the variant consisting of a serine-to-glycine amino acid change at position 9 in the protein is associated with the risk of tardive dyskinesia (Table 3). Moreover, a recent meta-analysis revealed a significant role for the glycine allele in the risk for tardive dyskinesia (137). Parallel support for the role of this particular D3 polymorphism is provided by data suggesting that the glycine allele has significantly higher affinity for dopamine than the serine allele in transfected cell lines (138). Additional intriguing support for the role of DRD3 in tardive dyskinesia comes from a study of positron emission tomography (PET) imaging of patients who were genotyped for the Ser9Gly variant (139). These patients were scanned with PET before and after treatment with haloperidol. After the haloperidol treatment, the patients with the glycine/glycine genotype had greater fluorodeoxyglucose metabolism in the anterior striatum than the haloperidol-treated patients with one or two copies of the serine allele. The regional localization of the differential activity according to genotype fits well with the role of the striatum in normal motor behavior as well as possibly the abnormal motor movements seen in tardive dyskinesia. In this PET study, the patients who exhibited increased brain activity in the striatum displayed the most severe tardive dyskinesia symptoms.
In addition to the D3 pharmacodynamic factor, pharmacokinetic factors have also been examined. CYP2D6 is a high-affinity but low-capacity metabolic enzyme for haloperidol, whereas CYP1A2 has a higher capacity to metabolize haloperidol in the liver. Thus, with long-term treatment, CYP1A2 may be the most important determining factor of steady-state haloperidol levels. In a study of these genes in tardive dyskinesia (140), patients who had been rated with the Abnormal Involuntary Movement Scale (AIMS) for the symptoms of tardive dyskinesia were genotyped for the four common alleles at CYP2D6; no significant association was found. However, when the polymorphism in CYP1A2 at position 784 in the first intron was genotyped, patients with the C allele had higher AIMS ratings. However, this finding with CYP1A2 was not replicated in a German sample (141).
Basile et al. (140) also examined the combination of DRD3 with CYP1A2 to determine whether these two genes interact to predict the risk for tardive dyskinesia. Although methods to examine gene-gene interactions are not well defined, a relatively straightforward additive interaction between DRD3 and CYP1A2 in the risk for tardive dyskinesia was identified. In other words, the patients who had both the glycine/glycine genotype at DRD3 and the C/C genotype at CYP1A2 had the most severe tardive dyskinesia. The patients with only one or the other risk genotype had intermediate levels of symptoms. The overall model of prediction of tardive dyskinesia severity, which includes age, sex, ethnicity, and smoking status, in addition to the DRD3 and CYP1A2 gene polymorphisms, accounted for greater than 50% of the variance in the risk for tardive dyskinesia. This type of predictive algorithm, which incorporates genetic and environmental as well as demographic factors, appears promising as a method for predicting the risk for medication side effects.
Weight gain
Weight gain is a serious problem in patients taking antipsychotic medication for extended periods of time (142–144) and can lead to treatment noncompliance, psychological morbidity, and medical problems, including type II diabetes, hypertension, cardiovascular disease, and respiratory difficulties. Not all treated patients gain weight, however, leading to the notion that the susceptibility may be due to genetic factors. Similar to the tardive dyskinesia algorithm described in the previous section, the tendency to gain weight is likely due to a combination of factors, including CNS, hepatic, peripheral tissue (e.g., adipose and muscle), and environmental. Most genetic studies of antipsychotic-induced weight gain thus far have examined genes that code for CNS proteins, primarily receptors.
The selection of candidate genes to be studied in antipsychotic-induced weight gain has been based primarily on the neurobiologic knowledge of satiety mechanisms. It is quite possible that the medications also operate on peripheral mechanisms such as metabolic rate and muscle tone to decrease calorie burning and/or may directly increase lipogenesis, for example. These peripheral mechanisms have only begun to be examined by using molecular genetic strategies (145). Most studies of weight gain have focused on the serotonergic mechanisms that are thought to be involved in satiety. Satiety signals converge on the hypothalamus from several areas of the body, including taste and olfaction receptors, the stomach, the gut, and the liver. A survey of the literature of the regulation of feeding behavior points to a major role for serotonin, with both animal and human investigations showing, in general, that increasing serotonin results in decreased feeding, with the reverse also true. Pharmacologic agonists of the 5-HT1 family of receptors, primarily 1A, cause increased feeding, while agonists of 5-HT2C lead to decreased feeding (146). It follows that 5-HT2C antagonists will increase food intake (147). Of interest, clozapine is a potent 5-HT2C antagonist as well as a 5-HT1A agonist. 5-HT2C and 5-HT1A receptor genes are thus prime targets for the molecular genetic investigation of clozapine-induced weight gain. The histamine system is another important area, since some data suggest an association between weight gain and histamine H1 antagonism (148).
Recently, Reynolds et al. (149) reported an association between the –759 T/C SNP in the upstream putative promoter region of the 5-HT2C receptor gene and antipsychotic-induced weight gain. Evidence has been presented suggesting that this site alters 5-HT2C expression (150). Reynolds and colleagues studied this genetic marker in a group of 110 first-episode Asian patients who had been treated with risperidone or chlorpromazine. They found that the C allele significantly predicted weight gain. Basile et al. (15) were not able to replicate this finding in a group of 80 Caucasian subjects who were treated with clozapine; however, these patients were not treatment-naive before taking clozapine. Clearly, this is an interesting beginning, and more work is necessary to confirm the role of the 5-HT2C gene in antipsychotic-induced weight gain.
Next Steps: From Pharmacogenetics to Pharmacogenomics
To date, psychiatric pharmacogenetic studies have been primarily restricted to relatively small data sets derived from ethnically heterogeneous populations, with genotyping of a single SNP or limited numbers of SNPs. Recent advances in genomic information, novel statistical genetic methods, and marked improvements in genotyping technologies now provide the means of considering far more comprehensive and powerful pharmacogenetic studies.
The Human Genome Sequence and SNP identification
The completion of the human genome sequence by the federally funded Human Genome Project (12) and a private biotechnology firm (151) is a landmark event in the history of science. Although complete sequence data are not yet available, the genome sequence that is complete is already providing the reference sequence for large-scale efforts aiming to identify genetic variation among individuals. In particular, there has been a focus on the identification of hundreds of thousands of SNPs within the genome.
Many of the SNPs that are being identified may be useful in pharmacogenetic studies. Cargill et al. (152) scanned the coding region of 106 genes, many with potential relevance to the CNS, and identified an average of 3.7 coding region SNPs per gene, of which nearly 50% were nonsynonymous; 41% of the nonsynonymous coding region SNPs had a minor allele frequency of greater than 5%. Nonsynonymous coding region SNPs may have a higher a priori probability of altering function and be the most powerful in pharmacogenetic studies. Therefore, these massive SNP identification efforts, coupled with data demonstrating that a significant subset of them may be useful in pharmacogenetic studies, suggest that a large number of novel SNP targets will soon be available for the next generation of pharmacogenetic studies.
Genomic Control Techniques
Most previous pharmacogenetic studies have used the case-control design in which, for example, unrelated subjects who respond to medication are compared to nonresponsive patients (13). Although powerful, this approach may be vulnerable to false positive results secondary to undetected ethnic stratification between study groups. Family-based approaches, in which DNA is collected from the parents or siblings of probands, are an alternative strategy that is not susceptible to stratification confounds (153) but are less feasible and less powerful than the traditional case-control method for pharmacogenetic studies. First, many of the patients participating in clinical trials of psychotropic drugs are already in their mid to late adult years; therefore, collecting DNA from family members may be difficult because of a lack of availability. Second, it has been suggested that probands selected from trios or other family-based designs may be subtly different from the general population of cases, thus reducing the generalizability of findings made with family-based designs (154). Finally, the case-control design has greater statistical power than family-based approaches (155). These data suggest that the case-control design would be optimal for pharmacogenetic studies; however, the potential for undetected ethnic stratification has tempered enthusiasm for this design (156).
