Localizing Gray Matter Deficits in Late-Onset Depression Using Computational Cortical Pattern Matching Methods
Abstract
OBJECTIVE: The authors used magnetic resonance imaging and an image analysis technique known as cortical pattern matching to map cortical gray matter deficits in elderly depressed patients with an illness onset after age 60 (late-onset depression). METHOD: Seventeen patients with late-onset depression (11 women and six men; mean age=75.24, SD=8.52) and 17 group-matched comparison subjects (11 women and six men; mean age=73.88, SD=7.61) were included. Detailed spatial analyses of gray matter were conducted across the entire cortex by measuring local proportions of gray matter at thousands of homologous cortical surface locations in each subject, and these patterns were matched across subjects by using elastic transformations to align sulcal topography. To visualize regional changes, statistical differences were mapped at each cortical surface location in three dimensions. RESULTS: The late-onset depression group exhibited significant gray matter deficits in the right lateral temporal cortex and the right parietal cortex, where decreases were most pronounced in sensorimotor regions. The statistical maps also showed gray matter deficits in the same regions of the left hemisphere that approached significance after permutation testing. No significant group differences were detected in frontal cortices or any other anatomical region. CONCLUSIONS: Regionally specific decreases of gray matter occur in late-onset depression, supporting the hypothesis that this subset of elderly patients with major depression presents with certain unique neuroanatomical abnormalities that may differ from patients with an earlier onset of illness.
A growing body of evidence from structural neuroimaging research suggests that cortical abnormalities are associated with elderly depression, mainly involving the prefrontal cortex (1–10) and temporal lobe structures such as the hippocampus (11–15).
In a subset of elderly patients with major depression, the first episode occurs late in life (16). This subgroup, frequently referred to as having late-onset depression, exhibits certain unique clinical, biological, and neuroimaging characteristics (17–22). More specifically, late-onset depression patients are less likely to have psychiatric comorbidity (23) or a family history of depression (20, 24) when compared with early-onset depression groups. On the other hand, late-onset depression patients are more likely to have associated medical comorbidity (23, 25–28), greater cognitive deficits (29), and increased risk of developing dementia (22). They also more frequently experience a loss of interest and apathy (19, 21).
Findings from structural magnetic resonance imaging (MRI) studies implicate frontal lobe atrophy among patients with late-onset major and minor depression (2, 3). There is also some evidence that patients with late-onset depression may have more pronounced atrophy patterns than subjects with depressive episodes that started early in life. Greater ventricular size (30) and smaller frontal lobe volumes (7) have been reported in late-onset depression when directly compared with elderly patients with early-onset depression. However, a previous report by our group could not detect a significant linear relationship between age at onset of the first episode and neuroanatomical abnormalities (31). One study has shown that among elderly depressed patients, those with older age at onset had smaller hippocampal volume (14).
In addition, high-intensity lesions in subcortical white matter are more common in late-onset depression than in elderly depressed patients with an earlier onset of illness (32–37). Taken together, these observations suggest that late-onset depression may represent a distinct subtype of depression in the elderly (17, 27, 38).
Previous structural neuroimaging studies that focused on late-onset depression have employed traditional volumetric analyses where regional boundaries must be identified. While these studies have provided some evidence for regional specificity of volume loss in late-onset depression, the question of spatial localization of gray matter deficits over the entire cortical surface in late-onset depression has not been addressed in the literature. Such information could provide more comprehensive insights into the neuroanatomical contributions to late-onset depression.
The goal of the present study was to use cortical pattern matching methods in order to perform detailed spatial analyses of gray matter abnormalities on lateral and interhemispheric brain surfaces in subjects with late-onset depression relative to group-matched comparison subjects. These brain mapping methods allow data from each individual to be analyzed with a series of manual and automated procedures that carefully match cortical anatomy across subjects and measure local proportions of gray matter at each of 65,536 anatomically matched points on the cortical surface of each cerebral hemisphere. An advantage of this technique is that subtle as well as spatially diffuse differences in regional gray matter can be identified over the entire cortical surface by closely matching structurally homologous brain regions across subjects. This is made possible through definition of cortical sulcal landmarks on the brain surface of each individual.
We used a similar methodological approach in a previous study of depressed elderly patients with an early onset of illness, which revealed significant brain size reduction in orbitofrontal cortices along with significant gray matter increases adjacent to areas of focal decreases of gray matter in the same anatomical region (9). Results from this previous study also showed significant gray matter increases in parietal cortices as well as the left temporal cortex.
We set out to examine whether regionally specific patterns of gray matter abnormalities are present in late-onset depression patients relative to nondepressed subjects of comparable age and gender, after carefully matching gyral anatomy across subjects. On the basis of the existing literature suggesting that late-onset depression is more likely to be associated with cortical atrophy, and considering our previous findings, we expected that late-onset depression would exhibit gray matter deficit patterns in frontal, temporal, and parietal cortices.
