Functional Connectivity Mapping for rTMS Target Selection in Depression
Abstract
Objective:
Repetitive transcranial magnetic stimulation (rTMS) protocols increasingly use subgenual anterior cingulate cortex (sgACC) functional connectivity to individualize treatment targets. However, the efficacy of this approach is unclear, with conflicting findings and varying effect sizes across studies. Here, the authors investigated the effect of the stimulation site’s functional connectivity with the sgACC (sgACC-StimFC) on treatment outcome to rTMS in 295 patients with major depression.
Methods:
The reliability and accuracy of estimating sgACC functional connectivity were validated with data from individuals who underwent extensive functional MRI testing. Electric field modeling was used to analyze associations between sgACC-StimFC and clinical improvement using standardized assessments and to evaluate sources of heterogeneity.
Results:
An imputation-based method provided reliable and accurate sgACC functional connectivity estimates. Treatment responses weakly but robustly correlated with sgACC-StimFC (r=−0.16), but only when the stimulated cortex was identified using electric field modeling. Surprisingly, this association was driven by patients with strong global signal fluctuations stemming from a specific periodic respiratory pattern (r=−0.49).
Conclusions:
Functional connectivity between the sgACC and the stimulated cortex was correlated with individual differences in treatment outcomes, but the association was weaker than those observed in previous studies and was accentuated in a subgroup of patients with distinct, respiration-related signal patterns in their scans. These findings indicate that in a large representative sample of patients with major depressive disorder, individual differences in sgACC-StimFC explained only ∼3% of the variance in outcomes, which may limit the utility of existing sgACC-based targeting protocols. However, these data also provide strong evidence for a true—albeit small—effect and highlight opportunities for incorporating additional functional connectivity measures to generate models of rTMS response with enhanced predictive power.
Repetitive transcranial magnetic stimulation (rTMS) to the left dorsolateral prefrontal cortex (DLPFC), a treatment approved by the U.S. Food and Drug Administration for major depressive disorder, induces antidepressant responses in some but not all individuals (1). To optimize treatment, approaches guided by functional MRI (fMRI) have been developed that select target sites based on their functional connectivity (FC) properties (2–10). These methods are increasingly being implemented in experimental rTMS research protocols.
The most common fMRI-guided targeting approach builds on studies associating negative functional connectivity of the stimulation site with the subgenual anterior cingulate cortex (sgACC-StimFC) with better treatment outcomes (11–15). However, strong effects in pioneering studies (3, 5, 6, 8, 16) have been intermixed with negative reports (4, 9, 17, 18). The median sample size for these studies was 25 subjects.
Precise effect size estimates are specifically important for novel biomarkers being used in clinical applications. This report extensively probes possible explanations for mixed associations between sgACC-StimFC and clinical outcomes. First, by examining sgACC connectivity in single subjects with large amounts of fMRI data (“precision functional mapping” data sets), we show that existing methods (2) compensate adequately for noisy sgACC signals. Second, by examining the association between sgACC-StimFC and treatment outcome in the largest data set to date—295 patients with major depressive disorder who received either 10 Hz repetitive transcranial magnetic stimulation (rTMS) or intermittent theta-burst stimulation (iTBS) (19)—we find the model used to estimate the stimulated area in the DLPFC to be a relevant source of variability. Third, by subsampling this large population, which showed a weak overall effect, we demonstrate that sampling variability alone can account for the variable efficacy reported in the literature. Finally, by examining the association between signal quality and treatment prediction, we show, unexpectedly, that a subsample of the population with pronounced global signal variation drives the relationship of sgACC-StimFC to clinical outcome; this subsample was characterized by an especially high variance in global fMRI signals that occur in association with a known pattern of breathing that is prominent in some patients with depression (20).
Collectively, these results provide strong evidence for a true association between FC of the stimulation site with the sgACC and clinical outcome but call into question the utility of current FC-based targeting protocols that rely on single-echo fMRI data and isolated sgACC FC measures, because our results imply that only about 3% of clinical outcome variability is modifiable by this approach.
Methods
Details on all methods and procedures can be found in the online supplement.
Validation of the Weight-Map Approach to Imputing sgACC Signals
Two densely sampled resting-state fMRI data sets were used to validate the weight-map approach (2): a single-echo fMRI data set (named the Midnight Scan Club [MSC] data set in the original publication) of 10 subjects (five female) each with 5 hours of scan time (21) and a multiecho fMRI data set (named the multiecho [ME] data set in the original publication) with five subjects (all male) each with up to 15 hours of scan time (22). See Figure S1 in the online supplement for a graphical explanation of the weight-map method.
Modeling of Stimulation Sites in the DLPFC
Estimating sgACC-StimFC requires a model of the cortical area within the DLPFC that was stimulated in an rTMS session. This is not a straightforward process, as parameters related to stimulation itself and an individual’s anatomy impact the shape of the effective electric field (E-field), which is often multifocal and does not evenly surround the target coordinate. Two competing methods were used to model where rTMS stimulation affected tissue in the DLPFC: E-field modeling performed with the MagVenture B70 coil file in SimNIBS (23) and modeling with the generic “weighted-cone” approximation of the E-field as a 12-mm distance-weighted hemisphere (2–6, 8, 9). For both modeling approaches, sgACC-StimFC was ultimately derived by averaging all of the sgACC FC features within the cortical surface area covered by the relevant E-field or weighted cone after applying thresholds for E-field strength or diameter, respectively.
