Polygenic Risk and Episode Polarity Among Individuals With Bipolar Disorder
Abstract
Objective:
The authors investigated associations between polygenic liabilities for bipolar disorder, major depression, and schizophrenia and episode polarity among individuals with bipolar disorder.
Methods:
The sample consisted of 2,705 individuals diagnosed with bipolar disorder at Danish psychiatric hospitals between January 1995 and March 2017. DNA was obtained from dried blood spots collected at birth as part of routine screening. Polygenic risk scores (PRSs) for bipolar disorder, major depression, and schizophrenia were generated using a meta-PRS method combining internally and externally trained components. Associations between PRS and polarity at first episode, polarity at any episode, and number of episodes with a given polarity were evaluated for each disorder-specific PRS using logistic and negative binominal regressions adjusted for the other two PRSs, age, sex, genotype platform, and five ancestral principal components.
Results:
PRS for bipolar disorder was positively associated with any manic episodes (odds ratio=1.23, 95% CI=1.09–1.38). PRS for depression was positively associated with any depressive (odds ratio=1.11, 95% CI=1.01–1.23) and mixed (odds ratio=1.15, 95% CI=1.03–1.28) episodes and negatively associated with any manic episodes (odds ratio=0.76, 95% CI=0.69–0.84). PRS for schizophrenia was positively associated with any manic episodes (odds ratio=1.13, 95% CI=1.01–1.27), but only when psychotic symptoms were present (odds ratio for psychotic mania: 1.27, 95% CI=1.05–1.54; odds ratio for nonpsychotic mania: 1.06, 95% CI=0.93–1.20). These patterns were similar for first-episode polarity and for the number of episodes within each pole.
Conclusions:
PRSs for bipolar disorder, major depression, and schizophrenia are associated with episode polarity and psychotic symptoms in a congruent manner among individuals with bipolar disorder.
Bipolar disorder is a severe, often debilitating mental illness affecting approximately 2% of the general population worldwide (1). Individuals with bipolar disorder can experience manic, depressive, and mixed episodes throughout their course of illness, which may or may not include psychotic symptoms (2, 3). Presenting an excess of one or more of these types of episodes is not uncommon, as it occurs in approximately half of all those with bipolar disorder (4). Most studies of polarity suggest that having more depressive episodes is the most prevalent phenotypic presentation (5, 6), but having more manic episodes is also common (7, 8). Episode polarity has been studied extensively and has been found to be associated with a wide variety of clinical outcomes, making it of interest as a phenotypic marker in the treatment of bipolar disorder (4).
Genetic factors account for a considerable proportion of the population-level variation in bipolar disorder (9), and previous studies have demonstrated shared genetic liability between bipolar disorder and other mental disorders, including major depressive disorder and schizophrenia (10, 11). Recently, polygenic risk scores (PRSs)—a weighted sum of genetic risk variants associated with a disorder or trait (12)—have been shown to be associated with clinically relevant outcomes such as progression to bipolar disorder in individuals with major depression (13), bipolar disorder subtypes (type I vs. type II) (14), psychosis in bipolar disorder (15), and response to lithium treatment (16). These findings support the idea that polarity in bipolar disorder may also in part be genetically determined.
Since the prognosis and ideal treatment varies for different episode polarities (17), information on predictors of phenotypic presentation, potentially including genetics, in bipolar disorder could aid clinicians in making more individualized treatment decisions (18). Therefore, the aim of this study was to assess the degree to which polygenic liabilities for bipolar disorder, major depression, and schizophrenia, measured using PRSs, are associated with episode polarity in individuals with bipolar disorder. Furthermore, we examined whether PRSs are associated with psychotic symptoms in the context of different episode polarities.
Methods
Data Source
Data for this study were obtained from the Lundbeck Foundation Initiative for Integrative Psychiatric Research iPSYCH2015 case-cohort sample, which is a newly updated version of the iPSYCH2012 sample (19). The iPSYCH2015 sample was drawn from a base population of all singletons born in Denmark between 1981 and 2008 who survived to their first birthday and had known mothers. The sample combines genetic and register-based data for all individuals within the base population who were diagnosed with certain mental disorders (affective disorder, schizophrenia, schizophrenia spectrum disorder, attention deficit hyperactivity disorder, autism, anorexia nervosa, or postpartum mental disorder) at Danish psychiatric hospitals during the period from January 1, 1995, to December 31, 2015 (N=93,608) (20). The sample also includes a randomly selected subset of 50,615 individuals drawn from the Danish base population. Members of the iPSYCH2015 case cohort were identified using data from the Danish Civil Registration System (21), which contains demographic and vital status information on all individuals born or residing in Denmark as well as the unique 10-digit personal registration number, which allows for individual linkage of information from all population-based registers in Denmark. Data on psychiatric diagnoses were obtained from the Danish Central Psychiatric Research Register (DCPRR), which includes information on all psychiatric diagnoses assigned in relation to inpatient treatment at psychiatric hospitals in Denmark since 1969 as well as outpatient and emergency treatment since 1995 (22). Diagnoses in the DCPRR are based on ICD-10 and are assigned by the treating psychiatrist as part of routine clinical care.