Method
Subjects
Our study group consisted of 17 patients with major depression (11 women and six men; mean age=75.24, SD=8.52) and 17 nondepressed comparison subjects (11 women and six men; mean age=73.88, SD=7.61) (Table 1). All patients were assessed with the Structured Clinical Interview for DSM-IV (SCID) and met DSM-IV criteria for major depressive disorder. Late-onset depression was defined as onset of the first major depressive episode after age 60. All depressed subjects scored 15 or greater (mean score=18.41, SD=4.26) on the 17-item Hamilton Depression Rating Scale (39). The mean age at onset of depression was 71.88 (SD=7.61). Information on prior depressive episodes and age at onset was obtained from patients and caregivers and corroborated, whenever necessary, with information from the spouse or a close relative. All patients were free of psychotropic medications for at least 2 weeks before imaging. None of the patients had histories of long-term antidepressant treatment or other prolonged psychotropic medications. Patients underwent a thorough medical examination and laboratory testing. Exclusion criteria were 1) another major psychiatric illness, such as bipolar disorder, schizophrenia, or schizoaffective disorder; 2) active alcohol or drug dependence; 3) primary neurologic illness, such as dementia, stroke, Parkinson’s disease, seizure disorder, or multiple sclerosis; 4) presence of a medical illness or medication use that could affect cognitive function; 5) physical disability that precluded cognitive testing; and 6) metal in the body that precluded MRI scanning. A psychiatric examination and a structured interview (SCID) for normal subjects were administered to all comparison subjects to rule out current or past psychopathology. Exclusionary conditions for comparison subjects were clinical evidence of dementia, suspected dementia, or any other brain disorder determined from history or mental status examination. None of the patients and comparison subjects had axis II disorders. All subjects had Mini-Mental State Examination (40) scores ≥24 (depressed patients: mean=28.53, SD=1.33; comparison group: mean=29.59, SD=0.71). Several of the depressed patients and comparison subjects had stable comorbid medical disorders such as hypertension, ischemic heart disease, or arthritis.
Patients were recruited through community outreach efforts that included local newspaper and radio advertisements and through referrals from the geriatric psychiatry ambulatory care programs at the UCLA Medical Center. Comparison subjects were recruited from the community through newspaper and radio advertisements. The study was performed in accordance with the policies of the UCLA Human Subject Protection Committees, and written informed consent was obtained from all subjects after the procedures had been fully explained.
Imaging
Subjects were scanned with a 1.5-T Signa magnet (GE Medical Systems, Milwaukee). The imaging protocol was a whole-brain, gradient-echo (spoiled gradient recall acquisition) T1-weighted series acquired coronally with section thickness of 1.4 mm, no gaps (repetition time=20 msec; echo time=6 msec; flip angle=45°; field of view=22 cm; number of excitations=1.5; matrix size=256×192 mm; in-plane resolution=0.86×0.86 mm). Quantification of white matter lesions was not assessed in this study.
Image Processing
1. | We have described the methods for image analysis in detail elsewhere (9, 41, 42), and will briefly summarize them here. | ||||
2. | We performed automatic whole brain extraction and interhemispheric brain extraction with removal of nonbrain tissue (43). Each brain slice was also manually edited such that only brain tissue and CSF remained in each image volume. Reliability of manual scalp editing was determined by calculating the intraclass correlation coefficients for brain volumes from 10 test brains that were randomly mixed in among a larger data set (42). Intra- and interrater reliability achieved in our laboratory were both >0.99. | ||||
3. | Each scan was corrected for signal intensity inhomogeneities. A radiofrequency bias field correction algorithm eliminated intensity drifts attributable to scanner field inhomogeneity, using a histogram spline sharpening method (44). | ||||
4. | Fully automated tissue classification used a partial volume correction method (45) to automatically classify voxels as most representative of gray matter, white matter, and CSF. | ||||
5. | Brain volumes were transformed into standard International Consortium for Brain Mapping-305 space using a 12-parameter linear, automated image registration algorithm (46). | ||||
6. | The cortical surface for each MR volume was extracted by using automated software (47, 48). | ||||
7. | As seen in Figure 1, we traced 17 sulcal and gyral landmarks on the lateral surface and 12 sulci and gyral landmarks on the interhemispheric surface of each hemisphere employing previously validated anatomic delineation protocols (41, 42, 49). In addition to contouring the major sulci, a set of six midline landmark curves bordering the longitudinal fissure were outlined on each hemisphere. Interrater variability of manual outlining was measured as the three-dimensional root mean square difference in millimeters between 100 equidistant points from each sulcal landmark traced in six test brains (H.D.) relative to a gold standard arrived at by a consensus of raters as previously reported (42, 49) (http://www.loni.ucla.edu/~esowell/new_sulcvar.html). Intrarater reliability was computed by comparing the three-dimensional root mean square distance between equidistant surface points from sulcal landmarks from one test brain traced six times by the same rater (H.D.). Three-dimensional root mean square disparities were <2 mm, and on average <1 mm, between points for all landmarks within and between raters. | ||||
8. | Cortical pattern matching used the sulcal/gyral landmarks and the cortical surface models from each subject to compute a three-dimensional vector deformation field, which reconfigures each subject’s anatomy into the average pattern of a given group by matching equivalent landmark points in x, y, and z coordinates (41, 50, 51). | ||||
9. | Gray matter proportion measurements used the three-dimensional deformation vector fields obtained from the cortical pattern matching methods to allow a local measurement of gray matter to be made at equivalent three-dimensional cortical surface locations in each subject, referencing corresponding point locations in spatially registered tissue classified scalp-edited brain volumes. Cortical gray matter was quantified by measuring the proportion (or density) of voxels segmenting as gray matter within a sphere with a fixed radius of 15 mm at homologous cortical surface points in each individual. Therefore, at each point on the cortical surface, a local measurement of gray matter is made that may be averaged and compared statistically to provide maps indexing very local differences in tissue proportion within and between groups (9, 41, 42, 49). |
Although patients and comparison subjects were group-matched for age and gender, all gray matter proportion analyses were performed in International Consortium for Brain Mapping-305 space to account for interindividual differences in overall brain size that might be related to demographic variables.
Statistical Analyses
For statistical comparisons, Student’s t tests were conducted at each cortical surface location in three dimensions (65,536 surface points) providing spatially detailed maps of gray matter proportion differences between diagnostic groups. In all statistical maps, a surface point significance threshold of p<0.05 (two-tailed) was used to visualize the regional specificity of gray matter changes in the cortex.