Fortunately, “genomic control” methods are under development to help account for ethnic stratification in case-control studies. Genomic control techniques are based upon the idea that study groups (patients versus control subjects, responders versus nonresponders, etc.) can be tested for the presence of stratification by assessing the allele frequency of markers that are unlinked to the phenotype of interest in each group. Pritchard and Rosenberg (157) determined that, in theory, no more than 40 unlinked markers, and perhaps less, are necessary to achieve a 95% probability of detecting stratification in study groups of over 200 subjects. If stratification is detected, subjects can be removed until stratification is not present or correction factors can be introduced that account for the level of stratification between groups (158). Both approaches reduce study power but minimize the risk that the case-control design results in a false positive or negative result. Several studies have appeared recently that used genomic controls to test for population stratification. Xu and colleagues (159) studied the relationship between SNP markers in the delta opioid receptor in Chinese subjects in a case-control design. As genomic control markers, they chose well-characterized SNPs in four additional genes. The SNPs were chosen because they showed variation in allele frequencies among Asian ethnic subgroups, as well as variation between Asians and Caucasians. Small and co-workers (160) examined adrenergic receptor polymorphisms and the risk for hypertension in a case-control design. Nine highly polymorphic short tandem-repeat loci were used to control for stratification, although it is not stated whether these markers show variation in allele frequencies among ethnic subpopulations.
Important issues remain regarding the use of genomic controls. First, should markers within genes be chosen, or should markers distant from any known gene be used? Markers within genes are likely to be better characterized, but in theory, they could be functionally associated with the outcome measure. The optimal degree of heterozygosity for genomic controls and variation in genomic control allele frequencies among ethnic groups and subgroups is also unclear. Finally, there are no standards as yet to either the number of control loci that should be tested or the number of positive results indicative of stratification. These issues will require further work.
Haplotypes
Pharmacogenetic studies that include hundreds—if not thousands—of SNPs in multiple candidate genes must address the issue of multiple testing. One potential method for data reduction is to organize SNPs within a gene, or a region of the genome, into haplotypes (or specific chromosomal combinations of individual alleles of each SNP across a region). Haplotypes provide more comprehensive examinations of single genes and may provide increased power to detect pharmacogenetic associations if rare alleles are contributing to phenotypic differences (161). In many genes studied to date, there are a limited number of common haplotypes (162), and this may reduce the extent of genotyping necessary to analyze a gene and also reduce the number of SNP comparisons that need to be conducted.
Progress on the identification of haplotypes within the human genome is under way. The National Institutes of Health is supporting a haplotype map-sequencing effort that will provide publicly available haplotype information across the genome (163). Moreover, private biotechnology firms have assessed large numbers of genes for haplotype structure (161), with many of these genes coding for CNS proteins that are thought to be involved in psychotropic drug response, and these data may be available for pharmacogenetic studies.
Clinical Applications
Although research in pharmacogenetics is growing rapidly, with intriguing preliminary data across a number of phenotypes, a major goal of psychiatric pharmacogenetics—clinical applicability—has not yet been fully realized. For this to be accomplished, more replicated data will be required, and the amount of variance explained by any single gene or set of genes will need to be significantly greater than yet observed. With this, pretreatment testing of patients may be possible, and more specific targets for new drug development may be achieved. Since the feasibility of genotyping is high, and the costs continue to decrease, it does seem likely that within the next few years pharmacogenetic data will begin to play an important role in clinical decision making about individual patient treatment.
Conclusions
Pharmacogenetic studies of psychotropic drug response have begun to identify several genes that may be implicated in diverse phenotypes, such as antipsychotic drug efficacy, antidepressant drug response, and development of drug-induced adverse events. These genes require further study, as well as careful functional genomics working to identify the specific molecular events that may produce clinical effects in order to contribute to future drug development strategies.
More dramatic progress, however, may be made with the next generation of molecular genetic studies of psychotropic drug response. It is now possible to collect DNA from patients enrolled in large-scale clinical trials of thousands of patients, select SNPs that occur in many of the genes expressed in the CNS, and compare SNP allele frequencies in large case-control designs. Moreover, as methods are developed to account for undetected ethnic stratification between groups, the amount of stratification (if any) can be quantified between groups and accounted for in the analyses. Improved bioinformatics tools and statistical methods will be required to deal with the complexity of data that will be generated, but it seems likely that such methods may become available as the level of interest in genetic approaches to complex diseases and to phenotypes such as drug response increases. With these developments in place, the first generation of pharmacogenetic studies that used SNPs in relatively limited numbers of candidate genes may be replaced by studies in which the complete genome is assessed, inaugurating the era of pharmacogenomic studies of psychotropic drug response.
![]() |
![]() |
![]() |
Received Jan. 22, 2003; revision received Aug. 20, 2003; accepted Aug. 22, 2003. From the Department of Psychiatry Research, Zucker Hillside Hospital, North Shore-Long Island Jewish Health System; Stanford University School of Medicine, Stanford, Calif.; and the Department of Psychiatry, University of Toronto, Toronto, Ont., Canada. Address reprint requests to Dr. Malhotra, Department of Psychiatry Research, Zucker Hillside Hospital, 75-59 263rd St., Glen Oaks, NY 11004; [email protected] (e-mail).
![]() |
APPENDIX 1
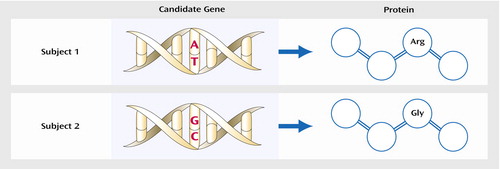
Figure 1. Testing of a Single Nucleotide Polymorphism (SNP) in the Coding Region of a Gene, Resulting in an Alteration of the Amino Acid Sequence of the Corresponding Proteina
aIn the figure, two individuals’ DNA sequences differ at one locus (an adenine-to-guanine SNP), with resultant amino acid substitution in the protein: arginine (Arg) versus glycine (Gly). Because these two subjects’ protein structures are distinct, this could result in phenotypic differences between the subjects, such as variation in response to medication.