Genotyping
DNA for members of the iPSYCH2015 case cohort was obtained from dried blood spots collected at birth as part of routine clinical testing for congenital disorders and stored in the Danish Newborn Screening Biobank (23). iPSYCH2012 samples were genotyped using the Illumina Infinium Psychchip v1.0 array (Illumina, San Diego), and iPSYCH2015 samples were genotyped using the Illumina Global Screening array. In both samples, genotyping was carried out according to the manufacturer’s protocols. Quality control was performed as follows: First, samples with a call rate ≤95% were excluded. Second, the genetically obtained sex was compared to the sex recorded in the Danish Civil Registration System. All samples that failed to match with the recorded sex without valid reason (e.g., aneuploidy or change of gender) were excluded from the sample. Imputation was conducted using the RICOPILI pipeline (24). After removing single-nucleotide polymorphisms (SNPs) with minor allele frequency <0.01 and Hardy-Weinberg p value <10−6 (χ2 [df=1] test statistic pHWE), we restricted SNPs to the HapMap3 variants. The final data set consisted of 1,184,443 SNPs and was then used for the genetic analyses. We then calculated the first five genetic principal components using the autoSVD algorithm available in the R package bigsnpr (25), following the guidelines outlined by Privé et al. (26).
Study Sample
A flowchart of the sample selection process is shown in Figure S1 in the online supplement. We identified all individuals from the iPSYCH2015 study who 1) received a main, secondary, or “underlying cause” diagnosis of bipolar disorder (ICD-10 codes F30–F31, F38.00) at age 10 or later in relation to inpatient or outpatient treatment at a psychiatric hospital in Denmark between January 1, 1995, and March 31, 2017; 2) had no prior diagnosis of schizophrenia or related disorders (ICD-10 codes F20–F29); and 3) were successfully genotyped. The majority of individuals in the iPSYCH2015 sample are of European ancestry. For the sake of statistical power, we did not exclude individuals of non-European ancestry, as previous research in this cohort has demonstrated that such exclusions have limited impact on the results (13, 27). Instead, we controlled for ancestry using principal components in the analyses and also performed sensitivity analyses excluding individuals of non-European ancestry. The final study sample comprised 2,705 individuals.
Episode Polarity
Information on episode polarity was obtained from the DCPRR (22). Episodes were defined on the basis of either an inpatient contact (admission) or a series of outpatient contacts. Episode polarity (depressive, manic, mixed, unspecified) was defined according to the ICD-10 diagnostic codes registered in the DCPRR. For post hoc analyses, we also created subcategories for psychotic symptoms in any polarity, psychotic mania, and psychotic depression. The ICD-10 codes used to define polarities are listed in Table S1 in the online supplement. Unipolar depressive episodes were included in the (bipolar) depression polarity category, as patients with bipolar disorder frequently receive a unipolar depression diagnosis prior to their first bipolar diagnosis, and these episodes can reasonably be considered bipolar depressive episodes in retrospect.
The DCPRR describes admissions and series of outpatient contacts that occasionally overlap in time or immediately follow each other. In the event of two separate but overlapping or back-to-back admissions or outpatient contacts with a diagnosis of bipolar disorder, we chose to merge those records with the same polarity that were separated by a period of less than 8 weeks. However, if the records had different polarities, we considered them separate episodes regardless of the interval between them. For this process of merging hospital contacts, we considered psychotic mania and psychotic depression as separate categories.
Calculation of Polygenic Risk Scores
PRSs for bipolar disorder, major depression, and schizophrenia were calculated using a meta-PRS method (28). The meta-PRS method combines two independently constructed PRSs, namely, a PRS trained on external (not including iPSYCH) genome-wide association study (GWAS) summary statistics and an internal PRS derived from individual-level genetic data (28). The external PRSs were generated using the most recent GWAS summary results from the Psychiatric Genomics Consortium (PGC) meta-analyses for bipolar disorder (14), major depression (29), and schizophrenia (30) using LDpred2-auto (31). The internal PRS was trained on the iPSYCH sample using BOLT-LMM (32), with a fivefold cross-validation scheme to avoid overfitting the model. The external and the internal PRSs were then combined into a meta-PRS with the formula w1*PRSinternal+w2*PRSexternal. The weights w1 and w2 were calculated from the individual-level data with a linear regression model using nested cross-validation, as described in the meta-PRS method from Albiñana et al. (28). This method was chosen because it increases polygenic prediction accuracy compared to methods using external data alone (28). The final PRSs were standardized (mean=0, SD=1) according the mean and standard deviation from the iPSYCH2015 random subcohort.