To correct for multiple comparisons (i.e., statistical tests at each of 65,536 surface points), subjects were randomly assigned to groups of 10,000 new randomized analyses (at each surface point), and the number of significant results (i.e., gray matter proportion at any surface point that significantly differed between groups at the threshold of p<0.05) that occurred in the real group difference test was compared with the null distribution of significant results that occurred by chance in the permutation analyses. In other words, the threshold for assessing significance of statistical maps based on permutation tests was determined objectively by calculating the surface area (number of surface points) of significant effects in the real group difference test. That surface area within any tested region of interest was used as the threshold for comparison with the random tests for that region of interest, and if <5% (i.e., p<0.05) of the results from random tests reached or exceeded the surface area of the real test, the statistical map (within regions of interest) was considered significant (9, 41, 49). The regions of interest used in the permutation analyses were chosen to test our a priori hypothesis that significant group differences would be observed in frontal, temporal, and parietal cortices. Gross frontal lobe, parietal lobe, temporal lobe, and occipital lobe regions of interest were therefore created for each individual from a probabilistic atlas (52).
Finally, t tests were used to examine differences between groups for total gray matter, white matter, and CSF volumes as well as total brain volumes.
Results
Statistical maps show significant differences in gray matter proportions (in International Consortium for Brain Mapping-305 space) at each point on the cortex between diagnostic groups (Figure 2). Probability values from the t tests, indexed in color, are mapped onto the group-averaged cortical surface model at each three-dimensional location. The areas in red reflect significant negative effects (gray matter proportion decreases) at a threshold of p<0.05 (two-tailed). Of note, the p maps reflect two-tailed probability values of signed t values representing negative effects only, since no significant gray matter increases were observed in late-onset depression throughout the cortical surface. The magnitude of differences in gray matter is also shown in percentage (Figure 3), again according to a color scale. In general, a difference greater than approximately –10% corresponded with significant negative effects of depression on gray matter exceeding the threshold of p<0.05.
In late-onset depression subjects, regional gray matter deficits, mapped at each cortical surface point in three dimensions, appeared spatially diffuse in the right temporal cortex (posterior portions of the superior and inferior temporal gyrus) (Figure 2), where decreases exceeded –10% (Figure 3). Focal patterns of gray matter deficits also appeared in posterior aspects of the left lateral temporal cortex. Significant decreases in right temporal gray matter were confirmed by permutation analyses (Table 2).
Gray matter loss in late-onset depression was also observed in parietal cortices, mainly sensorimotor regions, with most prominent deficits again occurring in the right hemisphere (with 10%–20% loss) (Figure 2 and Figure 3). Permutation analyses (Table 2) showed that the regional patterns of gray matter loss were significant in the right parietal cortex.
No other main effects were detected in any other anatomical region, including the frontal cortex. No significant differences between late-onset depression and comparison subjects were observed for total gray matter, white matter, CSF volumes, or total brain volume (Table 1).
Discussion
Two main positive findings emerged from our study: 1) significant gray matter deficits, confirmed with permutation testing, in the right lateral temporal cortex, mainly the posterior portions of the superior and inferior temporal gyrus, and 2) prominent decreases of gray matter in the right parietal cortex, where deficits were most pronounced in sensorimotor regions, again confirmed with permutation tests. In the same regions, similar patterns of gray matter deficits also appeared in the left hemisphere that approached significance with permutation testing.
Several structural MRI studies have demonstrated hippocampal or temporal lobe abnormalities in late-life depression (11–14), whereas others did not observe significant differences (1, 3, 53). Of all these studies, two focused on patients with first onset of depression after the age of 60 (3, 13), with one showing significantly more left medial temporal atrophy than early-onset patients (13), and the other showing differences in total temporal lobe volume that approached significance between patients with late-onset minor or major depression and comparison subjects (3). Manual delineation of the entire temporal lobe is difficult because the orientation and anatomic boundaries can be unclear. This may at least in part explain why in our previous study decreases in total temporal volume only approached statistical significance and why most region of interest studies examined specific temporal subregions such as the hippocampus, which can be more easily identified. A study by Steffens et al. (14) suggested that hippocampal volume atrophy may be greater in late-onset depression compared with early-onset depression. It is interesting that the anatomical regions within the temporal lobe where we saw the most pronounced gray matter deficits were the posterior aspects of the superior and inferior temporal gyrus, without any differences in ventral/medial areas. Although our study supports the hypothesis that temporal lobe atrophy is associated with late-onset depression, further studies are clearly warranted to examine which specific temporal subregions are most relevant in the context of late-onset depression.
In addition to our findings of gray matter decrease in lateral temporal regions, the present study is also the first to report gray matter deficits in parietal cortices, mainly sensorimotor regions, in late-onset depression. Lesions in the right temporal or parietal cortices have been shown to impair emotional experience and arousal (54–56) and to impair imagery for emotion (57–60). In addition, patients with tumors affecting temporoparietal cortices have reported pronounced negative mood states after surgery (61). There is evidence that both temporal and parietal cortices contribute to integrating viscerosensory information with affective signals (62, 63). Functional imaging studies in major depression have documented changes in regional cerebral flow or metabolism in the temporal cortex (64–66) as well as in the parietal cortex (67, 68). In geriatric depression, reduced superior temporal and anterior parietal activations have been reported (69).
Using cortical pattern matching methods, we also detected gray matter abnormalities in temporoparietal cortices in our previous report (9) that focused on elderly depressed patients with an early age at onset. However, widespread increases of gray matter instead of decreases were found in these regions. Furthermore, the distribution of gray matter changes in temporal lobe structures differed somehow from the pattern identified in late-onset depression, since gray matter increases were more pronounced in the left hemisphere and observed throughout the lateral surface, including the temporal poles, rather than being more right-lateralized and localized in more dorsal lateral aspects, as observed in late-onset depression.
Whereas gray matter increases may reflect abnormal neurodevelopmental processes, perhaps because of an excess of gray matter early in development that decreases over time yet remains above the normal threshold, the reduction of gray matter in late-onset depression is more likely to be associated with neurodegenerative events. It remains to be clarified whether the region-specific gray matter deficits observed here in late-onset depression are necessary for initiation of the illness or instead represent an epiphenomenon that perhaps appears with progressive accumulation of the number of stressors in later life (i.e., neuronal loss due to the aging process, concomitant medical illness, and grief).