Modeling Clinical Outcome in THREE-D Patients as a Function of sgACC-StimFC
Sample and procedure.
Details on the THREE-D study (examining the effectiveness of theta burst versus high-frequency rTMS in patients with depression), including the population and TMS treatment protocols, have been published elsewhere (19). Briefly, in the THREE-D study, 414 patients with major depressive disorder were randomly allocated in a 1:1 ratio to receive 20 treatments of either 10 Hz rTMS or iTBS to the left DLPFC. Targeted treatment was based on back-transformation of a predefined stereotactic coordinate (x=−38, y=44, z=26) in Montreal Neurological Institute (MNI) space (see Figure S2 in the online supplement). Depression severity was assessed with the Quick Inventory of Depressive Symptomatology (QIDS-SR) and the 17-item version of the Hamilton Depression Rating Scale (HAM-D). Although the HAM-D was the primary outcome measure in the THREE-D study, we focused on the QIDS-SR for the results reported in the main text for reasons described in the Methods section of the online supplement. However, we also report effects with the HAM-D in the online supplement. Details on inclusion criteria and rTMS targeting procedures are provided in the Methods section of the online supplement, and the sample characteristics of the 295 THREE-D subjects used in these retrospective analyses are presented in Table 1.
Measure | 10 Hz rTMS group (N=144) | iTBS group (N=151) | ||
---|---|---|---|---|
N | % | N | % | |
Female | 83 | 58 | 94 | 62 |
Male | 61 | 42 | 57 | 38 |
Mean | SD | Mean | SD | |
Age (years) | 43.3 | 12.6 | 42.5 | 10.4 |
Age at onset (years) | 20.8 | 11.2 | 20.9 | 11.3 |
Baseline HAM-D score | 23.4 | 4.6 | 23.6 | 4.4 |
Baseline QIDS-SR score | 17.4 | 3.8 | 16.7 | 4 |
Clinical improvement (% change in HAM-D score) | 42.0 | 30.9 | 42.7 | 33.3 |
Clinical improvement (% change in QIDS-SR score) | 37.1 | 30.2 | 36.9 | 37.4 |
Depressive episode duration (months) | 24.6 | 30.4 | 21.3 | 25.9 |
N | % | N | % | |
Previous electroconvulsive therapy | 2 | 1.4 | 14 | 9.3 |
Comorbid anxiety | 85 | 59.0 | 83 | 55.0 |
Receiving psychotherapy during treatment | 59 | 41.0 | 65 | 43.0 |
Receiving pharmacotherapy during treatment | 127 | 88.2 | 123 | 81.5 |
Antidepressant | 120 | 83.3 | 115 | 76.2 |
Benzodiazepine | 48 | 33.3 | 54 | 35.8 |
Antipsychotic augmentation | 28 | 19.4 | 26 | 17.2 |
Lithium augmentation | 5 | 3.5 | 3 | 2.0 |
Anticonvulsant | 6 | 4.2 | 4 | 2.6 |
TABLE 1. Demographic and clinical sample characteristics in the repetitive transcranial magnetic stimulation (rTMS) and intermittent theta-burst stimulation (iTBS) groups of patients with major depressiona
MRI data processing.
All subjects had received a structural brain scan and two 10-minute single-echo resting-state fMRI scans (with the instruction to remain still, stay awake with their eyes closed, and avoid thinking about anything in particular) before and after treatment. MRI data acquisition parameters and scanner type (3T, GE HDx system, 8-channel coil, TR=2 seconds, 32 axial slices, thickness=5 mm) were identical for THREE-D subjects from both sites (Centre for Addiction and Mental Health [CAMH] and University Health Network [UHN]) included in our analyses (19). Acquisition parameters, structural and functional image processing, and denoising procedures are detailed in the Methods section of the online supplement. Unless otherwise specified, r values throughout the Results section represent Pearson correlation coefficients.
Investigating the Impact of Signal Properties on sgACC-StimFC
Effects of common signal-quality metrics (framewise displacement and temporal signal-to-noise ratio [tSNR] [24]) were tested through subsampling across percentiles of each metric. All 590 fMRI scans were independently rated for global signal fluctuations related to burst breathing and deep breaths by three study authors (J.D.P., I.G.E., and C.J.L.) using a 3-point scale indicating the certain presence (2 points), likely presence (1 point), or absence (0 points) of a pattern. The maximum possible score was 12 if all three raters were certain that a particular pattern was present on both scans.