1. Kane JM: Pharmacological treatment of schizophrenia. Biol Psychiatry 1999; 46:1396–1408Crossref, Medline, Google Scholar
2. Malhotra AK, Litman RE, Pickar D: Adverse effects of antipsychotic drugs. Drug Safety 1993; 9:429–436Crossref, Medline, Google Scholar
3. Conley RR, Carpenter WT Jr, Tamminga CA: Time to clozapine response in a standardized trial. Am J Psychiatry 1997; 154:1243–1247Link, Google Scholar
4. Kaplan HI, Sadock BJ, Grebb JA: Kaplan and Sadock’s Synopsis of Psychiatry: Behavioral Sciences and Clinical Psychiatry, 7th ed. Baltimore, Williams & Wilkins, 1994Google Scholar
5. Bowers MB, Swigar ME, Jatlow PI, Goicoechea N: Plasma catecholamine metabolites and early response to haloperidol. J Clin Psychiatry 1984; 45:248–251Medline, Google Scholar
6. Buchsbaum MS, Potkin SG, Marshall JF, Lottenberg S, Teng C, Heh CW, Tafalla R, Reynolds C, Abel L, Plon L: Effects of clozapine and thiothixene on glucose metabolic rate in schizophrenia. Neuropsychopharmacology 1992; 6:155–163Medline, Google Scholar
7. Kahn RS, Davidson M, Siever L, Gabriel S, Apter S, Davis KL: Serotonin function and treatment response to clozapine in schizophrenic patients. Am J Psychiatry 1993; 150:1337–1342Link, Google Scholar
8. Pickar D, Labaraca R, Linnoila M, Roy A, Hommer D, Everett D, Paul SM: Neuroleptic-induced decrease in plasma homovanillic acid and antipsychotic activity in schizophrenic patients. Science 1984; 225:954–957Crossref, Medline, Google Scholar
9. Szymanski S, Lieberman J, Pollack S, Safferman A, Munne R, Umbricht D, Kane J, Kronig M, Chakos M, Cooper T: Clozapine effects on neuroendocrine response to apomorphine challenge testing in chronic neuroleptic nonresponsive schizophrenia: preliminary findings. Biol Psychiatry 1995; 37:52–55Crossref, Medline, Google Scholar
10. Strachan T, Read AP: Mutation and instability of human DNA. Hum Mol Genet 1996; 10:241–273Google Scholar
11. Ranade K, Chang MS, Ting CT, Pei D, Hsiao CF, Olivier M, Pesich R, Herbert J, Chen YD, Dzau VJ, Curb D, Olshen R, Risch N, Cox DR, Botstein D: High-throughput genotyping with single nucleotide polymorphisms. Genome Res 2001; 11:1262–1268Medline, Google Scholar
12. Lander ES, Linton LM, Birren B, Nusbaum C, Zody MC, Baldwin J, Devon K, Dewar K, Doyle M, FitzHugh W, et al: Initial sequencing and analysis of the human genome. Nature 2001; 409:860–921; corrections, 411:7204 and 412:565Crossref, Medline, Google Scholar
13. Malhotra AK, Goldman D: Benefits and pitfalls encountered in psychiatric genetic association studies. Biol Psychiatry 1999; 45:544–550Crossref, Medline, Google Scholar
14. Berrettini W: Psychiatric pharmacogenetics: a developing science. Neuropsychopharmacology 2002; 26:128–129Crossref, Medline, Google Scholar
15. Basile VS, Masellis M, De Luca V, Meltzer HY, Kennedy JL: 759 C/T genetic variation of 5HT(2C) receptor and clozapine-induced weight gain. Lancet 2002; 360:1790–1791Crossref, Medline, Google Scholar
16. Plomin R, Owen MJ, McGuffin P: The genetic basis of complex human behaviors. Science 1994; 264:1733–1739Crossref, Medline, Google Scholar
17. Gottesman II: Schizophrenia Genesis: The Origins of Madness. New York, WH Freeman, 1991Google Scholar
18. Angst J: Antidepressiver effect und genetische faktoren. Arzneimittelforschung 1964; 14(June suppl):496–500Google Scholar
19. Pare CMB, Rees L, Sainsbury MJ: Differentiation of two genetically specific types of depression by the response to anti-depressants. Lancet 1962; 2:1340–1343Medline, Google Scholar
20. Pare CMB, Mack JW: Differentiation of two genetically specific types of depression by the response to antidepressant drugs. J Med Genet 1971; 8:306–309Crossref, Medline, Google Scholar
21. O’Reilly RL, Bogue L, Singh SM: Pharmacogenetic response to antidepressants in a multi-case family with affective disorder. Biol Psychiatry 1994; 36:467–471Crossref, Medline, Google Scholar
22. Franchini L, Serretti A, Gasperini M, Smeraldi E: Familial concordance of fluvoxamine response as a tool for differentiating mood disorder pedigrees. J Psychiatr Res 1998; 32:255–259Crossref, Medline, Google Scholar
23. Emsley RA, Roberts MC, Rataemane S, Pretorius J, Oosthuizen PP, Turner J, Niehaus DJ, Keyter N, Stein DJ: Ethnicity and treatment response in schizophrenia: a comparison of 3 ethnic groups. J Clin Psychiatry 2002; 63:9–14Crossref, Medline, Google Scholar
24. Kuno E, Rothbard AB: Racial disparities in antipsychotic prescription patterns for patients with schizophrenia. Am J Psychiatry 2002; 159:567–572Link, Google Scholar
25. DeLisi LE, Dauphinais D: Neuroleptic responsiveness in siblings concordant for schizophrenia (letter). Arch Gen Psychiatry 1989; 46:477Crossref, Medline, Google Scholar
26. Vojvoda D, Grimmell K, Sernyak M, Mazure CM: Monozygotic twins concordant for response to clozapine (letter). Lancet 1996; 347:61; corrections, 347:482 and 347:1054Crossref, Medline, Google Scholar
27. Mata I, Madoz V, Arranz MJ, Sham P, Murray RM: Olanzapine: concordant response in monozygotic twins with schizophrenia (letter). Br J Psychiatry 2001; 178:86Crossref, Google Scholar
28. Kane JM, Honigfeld G, Singer J, Meltzer H: Clozapine for the treatment-resistant schizophrenic: a double blind comparison with chlorpromazine. Arch Gen Psychiatry 1988; 45:789–796Crossref, Medline, Google Scholar
29. Malhotra AK, Goldman D, Ozaki N, Breier A, Buchanan R, Pickar D: Lack of association between polymorphisms in the 5-HT2A receptor gene and the antipsychotic response to clozapine. Am J Psychiatry 1996; 153:1092–1094Link, Google Scholar
30. Malhotra AK, Goldman D, Ozaki N, Rooney W, Clifton A, Buchanan RW, Breier A, Pickar D: Clozapine response and the 5HT2C Cys23Ser polymorphism. Neuroreport 1996; 7:2100–2102Crossref, Medline, Google Scholar
31. Arranz M, Collier D, Sodhi M, Ball D, Roberts G, Price J, Sham P, Kerwin R: Association between clozapine response and allelic variation in 5-HT2A receptor gene. Lancet 1995; 346:281–282Crossref, Medline, Google Scholar
32. Sodhi MS, Arranz MJ, Curtis D, Ball DM, Sham P, Roberts GW, Price J, Collier DA, Kerwin RW: Association between clozapine response and allelic variation in the 5-HT2C receptor gene. Neuroreport 1995; 7:169–172Crossref, Medline, Google Scholar