Statistical Analysis
Associations between episode polarity and PRS variables were examined in three ways. First, we ran binary logistic regression models to estimate the associations between PRS variables and the odds of having a given polarity versus not having that polarity at the first recorded hospital contact for bipolar disorder. Second, we ran binary logistic regression models examining associations between PRS variables and the odds of having a given polarity at any hospital contact for bipolar disorder versus never having it. Third, we ran negative binomial regression models to examine the associations between PRS variables and the number of episodes with a given polarity (or with psychotic symptoms) recorded for each individual. All models were adjusted for gender, age at first bipolar diagnosis, the first five principal components, and genotyping platform. Logistic regression models were additionally adjusted for the total number of episodes. All three PRS variables were included in the models to control for shared genetic liabilities between the disorders. Individual associations between PRS variables and polarity (adjusted for the first five principal components and genotyping platform) are shown in Figures S2 and S3 and Table S2 in the online supplement. Analyses were performed using SAS, version 9.4.0.
Sensitivity Analyses
To assess how our sampling choices affected the association between PRSs and episode polarity, we ran two separate sensitivity analyses. First, we examined the association only among the fraction of the sample who had parents born in Denmark. Second, we examined the associations between PRSs and polarity of episodes in the 3-year period following initial diagnosis among the subset of the sample with at least 3 years of follow-up, to ensure equal follow-up time for all patients.
Ethics
The Danish Scientific Ethics Committee, the Danish Health Data Authority, the Danish Data Protection Agency, the Danish Neonatal Screening Biobank steering committee, and Statistics Denmark approved this study (20).
Results
Sample Characteristics
The demographic and clinical characteristics of the study sample are summarized in Table 1. The patients in the sample were young (median age, 22 years) and predominantly female (65%). Follow-up time ranged from 1 day to 22 years, with a median of 5 years (interquartile range, 3–8 years). Most patients in the sample (83%) were followed for 3 or more years. The majority of the patients (72%) were treated in an outpatient setting, and almost half (45%) either had a depressive episode as the first registered episode of bipolar disorder or were registered with a diagnosis of unipolar depression prior to the first diagnosis of bipolar disorder.
Characteristic | ||
---|---|---|
Median | IQR | |
Age (years) at first diagnosis of bipolar disorder or unipolar depression | 22 | 19–25 |
Time in follow-up (years) | 5 | 3–8 |
N | % | |
Female | 1,761 | 65.1 |
Inpatient treatment setting at first diagnosis | 763 | 28.2 |
Number of episodes of bipolar disorder or unipolar depression | ||
1 | 971 | 35.9 |
2 | 745 | 27.6 |
3 | 447 | 16.5 |
4 | 241 | 9.0 |
5 | 116 | 4.2 |
6 | 67 | 2.5 |
7 | 47 | 1.7 |
8 | 16 | 0.6 |
9 | 23 | 0.9 |
≥10 | 32 | 1.2 |
Polarity at first episode | ||
Nonpsychotic depression | 1,227 | 45.4 |
Psychotic depression | 50 | 1.9 |
Nonpsychotic mania | 290 | 10.7 |
Psychotic mania | 117 | 4.3 |
Mixed | 226 | 8.4 |
Unspecified | 795 | 29.4 |
Polarity at any episode | ||
Nonpsychotic depression | 1,593 | 58.9 |
Psychotic depression | 148 | 5.5 |
Nonpsychotic mania | 562 | 20.8 |
Psychotic mania | 223 | 8.2 |
Mixed | 560 | 20.7 |
Unspecified | 1,709 | 63.2 |
Number of polarities in patients with >1 episode | ||
1 | 278 | 16.0 |
2 | 1,090 | 62.9 |
3 | 309 | 17.8 |
4 | 57 | 3.3 |
TABLE 1. Sample characteristics of 2,705 patients diagnosed with bipolar disorder in inpatient or outpatient hospital settings between 1995 and 2017a
Approximately one-third (N=971, 36%) of the sample had only one episode of bipolar disorder treated in a hospital setting during the study period. Among the patients with two or more episodes, 16% (N=278) had episodes of the same polarity type, 63% (N=1,090) had episodes of two different polarities, 18% (N=309) had episodes of three polarities, and 3% (N=57) had episodes in all four polarity categories. Figure 1 shows the distribution of polarity patterns among the 1,734 patients with bipolar disorder who were registered with two or more episodes during the study period. Depressive plus unspecified bipolar episodes constituted by far the most common polarity pattern observed in the sample.
