Patient Casemix Classification for Medicare Psychiatric Prospective Payment
Abstract
Objective: For a proposed Medicare prospective payment system for inpatient psychiatric facility treatment, the authors developed a casemix classification to capture differences in patients’ real daily resource use. Method: Primary data on patient characteristics and daily time spent in various activities were collected in a survey of 696 patients from 40 inpatient psychiatric facilities. Survey data were combined with Medicare claims data to estimate intensity-adjusted daily cost. Classification and Regression Trees (CART) analysis of average daily routine and ancillary costs yielded several hierarchical classification groupings. Regression analysis was used to control for facility and day-of-stay effects in order to compare hierarchical models with models based on the recently proposed payment system of the Centers for Medicare & Medicaid Services. Results: CART analysis identified a small set of patient characteristics strongly associated with higher daily costs, including age, psychiatric diagnosis, deficits in daily living activities, and detox or ECT use. A parsimonious, 16-group, fully interactive model that used five major DSM-IV categories and stratified by age, illness severity, deficits in daily living activities, dangerousness, and use of ECT explained 40% (out of a possible 76%) of daily cost variation not attributable to idiosyncratic daily changes within patients. A noninteractive model based on diagnosis-related groups, age, and medical comorbidity had explanatory power of only 32%. Conclusions: A regression model with 16 casemix groups restricted to using “appropriate” payment variables (i.e., those with clinical face validity and low administrative burden that are easily validated and provide proper care incentives) produced more efficient and equitable payments than did a noninteractive system based on diagnosis-related groups.
The Balanced Budget Refinement Act of 1999 mandated that the Centers for Medicare & Medicaid Services (CMS) develop a per diem prospective payment system for inpatient psychiatric facility care. These facilities are currently exempt from the Medicare prospective payment system. In particular, the new system for inpatient psychiatric facility care is to include “an adequate patient classification system that reflects the differences in patient resource use among such hospitals and shall maintain budget neutrality” (1) . The current cost-based system for facilities exempt from the prospective payment system has no casemix adjustments and has come under heightened criticism as inefficient and inequitable (2 – 5) . In November 2003, CMS proposed using several psychiatric and substance use diagnosis-related groups in conjunction with age and several medical comorbidity subgroups to classify inpatient psychiatric facility patients for payment (6) . The final rule, published in November 2004, refined the proposed prospective payment system to include an adjustment for ECT use (7) . Unfortunately, these diagnosis-related groups have remained essentially unchanged since 1984, and the organization of diagnostic codes does not follow DSM-IV classification of psychiatric disorders.
Over two decades of research has identified several factors driving inpatient mental health costs. Earlier research focused on length of stay and diagnosis-related groups to explain per-case cost differences (8 – 11) . The use of claims-based characteristics only marginally improved the explanatory power of diagnosis-related groups. Later studies found that degree of social support (12) , assistance with activities of daily living (13) , illness severity (13) , legal status and referral source (14) , and dangerous behavior (15) enhanced the predictive power of models for length of stay and cost. A previous article by Lave (5) summarized lessons from these studies.
It is now clear that claims-based classification systems, calibrated using existing administrative data, cannot adequately capture true cost differences. About 85% of Medicare psychiatric inpatient costs are incurred in routine, rather than ancillary, cost centers (16) . Claims-based analyses, which must assume a uniform per diem routine cost across all patients in a facility, cannot explain variation in this major contributor to patient cost. Potentially high-cost patients with behavioral problems are overlooked, and their true cost will be underestimated. A high-cost outlier payment could address some of the problem but still underestimates differences in routine costs.
The aims of this study were to 1) collect primary data on daily, patient-specific, routine staffing intensity for a nationwide sample of patients; 2) collect corresponding information from medical records on patient diagnostic, demographic, and physical and mental status; and 3) supplement these datasets with claims that capture ancillary and overhead costs in order to develop a casemix classification for a per diem Medicare prospective payment system for inpatient psychiatric facility care.