33. Nimgaonkar VL, Zhang XR, Brar JS, DeLeo M, Ganguli R: 5-HT2 receptor gene locus: association with schizophrenia or treatment response not detected. Psychiatr Genet 1996; 6:23–27Crossref, Medline, Google Scholar
34. Shaikh S, Collier DA, Sham PC, Ball D, Aitchison K, Vallada H, Smith I, Gill M, Kerwin RW: Allelic association between a Ser-9-Gly polymorphism in the dopamine D3 receptor gene and schizophrenia. Hum Genet 1996; 97:714–719Crossref, Medline, Google Scholar
35. Nothen MM, Rietschel M, Erdmann J, Oberlander H, Moller HJ, Nober D, Propping P: Genetic variation of the 5-HT2A receptor and response to clozapine. Lancet 1995; 346:908–909Google Scholar
36. Lin CH, Tsai SJ, Yu YW, Song HL, Tu PC, Sim CB, Hsu CP, Yang KH, Hong CJ: No evidence for association of serotonin-2A receptor variant (102T/C) with schizophrenia or clozapine response in a Chinese population. Neuroreport 1999; 10:57–60Crossref, Medline, Google Scholar
37. Masellis M, Basile V, Meltzer HY, Lieberman JA, Sevy S, Macciardi FM, Cola P, Howard A, Badri F, Nothen MM, Kalow W, Kennedy JL: Serotonin subtype 2 receptor genes and clinical response to clozapine in schizophrenia patients. Neuropsychopharmacology 1998; 19:123–132Crossref, Medline, Google Scholar
38. Burnet PW, Harrison PJ: Genetic variation of the 5-HT2A receptor and response to clozapine (letter). Lancet 1995; 346:909Crossref, Google Scholar
39. Lane H-Y, Chang Y-C, Chiu C-C, Chen M-L, Hsieh M-H, Chang W-H: Association of risperidone treatment response with a polymorphism in the 5-HT2A receptor gene. Am J Psychiatry 2002; 159:1593–1595Link, Google Scholar
40. Masellis M, Paterson AD, Badri F, Lieberman JA, Meltzer HY, Cavazzoni P, Kennedy JL: Genetic variation of 5-HT2A receptor and response to clozapine (letter). Lancet 1995; 346:1108Google Scholar
41. Arranz MJ, Munro J, Owen MJ, Spurlock G, Sham PC, Zhao J, Kirov G, Collier DA, Kerwin RW: Evidence for association between polymorphisms in the promoter and coding regions of the 5-HT2A receptor gene and response to clozapine. Mol Psychiatry 1998; 3:61–66Crossref, Medline, Google Scholar
42. Arranz MJ, Munro J, Sham P, Kirov G, Murray RM, Collier DA, Kerwin RW: Meta-analysis of studies on genetic variation in 5-HT2A receptors and clozapine response. Schizophr Res 1998; 32:93–99Crossref, Medline, Google Scholar
43. Reitschel M, Naber D, Fimmers R, Moller HJ, Propping P, Nothen MM: Efficacy and side-effects of clozapine not associated with variation in the 5-HT2C receptor. Neuroreport 1997; 8:1999–2003Crossref, Medline, Google Scholar
44. Yu YW, Tsai SJ, Lin CH, Hsu CP, Yang KH, Hong CJ: Serotonin-6 receptor variant (C267T) and clinical response to clozapine. Neuroreport 1999; 10:1231–1233Crossref, Medline, Google Scholar
45. Masellis M, Basile VS, Meltzer HY, Lieberman JA, Sevy S, Goldman DA, Hamblin MW, Macciardi FM, Kennedy JL: Lack of association between the T→C 267 serotonin 5-HT6 receptor gene (HTR6) polymorphism and prediction of response to clozapine in schizophrenia. Schizophr Res 2001; 47:49–58Crossref, Medline, Google Scholar
46. Tsai SJ, Hong CJ, Yu YW-Y, Lin C, Song H, Lai H, Yang K: Association study of a functional serotonin transporter gene polymorphism with schizophrenia, psychopathology and clozapine response. Schizophr Res 2000; 44:177–181Crossref, Medline, Google Scholar
47. Arranz MJ, Bolonna AA, Munro J, Curtis CJ, Collier DA, Kerwin RW: The serotonin transporter and clozapine response. Mol Psychiatry 2000; 5:124–125Crossref, Medline, Google Scholar
48. Rao PA, Pickar D, Gejman PV, Ram A, Gershon ES, Gelernter J: Allelic variation in the D4 dopamine receptor (DRD4) gene does not predict response to clozapine. Arch Gen Psychiatry 1994; 51:912–917Crossref, Medline, Google Scholar
49. Shaikh S, Collier DA, Sham P, Pilowsky L, Sharma T, Lin LK, Crocq MA, Gill M, Kerwin R: Analysis of clozapine response and polymorphisms of the dopamine D4 receptor gene (DRD4) in schizophrenic patients. Am J Med Genet 1995; 60:541–550Crossref, Medline, Google Scholar
50. Van Tol HH, Bunzow JR, Guan HC, Sunahara RK, Seeman P, Niznik HB, Civelli O: Cloning of the gene for a human dopamine D-4 receptor with high affinity for the antipsychotic clozapine. Nature 1991; 350:610–614Crossref, Medline, Google Scholar
51. Van Tol HH, Wu CM, Guan HC, Ohara K, Bunzow, JR, Civelli O, Kennedy J, Seeman P, Niznik HB, Jovanovic V: Multiple dopamine D4 receptor variants in the human population. Nature 1992; 358:149–152Crossref, Medline, Google Scholar
52. Reitschel M, Naber D, Oberlander H, Holzbach R, Fimmers R, Eggermann K, Moller HJ, Propping P, Nothen MM: Efficacy and side-effects of clozapine: testing for association with allelic variation in the dopamine D4 receptor gene. Neuropsychopharmacology 1996; 15:491–496Crossref, Medline, Google Scholar
53. Kohn Y, Ebstein RP, Heresco-Levy U, Shapira B, Nemanov L, Gritsenko I, Avnon M, Lerer B: Dopamine D4 receptor gene polymorphisms: relation to ethnicity, no association with schizophrenia and response to clozapine in Israeli subjects. Eur Neuropsychopharmacol 1997; 1:39–43Google Scholar
54. Ozdemir V, Masellis M, Basile VS, Kalow W, Meltzer HY, Lieberman JA, Kennedy JL: Variability in response to clozapine: potential role of cytochrome P450 1A2 and the dopamine D4 receptor gene. CNS Spectrums 1999; 4:30–56Google Scholar
55. Kramer MS, Last B, Getson A, Reines SA, Zimbroff D, Hafez H, Alpert M, Rotrosen J, McEvoy J, Kane J, Kronig M, Merideth C, Ereshefsky L, Marder S, Ames D: The effects of a selective D4 dopamine receptor antagonist (L-745,870) in acutely psychotic inpatients with schizophrenia. Arch Gen Psychiatry 1997; 54:567–572Crossref, Medline, Google Scholar
56. Creese I, Burt DR, Snyder SH: Dopamine receptor binding predicts clinical and pharmacological potencies of antischizophrenic drugs. Science 1976; 192:481–483Crossref, Medline, Google Scholar
57. Seeman P: Dopamine receptor sequences: therapeutic levels of neuroleptics occupy D2 receptors, clozapine occupies D4. Neuropsychopharmacology 1992; 7:261–284Medline, Google Scholar
58. Kapur S, Seeman P: Does fast dissociation from the dopamine D2 receptor explain the action of atypical antipsychotics? a new hypothesis. Am J Psychiatry 2001; 158:360–369Link, Google Scholar