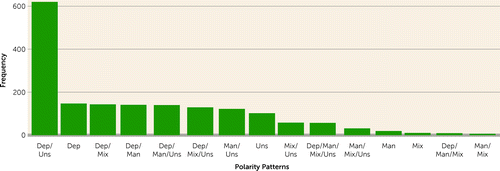
FIGURE 1. Patterns of polarity among patients with two or more episodes of bipolar disorder (N=1,734) in inpatient or outpatient psychiatric treatment between 1995 and 2017a
aDep=depressive polarity; Man=manic polarity; Mix=mixed polarity; Uns=unspecified polarity.
The mean observed PRSs for major depression (0.36 [SD=0.95]), bipolar disorder (0.29 [SD=0.96]), and schizophrenia (0.14 [SD=0.95]) were each elevated in patients with bipolar disorder relative to the background population in which mean=0 and SD=1 (standardized values). As in past studies (14), we found statistically significant correlations between all three PRS variables (see Table S3 in the online supplement), with the strongest correlation between bipolar disorder PRS and schizophrenia PRS (Pearson’s r=0.52, p<0.0001). Similar to a recent study (33), we found that higher bipolar disorder PRS (β=0.05, p=0.009) and schizophrenia PRS (β=0.03, p=0.0484) but not major depression PRS (β=−0.01, p=0.46) were associated with more episodes of bipolar disorder, regardless of polarity.
PRS and Episode Polarity
Figure 2 shows the associations between PRSs and episode polarity. Higher bipolar disorder PRS (odds ratio per standard deviation increase in bipolar disorder PRS, 1.23, 95% CI=1.09–1.38, p=0.0007) and schizophrenia PRS (odds ratio=1.13, 95% CI=1.01–1.27, p=0.04) were associated with statistically significantly increased odds of experiencing any episodes with manic polarity during the study time frame. Higher major depression PRS was associated with statistically significantly increased odds of experiencing any episode of depressive (odds ratio=1.11, 95% CI=1.01–1.23, p=0.03) and mixed polarity (odds ratio=1.15, 95% CI=1.03–1.28, p=0.01) but decreased odds of manic polarity (odds ratio=0.76, 95% CI=0.69–0.84, p<0.0001). There were no statistically significant associations between any of the three PRSs and unspecified polarity. The pattern of results was similar for episode polarity at the first diagnosis of bipolar disorder (Figure 2A) and episode polarity at any episode (Figure 2B), although the confidence intervals were wider for first diagnosis.
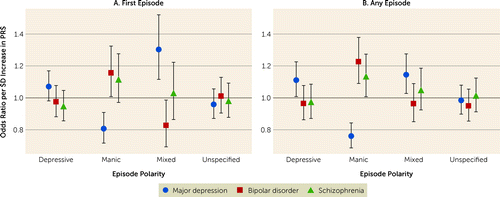
FIGURE 2. Associations between polygenic liabilities for bipolar disorder, schizophrenia, and major depression and polarity at first episode and at any episode in 2,705 patients with bipolar disordera
aEstimates were obtained from logistic regression models including all three PRS variables, gender, age at first bipolar diagnosis, the first five principal components, and genotyping platform (iPSYCH2012 vs. iPSYCH2015). PRS=polygenic risk score.
PRS and Number of Episodes of a Given Polarity
The pattern of results was similar when we looked at the number of episodes of a given polarity (Table 2; see also Figure S4 in the online supplement). Higher bipolar disorder PRS (β=0.21, p<0.0001) and schizophrenia PRS (β=0.14, p=0.01) were associated with more manic episodes. Higher major depression PRS was associated with more mixed episodes (β=0.09, p=0.048) and fewer manic episodes (β=−0.25, p<0.0001). Although the association with number of depressive episodes trended in the same direction, it was not statistically significant (β=0.04, p=0.09). Again, there were no statistically significant associations between any of the three PRSs and episodes of unspecified polarity.