Contrary to our predictions regarding frontal gray matter deficits in late-onset depression, no significant group differences were found throughout this anatomical region. Several previous MRI volumetric studies that implicated structural abnormalities of the frontal lobe, particularly the orbitofrontal cortex, in geriatric depression (1, 5, 8, 70) mainly included patients with long histories of depression, and it is not clear what proportion of their samples had late-onset depression. Of the three previous MRI studies focused on late-onset depression (defined as illness onset after age 60), all examined frontal lobe atrophy using traditional volumetric methods, although results remain mixed (3, 7, 31). Indeed, in an earlier report by our group (3), late-onset depression was associated with greater bilateral frontal atrophy relative to that of nondepressed comparison subjects. However, in a subsequent study (31) we reported that lobar structural abnormalities in the frontal regions did not change with an increasing age at onset of illness. Instead, Almeida et al. (7) found that patients with late-onset depression had significantly more right frontal atrophy than their early-onset counterparts of similar age, without observing significant differences between late-onset depression and nondepressed comparison subjects. However, our results are not fully comparable with previous region of interest studies in late-onset depression, given that these studies examined frontal lobe volumes as a whole and did not specifically address gray matter differences. It is therefore possible that subcortical abnormalities, such as white matter lesions, may have contributed to the evidence of atrophy in total frontal volume in previous reports. For example, one study showed that subcortical lesions may decrease orbitofrontal cortex volume in geriatric depression (70). Although white matter lesions were not specifically addressed in the present study, global white matter volumes did not differ between groups (Table 1). Future studies are needed to understand how subcortical lesions may be related to cortical gray matter changes. Indeed, based on the results of an earlier report (71), comorbid medical disorders may have only a modest impact on brain volume reductions. The differences in the results between our earlier region of interest study in late-onset depression (3) and the present report may additionally arise from differences in the demographic or clinical characteristics of the populations of depressed patients examined. While our earlier study included inpatients, the patients in the study reported here were exclusively outpatients and had an average of less than one prior major depressive episode.
Regarding frontal cortices, it is noteworthy that the results in late-onset depression differ from the pattern identified among elderly patients with early-onset depression in previous research that used computational cortical pattern matching (9). More specifically, the latter study showed a prominent regional size reduction in the orbitofrontal cortex, together with significant increases in cortical gray matter adjacent to focal decreases of gray matter in the same region. In late-onset depression, we expected to find gray matter loss rather than gray matter increase in frontal regions because this subtype of depression is thought to be associated with greater cortical atrophy than early-onset groups (22).
Although the division between “early” and “late” onset is still considered somewhat arbitrary and not based on reliable clinical and biological data, it remains possible that the patients in the study reported here may not show discernible frontal gray matter deficits because such abnormalities could require more prolonged or intense exposure to potentially neurotoxic events. Whether and to what extent disease severity or longer total history of depression might contribute to frontal abnormalities is yet to be unraveled. On the other hand, there is evidence that the effects of hypercortisolemia in major depression exhibit regional specificity in cortical regions, with intensity of these effects differing according to anatomical site (72). Temporal and parietal cortices of late-onset depression may therefore be, for some reason, more sensitive to the potentially toxic effects of such insults.
There are now a number of studies supporting the view that late-onset depression patients are more likely to have associated cognitive impairment and are more likely to progress to dementia (22). None of our patients had a history of mental status examination results suggesting dementia, although it is possible that some of them will develop progressive cognitive impairment over time (73). Nevertheless, it is noteworthy that the gray matter changes observed here do not show the same patterns as the changes seen in patients with Alzheimer’s disease. Indeed, previously published longitudinal Alzheimer’s disease studies using cortical pattern matching or voxel-based morphometry demonstrated that gray matter loss appears in ventral/medial regions of the temporal cortex in the earliest stage of the illness (41, 74), whereas here no group differences were detected in these regions. Furthermore, sensorimotor regions are relatively spared in Alzheimer’s disease (41, 51), whereas these are the regions within parietal cortices where the gray matter deficit was most pronounced in late-onset depression. This may suggest that the gray matter abnormalities reported here might be indeed disease specific.
Limitations of this study should be noted. The study groups were relatively small, a consideration that suggests caution when interpreting results. That is, it is possible that we did not have sufficient power to detect subtle differences in gray matter that may exist in other cortical regions in late-onset depression. Notwithstanding, since this study is, to our knowledge, the first to identify specific gray matter deficits in temporal and parietal cortices in late-onset depression, replication with a large homogenous group of subjects may serve to confirm the findings reported here. In addition, approximately two-thirds of the subjects were women. We corrected for potential interindividual differences in brain size that might be associated with gender by performing our analyses in International Consortium for Brain Mapping-305 space. However, future studies may be needed to investigate the specific contribution of gender to gray matter abnormalities in late-onset depression. Information on handedness was not available. Future research may further investigate laterality effects, given that larger gray matter differences were observed in the right hemisphere. Our findings in the present context, however, may reflect that power is lacking and not necessarily that laterality effects are present.
Measurements of gray matter obtained after cortical pattern matching algorithms are constrained by size of the sphere used to sample local gray matter proportions. In this study, we chose a 15-mm kernel size to increase signal to noise for local measurements of gray matter (41, 42, 49). Previous studies demonstrated that tissue proportion measurements using this kernel size are comparable to those found with manual delineation procedures. Indeed, Sowell et al. (75) documented that measures of gray matter concentration and gray matter volume are similar when the manually delineated region of interest is small and at the same cortical surface location.