Method
Primary Data Collection
The unique primary data used in this study came from a national sample of inpatient psychiatric facilities. The observational unit was the 24-hour patient day. For a 7-day period at each participating facility, data on patient and staff time spent in various activities were collected. These data were linked to information from medical records that provided detailed demographic, diagnostic (psychiatric and medical), and behavioral information. A stratified multistage sampling design was employed, with facilities as primary sampling units and units and patients as subsamples. The sample frame consisted of 1,846 inpatient psychiatric facilities with one or more units exempt from prospective payment systems and was stratified by the nine Census divisions to ensure the geographical representativeness of our results. Probability proportional to size sampling (based on each facility’s Medicare-covered psychiatric days) was used to select a facility sample. Facilities with fewer than 10 beds were excluded. Overall, 40 facilities with a total of 65 units (general adult [N=37], geriatric [N=15], consultation/liaison [N=4], forensic [N=1], other [N=8]) were included in the sample. Most (N=42) of the sampled units were in acute general hospitals. The remaining 23 units were split between private (N=17) and public (N=6) psychiatric hospitals. Between 1 and 7 days of data were available per patient. Sampling weights were developed to account for differing sampling proportions (17) .
Direct observation by the study team was infeasible because of patient confidentiality concerns and disruptions to patient care. Instead, nursing staff on day, evening, and night shifts were trained to complete the data collection instruments. A site coordinator, usually a nurse, was trained intensively to provide future staff trainings, manage data collection, and ensure quality control and patient confidentiality.
Three forms captured routine staff and patient time spent in activities on and off the unit during each 8-hour shift. Site coordinators checked forms daily for completeness and accuracy. On one form unit staff reported their group and milieu activities. A second form recorded very time-intensive activities with individual patients (e.g., one-to-one assigned observation). Nurses and mental health specialists also completed a third form that tracked time each of their assigned patients spent in each activity. Nonunit staff time for individual patients (e.g., consulting with physicians, lab technicians) was recorded in a log.
A fourth form, collected once for each Medicare patient at the end of the study or at discharge, recorded demographic data and information about behavioral and other characteristics (e.g., suicidality, legal status) for every Medicare-eligible patient. It also included all five DSM-IV axes.
Additional Data Sources
National claims data from Medicare inpatient files for 2001 and 2002 were matched to 696 patients by their Medicare ID number and dates of service. The claims were supplemented with Medicare cost report information on routine stepped-down costs and average length of stay. Ancillary service charges incurred during the stay were converted into costs by using facility average cost-to-charge ratios.
Resource Intensity and Routine Cost
Individual staff time spent with patients daily was weighted by each occupation’s nurse-relative hourly wage to produce a patient-specific resource intensity measure. The occupational relative weights vary from 0.5 for mental health specialists to over 3.6 for psychiatrists. Because the prospective payment system covers only hospital services, only psychiatrists’ administrative time is included in this analysis. Weighting by relative wages produces a resource intensity measure that is unaffected by geographic and provider wage differences, making it a measure of real resource use more comparable across facilities.
Patients’ daily routine cost was computed by dividing their resource intensity for each day by the average resource intensity for all Medicare study days in the facility then multiplying by the facility’s average (constant) routine per diem cost. Consequently, patient days that are twice as staff intensive as the facility’s average day are assumed to have twice the routine cost. The total cost for each patient day is the sum of two components: the routine and the average ancillary per diem costs.
Diagnostic Characteristics
The psychiatric domain included principal diagnosis plus mania indicator, illness severity, and Global Assessment of Functioning score. Axis I principal diagnoses were subdivided into five categories: schizophrenia and other psychotic disorders, dementias and delirium, mood disorders, substance-related disorders, and “residual” (including eating disorders, posttraumatic stress disorders, anxiety disorders). Study clinicians developed a list of 26 severe psychiatric conditions likely to be resource-intensive (available upon request). These included all five-digit DSM-IV codes with “severe,” “profound,” or “pervasive” qualifiers. Additional codes were identified by ranking all potentially severe diagnoses by average daily resource intensity and including those with above-average intensity (e.g., impulse control and borderline personality disorder). Dual diagnosis patients included patients with a principal psychiatric diagnosis complicated by a primary substance use disorder and those with substance use disorders with a complicating psychiatric diagnosis.
The physical and medical domain was constructed analogously to the complicating conditions in the current inpatient prospective payment system. Clinical staff identified a list of nursing-intensive conditions (e.g., insulin-dependent diabetes and nonhealing wounds; the list is available upon request). Patient age, deficits in daily living activities, and any history of falls were also indicators of patients’ physical needs.
Other Patient Characteristics
The behavioral domain measured behavioral aspects of psychiatric functioning. Behaviors during the stay affecting resource intensity included four indicators of safety risk: suicidal (hopeless, wanted to kill self ASAP, made recent attempts), assaultive (ratings of “most severe” for level of physical aggression, lethality of threats, or agitation), likelihood of elopement (those described as a “serious elopement threat” by clinical staff), or self-neglecting (exhibiting behavior identified by clinical staff as “extreme self-neglect” [e.g., not eating]). It also included whether the patient required one-to-one observation or hourly attention beyond routine monitoring for most of the day. Other indicators included treatment compliance, disruptiveness, and cognitive impairment.