Polygenic Risk Score | |||||||||
---|---|---|---|---|---|---|---|---|---|
Bipolar Disorder | Major Depression | Schizophrenia | |||||||
Polarity | β | SE | p | β | SE | p | β | SE | p |
Depressive | 0.01 | 0.03 | 0.7909 | 0.04 | 0.02 | 0.0939 | 0.00 | 0.03 | 0.9433 |
Manic | 0.21 | 0.05 | <0.0001 | −0.25 | 0.04 | <0.0001 | 0.14 | 0.05 | 0.0061 |
Mixed | 0.01 | 0.05 | 0.9191 | 0.09 | 0.05 | 0.0484 | 0.06 | 0.05 | 0.2485 |
Unspecified | 0.03 | 0.03 | 0.2379 | −0.01 | 0.02 | 0.5194 | 0.02 | 0.03 | 0.5513 |
TABLE 2. Polygenic risk score and number of episodes of a given polarity in 2,705 patients diagnosed with bipolar disorder in inpatient or outpatient hospital settings between 1995 and 2017a
PRS and Psychotic Symptoms
Figure 3 shows the associations between PRSs and manic or depressive episodes with and without psychotic symptoms. Higher bipolar disorder PRS was associated with increased odds of manic polarity, both with (odds ratio=1.27, 95% CI=1.06–1.54, p=0.01) and without (odds ratio=1.22, 95% CI=1.07–1.38, p=0.002) psychotic symptoms. Schizophrenia PRS, however, was associated with mania only when psychotic symptoms were present (odds ratio=1.27, 95% CI= 1.05–1.54, p=0.01). In contrast, major depression PRS was positively associated with depressive polarity when psychotic symptoms were absent (odds ratio=1.13, 95% CI=1.02–1.24, p=0.01) and negatively associated with depressive polarity in the presence of psychotic symptoms (odds ratio=0.80, 95% CI=0.66–0.96, p=0.02). Similar patterns were observed for the number of episodes (see Table S4 in the online supplement).
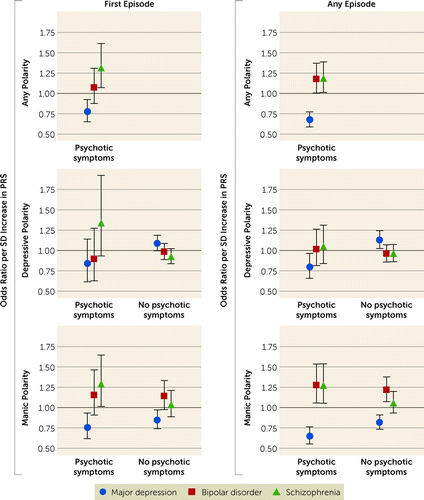
FIGURE 3. Associations between polygenic liabilities for bipolar disorder, schizophrenia, and major depression and psychotic symptoms at the first or any episode in 2,705 patients with bipolar disordera
a Estimates were obtained from logistic regression models including all three PRS variables, gender, age at first bipolar diagnosis, the first five principal components, and genotyping platform (iPSYCH2012 vs. iPSYCH2015). PRS=polygenic risk score.
Sensitivity Analyses
In the subsample of patients with Danish-born parents (N=2,366), the findings were similar to those of the main analyses reported above (see Tables S5–S8 in the online supplement). In the subsample of patients with at least 3 years of follow-up (N=1,926), all findings were similar to those of the main analyses except that schizophrenia PRS was also significantly associated with increased odds of nonpsychotic mania (see Tables S9–S12 in the online supplement).
Discussion
In this study, we examined whether polygenic liabilities for bipolar disorder, major depression, and schizophrenia are associated with episode polarity and psychotic symptoms in individuals with bipolar disorder. The results show that polygenic liability for bipolar disorder was positively associated with manic polarity, while polygenic liability for depression was positively associated with depressive and mixed polarities and negatively associated with manic polarity. Polygenic liability for schizophrenia was positively associated with manic polarity, but only when psychotic symptoms were present. These findings suggest that episode polarity and psychotic symptoms in individuals with bipolar disorder are, at least in part, genetically determined, and provide further evidence that phenotypic heterogeneity in bipolar disorder is related to underlying genetic heterogeneity.
Previous studies identified positive correlations between PRSs for bipolar disorder and schizophrenia and psychotic symptoms in bipolar disorder (34, 35). This could suggest that polygenic liabilities for both bipolar disorder and schizophrenia drive the psychotic phenotype to some degree, which is consistent with the findings of a study by Musliner et al. (13), who report that having high polygenic liability for both bipolar disorder and schizophrenia aligned with the greatest risk of developing affective psychosis in patients with unipolar depression. It is also consistent with findings from the Bipolar Disorder Working Group of the PGC (36), who showed that psychotic symptoms were associated with high bipolar disorder PRS and schizophrenia PRS, while manic symptoms were only associated with bipolar disorder PRS. Although still preliminary, these findings add to a growing body of evidence, including family studies assessing parental psychotic and affective disorders as risk factors for these same disorders in offspring, suggesting that both the affective and the psychotic genetic components play a role in the phenotypical presentation and time of onset of affective disorders (13, 37–39). The findings could, however, also be related to the large symptom overlap between psychotic and affective disorders (40), and to the fact that some individuals in our sample will go on to develop a schizophrenia spectrum disorder later in life (41).