Results
Validation of Imputed sgACC Signal via Weight Maps
One explanation for variable results in studies relating sgACC-StimFC to clinical outcome is that the sgACC signal is degraded and noisy because of its proximity to sinuses in the skull (22). To address this issue, less noisy sgACC signals can be obtained by using a “weight-map” method to impute sgACC signals (2). In brief, this method approximates the sgACC signal by substituting it with the weighted average of all nodes in a scan with time series that are correlated with the sgACC signal in 1,200 subjects from the Human Connectome Project (HCP) (see Figure S1 in the online supplement). We validated this approach in a data set of subjects scanned for up to 15 hours using multiecho fMRI (ME data set), a type of fMRI scan with greatly improved signal qualities in the sgACC (22). In these subjects, individual seed maps of the sgACC were highly reliable across scans (see Figure S3A in the online supplement). This reliability allowed us to construct a “ground truth” sgACC FC map for each subject with their concatenated data sets. The weight-map imputation of the sgACC closely resembled the ground truth seed maps (Figure 1A). In another public data set with 10 30-minute single-echo scans per subject (MSC data set), sgACC seed maps based on the concatenated data (5 hours) were very noisy and were unreliable from scan to scan; in contrast, weight-map approaches with these data produced reliable results in nearly all subjects, with obvious resemblance to the multiecho ground truth (see Figure 1B for representative subjects; see Figure S4 in the online supplement for the full sample). Between-subject variability was preserved with the weight-map method. The effects of sex and breathing patterns on the reliability and accuracy of the weight-map method were also assessed (see the online supplement). Collectively, these results lend strong support to the use of weight maps to impute sgACC signals and to construct reliable sgACC-StimFC maps.
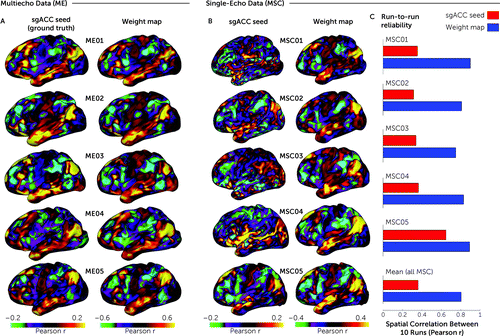
FIGURE 1. Reliability and accuracy of determining subgenual anterior cingulate cortex (sgACC) functional connectivity (FC) with the weight-map methoda
a We used multiecho fMRI data (ME) to investigate how accurately the weight-map method approximates true sgACC functional connectivity (FC). Panel A shows cortical surface maps depicting sgACC FC for five densely sampled subjects (ME01–ME05), each with up to 15 hours of concatenated multiband multiecho fMRI data. Because the simple sgACC seed yielded reliable FC maps in the concatenated multiecho fMRI data (22), we were able to test how accurately the FC maps derived from the weight-map approach (right column) approximated the “ground truth” sgACC seed-derived FC maps (left column). Qualitatively, there was a high correspondence between the FC maps generated by the two methods. The median spatial correlation (r) between the resulting maps for the two methods was 0.95. As shown in panel B, we next tested the performance of the weight-map method with single-echo fMRI data from the publicly available Midnight Scan Club (MSC) data set (21) that consists of data from 10 densely sampled individuals, each with 10 30-minute single-echo fMRI scans. Cortical surface maps depict FC for all MSC subjects from concatenated time series (5 hours) derived from either a simple sgACC seed (left column) or the weight-map method (right column). Maps derived from the sgACC seed were very noisy, whereas the weight-map method yielded FC maps with a consistent default mode network-like network configuration resembling the multiecho results. Note that the weight-map method produced higher absolute FC values with both the MSC and ME data sets. Panel C shows the test-retest reliability in terms of the mean spatial correlation (r) across each subject’s 10 fMRI sessions for a simple spherical sgACC seed (red) and the weight-map method (blue). Note the consistent improvement in reliability with the weight-map method across subjects, with a mean improvement from r=0.38 to r=0.81 for the total sample. Panels B and C show results for only five of 10 MSC subjects; results of the entire sample are shown in Figure S4 in the online supplement.
DLPFC Stimulation Modeling Detects Correlation With Treatment Outcomes
Another explanation for variability in relating clinical outcomes to sgACC-StimFC is uncertainty in modeling the stimulated tissue from which the DLPFC signal is composed. We compared two methods of estimating the stimulated DLPFC area in 295 subjects from the THREE-D trial (19) (Table 1). The first method is a heuristic that was often used in earlier studies (2–6, 8, 9) and amounts to a distance-weighted hemisphere (“weighted cone”). The second method involves a biophysical E-field model based on each individual subject’s anatomy and the stimulation parameters. Our primary analysis used the percent improvement in depressive symptoms as measured by the QIDS-SR after completion of the final TMS treatment session as defined in the THREE-D trial. Although the HAM-D was the primary outcome measure in the THREE-D study, our analyses focused on the QIDS-SR (for a rationale, see the “Clinical Outcome Measures” section in the online supplement), and supplemental analyses confirmed similar findings with the HAM-D, as described below.
There was no significant association between sgACC-StimFC and clinical improvement when a generic 12-mm weighted cone was used to approximate the stimulated portion of the DLPFC (Figure 2; see Figure S5 in the online supplement for a range of cone radius thresholds). In contrast, when using individual-specific E-field predictions to define the portion of the DLPFC that was most strongly stimulated (99th percentile of E-field), individual differences in treatment response were correlated with sgACC-StimFC (r=−0.16, p=0.006) (Figure 2). This was true across a range of E-field thresholds (see Figure S5 in the online supplement), in combined (Figure 2) and pretreatment fMRI scans, and after the inclusion of relevant covariates (including treatment condition and sex) with either the QIDS-SR or the HAM-D results as dependent variables (see Results section in the online supplement). No significant results were obtained when using a simple sgACC seed as opposed to the signal imputed from the weight-map approach (see Figure S6A in the online supplement) or when the global cortical signal—removed in previously published positive reports (3, 5, 6, 8)—was not removed (see Figure S6B in the online supplement). Likewise, no association was found when the spatial distance to the most negative sgACC FC site (“optimal site”) was used as a predictor instead of FC strength, which is an approach proposed in recent studies (6, 9) (see Figure S6C in the online supplement).