59. Gejman PV, Ram A, Gelernter J, Friedman E, Cao Q, Pickar D, Blum K, Noble EP, Kranzler HR, O’Malley S: No structural mutation in the dopamine D2 receptor gene in alcoholism or schizophrenia: analysis using denaturing gradient gel electrophoresis. JAMA 1994; 271:204–208Crossref, Medline, Google Scholar
60. Malhotra AK, Buchanan RW, Kim S, Kestler L, Breier A, Pickar D, Goldman D: Allelic variation in the promoter region of the dopamine D2 receptor gene and clozapine response. Schizophr Res 1999; 36:92–93Google Scholar
61. Suzuki A, Mihara K, Kondo T, Tanaka O, Nagashima U, Otani K, Kaneko S: The relationship between dopamine D2 receptor polymorphism at the Taq1 A locus and therapeutic response to nemonapride, a selective dopamine antagonist, in schizophrenic patients. Pharmacogenetics 2000; 10:335–341Crossref, Medline, Google Scholar
62. Schäfer M, Rujescu D, Giegling I, Guntermann A, Erfurth A, Bondy B, Möller H-J: Association of short-term response to haloperidol treatment with a polymorphism in the dopamine D2 receptor gene. Am J Psychiatry 2001; 158:802–804Link, Google Scholar
63. Mata I, Arranz MJ, Lai T, Mancama D, Arrondo J, Beperet M, Villavicencio R, Munro J, Osborne S, Kerwin RW: The serotonergic system influences individual’s response to risperidone. Am J Med Genet 2002; 114:728Google Scholar
64. Suzuki A, Kondo T, Mihara K, Yasui-Furukori N, Ishida M, Furukori H, Kaneko S, Inoue Y, Otani K: The -141C Ins/Del polymorphism in the dopamine D2 receptor gene promoter region is associated with anxiolytic and antidepressive effects during treatment with dopamine antagonists in schizophrenic patients. Pharmacogenetics 2001; 11:545–550Crossref, Medline, Google Scholar
65. Arranz MJ, Li T, Munro J, Liu X, Murray R, Collier DA, Kerwin RW: Lack of association between a polymorphism in the promoter region of the dopamine-2 receptor gene and clozapine response. Pharmacogenetics 1998; 8:481–484Crossref, Medline, Google Scholar
66. Arranz MJ, Munro J, Birkett J, Bolonna A, Mancama D, Sodhi M, Lesch KP, Meyer JF, Sham P, Collier DA, Murray RM, Kerwin RW: Pharmacogenetic prediction of clozapine response. Lancet 2000; 355:1615–1616Crossref, Medline, Google Scholar
67. Schumacher J, Schulze TG, Wienker TF, Rietschel M, Nothen MM: Pharmacogenetics of the clozapine response. Lancet 2000; 356:506–507Google Scholar
68. Bertilsson L, Dahl ML, Dalen P, Al-Shurbaji A: Molecular genetics of CYP2D6: clinical relevance with focus on psychotropic drugs. Br J Clin Pharmacol 2002; 53:111–122Crossref, Medline, Google Scholar
69. Sachse C, Brockmoller J, Bauer S, Roots I: Cytochrome P450 2D6 variants in a Caucasian population: allele frequencies and phenotypic consequences. Am J Hum Genet 1997; 60:284–295Medline, Google Scholar
70. Marez D, Legrand M, Sabbagh N, Guidice JM, Spire C, Lafitte JJ, Meyer UA, Broly F: Polymorphism of the cytochrome P450 CYP2D6 gene in a European population: characterization of 48 mutations and 53 alleles, their frequencies and evolution. Pharmacogenetics 1997; 7:193–202Crossref, Medline, Google Scholar
71. Raimundo S, Fischer J, Eichelbaum M, Griese EU, Schwab M, Zanger UM: Elucidation of the genetic basis of the common “intermediate metabolizer” phenotype for drug oxidation by CYP2D6. Pharmacogenetics 2000; 10:577–581Crossref, Medline, Google Scholar
72. Johansson I, Lundqvist E, Bertilsson L, Dahl ML, Sjoqvist F, Ingelman-Sundberg M: Inherited amplification of an active gene in the cytochrome P450 CYP2D locus as a cause of ultrarapid metabolism of debrisoquine. Proc Natl Acad Sci USA 1993; 90:11825–11829Crossref, Medline, Google Scholar
73. Dalen P, Dahl ML, Ruiz ML, Nordin J, Bertilsson L: 10-Hydroxylation of nortriptyline in white persons with 0, 1, 2, 3, and 13 functional CYP2D6 genes. Clin Pharmacol Ther 1998; 63:444–452Crossref, Medline, Google Scholar
74. Sindrup SH, Brosen K, Gram LF: Pharmacokinetics of the selective serotonin reuptake inhibitor paroxetine: nonlinearity and relation to the sparteine oxidation polymorphism. Clin Pharmacol Ther 1992; 51:288–295Crossref, Medline, Google Scholar
75. Yue QY, Zhong ZH, Tybring G, Dalen P, Dahl ML, Bertilsson L, Sjoqvist F: Pharmacokinetics of nortriptyline and its 10-hydroxy metabolite in Chinese subjects of different CYP2D6 genotypes. Clin Pharmacol Ther 1998; 64:384–390Crossref, Medline, Google Scholar
76. Fukuda T, Yamamoto I, Nishida Y, Zhou Q, Ohno M, Takada K, Azuma J: Effect of the CYP2D6*10 genotype on venlafaxine pharmacokinetics in healthy adult volunteers. Br J Clin Pharmacol 1999; 47:450–453Crossref, Medline, Google Scholar
77. Murphy GM Jr, Pollock BG, Kirshner MA, Pascoe N, Cheuk W, Mulsant BH, Reynolds CF III: CYP2D6 genotyping with oligonucleotide microarrays and nortriptyline concentrations in geriatric depression. Neuropsychopharmacology 2001; 25:737–743Crossref, Medline, Google Scholar
78. Chou WH, Yan FX, de Leon J, Barnhill J, Rogers T, Cronin M, Pho M, Xiao V, Ryder TB, Liu WW, Teiling C, Wedlund PJ: Extension of a pilot study: impact from the cytochrome P450 2D6 polymorphism on outcome and costs associated with severe mental illness. J Clin Psychopharmacol 2000; 20:246–251Crossref, Medline, Google Scholar
79. Murphy GM Jr, Kremer C, Rodrigues HE, Schatzberg AF: Pharmacogenetics of antidepressant medication intolerance. Am J Psychiatry 2003; 160:1830–1835Link, Google Scholar
80. Solai LK, Pollock BG, Mulsant BH, Frye RF, Miller MD, Sweet RA, Kirshner M, Sorisio D, Begley A, Reynolds CF III: Effect of nortriptyline and paroxetine on CYP2D6 activity in depressed elderly patients. J Clin Psychopharmacol 2002; 22:481–486Crossref, Medline, Google Scholar
81. Yoon YR, Cha IJ, Shon JH, Kim KA, Cha YN, Jang IJ, Park CW, Shin SG, Flockhart DA, Shin JG: Relationship of paroxetine disposition to metoprolol metabolic ratio and CYP2D6*10 genotype of Korean subjects. Clin Pharmacol Ther 2000; 67:567–576Crossref, Medline, Google Scholar
82. Alfaro CL, Lam YW, Simpson J, Ereshefsky L: CYP2D6 inhibition by fluoxetine, paroxetine, sertraline, and venlafaxine in a crossover study: intraindividual variability and plasma concentration correlations. J Clin Pharmacol 2000; 40:58–66Crossref, Medline, Google Scholar
83. Ozdemir V, Naranjo CA, Shulman RW, Herrmann N, Sellers EM, Reed K, Kalo W: Determinants of interindividual variability and extent of CYP2D6 and CYP1A2 inhibition by paroxetine and fluvoxamine in vivo. J Clin Psychopharmacol 1998; 18:198–207Crossref, Medline, Google Scholar