Our findings indicate that patients with bipolar disorder who have higher polygenic liability for depression are significantly less likely to experience psychotic symptoms in either depressive or manic episodes. This suggests that psychotic depression may be more genetically similar to bipolar disorder or schizophrenia than to nonpsychotic depression—an interpretation supported by previous research showing that a parental history of bipolar disorder was a risk factor for psychotic depression but not for nonpsychotic depression (37). This idea that psychotic depression may be a somewhat separate genetic entity from more common phenotypic presentations of depression (i.e., nonpsychotic) (42) is also supported by findings from Coombes et al. (43), who showed that individuals with bipolar disorder who had an increased polygenic score for anhedonia (a core symptom of depression) were less likely to have a history of psychotic symptoms.
We found that the polygenic liability for depression was positively associated with mixed episodes, which often have severe symptoms and poor clinical outcomes (44). Interestingly, pharmacological treatment of mixed episodes is more similar to treatments of mania and psychosis than to treatments of depression (45), and yet our findings suggest that mixed episodes may be more similar genetically to major depression than to bipolar disorder or schizophrenia. Additional research is needed to explore this somewhat unexpected finding further. Finally, none of the three PRSs showed any type of association with episodes of unspecified polarity. This makes intuitive sense, as unspecified polarity likely represents a mix of the specified polarities (depression, mania, and mixed), possibly of less severity. The unspecified polarity category alone is therefore of limited interest. However, the lack of association between the three PRSs and episodes of unspecified polarity does indirectly support the validity of the associations between PRSs and the congruent episode polarities.
The results of this study suggest that PRSs may be useful in the prediction of the clinical course of bipolar disorder. Since optimal treatment differs for different types of episodes in the context of bipolar disorder (17, 46), we hypothesize that PRSs may also play a future role in more personalized treatment choices (18). Moreover, new evidence suggests that information on PRSs may also be of benefit for patients in other ways. A recent study by Putt et al. (47) found that access to information on PRSs was associated with improved awareness of symptoms, decreased stigma, and improved treatment adherence among patients with bipolar disorder.
Our study has several limitations that need to be acknowledged. First, our sample did not include individuals with bipolar disorder who were treated solely by general practitioners or private-practice psychiatrists, as these practitioners do not report data to the DCPRR. However, since bipolar disorder is a fairly severe mental illness and psychiatric hospital treatment in Denmark is free (tax-funded), the proportion of individuals who are untreated or treated only by general practitioners or private-practice psychiatrists in Denmark can be assumed to be relatively small. Nevertheless, the patients in the iPSYCH2015 cohort likely represent the more severely ill fraction, and the reported associations between polygenic liability and episode polarity may therefore not be representative of bipolar disorder in general.
Second and relatedly, any episode of bipolar disorder that is not severe enough to have led to hospital contact is not registered in the DCPRR. This will likely have affected the validity of the outcomes of our study—namely, the first registered episode of bipolar disorder, any episode of a given polarity, and the number of episodes of a given polarity—as none of these include episodes that did not lead to hospital contacts. It can be argued that they should therefore rather be referred to as the first registered severe episode of bipolar disorder, any severe episode of a given polarity, and the number of severe episodes of a given polarity. Despite this “severity bias,” we would argue that the results remain of interest from both an etiological and a clinical perspective.
Third, we could not examine differences between patients with bipolar I and bipolar II disorders, as diagnoses in the registers are based on ICD-10, which does not explicitly distinguish between bipolar I and II disorders. Fourth, as data on symptom levels over time are not available in the registers, we were unable to investigate the relationship between the three PRSs and symptom levels in bipolar disorder. If such data had been available, and if the PRSs had had a better fit to the symptom data compared with the polarity data, it would have offered further support for the dimensional (diagnostic) approach to bipolar disorder. It can be argued, however, that our finding of psychotic mania (as opposed to nonpsychotic mania) driving the association between the PRS for schizophrenia and manic polarity already offers some support for the dimensional model.
Fifth, all individuals in the sample were between 10 and 35 years old when they had their first episode of bipolar disorder. Hence, some of these individuals may go on to develop a disorder in the schizophrenia spectrum later in life (41), which may drive some of the association between the schizophrenia PRS and psychotic mania. Sixth, the predictive accuracy of the polygenic scores is affected by both the training data sets and the method used to train the scores. In this study we followed the approach of Albiñana et al. (28) and used a combination of the PGC GWAS summary statistics and iPSYCH individual-level data (using cross-validation) as training data. Using other methods and training data would lead to different results, and potentially also to different conclusions. Relatedly, as the results from larger GWASs become available, it is likely that the prediction of episode polarity and psychosis in bipolar disorder will improve.