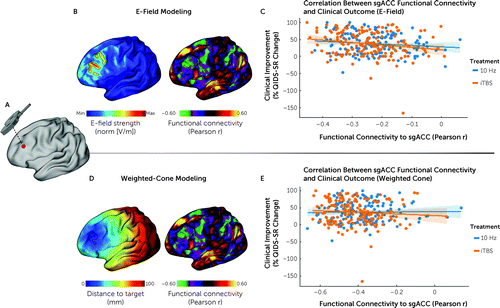
FIGURE 2. Subgenual anterior cingulate cortex (sgACC) functional connectivity (FC) with the stimulation site predicts individual differences in treatment outcomesa
a Panels A–B show in a representative subject how the individual rTMS stimulation coordinates on an inflated cortical surface (panel A) relate to the relative distribution of the induced electric field (E-field) (panel B, image at left), with the cortical area receiving the 99th percentile strongest E-field outlined in black. The image at right in panel B illustrates how the stimulated area of the dorsolateral prefrontal cortex (DLPFC) (with the 99th percentile strongest E-field outlined in black) maps onto the same subject’s sgACC FC map (derived with the weight-map approach [2, 31]). As shown in panel C, sgACC FC with the stimulation site as estimated by E-field modeling was negatively correlated with treatment outcomes (% improvement in total score on the 16-item Quick Inventory of Depressive Symptomatology [QIDS-SR]) (r=−0.16, p=0.006). Panels D and E show the corresponding results for the weighted-cone approach, in which a 12-mm distance-weighted hemisphere around the target coordinate is used to average FC features (2). Panel D (image at left) illustrates the borders of a 12-mm weighted cone on the inflated cortex, where the distance to the stimulation target site is color coded, and shows results markedly different from those of the projected E-field estimation. The apparent asymmetry of the radius is due to the projection from a folded cortex to a flattened cortex. The image at right in panel D illustrates how the 12-mm weighted cone maps to the sgACC-StimFC within the DLPFC. As shown in panel E, sgACC FC with the stimulation site as estimated by the weighted-cone approach was not associated with treatment outcomes (r=−0.03, p=0.56); x-axis values denote weighted averages.
In summary, a modest association between sgACC-StimFC and treatment outcome was found only when sgACC FC and the stimulated DLPFC area were carefully modeled. For the above analyses, data across treatment conditions and both of a subject’s fMRI scans were collapsed to increase the power to detect a true effect through sample size. This was supported by the absence of rTMS treatment modality effects on sgACC-StimFC (minimal pFDR=0.29), which was consistent with previous reports (6). However, when analyzed separately, effect sizes were similar across scans (pretreatment scans: r=−0.14; posttreatment scans: r=−0.14), across treatment conditions (iTBS group: r=−0.19; 10 Hz rTMS group: r=−0.13), and across study sites (CAMH sample: r=−0.18; UHN sample: r=−0.13).
To better understand whether the functional connections of the stimulation site to areas other than the sgACC may provide additional treatment prediction, we extended our analysis to the entire brain, using a previously validated multimodal brain parcellation (25). FC of each parcel with the stimulation site was derived by the same weight-map method detailed above for the sgACC analysis. The neuroanatomical distribution and network affiliation of all parcels and the correlation between FC and clinical improvement are depicted in Figure S7 in the online supplement. Seventy parcels showed a nominally significant correlation; the majority of negative correlations mapped onto the default mode network, and the majority of positive correlations mapped onto the cingulo-opercular network and dorsal attention network. Overall, correlations with the left and right sgACC parcels were among the strongest correlations (r=−0.18, rank 4/360, and r=−0.16, rank 18/360, respectively). Taken together, these results indicate that the association of the sgACC with clinical improvement is not a focal property of this brain region but is part of a larger FC signature that involves the default mode network and its anticorrelated networks.
Small Samples Lead to Overestimation and Underestimation of the Whole-Sample Effect
As noted above, previous smaller studies have reported considerably stronger associations between sgACC-StimFC and treatment outcome than the associations reported here. To understand whether sample size limitations might partially explain these conflicting results, we evaluated the impact of sample size on effect size estimates with the THREE-D data set by analyzing the correlation between treatment outcome and sgACC-StimFC in 10,000 random draws for sample sizes ranging from 15 to 280 patients. This analysis revealed substantially greater effect size variance in smaller than larger subsamples, with a wide range of effect sizes at a sample size of 25, which is the median sample size in previous studies (Figure 3A). Even with 50 patients, effects of r=−0.50 or larger were commonly observed. These results suggest that sample sizes alone can account for apparently conflicting results.