84. Sindrup SH, Brosen K, Gram LF, Hallas J, Skjelbo E, Allen A, Allen GD, Cooper SM, Mellows G, Tasker TC, et al: The relationship between paroxetine and the sparteine oxidation polymorphism. Clin Pharmacol Ther 1992; 51:278–287Crossref, Medline, Google Scholar
85. DeVane CL: Pharmacokinetics, drug interactions, and tolerability of paroxetine and paroxetine CR. Psychopharmacol Bull 2003; 37:29–41Google Scholar
86. Kirchheiner J, Brosen K, Dahl ML, Gram LF, Kasper S, Roots I, Sjoqvist F, Spina E, Brockmoller J: CYP2D6 and CYP2C19 genotype-based dose recommendations for antidepressants: a first step towards subpopulation-specific dosages. Acta Psychiatr Scand 2001; 104:173–192Crossref, Medline, Google Scholar
87. Nemeroff CB, DeVane CL, Pollock BG: Newer antidepressants and the cytochrome P450 system. Am J Psychiatry 1996; 153:311–320Link, Google Scholar
88. Preskorn SH, Magnus RD: Inhibition of hepatic P-450 isoenzymes by serotonin selective reuptake inhibitors: in vitro and in vivo findings and their implications for patient care. Psychopharmacol Bull 1994; 30:251–259Medline, Google Scholar
89. Alderman J, Preskorn SH, Greenblatt DJ, Harrison W, Penenberg D, Allison J, Chung M: Desipramine pharmacokinetics when coadministered with paroxetine or sertraline in extensive metabolizers. J Clin Psychopharmacol 1997; 17:284–291Crossref, Medline, Google Scholar
90. Preskorn SH, Baker B: Fatality associated with combined fluoxetine-amitriptyline therapy (letter). JAMA 1997; 277:1682Google Scholar
91. Meyer UA, Amrein R, Balant LP, Bertilsson L, Eichelbaum M, Guentert TW, Henauer S, Jackson P, Laux G, Mikkelsen H, Peck C, Pollock BG, Priest R, Sjoqvist F, Delini-Stula A: Antidepressants and drug-metabolizing enzymes—expert group report. Acta Psychiatr Scand 1996; 93:71–79Crossref, Medline, Google Scholar
92. DeVane CL, Markowitz JS, Liston HL, Risch SC: Charleston Antidepressant Drug Interactions Surveillance Program (CADISP). Psychopharmacol Bull 2001; 35:50–61Medline, Google Scholar
93. Lamba JK, Lin YS, Thummel K, Daly A, Watkins PB, Strom S, Zhang J, Schuetz EG: Common allelic variants of cytochrome P4503A4 and their prevalence in different populations. Pharmacogenetics 2002; 12:121–132Crossref, Medline, Google Scholar
94. Brosen K, Naranjo CA: Review of pharmacokinetic and pharmacodynamic interaction studies with citalopram. Eur Neuropsychopharmacol 2001; 11:275–283Crossref, Medline, Google Scholar
95. Timmer CJ, Sitsen JM, Delbressine LP: Clinical pharmacokinetics of mirtazapine. Clin Pharmacokinet 2000; 38:461–474Crossref, Medline, Google Scholar
96. Burke MJ, Preskorn SH: Therapeutic drug monitoring of antidepressants: cost implications and relevance to clinical practice. Clin Pharmacokinet 1999; 37:147–165Crossref, Medline, Google Scholar
97. Smeraldi E, Zanardi R, Benedetti F, Di Bella D, Perez J, Catalano M: Polymorphism within the promoter of the serotonin transporter gene and antidepressant efficacy of fluvoxamine. Mol Psychiatry 1998; 3:508–511Crossref, Medline, Google Scholar
98. Pollock BG, Ferrell RE, Mulsant BH, Mazumdar S, Miller M, Sweet RA, Davis S, Kirshner MA, Houck PR, Stack JA, Reynolds CF, Kupfer DJ: Allelic variation in the serotonin transporter promoter affects onset of paroxetine treatment response in late-life depression. Neuropsychopharmacology 2000; 23:587–590Crossref, Medline, Google Scholar
99. Zanardi R, Benedetti F, Di Bella D, Catalano M, Smeraldi E: Efficacy of paroxetine in depression is influenced by a functional polymorphism within the promoter of the serotonin transporter gene. J Clin Psychopharmacol 2000; 20:105–107Crossref, Medline, Google Scholar
100. Kim DK, Lim SW, Lee S, Sohn SE, Kim S, Hahn CG, Carroll BJ: Serotonin transporter gene polymorphism and antidepressant response. Neuroreport 2000; 11:215–219Crossref, Medline, Google Scholar
101. Minov C, Baghai TC, Schule C, Zwanzger P, Schwarz MJ, Zill P, Rupprecht R, Bondy B: Serotonin-2A-receptor and -transporter polymorphisms: lack of association in patients with major depression. Neurosci Lett 2001; 303:119–122Crossref, Medline, Google Scholar
102. Yoshida K, Ito K, Sato K, Takahashi H, Kamata M, Higuchi H, Shimizu T, Itoh K, Inoue K, Tezuka T, Suzuki T, Ohkubo T, Sugawara K, Otani K: Influence of the serotonin transporter gene-linked polymorphic region on the antidepressant response to fluvoxamine in Japanese depressed patients. Prog Neuropsychopharmacol Biol Psychiatry 2002; 26:383–386Crossref, Medline, Google Scholar
103. Greenberg BD, Tolliver TJ, Huang SJ, Li Q, Bengel D, Murphy DL: Genetic variation in the serotonin transporter promoter region affects serotonin uptake in human blood platelets. Am J Med Genet 1999; 88:83–87Crossref, Medline, Google Scholar
104. Heils A, Teufel A, Petri S, Stober G, Riederer P, Bengel D, Lesch KP: Allelic variation of human serotonin transporter gene expression. J Neurochem 1996; 66:2621–2624Crossref, Medline, Google Scholar
105. Serretti A, Zanardi R, Cusin C, Rossini D, Lorenzi C, Smeraldi E: Tryptophan hydroxylase gene associated with paroxetine antidepressant activity. Eur Neuropsychopharmacol 2001; 11:375–380Crossref, Medline, Google Scholar
106. Serretti A, Zanardi R, Rossini D, Cusin C, Lilli R, Smeraldi E: Influence of tryptophan hydroxylase and serotonin transporter genes on fluvoxamine antidepressant activity. Mol Psychiatry 2001; 6:586–592Crossref, Medline, Google Scholar
107. Stahl SM: Basic psychopharmacology of antidepressants, part 1: antidepressants have seven distinct mechanisms of action. J Clin Psychiatry 1998; 59(suppl 4):5–14Google Scholar
108. Cusin C, Serretti A, Zanardi R, Lattuada E, Rossini D, Lilli R, Lorenzi C, Smeraldi E: Influence of monoamine oxidase A and serotonin receptor 2A polymorphisms in SSRI antidepressant activity. Int J Neuropsychopharmacol 2002; 5:27–35Crossref, Medline, Google Scholar
109. Blakely RD: Physiological genomics of antidepressant targets: keeping the periphery in mind. J Neurosci 2001; 21:8319–8323Crossref, Medline, Google Scholar
110. Zill P, Baghai TC, Zwanzger P, Schule C, Minov C, Riedel M, Neumeier K, Rupprecht R, Bondy B: Evidence for an association between a G-protein beta3-gene variant with depression and response to antidepressant treatment. Neuroreport 2000; 11:1893–1897Crossref, Medline, Google Scholar