Actual care and treatment regimens, as well as various factors present at admission, also affect staff intensity. Treatment indicators included number of medications at time of discharge or end of study and whether detox, ECT, short- or long-term intravenous lines, glucose monitoring, wound care, or neurological checks were required. Status indicators upon admission included gender plus residence prior to admission (e.g., nursing home), “first break” (first psychiatric admission), and commitment type (voluntary or involuntary).
Analysis
Classification system development proceeded in two stages. First, several hierarchical classification models of patients’ average daily institutional costs were constructed by using version 4.0 of the CART (Classification and Regression Trees) software (18 , 19) . The patient was the unit of analysis for the CART analyses, since very few patient characteristics vary daily. In CART analysis, the patient sample is progressively split according to characteristic values that best separate patients into homogeneous groups with respect to per diem cost. The sample is first split into two groups on the basis of the characteristic that best separates high-cost and low-cost patients. Each resulting subgroup can then be split into further subgroups according to (generally different) characteristics that next best divide patients by average cost. The process continues until the best split of each subgroup would be statistically nonsignificant.
Second, linear regression models of per diem costs were estimated. The unit of analysis in this second stage of analysis was the patient day. Per diem cost exhibited marked skewness, and a log transform of cost was used in all regression models. Explanatory variables, besides CART classification group indicators, included day of stay groups, a weekend indicator, facility ownership, teaching status, size, urbanicity, area hospital wage rates, occupancy rate, and Medicare disproportional share ratio. The day-of-stay indicators permit the estimation of “declining block pricing” rates (5 , 10 , 20 , 21) .
Three patient classification systems were created with CART using different sets of explanatory variables. Inclusion of explanatory variables in the three models was based on the ability to explain variation in per diem cost and on “appropriateness” for a payment system (i.e., clinical face validity, easily validated, low administrative burden, and providing proper care incentives to providers).
One CART-based model was the benchmark for comparing the impact of restricting to “appropriate” explanatory variables. This “all characteristics” model featured 74 groups constructed with CART using all 30 available casemix variables, including some inappropriate for payment. The other two CART-based models (“principal characteristics” models) retained a limited set of characteristics yielding strong, clinically consistent results. One model excluded ECT and dangerousness, since their use in a payment system may be controversial. From the larger set of CART groups, a more parsimonious group was derived by collapsing categories with similar regression coefficients.
Four other regression models were estimated for comparison purposes. Three models were based on the CMS proposed rule (6) that included day of stay and facility characteristics, patient age and medical comorbidity, and diagnosis-related groups (the final rule was not available for these analyses). Some uncommon diagnosis-related groups and several rare comorbid conditions in the CMS model were not present in our sample. To avoid overfitting, the 17 comorbid conditions in the CMS proposed rule model were aggregated into three groups. The fourth model used patient fixed effects to represent the maximum power of any patient-based model in explaining daily cost variation.
Because of the complex sample design, the standard error estimates of all regression coefficients were adjusted using the Taylor linearization method (22) . Casemix classification significance (gain in R 2 ) was tested with type III sum of squared residuals adjusting for the complex sample design (23) . Statistical tests of estimated relative weights (against a null hypothesis of 1.0) used the “delta method” for a Wald test of nonlinear restrictions (24) .
Results
CART Casemix Classification
For the all characteristics benchmark model ( Figure 1 ), the most powerful split was by age (under 65 versus 65 and over) followed by either major diagnosis (for those under age 65) or one-to-one versus no observation (for those 65 and over). Splits to the right in Figure 1 are always more costly. Nonelderly patients with schizophrenia or substance-related disorders (208 patients) were further split by whether or not they had legal problems. Patients without legal problems were further split by whether or not they required one-to-one observation and if not then by medical condition severity. Nonelderly patients with dementia, mood disorders, and residual diagnoses were first split by whether or not they required one-to-one observation and if not then by suicidal behavior.

Of the elderly (≥65) patients not requiring observation, one-third were split off into a group comprising four relatively small subgroups: those requiring detox, few checks, or ECT or who had discharge referral problems. The remaining two-thirds of this group were further split into two groups according to psychiatric disorder severity.