In conclusion, the sensitive cortical pattern methods employed in this study were able to detect prominent local gray matter deficits in the right lateral temporal cortex and parietal cortex, most pronounced in sensorimotor areas, in subjects with late-onset depression relative to nondepressed comparison subjects. These novel findings imply specific neurodegenerative processes in late-onset depression that differ from the pattern commonly seen in dementia. Only longitudinal follow-up evaluations, however, will be able to make this distinction, with important implications from both therapeutic and research standpoints.
![]() |
![]() |
Received Nov. 17, 2003; revision received Feb. 23, 2004; accepted March 4, 2004. From the Laboratory of Neuro Imaging, Department of Neurology, Neuropsychiatric Institute, David Geffen UCLA School of Medicine, Los Angeles; Program in Neuroscience, Brescia University School of Medicine, Brescia, Italy; and Kliniken Schmieder Konstanz, Psychotherapy Unit, Constance, Germany. Address correspondence and reprint requests to Dr. Toga, Rm. 4238, Reed Neurological Research Center, Laboratory of Neuro Imaging, Department of Neurology, David Geffen UCLA School of Medicine, 710 Westwood Plaza, Los Angeles, CA 90095–1769; [email protected] (e-mail). Supported by research grants from NIMH (MH-61567, MH-63764, and KO2 MH-02043) to Dr. Kumar; grants from the National Library of Medicine and National Institute on Aging (R01 LM-05639); and resource grants from the National Center for Research Resources (P41 RR-013642 and M01 RR-000865) to Dr. Toga. Algorithm development was partially supported by exploratory/developmental research grant awards from the National Center for Research Resources (RR-19771) and National Institute of Biomedical Imaging and Bioengineering (EB-01561) to Dr. Thompson. Additional support was provided by the National Institute of Biomedical Imaging and Bioengineering, National Institute of Neurological Disorders and Stroke, and an NIMH grant (P01 EB-001955) to Dr. Toga.
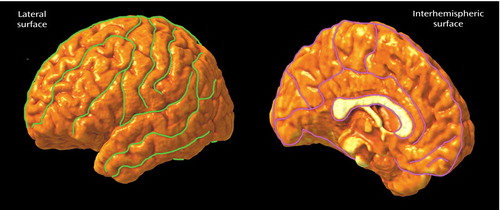
Figure 1. Sulcal and Gyral Landmarks Traced on the Lateral and Interhemispheric Surfaces of Cortical Surface Models From Elderly Patients With Late-Onset Depression (N=17) and a Matched Group of Nondepressed Comparison Subjects (N=17)
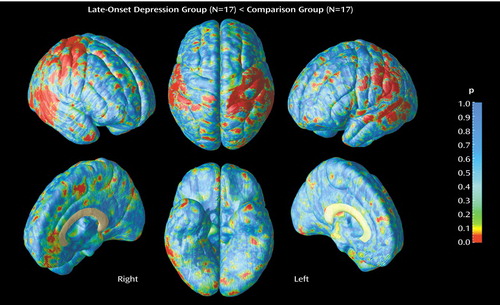
Figure 2. Gray Matter Differences Between Elderly Patients With Late-Onset Depression (N=17) and a Matched Group of Nondepressed Comparison Subjects (N=17)a
aRight-side lateral and interhemispheric, top and bottom, and left-side lateral and interhemispheric views are shown. Regions illustrated in red correspond to significant deficits in the patient group at a threshold of p<0.05 (two-tailed).
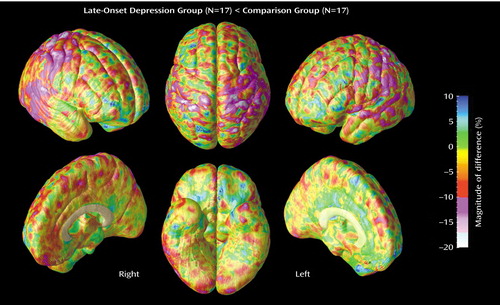
Figure 3. Magnitude of Gray Matter Differences Between Elderly Patients With Late-Onset Depression (N=17) and a Matched Group of Nondepressed Comparison Subjects (N=17)a
aRight-side lateral and interhemispheric, top and bottom, and left-side lateral and interhemispheric views are shown. Differences greater than approximately –10% correspond with the threshold significance level (p<0.05).