111. Serretti A, Zanardi R, Cusin C, Rossini D, Lilli R, Lorenzi C, Lattuada E, Smeraldi E: No association between dopamine D(2) and D(4) receptor gene variants and antidepressant activity of two selective serotonin reuptake inhibitors. Psychiatry Res 2001; 104:195–203Crossref, Medline, Google Scholar
112. Bymaster FP, Zhang W, Carter PA, Shaw J, Chernet E, Phebus L, Wong DT, Perry KW: Fluoxetine, but not other selective serotonin uptake inhibitors, increases norepinephrine and dopamine extracellular levels in prefrontal cortex. Psychopharmacology (Berl) 2002; 160:353–361Crossref, Medline, Google Scholar
113. Shaldubina A, Agam G, Belmaker RH: The mechanism of lithium action: state of the art, ten years later. Prog Neuropsychopharmacol Biol Psychiatry 2001; 25:855–866Crossref, Medline, Google Scholar
114. Phiel CJ, Klein PS: Molecular targets of lithium action. Annu Rev Pharmacol Toxicol 2001; 41:789–813Crossref, Medline, Google Scholar
115. Steen VM, Lovlie R, Osher Y, Belmaker RH, Berle JO, Gulbrandsen AK: The polymorphic inositol polyphosphate 1-phosphatase gene as a candidate for pharmacogenetic prediction of lithium-responsive manic-depressive illness. Pharmacogenetics 1998; 8:259–268Medline, Google Scholar
116. Serretti A, Lilli R, Lorenzi C, Franchini L, Smeraldi E: Dopamine receptor D3 gene and response to lithium prophylaxis in mood disorders. Int J Neuropsychopharmacol 1998; 1:125–129Crossref, Medline, Google Scholar
117. Serretti A, Lilli R, Lorenzi C, Franchini L, Di Bella D, Catalano M, Smeraldi E: Dopamine receptor D2 and D4 genes, GABA(A) alpha-1 subunit genes and response to lithium prophylaxis in mood disorders. Psychiatry Res 1999; 87:7–19Crossref, Medline, Google Scholar
118. Serretti A, Lorenzi C, Lilli R, Smeraldi E: Serotonin receptor 2A, 2C, 1A genes and response to lithium prophylaxis in mood disorders. J Psychiatr Res 2000; 34:89–98Crossref, Medline, Google Scholar
119. Serretti A, Lilli R, Mandelli L, Lorenzi C, Smeraldi E: Serotonin transporter gene associated with lithium prophylaxis in mood disorders. Pharmacogenomics J 2001; 1:71–77Crossref, Medline, Google Scholar
120. Craddock N, Jones I: Molecular genetics of bipolar disorder. Br J Psychiatry Suppl 2001; 41:S128-S133Google Scholar
121. Mendlewicz J, Verbanck P, Linkowski P, Wilmotte J: Lithium accumulation in erythrocytes of manic-depressive patients: an in vivo twin study. Br J Psychiatry 1978; 133:436–444Crossref, Medline, Google Scholar
122. Grof P, Alda M, Grof E, Zvolsky P, Walsh M: Lithium response and genetics of affective disorders. J Affect Disord 1994; 32:85–95Crossref, Medline, Google Scholar
123. Turecki G, Grof P, Grof E, D’Souza V, Lebuis L, Marineau C, Cavazzoni P, Duffy A, Betard C, Zvolsky P, Robertson C, Brewer C, Hudson TJ, Rouleau GA, Alda M: Mapping susceptibility genes for bipolar disorder: a pharmacogenetic approach based on excellent response to lithium. Mol Psychiatry 2001; 6:570–578Crossref, Medline, Google Scholar
124. Mundo E, Walker M, Cate T, Macciardi F, Kennedy JL: The role of serotonin transporter protein gene in antidepressant-induced mania in bipolar disorder. Arch Gen Psychiatry 2001; 58:539–544Crossref, Medline, Google Scholar
125. Casey DE: Tardive dyskinesia: pathophysiology and animal models. J Clin Psychiatry 2000; 61(suppl 4):5–9Google Scholar
126. Hori H, Ohmori O, Shinkai T, Kojima H, Nakamura J: Association between three functional polymorphisms of dopamine D2 receptor gene and tardive dyskinesia in schizophrenia. Am J Med Genet 2001; 105:774–778Crossref, Medline, Google Scholar
127. Chen CH, Wei FC, Koong FJ, Hsiao KJ: Association of TaqI A polymorphism of dopamine D2 receptor gene and tardive dyskinesia in schizophrenia. Biol Psychiatry 1997; 41:827–829Crossref, Medline, Google Scholar
128. Gaitonde EJ, Morris A, Sivagnanasundaram S, McKenna PJ, Hunt DM, Mollon JD: Assessment of association of D3 dopamine receptor MscI polymorphism with schizophrenia: analysis of symptom ratings, family history, age at onset, and movement disorders. Am J Med Genet 1996; 67:455–458Crossref, Medline, Google Scholar
129. Steen VM, Lovlie R, MacEwan T, McCreadie RG: Dopamine D3-receptor gene variant and susceptibility to tardive dyskinesia in schizophrenic patients. Mol Psychiatry 1997; 2:139–145Crossref, Medline, Google Scholar
130. Inada T, Dobashi I, Sugita T, Inagaki A, Kitao Y, Matsuda G, Kato S, Takano T, Yagi G, Asai M: Search for a susceptibility locus for tardive dyskinesia. Hum Psychopharmacol 1997; 12:35–39Crossref, Google Scholar
131. Basile VS, Masellis M, Badri F, Paterson AD, Meltzer HY, Lieberman JA, Potkin SG, Macciardi F, Kennedy JL: Association of the MscI polymorphism of the dopamine D3 receptor gene with tardive dyskinesia in schizophrenia. Neuropsychopharmacology 1999; 21:17–27Crossref, Medline, Google Scholar
132. Segman R, Neeman T, Heresco-Levy U, Finkel B, Karagichev L, Schlafman M, Dorevitch A, Yakir A, Lerner A, Shelevoy A, Lerer B: Genotypic association between the dopamine D3 receptor and tardive dyskinesia in chronic schizophrenia. Mol Psychiatry 1999; 4:247–253Crossref, Medline, Google Scholar
133. Lovlie R, Daly AK, Blennerhassett R, Ferrier N, Steen VM: Homozygosity for the Gly-9 variant of the dopamine D3 receptor and risk for tardive dyskinesia in schizophrenic patients. Int J Neuropsychopharmacol 2000; 3:61–65Crossref, Medline, Google Scholar
134. Rietschel M, Krauss H, Muller DJ, Schulze TG, Knapp M, Marwinski K, Maroldt AO, Paus S, Grunhage F, Propping P, Maier W, Held T, Nothen MM: Dopamine D3 receptor variant and tardive dyskinesia. Eur Arch Psychiatry Clin Neurosci 2000; 250:31–35Crossref, Medline, Google Scholar
135. Liao DL, Yeh YC, Chen HM, Chen H, Hong CJ, Tsai SJ: Association between the Ser9Gly polymorphism of the dopamine D3 receptor gene and tardive dyskinesia in Chinese schizophrenic patients. Neuropsychobiology 2001; 44:95–98Crossref, Medline, Google Scholar
136. Garcia-Barcelo MM, Lam LC, Ungvari GS, Lam VK, Tang WK: Dopamine D3 receptor gene and tardive dyskinesia in Chinese schizophrenic patients. J Neural Transm 2001; 108:671–677Crossref, Medline, Google Scholar