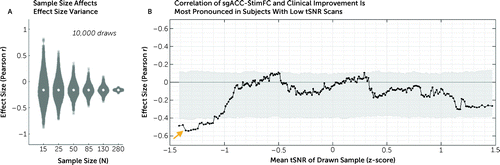
FIGURE 3. Sources of effect size variancea
a Panel A shows the effect of sample size on effect size variance in 10,000 bootstrapped analyses per sample size. In samples of 25 subjects, the median sample size in previous studies, effect sizes (r) of −0.5 are often found by chance. As shown in panel B, functional connectivity of the stimulated site with the sgACC (sgACC-StimFC) is highly predictive of clinical improvement in the subset of subjects with the lowest temporal signal-to-noise ratio (tSNR) (orange arrow). For this analysis, subjects were first ordered by z-scored tSNR values. Each marker represents the correlation strength between sgACC-StimFC and clinical improvement in a subsample of 50 subjects. Samples were then drawn in ascending order, based on tSNR values in an overlapping sliding window manner. Gray shading represents 95% of the null distribution of r values in 10,000 random draws of 50 subjects. No dependence of the effect on movement (mean framewise displacement) was found (see Results section in the online supplement).
sgACC-StimFC Predicts Treatment Outcomes in Patients With Large Global Signal Fluctuations
Another explanation for variability across studies is data quality (24). We tested this by first sorting subjects by the tSNR (mean intensity over the variance in a scan) of their scans. We then computed the effect of sgACC-StimFC on clinical improvement in overlapping subsamples of 50 subjects, going from subjects with the lowest to the highest tSNR values. Unexpectedly, the association between sgACC-StimFC and treatment outcome was strongest in subjects with the lowest tSNR values (Figure 3B). No such effect was found when subjects were sorted by framewise displacement values (see Figure S8 in the online supplement). These results indicate that the effect is accentuated in seemingly lower-quality data. The remainder of this section details our efforts to understand what this subpopulation might represent.
We first disentangled the signal characteristics underlying tSNR in our data and found it to be mainly determined by differences in signal variance (Figure 4A). One major source of variance in fMRI signals stems from common irregular breathing patterns involving deep breaths and burst breathing, which were recently linked to specific blood-oxygen-level-dependent (BOLD) signals (20). While deep breaths cause isolated bands of global BOLD signal fluctuations, burst breathing—characterized by cycles of spindle-like increases and decreases in respiratory depth—produces repeated, serial bands of time-lagged brain-wide BOLD signals. These signals are easily quantifiable when the fMRI time series is visualized as a two-dimensional gray-scaled plot, with cortical nodes as rows and time as columns (“carpet-plot” format). For details on scoring, and a detailed explanation of the carpet-plot format, see Figure S9 in the online supplement and (20). Using the carpet-plot format, three blinded raters (J.D.P., C.J.L., and I.G.E.) scored the BOLD signal time series of all 295 subjects (590 fMRI scans) for global signal patterns indicative of burst breathing and deep breaths (Figure 4B). Ratings were highly similar across raters (Cohen’s kappa values ranged from 0.59 to 0.75) (Figure 4C, left panel) and replicated previously observed sex biases (20) (Figure 4C, right panel). Together, these results validate our approach to identifying global signal signatures that have been previously linked to two forms of irregular breathing.
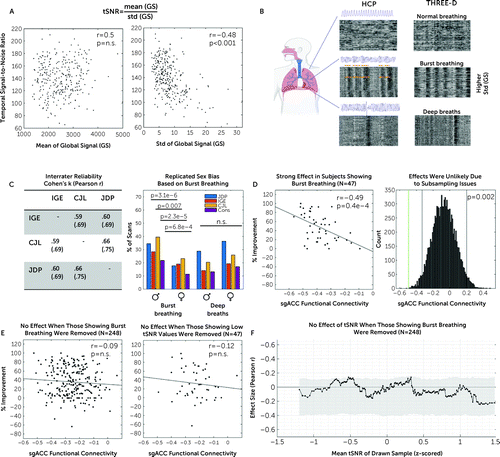
FIGURE 4. Association between functional connectivity of the stimulated site with the subgenual anterior cingulate cortex (sgACC-StimFC) and clinical improvement is carried by a subpopulation of subjects with high breathing-specific signal variancea
a As shown in panel A, low temporal signal-to-noise ratio (tSNR) in our sample was explained by differences in signal variance (image at right) as opposed to differences in mean intensity of the fMRI signal (image at left). Panel B shows that a major source of variance in the global fMRI signal stems from breathing (20). Three blinded raters scored all 590 fMRI scans (two per subject) for the presence of two common irregular breathing patterns: burst breathing and deep breaths. Representative examples of carpet plots (rows are voxels, and columns are time points; intensity represents signal strength) are shown for normal breathing, burst breathing, and deep breaths of individuals included in the Human Connectome Project (HCP) data set (used and reproduced from [20]) and the THREE-D data set. Note the strong correspondence of characteristic patterns that are clearly identifiable without a breathing-belt trace. Panel C shows the high interrater reliability that was obtained between each pair of raters for each of the breathing patterns (Cohen’s kappa and r; table at left). A sex bias was found for the occurrence of burst breathing but not deep breaths (figure at right), which exactly replicated a previous study (20). Panel D shows that among the 47 subjects showing signal fluctuations indicative of burst breathing, sgACC-StimFC was highly predictive of clinical improvement (% improvement on the 16-item Quick Inventory of Depressive Symptomatology [QIDS-SR]) (figure at left). The probability of finding an effect of this strength in a random subsample of 47 subjects was p=0.002 in 10,000 bootstrapped subsamples. Gray vertical bars present nominally significant effect sizes, and the green vertical bar marks the actual effect size (figure at right). Panel E shows that when these 47 subjects with signal fluctuations indicative of burst breathing were removed from the total sample, no significant association between sgACC-StimFC and clinical improvement remained (figure at left). The strong association was specific to samples that included those showing burst breathing and not present in a control sample of 47 subjects with equally low tSNR values (105.56 vs. 117.48 in those showing burst breathing) that did not show evidence for burst breathing (figure at right). Conversely, when this control sample was removed from the total sample, the association between sgACC-StimFC and clinical improvement was unchanged (r=−0.14, p=0.03; data not shown). As shown in panel F, the dependency of the association between sgACC-StimFC and clinical improvement on tSNR was removed in the absence of the 47 subjects with evidence for burst breathing, indicating that this phenomenon was driven by the presence of individuals showing burst breathing in the sample rather than being a consequence of nonspecific sources of high signal variance. One subject with a QIDS-SR improvement of −150% was omitted for illustrative purposes; the subject was included in all test statistics.