1. Coffey CE, Wilkinson WE, Weiner RD, Parashos IA, Djang WT, Webb MC, Figiel GS, Spritzer CE: Quantitative cerebral anatomy in depression: a controlled magnetic resonance imaging study. Arch Gen Psychiatry 1993; 50:7–16Crossref, Medline, Google Scholar
2. Kumar A, Schweizer E, Jin Z, Miller D, Bilker W, Swan LL, Gottlieb G: Neuroanatomical substrates of late-life minor depression: a quantitative magnetic resonance imaging study. Arch Neurol 1997; 54:613–617Crossref, Medline, Google Scholar
3. Kumar A, Zin Z, Bilker W, Udupa J, Gottlieb G: Late onset minor and major depression: early evidence for common neuroanatomical substrates detected by using MRI. Proc Natl Acad Sci USA 1998; 95:7654–7658Crossref, Medline, Google Scholar
4. Kumar A, Thomas A, Lavretsky H, Yue K, Huda A, Curran J, Venkatraman T, Estanol L, Mintz J, Mega M, Toga A: Frontal white matter biochemical abnormalities in late-life major depression detected with proton magnetic resonance spectroscopy. Am J Psychiatry 2002; 159:630–636Link, Google Scholar
5. Lai TJ, Payne ME, Byrum CE, Steffens DC, Krishnan KRR: Reduction of orbital frontal cortex volume in geriatric depression. Biol Psychiatry 2000; 48:971–975Crossref, Medline, Google Scholar
6. MacFall JR, Payne ME, Provenzale JE, Krishnan KRR: Medial orbital frontal lesions in late-onset depression. Biol Psychiatry 2001; 49:803–806Crossref, Medline, Google Scholar
7. Almeida OP, Burton EJ, Ferrier N, McKeith IG; O’Brien JT: Depression with late onset is associated with right frontal atrophy. Psychol Med 2002; 33:675–681Crossref, Google Scholar
8. Taylor WD, Steffens DC, McQuoid DR, Payne ME, Lee SH, Lai TJ, Krishnan KRR: Smaller orbital frontal cortex volumes associated with functional disability in depressed elders. Biol Psychiatry 2003; 53:144–149Crossref, Medline, Google Scholar
9. Ballmaier M, Sowell ER, Thompson PM, Kumar A, Narr KL, Lavretsky H, Welcome SE, DeLuca H, Toga AW: Mapping brain size and cortical gray matter changes in elderly depression. Biol Psychiatry 2004; 55:382–389Crossref, Medline, Google Scholar
10. Ballmaier M, Toga AW, Blanton RE, Sowell ER, Lavretsky H, Peterson J, Pham D, Kumar A: Anterior cingulate, gyrus rectus, and orbitofrontal abnormalities in elderly depressed patients: an MRI-based parcellation of the prefrontal cortex. Am J Psychiatry 2004; 161:99–108Link, Google Scholar
11. Rabins PV, Pearlson GD, Aylward E, Kumar AJ, Dowell K: Cortical magnetic resonance imaging changes in elderly inpatients with major depression. Am J Psychiatry 1991; 148:617–620Link, Google Scholar
12. O’Brien JT, Desmond P, Ames D, Schweitzer I, Tuckwell V, Tress B: The differentiation of depression from dementia by temporal lobe magnetic resonance imaging. Psychol Med 1994; 24:633–640Crossref, Medline, Google Scholar
13. Greenwald BS, Kramer-Ginsberg E, Bogerts B, Ashtaru M, Aupperle PM, Wu H, Allen L, Zeman D, Patel M: Qualitative magnetic resonance imaging findings in geriatric depression: possible link between later-onset depression and Alzheimer’s disease? Psychol Med 1997; 27:421–431Crossref, Medline, Google Scholar
14. Steffens DC, Byrum CE, McQuoid DR, Greenberg DL, Payne ME, Blitchington TF, MacFall JR, Krishnan KRR: Hippocampal volume in geriatric depression. Biol Psychiatry 2000; 48:301–309Crossref, Medline, Google Scholar
15. Bell-McGinty S, Butters MA, Meltzer CC, Greer PJ, Reynolds CF III, Becker JT: Brain morphometric abnormalities in geriatric depression: long-term neurobiological effects of illness duration. Am J Psychiatry 2002; 159:1424–1427Link, Google Scholar
16. Alexopoulos GS: Clinical and biological findings in late-onset depression, in American Psychiatric Press Review of Psychiatry. Edited by Tasman A, Goldfinger SM, Kaufmann CA. Washington, DC, American Psychiatric Press, 1990, pp 249–262Google Scholar
17. Alexopoulos GS, Young RC, Meyers BS, Abrams R, Shamoian CA: Late-onset depression. Psychiatr Clin North Am 1988; 11:101–115Crossref, Medline, Google Scholar
18. Burvill PW, Hall WD, Stampfer HG, Emmerson JP: A comparison of early-onset and late-onset depressive illness in the elderly. Br J Psychiatry 1989; 155:673–679Crossref, Medline, Google Scholar
19. Krishnan KRR, Hays JC, Tupler LA, George LK, Blazer DG: Clinical and phenomenological comparisons of late-onset and early-onset depression. Am J Psychiatry 1995; 152:785–788Link, Google Scholar
20. Krishnan KRR, Hays JC, Blazer DG: MRI-defined vascular depression. Am J Psychiatry 1997; 154:497–501Link, Google Scholar
21. Heun R, Kockler M, Papassotiropoulos A: Distinction of early- and late-onset depression in the elderly by their lifetime symptomatology. Int J Geriatr Psychiatry 2000; 15:1138–1142Crossref, Medline, Google Scholar
22. Schweitzer I, Tickwell V, O’Brien J, Ames D: Is late onset depression a prodrome of dementia? Int J Geriatr Psychiatry 2002; 17:997–1005Crossref, Medline, Google Scholar
23. Lyness JM, Pearson JL, Lebowitz BD, Kupfer DJ: Age at onset of late-life depression. Am J Geriatr Psychiatry 1994; 2:4–8Crossref, Google Scholar
24. Brown RP, Sweeney J, Loutsch E, Kocsis J, Frances A: Involutional melancholia revisited. Am J Psychiatry 1984; 141:24–28Link, Google Scholar
25. Emery VO, Oxman TE: Update on the dementia spectrum of depression. Am J Psychiatry 1992; 149:305–317Link, Google Scholar
26. Alexopoulos GS, Meyers B, Young RC, Campbell S, Sibersweig D, Charlson M: “Vascular depression” hypothesis. Arch Gen Psychiatry 1997; 54:915–922Crossref, Medline, Google Scholar