The present study also has numerous strengths. iPSYCH2015 is currently one of the largest and most comprehensive samples for studying the genetics of mental disorders (20). Furthermore, the population-based sampling approach of iPSYCH2015 minimizes selection bias and increases generalizability to broader populations. The study also uses validated (48), clinician-derived diagnoses as the outcome, which is particularly relevant for interpreting the potential of PRSs in a real-world clinical context. Lastly, since the iPSYCH2015 sample is mostly of Danish or European ancestry, it reduces the possibility of confounding by population stratification.
In summary, PRSs for bipolar disorder, major depression, and schizophrenia were found to be positively associated with episode polarity and psychotic symptoms in individuals with bipolar disorder in a congruent manner. These findings provide preliminary evidence that episode polarity and psychotic symptoms in patients with bipolar disorder are partly genetically determined. Although PRSs are not yet ready to be applied in clinical practice, the performance of PRSs is expected to improve as larger GWAS discovery data sets and improved calculation methods become available.
1. : Prevalence and correlates of bipolar spectrum disorder in the World Mental Health Survey Initiative. Arch Gen Psychiatry 2011; 68:241–251Crossref, Medline, Google Scholar
2. : The long-term natural history of the weekly symptomatic status of bipolar I disorder. Arch Gen Psychiatry 2002; 59:530–537Crossref, Medline, Google Scholar
3. : The association between psychotic mania, psychotic depression, and mixed affective episodes among 14,529 patients with bipolar disorder. J Affect Disord 2013; 147:44–50Crossref, Medline, Google Scholar
4. : Predominant polarity as a course specifier for bipolar disorder: a systematic review. J Affect Disord 2014; 163:56–64Crossref, Medline, Google Scholar
5. : Clinical and therapeutic implications of predominant polarity in bipolar disorder. J Affect Disord 2006; 93:13–17Crossref, Medline, Google Scholar
6. : Predominant polarity in bipolar I and II disorders: a five-year follow-up study. J Affect Disord 2019; 246:806–813Crossref, Medline, Google Scholar
7. : Predominant polarity and temperament in bipolar and unipolar affective disorders. J Affect Disord 2009; 119:28–33Crossref, Medline, Google Scholar
8. : Predominant recurrence polarity among 928 adult international bipolar I disorder patients. Acta Psychiatr Scand 2012; 125:293–302Crossref, Medline, Google Scholar
9. : A population-based heritability estimate of bipolar disorder in a Swedish twin sample. Psychiatry Res 2019; 278:180–187Crossref, Medline, Google Scholar
10. : Genetic relationship between five psychiatric disorders estimated from genome-wide SNPs. Nat Genet 2013; 45:984–994Crossref, Medline, Google Scholar
11. : Common polygenic variation contributes to risk of schizophrenia that overlaps with bipolar disorder. Nature 2009; 460:748–752Crossref, Medline, Google Scholar
12. : From basic science to clinical application of polygenic risk scores: a primer. JAMA Psychiatry 2021; 78:101–109Crossref, Medline, Google Scholar
13. : Polygenic risk and progression to bipolar or psychotic disorders among individuals diagnosed with unipolar depression in early life. Am J Psychiatry 2020; 177:936–943Link, Google Scholar
14. : Genome-wide association study identifies 30 loci associated with bipolar disorder. Nat Genet 2019; 51:793–803Crossref, Medline, Google Scholar
15. : Association of schizophrenia polygenic risk score with manic and depressive psychosis in bipolar disorder. Transl Psychiatry 2018; 8:188Crossref, Medline, Google Scholar
16. : Association of polygenic score for major depression with response to lithium in patients with bipolar disorder. Mol Psychiatry 2021; 26:2457–2470Crossref, Medline, Google Scholar
17. : Treatment of bipolar disorder. Lancet 2013; 381:1672–1682Crossref, Medline, Google Scholar
18. : Personalized management of bipolar disorder. Neurosci Lett 2018; 669:3–9Crossref, Medline, Google Scholar
19. : The iPSYCH2012 case-cohort sample: new directions for unravelling genetic and environmental architectures of severe mental disorders. Mol Psychiatry 2018; 23:6–14Crossref, Medline, Google Scholar
20. : The iPSYCH2015 Case-Cohort sample: updated directions for unravelling genetic and environmental architectures of severe mental disorders. medRxiv, December 2, 2020. https://doi.org/10.1101/2020.11.30.20237768 Google Scholar