Comparisons between subjects with strong evidence for burst breathing (N=47) relative to the remainder of the sample (N=248) revealed several interesting observations. First, global signal patterns indicative of burst breathing were associated with lower tSNR, as hypothesized, given the increased BOLD signal variance induced by bursting (see Figure S10 in the online supplement). Second, among the subjects displaying global signal patterns related to burst breathing, sgACC-StimFC was strongly correlated with clinical improvement (r=−0.49, p=0.0004) (Figure 4D, left panel) (see Figure S11 in the online supplement for a range of cutoff values to assess burst certainty). The probability of drawing a subsample of that size with an effect this strong by chance is low (p=0.002 from 10,000 samples selected at random without replacement) (Figure 4D, right panel). Moreover, randomly subsampling subjects across percentiles of bursting scores showed the effect to increase as a function of a sample’s bursting score (see Figure S12 in the online supplement). Third, when the subsample of 47 subjects displaying global signal fluctuations reflecting burst breathing was removed, the association between sgACC-StimFC and clinical improvement was clearly weakened in the remaining sample (N=248, r=−0.09, p=0.32) (Figure 4E, left panel). These effects were specific to subjects with evidence of bursting and not a common property of subjects with low tSNR scans: a control group of 47 subjects with equally low tSNR (105.56 vs. 117.48 in those showing burst breathing) but no evidence of burst breathing did not show an accentuated effect (N=47, r=−0.12, p=0.42) (Figure 4E, right panel). Lastly, the dependency of the effect on tSNR was removed with the absence of the 47 subjects showing evidence of burst breathing (Figure 4F), indicating that this phenomenon was driven by the presence of burst breathing in the sample rather than being a consequence of nonspecific sources of high signal variance. Taken together, these results indicate that the link between sgACC-StimFC and clinical improvement in our sample is explained by a specific subset of subjects with global signal fluctuations that have previously been linked to burst breathing.
Discussion
Here, in a large rTMS data set, we found a relationship between sgACC-StimFC and treatment outcome, as previously reported. The effect we observed was substantially smaller than that described in several previous reports and accounted for only 3% of treatment response variance, hence calling into question the utility of this marker in its current form. Furthermore, this relationship was obtained only when weight maps were used to impute otherwise noisy sgACC FC, when the stimulated part of the DLPFC was estimated with E-field modeling, and when the sample included certain subjects, namely, those with low tSNR due to high signal fluctuations that have been specifically linked to irregular breathing. The interpretation of these findings is multifaceted. On the one hand, the association between sgACC-StimFC and treatment outcomes was modest in this sample and was contingent on certain choices. On the other hand, the effect was strengthened by more accurate modeling approaches, and it was robust to the partitioning of the data, robust across measurement scales, and robust to the inclusion of covariates. Furthermore, it was concentrated in a particular set of subjects who display identifiable characteristics. Our interpretation is at once tempered and hopeful: striking, strong linkages of FC to outcome in a large sample were not found, but the concentration of these linkages in a subpopulation suggests potential avenues toward understanding who is responsive to treatment and why.
Unexpectedly, the predictive value of sgACC-StimFC was strongest in patients with patterns of global signal variance associated with burst breathing. One possible explanation derives from the recent observation that burst breathing coincides with high-amplitude BOLD events that are time-lagged between networks (20). The spatiotemporal structure of these time-lagged events is clearly revealed after removing CO2-related global signal fluctuations and is such that it accentuates negative FC between the default mode network (of which the sgACC is commonly a part) and networks represented in the DLPFC (salience and frontoparietal networks). Consistent with this notion, subjects with prominent burst breathing had more negative sgACC-StimFC (see Figure S10B in the online supplement), and omission of global signal regression removed the observed effect (see Figure S6B in the online supplement). Hence, the anticorrelation in FC between the stimulation site and the sgACC might be an indirect index of what intrinsic networks have been engaged by TMS, which might be the true mediator of the effect. This would be aligned with a rich literature linking individually variable functional network topology to various behavioral domains (21, 26, 27). Future work might elucidate the utility of these more comprehensive measures of functional brain organization that are only obtainable from high-quality data, such as multiecho fMRI scans. Alternatively, a propensity for burst-like respiration may differentiate a subsample of depressed patients for whom sgACC FC strongly mediates rTMS outcomes. We did not find strong evidence in the MSC and ME data for burst breathing having an impact on the validity of the weight-map method, besides a minimally better run-to-run reliability in male versus female MSC subjects (which could be indirect evidence, as burst breathing is more prevalent in males; see Results section in the online supplement). If reliability is increased in subjects with burst breathing, this could increase the power to detect a true effect in these subjects. All of these factors may contribute to the effect. It is also possible that burst breathing may be influenced by somnolence or fluctuating levels of arousal during the scan. However, at present, there is no evidence linking burst breathing to low vigilance or somnolence, and several observations in the HCP data speak against it (20), rendering vigilance an unlikely mediator of these effects.