27. Krishnan KRR: Biological risk factors in late life depression. Biol Psychiatry 2002; 52:185–192Crossref, Medline, Google Scholar
28. Sheline YI: Neuroimaging studies of mood disorder: effects on the brain. Biol Psychiatry 2003; 54:338–352Crossref, Medline, Google Scholar
29. Salloway S, Malloy P, Kohn R, Gillard E, Duffy J, Dowell K: MRI and neuropsychological differences in early- and late-onset depression. Neurology 1996; 46:1567–1574Crossref, Medline, Google Scholar
30. Alexopoulos GS, Young RC, Shindledecker RD: Brain computed tomography findings in geriatric depression and primary degenerative dementia. Biol Psychiatry 1992; 31:591–599Crossref, Medline, Google Scholar
31. Kumar A, Bilker W, Jin Z, Udupa J, Gottlieb G: Age of onset of depression and quantitative neuroanatomic measures: absence of specific correlates. Psychiatry Res 1999; 31:101–110Crossref, Google Scholar
32. Figiel GS, Krishnan KRR, Doraiswamy MP, Rao VP, Nemeroff CB, Boyko BO: Subcortical hyperintensities on brain magnetic resonance imaging: a comparison between late age onset and early onset elderly depressed subjects. Neurobiol Aging 1991; 26:245–247Crossref, Google Scholar
33. Lesser IM, Mena I, Boone KB, Miller BL, Mehringer CM, Wohl M: Reduction of cerebral blood flow in older depressed patients. Arch Gen Psychiatry 1994; 51:677–686Crossref, Medline, Google Scholar
34. Lesser IM, Boone KB, Mehringer CM, Wohl MA, Miller BL, Berman NG: Cognition and white matter hyperintensities in older depressed patients. Am J Psychiatry 1996; 153:1280–1287Link, Google Scholar
35. O’Brien J, Desmond P, Ames D, Schweitzer I, Harrigan S, Tress B: A magnetic resonance imaging study of white matter lesions in depression and Alzheimer’s disease. Br J Psychiatry 1996; 168:477–485Crossref, Medline, Google Scholar
36. deGroot JC, de Leeuw FE, Oudkerk M, Hofman A, Jolles J, Breteler MM: Cerebral white matter lesions and depressive symptoms in elderly adults. Arch Gen Psychiatry 2000; 57:1071–1076Crossref, Medline, Google Scholar
37. Salloway S, Correia S, Boyle P, Malloy P, Schneider L, Lavretsky H, Sackeim HA, Roose S, Krishnan KRR: MRI subcortical hyperintensities in old and very old depressed outpatients: the important role of age in late-life depression. J Neurol Sci 2002; 203/204:227–233Google Scholar
38. Grace J, O’Brien JT: Association of life events and psychosocial factors with early but not late onset depression in the elderly. Int J Geriatr Psychiatry 2003; 18:473–478Crossref, Medline, Google Scholar
39. Hamilton M: Development of a rating scale for primary depressive illness. Br J Soc Clin Psychol 1967; 6:278–296Crossref, Medline, Google Scholar
40. Folstein MF, Folstein SE, McHugh PR: “Mini-Mental State”: a practical method for grading the cognitive state of patients for the clinician. J Psychiatr Res 1975; 12:189–198Crossref, Medline, Google Scholar
41. Thompson PM, Hayashi KM, de Zubicaray G, Janke AL, Rose SE, Semple J, Herman D, Hong MS, Dittmer SS, Doddrell DM, Toga AW: Dynamics of gray matter loss in Alzheimer’s disease. J Neurosci 2003; 23:994–1005Crossref, Medline, Google Scholar
42. Narr KL, Sharma T, Woods RP, Thompson PM, Sowell ER, Rex D, Kim S, Asuncion D, Jang S, Mazziotta J, Toga AW: Increases in regional subarachnoid CSF without apparent cortical gray matter deficits in schizophrenia: modulating effects of sex and age. Am J Psychiatry 2003; 160:2169–2180Link, Google Scholar
43. Shattuck DW, Leahy RM: BrainSuite: an automated cortical surface identification tool. Med Image Anal 2002; 6:129–142Crossref, Medline, Google Scholar
44. Sled JG, Zijdenbos AP, Evans AC: A non-parametric method for automatic correction of intensity non-uniformity in MRI data. IEEE Trans Med Imaging 1998; 17:87–97Crossref, Medline, Google Scholar
45. Shattuck DW, Sandor-Leahy SR, Schaper KA, Rottenberg DA, Leahy RM: Magnetic resonance image tissue classification using a partial volume model. Neuroimage 2001; 13:856–876Crossref, Medline, Google Scholar
46. Woods RP, Grafton ST, Watson JD, Sicotte NL, Mazziotta JC: Automated image registration, II: intersubject validation of linear and nonlinear models. J Comput Assist Tomogr 1998; 22:153–165Crossref, Medline, Google Scholar
47. MacDonald D, Avis D, Evans A: Multiple surface identification and matching in magnetic resonance images, in Proceedings of the SPIE Conference on Visualization in Biomedical Computing. Edited by Robb RA. Rochester, Minn, International Society for Optical Engineering, 1994, pp 160–169Google Scholar
48. Mazziotta JC, Toga AW, Evans A, Fox P, Lancaster J (International Consortium for Brain Mapping [ICBM]): A probabilistic atlas of the human brain: theory and rationale for its development. Neuroimage 1995; 2:89–101Crossref, Medline, Google Scholar
49. Sowell ER, Peterson BS, Thompson PM, Welcome SE, Henkenius AL, Toga AW: Mapping cortical changes across the human life span. Nat Neurosci 2003; 6:309–315Crossref, Medline, Google Scholar
50. Thompson PM, Woods RP, Mega MS, Toga AW: Mathematical/computational challenges in creating deformable and probabilistic atlases of the human brain. Hum Brain Mapp 2000; 9:81–92Crossref, Medline, Google Scholar
51. Thompson PM, Mega MS, Woods RP, Zoumalan CI, Lindshield CJ, Blanton RE, Moussai J, Holmes CJ, Cummings JL, Toga AW: Cortical change in Alzheimer’s disease detected with a disease-specific population-based brain atlas. Cereb Cortex 2001; 11:1–16Crossref, Medline, Google Scholar