137. Lerer B, Segman RH, Fangerau H, Daly AK, Basile VS, Cavallaro R, Aschauer HN, McCreadie RG, Ohlraun S, Ferrier N, Masellis M, Verga M, Scharfetter J, Rietschel M, Lovlie R, Levy UH, Meltzer HY, Kennedy JL, Steen VM, Macciardi F: Pharmacogenetics of tardive dyskinesia. Combined analysis of 780 patients supports association with dopamine D3 receptor gene Ser9Gly polymorphism. Neuropsychopharmacology 2002; 27:105–119Crossref, Medline, Google Scholar
138. Lundstrom K, Turpin MP: Proposed schizophrenia-related gene polymorphism: expression of the Ser9Gly mutant human dopamine D3 receptor with the Semliki Forest virus system. Biochem Biophys Res Commun 1996; 225:1068–1072Crossref, Medline, Google Scholar
139. Potkin SG, Kennedy JL, Basile VS: Combining brain imaging and pharmacogenetics in understanding clinical response in Alzheimer’s disease and schizophrenia, in Pharmacogenetics of Psychotropic Drugs. Edited by Lerer B. New York, Cambridge University Press, 2002, pp 391–400Google Scholar
140. Basile VS, Ozdemir V, Masellis M, Walker ML, Meltzer HY, Lieberman JA, Potkin SG, Alva G, Kalow W, Macciardi FM, Kennedy JL: A functional polymorphism of the cytochrome P450 1A2 (CYP1A2) gene: association with tardive dyskinesia in schizophrenia. Mol Psychiatry 2000; 5:410–417Crossref, Medline, Google Scholar
141. Schulze TG, Schumacher J, Muller DJ, Krauss H, Alfter D, Maroldt A, Ahle G, Maroldt AO, Novo Y, Fernandez A, Weber T, Held T, Propping P, Maier W, Nothen MM, Rietschel M: Lack of association between a functional polymorphism of the cytochrome P450 1A2 (CYP1A2) gene and tardive dyskinesia in schizophrenia. Am J Med Genet 2001; 105:498–501Crossref, Medline, Google Scholar
142. Allison DB, Mentore JL, Heo M, Chandler LP, Cappelleri JC, Infante MC, Weiden PJ: Antipsychotic-induced weight gain: a comprehensive research synthesis. Am J Psychiatry 1999; 156:1686–1696Abstract, Google Scholar
143. Leadbetter R, Shutty M, Pavalonis D, Vieweg V, Higgins P, Downs M: Clozapine-induced weight gain: prevalence and clinical relevance. Am J Psychiatry 1992; 149:68–72Link, Google Scholar
144. Umbricht DS, Pollack S, Kane JM: Clozapine and weight gain. J Clin Psychiatry 1994; 55:157–160Medline, Google Scholar
145. Basile VS, Masellis M, McIntyre RS, Meltzer HY, Lieberman JA, Kennedy JL: Genetic dissection of atypical antipsychotic-induced weight gain: novel preliminary data on the pharmacogenetic puzzle. J Clin Psychiatry 2001; 62:45–66Medline, Google Scholar
146. Davis R, Faulds D: Dexfenfluramine: an updated review of its therapeutic use in the management of obesity. Drugs 1996; 52:696–724Crossref, Medline, Google Scholar
147. Yamada J, Sugimoto Y, Yoshikawa T, Noma T, Horisaka K: The effects of peripheral serotonin2 receptor agonist on food intake of rats. Adv Exp Med Biol 1996; 398:555–557Crossref, Medline, Google Scholar
148. Wirshing DA, Wirshing WC, Kysar L, Berisford MA, Goldstein D, Pashdag J, Mintz J, Marder SR: Novel antipsychotics: comparison of weight gain liabilities. J Clin Psychiatry 1999; 60:358–363Crossref, Medline, Google Scholar
149. Reynolds GP, Zhang ZJ, Zhang XB: Association of antipsychotic drug-induced weight gain with a 5-HT2C receptor gene polymorphism. Lancet 2002; 359:2086–2087Crossref, Medline, Google Scholar
150. Yuan X, Yamada K, Ishiyama-Shigemoto S, Koyama W, Nonaka K: Identification of polymorphic loci in the promoter region of the serotonin 5-HT2C receptor gene and their association with obesity and type II diabetes. Diabetologia 2000; 43:373–376Crossref, Medline, Google Scholar
151. Venter JC, Adams MD, Myers EW, Li PW, Mural RJ, Sutton GG, Smith HO, Yandell M, Evans CA, Hold RA: The sequence of the human genome. Science 2001; 291:1304–1315Crossref, Medline, Google Scholar
152. Cargill M, Altshuler D, Ireland J, Sklar P, Ardlie K, Patil N, Shaw N, Lane CR, Lim EP, Kalyanaraman N, Nemesh J, Ziaugra L, Friedland L, Rolfe A, Warrington J, Lipshutz R, Daley GQ, Lander ES: Characterization of single-nucleotide polymorphisms in coding regions of human genes. Nat Genet 1999; 22:231–238Crossref, Medline, Google Scholar
153. Spielman RS, Ewens WJ: The TDT and other family-based tests for linkage disequilibrium and association. Am J Hum Genet 1996; 59:983–989Medline, Google Scholar
154. Brunn TG, Ewald H: Selection bias of susceptibility genes possible when using parent-offspring trios in genetic association studies (letter). Mol Psychiatry 1999; 4:415–416Medline, Google Scholar
155. Risch N, Teng J: The relative power of family-based and case-control designs for linkage disequilibrium studies of complex human diseases, I: DNA pooling. Genome Res 1998; 8:1273–1288Crossref, Medline, Google Scholar
156. Paterson AD: Case-control association studies in complex traits: the end of an era? Mol Psychiatry 1997; 2:277–278Crossref, Medline, Google Scholar
157. Pritchard JK, Rosenberg NA: Use of unlinked genetic markers to detect population stratification in association studies. Am J Hum Genet 1999; 65:220–228Crossref, Medline, Google Scholar
158. Devlin B, Roeder K: Genomic control for association studies. Biometrics 1999; 55:997–1004Crossref, Medline, Google Scholar
159. Xu K, Liu XH, Nagarajan S, Gu XY, Goldman D: Relationship of the delta-opioid receptor gene to heroin abuse in a large Chinese case/control sample. Am J Med Genet 2002; 110:45–50Crossref, Medline, Google Scholar
160. Small KM, Wagoner LE, Levin AM, Kardia SL, Liggett SB: Synergistic polymorphisms of beta1- and alpha2C-adrenergic receptors and the risk of congestive heart failure. N Engl J Med 2002; 347:1135–1142Crossref, Medline, Google Scholar
161. Athanasiou MC, Malhotra AK, Xu C, Stephens JC: Discovery and utilization of haplotypes for pharmacogenetics studies of psychotropic drug response. Psychiatr Genet 2002; 12:89–96Crossref, Medline, Google Scholar
162. Stephens JC, Schneider JA, Tanguay DA, Choi J, Acharya T, Stanley SE, Jiang R, Messer CJ, Chew A, Han JH, Duan J, Carr JL, Lee MS, Koshy B, Kumar AM, Zhang G, Newell WR, Windemuth A, Xu C, Kalbfleisch TS, Shaner SL, Arnold K, Schulz V, Drysdale CM, Nandabalan K, Judson RS, Ruano G, Vovis GF: Haplotype variation and linkage disequilibrium in 313 human genes. Science 2001; 293:489–493Crossref, Medline, Google Scholar
163. Couzin J: Human genome HapMap launched with pledges of $100 million. Science 2002; 298:941–942Medline, Google Scholar