With regard to data quality, it should be noted that the mean tSNR values were high for BOLD fMRI data, which was likely due to the somewhat larger-than-average voxel size. In terms of acquisition parameters, the THREE-D data compare favorably to data from previous studies (5, 6, 9, 16–18), with the shortest TR (2 seconds) and second-longest acquisition time (total of 20 minutes) among those studies. These factors render data quality an unlikely candidate to explain effect size discrepancies between our findings and those of previous studies.
We discuss other sources of heterogeneity that potentially contribute to the discrepant effect sizes between those of our study and previous studies in the Discussion section in the online supplement. One possible source of heterogeneity is the study population: major depressive disorder is not a unitary disease entity, and sgACC-StimFC might be more relevant for predicting rTMS outcome in particular subpopulations with major depressive disorder. The THREE-D sample comprised patients with major depressive disorder and a relatively high rate of comorbid anxiety and polypharmacy (19), as seen in naturalistic samples. However, previous reports of large effect sizes also included populations with similarly high rates of comorbid anxiety and polypharmacy (5, 6), indicating that these variables alone are unlikely to explain the small effect we observed. The effect size we report (r=−0.16) represents a “best-case scenario” in our sample, where data across scans and treatment conditions were concatenated to increase power, and results with the QIDS-SR, which yielded stronger effects than the HAM-D, were used. Consequently, the small effect size might itself be inflated and likely represents the upper bound of a true effect obtainable in this data set.
Several limitations should be noted. First, like all previous studies, this was a retrospective analysis as opposed to a prospective analysis with a preformulated analysis pipeline. Second, subjects in the THREE-D study received either 10 Hz rTMS or iTBS, while previous studies of sgACC FC investigated the effects with 10 Hz rTMS only. However, we found a similar effect in both conditions, which suggests that our conclusions apply to both 10 Hz rTMS and iTBS treatment modalities. Third, like other studies of sgACC FC and rTMS, the THREE-D study did not include a sham control condition. Both the sgACC and DLPFC have been implicated in placebo responses (28, 29), and our observation of a modest correlation between symptom improvement and sgACC-StimFC might be attributable to treatment responses, placebo responses, or both. The DLPFC, in particular, has shown consistent involvement in placebo antidepressant responses across metabolic, perfusion-based, and fMRI studies (30). On the other hand, we also found that accurate E-field modeling strengthened the association between sgACC-StimFC and outcome, which may point to a treatment effect. E-field modeling of the stimulation site decreases variance by sampling FC features that were stimulated with TMS but increases subject-to-subject variance with respect to which FC features are being averaged within the DLPFC (i.e., the chosen features depend on each subject’s unique E-field distribution and shape). Since the neuroanatomical substrate of a placebo response is unlikely to move from subject to subject in line with their E-field, we interpret this as supporting evidence for a TMS treatment effect and not just a placebo response. Fourth, neither our study nor earlier ones included pupillometric or electrophysiological monitoring of vigilance, which can have marked confounding effects on the global signal and FC. A further limitation was the lack of respiratory belt traces in the THREE-D data. However, bursting signal patterns closely resembled previously reported patterns in the HCP data (Figure 4B), and we exactly replicated a burst breathing–specific sex bias previously reported in the HCP data (20), which is supportive evidence for the correct identification of irregular breathing patterns in the THREE-D data set (Figure 4C). Lastly, the validity of our results—and those of previous studies—rests on the accurate identification of the stimulated cortex, which is an active area of research (23). We used a biophysical E-field model that is, to our knowledge, the primary method for estimating the spatial distribution of TMS effects. However, the extent to which this model accurately predicts neuronal populations modulated by rTMS is still partially unknown, and future models might yield better approximations. Additional discussion of alternative explanations of our findings associated with burst breathing, our effect size estimate, and analytical choices is provided in the Discussion section in the online supplement.
Together, these results suggest the need to reevaluate the utility of current sgACC FC-based targeting approaches for rTMS and elucidate relevant sources of variability that might have led to mixed results in previous studies. At the same time, these results provide strong evidence for the existence of a true—albeit weak—association. This highlights the need to further explore fMRI predictors for rTMS targeting, possibly involving additional network measures and fMRI data types. Methodological improvements, including multiecho fMRI and dense-sampling approaches, have already shown promise for enabling more comprehensive and personalized characterizations of functional brain organization that could be used to make better treatment predictions in the future. Prospective clinical trials designed to evaluate the clinical utility of fMRI-guided targeting strategies in naturalistic, real-world settings will be critical.