52. Evans AC, Kamber M, Collins DL, MacDonald D: An MRI-based probabilistic atlas of the neuroanatomy, in Magnetic Resonance Scanning and Epilepsy. Edited by Shorvon SD, Fish DR, Andermann F, Bydder GM. Dordrecht, Netherlands, Kluwer Academic, 1994, pp 263–274Google Scholar
53. Pantel J, Schröder J, Essig M, Popp D, Dech H, Knopp MV, Schad LR, Eysenbach K, Backenstraß M, Friedlinger M: Qualitative magnetic resonance imaging in geriatric depression and primary degenerative dementia. J Affect Disord 1997; 42:221–229Crossref, Google Scholar
54. Heilman KM, Watson RT: Arousal and emotions, in Handbook of Neuropsychology, vol 3. Edited by Boller F, Grafman J. Amsterdam, Elsevier, 1989, pp 403–417Google Scholar
55. Heller W: Neuropsychological mechanisms of individual differences in emotion, personality, and arousal. Neuropsychology 1993; 7:476–489Crossref, Google Scholar
56. Meadows ME, Kaplan RF: Dissociation of autonomic and subjective responses to emotional slides in right hemisphere damaged patients. Neuropsychologia 1994; 32:847–856Crossref, Medline, Google Scholar
57. Blonder LX, Bowers D, Heilman K: The role of the right hemisphere in emotional communication. Brain 1991; 114:1115–1127Crossref, Medline, Google Scholar
58. Bowers D, Blonder LX, Feinberg T, Heilman KM: Differential impact of right and left hemisphere lesions on facial emotion and object imagery. Brain 1987; 114:2593–2609Crossref, Google Scholar
59. Adolphs R, Damasio H, Tranel D, Damasio AR: Cortical systems in the recognition of emotion in facial expression. J Neurosci 1996; 16:7678–7687Crossref, Medline, Google Scholar
60. Adolphs R, Damasio H, Tranel D, Cooper G, Damasio AR: A role for somatosensory cortices in the visual recognition of emotion as revealed by three-dimensional lesion mapping. J Neurosci 2000; 20:2683–2690Crossref, Medline, Google Scholar
61. Irle E, Peper M, Wowra B, Kunze S: Mood changes after surgery for tumors of the cerebral cortex. Arch Neurol 1994; 51:164–174Crossref, Medline, Google Scholar
62. Morecraft RJ, Geula C, Mesulam MM: Cytoarchitecture and neural afferents of OFC in the brain of the monkey. J Comp Neurol 1992; 323:341–358Crossref, Medline, Google Scholar
63. Price J: Prefrontal cortical networks related to visceral function and mood. Ann NY Acad Sci 1999; 877:383–396Crossref, Medline, Google Scholar
64. Yazici KM, Kapucu O, Erbas B, Varoglu E, Gulec C, Bekdik CF: Assessment of changes in regional cerebral blood flow in patients with major depression using the 99mTc-HMPAO single photon emission tomography method. Eur J Nucl Med 1992; 19:1038–1043Crossref, Medline, Google Scholar
65. Kimbrell TA, Ketter TA, George MS, Little JT, Benson BE, Willis MW, Herscovitch P, Post RM: Regional cerebral glucose utilization in patients with a range of severities of unipolar depression. Biol Psychiatry 2002; 51:237–252Crossref, Medline, Google Scholar
66. Oda K, Okubo Y, Ishida R, Murata Y, Ohta K, Matsuda T, Matsushima E, Ichimiya T, Suhara T, Shibuya H, Nishikawa T: Regional cerebral blood flow in depressed patients with white matter magnetic resonance hyperintensity. Biol Psychiatry 2003; 53:150–156Crossref, Medline, Google Scholar
67. Biver F, Goldman S, Delvenne V, Luxen A, De Maertelaer V, Hubain P, Mendlewicz J, Lotstra F: Frontal and parietal metabolic disturbances in unipolar depression. Biol Psychiatry 1994; 36:381–388Crossref, Medline, Google Scholar
68. Dolan RJ, Bench CJ, Liddle PF, Friston FJ, Frith CD, Grasby PM, Frackowiak RS: Dorsolateral prefrontal cortex dysfunction in the major psychoses; symptom or disease specificity. J Neurol Neurosurg Psychiatry 1993; 56:1290–1294Crossref, Medline, Google Scholar
69. Sackeim HA, Prohovnik I, Moeller JR, Brown RP, Apter S, Prudic J, Devanand DP, Mukherjee S: Regional cerebral blood flow in mood disorders, I: comparison of major depressives and normal controls at rest. Arch Gen Psychiatry 1990; 47:60–70Crossref, Medline, Google Scholar
70. Lee S, Payne ME, Steffens DC, McQuoid DR, Lai TL, Provenzale JM, Krishnan KRR: Subcortical lesion severity and orbitofrontal cortex volume in geriatric depression. Biol Psychiatry 2003; 54:529–533Crossref, Medline, Google Scholar
71. Kumar A, Mintz J, Bilker W, Gottlieb G: Autonomous neurobiological pathways to late-life major depressive disorder: clinical and pathophysiological implications. Neuropsychopharmacology 2002; 26:229–236Crossref, Medline, Google Scholar
72. Webster MJ, Knable MB, O’Grady J, Orthmann J, Weickert CS: Regional specificity of brain glucocorticoid receptor mRNA alterations in subjects with schizophrenia and mood disorders. Mol Psychiatry 2002; 7:985–994Crossref, Medline, Google Scholar
73. Alexopoulos GS, Meyers BS, Young RC, Kalayam B, Kakuma T, Gabrielle M, Sirey JA, Hull J: Executive dysfunction and long-term outcomes of geriatric depression. Arch Gen Psychiatry 2000; 57:285–290Crossref, Medline, Google Scholar
74. Scahill RI, Schott JM, Stevens JM, Rossor MN, Fox NC: Mapping the evolution of regional atrophy in Alzheimer’s disease: unbiased analysis of fluid-registered serial MRI. Proc Natl Acad Sci USA 2003; 99:4703–4707Crossref, Google Scholar
75. Sowell ER, Thompson PM, Mattson SN, Tessner KD, Jernigan TL, Riley EP, Toga AW: Regional shape abnormalities persist into adolescence after heavy prenatal alcohol exposure. Cereb Cortex 2002; 12:856–865Crossref, Medline, Google Scholar