21. : The Danish Civil Registration System. Scand J Public Health 2011; 39(suppl 7):22–25Crossref, Medline, Google Scholar
22. : The Danish Psychiatric Central Research Register. Scand J Public Health 2011; 39(suppl 7):54–57Crossref, Medline, Google Scholar
23. : Storage policies and use of the Danish Newborn Screening Biobank. J Inherit Metab Dis 2007; 30:530–536Crossref, Medline, Google Scholar
24. : RICOPILI: Rapid Imputation for Consortias Pipeline. Bioinformatics 2020; 36:930–933Crossref, Medline, Google Scholar
25. : Efficient analysis of large-scale genome-wide data with two R packages: bigstatsr and bigsnpr. Bioinformatics 2018; 34:2781–2787Crossref, Medline, Google Scholar
26. : Efficient toolkit implementing best practices for principal component analysis of population genetic data. Bioinformatics 2020; 36:4449–4457Crossref, Medline, Google Scholar
27. : Polygenic risk score, psychosocial environment, and the risk of attention-deficit/hyperactivity disorder. Transl Psychiatry 2020; 10:335Crossref, Medline, Google Scholar
28. : Leveraging both individual-level genetic data and GWAS summary statistics increases polygenic prediction. Am J Hum Genet 2021; 108:1001–1011Crossref, Medline, Google Scholar
29. : Genome-wide meta-analysis of depression identifies 102 independent variants and highlights the importance of the prefrontal brain regions. Nat Neurosci 2019; 22:343–352Crossref, Medline, Google Scholar
30. : Biological insights from 108 schizophrenia-associated genetic loci. Nature 2014; 511:421–427Crossref, Medline, Google Scholar
31. : LDpred2: better, faster, stronger. Bioinformatics 2020; 36:5424–5431Crossref, Medline, Google Scholar
32. : Efficient Bayesian mixed-model analysis increases association power in large cohorts. Nat Genet 2015; 47:284–290Crossref, Medline, Google Scholar
33. : Genetic risk for psychiatric illness is associated with the number of hospitalizations of bipolar disorder patients. J Affect Disord 2022; 296:532–540Crossref, Medline, Google Scholar
34. : Association between schizophrenia-related polygenic liability and the occurrence and level of mood-incongruent psychotic symptoms in bipolar disorder. JAMA Psychiatry 2018; 75:28–35Crossref, Medline, Google Scholar
35. : Polygenic risk scores in bipolar disorder subgroups. J Affect Disord 2015; 183:310–314Crossref, Medline, Google Scholar
36. : Genomic dissection of bipolar disorder and schizophrenia, including 28 subphenotypes. Cell 2018; 173:1705–1715.e16Crossref, Medline, Google Scholar
37. : Environmental and familial risk factors for psychotic and non-psychotic severe depression. J Affect Disord 2013; 147:232–240Crossref, Medline, Google Scholar
38. : Patterns and predictors of conversion to bipolar disorder in 91,587 individuals diagnosed with unipolar depression. Acta Psychiatr Scand 2018; 137:422–432Crossref, Medline, Google Scholar
39. : Progression from unipolar depression to schizophrenia. Acta Psychiatr Scand 2017; 135:42–50Crossref, Medline, Google Scholar
40. : Exploring comorbidity within mental disorders among a Danish national population. JAMA Psychiatry 2019; 76:259–270Crossref, Medline, Google Scholar
41. : Diagnostic stability in bipolar disorder in clinical practise as according to ICD-10. J Affect Disord 2005; 85:293–299Crossref, Medline, Google Scholar
42. : Considerations on the ICD-11 classification of psychotic depression. Psychother Psychosom 2012; 81:135–144Crossref, Medline, Google Scholar
43. : Dissecting clinical heterogeneity of bipolar disorder using multiple polygenic risk scores. Transl Psychiatry 2020; 10:314Crossref, Medline, Google Scholar
44. : Bipolar mixed states: an International Society for Bipolar Disorders task force report of symptom structure, course of illness, and diagnosis. Am J Psychiatry 2013; 170:31–42Link, Google Scholar
45. : Mixed states in bipolar disorder: etiology, pathogenesis, and treatment. Chonnam Med J 2017; 53:1–13Crossref, Medline, Google Scholar
46. : Psychopharmacological treatment of psychotic mania and psychotic bipolar depression compared to non-psychotic mania and non-psychotic bipolar depression. Bipolar Disord 2017; 19:505–512Crossref, Medline, Google Scholar
47. : Exploration of experiences with and understanding of polygenic risk scores for bipolar disorder. J Affect Disord 2020; 265:342–350Crossref, Medline, Google Scholar
48. : Validity of diagnoses and other clinical register data in patients with affective disorder. Eur Psychiatry 1998; 13:392–398Crossref, Medline, Google Scholar