1. : Effectiveness of transcranial magnetic stimulation in clinical practice post-FDA approval in the United States: results observed with the first 100 consecutive cases of depression at an academic medical center. J Clin Psychiatry 2012; 73:e567–e573Crossref, Medline, Google Scholar
2. : Identification of reproducible individualized targets for treatment of depression with TMS based on intrinsic connectivity. Neuroimage 2013; 66:151–160Crossref, Medline, Google Scholar
3. : Efficacy of transcranial magnetic stimulation targets for depression is related to intrinsic functional connectivity with the subgenual cingulate. Biol Psychiatry 2012; 72:595–603Crossref, Medline, Google Scholar
4. : Distinct symptom-specific treatment targets for circuit-based neuromodulation. Am J Psychiatry 2020; 177:435–446Link, Google Scholar
5. : Subgenual functional connectivity predicts antidepressant treatment response to transcranial magnetic stimulation: independent validation and evaluation of personalization. Biol Psychiatry 2019; 86:e5–e7Crossref, Medline, Google Scholar
6. : Functional magnetic resonance imaging-guided personalization of transcranial magnetic stimulation treatment for depression. JAMA Psychiatry 2021; 78:337–339Crossref, Medline, Google Scholar
7. : Stanford Accelerated Intelligent Neuromodulation Therapy for treatment-resistant depression. Am J Psychiatry 2020; 177:716–726Link, Google Scholar
8. : Prospective validation that subgenual connectivity predicts antidepressant efficacy of transcranial magnetic stimulation sites. Biol Psychiatry 2018; 84:28–37Crossref, Medline, Google Scholar
9. : Identification of personalized transcranial magnetic stimulation targets based on subgenual cingulate connectivity: an independent replication. Biol Psychiatry 2021; 90:e55–e56Crossref, Medline, Google Scholar
10. : Combining transcranial magnetic stimulation with functional magnetic resonance imaging for probing and modulating neural circuits relevant to affective disorders. Wiley Interdiscip Rev Cogn Sci 2021; 12:e1553Crossref, Medline, Google Scholar
11. : The subgenual anterior cingulate cortex in mood disorders. CNS Spectr 2008; 13:663–681Crossref, Medline, Google Scholar
12. : Deep brain stimulation for treatment-resistant depression. Neuron 2005; 45:651–660Crossref, Medline, Google Scholar
13. : Frontocingulate dysfunction in depression: toward biomarkers of treatment response. Neuropsychopharmacology 2011; 36:183–206Crossref, Medline, Google Scholar
14. : Depression: perspectives from affective neuroscience. Annu Rev Psychol 2002; 53:545–574Crossref, Medline, Google Scholar
15. : Accelerated HF-rTMS in treatment-resistant unipolar depression: insights from subgenual anterior cingulate functional connectivity. World J Biol Psychiatry 2014; 15:286–297Crossref, Medline, Google Scholar
16. : Functional connectivity of the anterior cingulate cortex predicts treatment outcome for rTMS in treatment-resistant depression at 3-month follow-up. Brain Stimul 2020; 13:206–214Crossref, Medline, Google Scholar
17. : Personalized prediction of transcranial magnetic stimulation clinical response in patients with treatment-refractory depression using neuroimaging biomarkers and machine learning. J Affect Disord 2021; 290:261–271Crossref, Medline, Google Scholar
18. : Network mechanisms of clinical response to transcranial magnetic stimulation in posttraumatic stress disorder and major depressive disorder. Biol Psychiatry 2018; 83:263–272Crossref, Medline, Google Scholar
19. : Effectiveness of theta burst versus high-frequency repetitive transcranial magnetic stimulation in patients with depression (THREE-D): a randomised non-inferiority trial. Lancet 2018; 391:1683–1692Crossref, Medline, Google Scholar
20. : Prevalent and sex-biased breathing patterns modify functional connectivity MRI in young adults. Nat Commun 2020; 11:5290Crossref, Medline, Google Scholar
21. : Precision functional mapping of individual human brains. Neuron 2017; 95:791–807Crossref, Medline, Google Scholar
22. : Rapid precision functional mapping of individuals using multi-echo fMRI. Cell Rep 2020; 33:108540Crossref, Medline, Google Scholar
23. : Field modeling for transcranial magnetic stimulation: a useful tool to understand the physiological effects of TMS? Annu Int Conf IEEE Eng Med Biol Soc 2015; 2015:222–225Medline, Google Scholar
24. : Recent progress and outstanding issues in motion correction in resting state fMRI. Neuroimage 2015; 105:536–551Crossref, Medline, Google Scholar
25. : A multi-modal parcellation of human cerebral cortex. Nature 2016; 536:171–178Crossref, Medline, Google Scholar
26. : Plasticity and spontaneous activity pulses in disused human brain circuits. Neuron 2020; 107:580–589Crossref, Medline, Google Scholar
27. : Individual-specific functional connectivity of the amygdala: a substrate for precision psychiatry. Proc Natl Acad Sci U S A 2020; 117:3808–3818Crossref, Medline, Google Scholar
28. : Neurobiological mechanisms of the placebo effect. J Neurosci 2005; 25:10390–10402Crossref, Medline, Google Scholar
29. : Meta-analysis of neural systems underlying placebo analgesia from individual participant fMRI data. Nat Commun 2021; 12:1391Crossref, Medline, Google Scholar
30. : Functional neuroimaging correlates of placebo response in patients with depressive or anxiety disorders: a systematic review. Int J Neuropsychopharmacol 2022; 25:433–447Crossref, Medline, Google Scholar
31. : Using brain imaging to improve spatial targeting of transcranial magnetic stimulation for depression. Biol Psychiatry 2021; 90:689–700Crossref, Medline, Google Scholar