The principal characteristics models limited the all characteristics benchmark model to a much smaller set of explanatory variables. Prior residence, gender, involuntary versus voluntary commitment status, first break, cognitive impairment, self-neglect, and any psychiatric admission within the preceding year were omitted because of inappropriateness or inconsistent results. Key cost drivers varied by diagnosis. For schizophrenia, eight clinically consistent subgroups were constructed ( Figure 2 ), ranging in per diem cost from $477 (few deficits in daily living activities, under age 65, and low illness severity) to $783 (many deficits in daily living activities, over age 65, and high medical comorbidity).
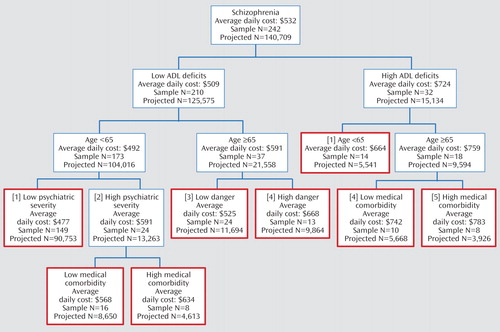
a Boxes with red borders are terminal groups used in initial per diem cost regressions. Numbers in brackets correspond to classification groups in Table 2.
For dementia patients ( Figure 3 ), the principal characteristics model identified seven consistent subgroups. Costs per diem ranged from $610 for patients with few deficits in daily living activities and low illness severity to a very high-cost ($815) group with many deficits in daily living activities, high medical comorbidity, and a high level of dangerousness.

a Boxes with red borders are terminal groups used in initial per diem cost regressions. Numbers in brackets correspond to classification groups in Table 2.
For mood disorder patients ( Figure 4 ), nine subgroups were formed with the principal characteristics model. Costs per diem ranged from $538 (for patients under age 65 with low medical comorbidity and not undergoing ECT or detox) to $910 (for those over age 65 with high illness severity and high medical comorbidity). ECT was a prominent cost driver for younger mood disorder patients with low medical comorbidity and older patients with high illness severity, low medical comorbidity, and a low level of dangerousness.

a Boxes with red borders are terminal groups used in initial per diem cost regressions. Numbers in brackets correspond to classification groups in Table 2.
Patients with substance-related disorders were split consistently only by age. No characteristic consistently split the very small group of patients with residual diagnoses.
Regression Analyses
Table 1 summarizes the explanatory power of the casemix classification models. The maximum possible explanatory power for a patient classification system using patient characteristics and day-of-stay groups is 76% (patient fixed effects model), implying that 24% of cost variation is due to idiosyncratic daily changes within patients. Facility characteristics and day of stay alone explained 23% of cost variation. Adding age and medical comorbidity to facility characteristics and day of stay increased explanatory power from 23% to 31%. When diagnosis-related groups were added, explanatory power rose only one percentage point. Since diagnosis-related groups were not developed to explain patient-level differences in routine care intensity (but rather were developed using per diem costs unadjusted for patient-specific resource intensity, since existing claims did not have the necessary data), this finding is not surprising. However, as noted previously, routine care costs comprise 85% of costs for these patients; developing a casemix classification system that attempts to explain these costs in addition to the remaining 15% is presumably important.
The 14-group principal characteristics model that used five major DSM-IV categories and stratified by age, illness severity, and deficits in daily living activities had an R 2 of 0.38, a 20% improvement over the CMS proposed rule model. The 16-group model that added use of ECT and dangerousness explained 40% of daily cost, a 25% improvement over the CMS proposed rule model. Sensitivity analyses showed superior explanatory power of the present study’s separate grouping of schizophrenia and mood disorders compared with one that combined patients with mania, mixed bipolar mood, and schizophrenia.
Table 2 compares the relative weights derived from the regression analyses for the 16-group principal characteristics model. The last column reports the percent of Medicare days in each casemix group. The schizophrenia group includes five terminal categories from Figure 2 . Schizophrenia patients were about 19% less costly per day than average. The largest payment group (subjects <65 years of age with either high deficits in daily activities or with low deficits in daily activities and low illness severity) had a relative weight of 0.85 (15% below average).
Dementia patients, overall, were the most costly patients (18% above average). Deficits in daily living activities and medical and psychiatric severity were powerful determinants of their costliness.
The cost weights for the five mood disorder groups ranged from a high of 1.41 to a low of 0.93. Patients undergoing ECT were 38% more expensive.
Patients with a residual diagnosis were 15% more costly on average. Relative weights for the very few elderly patients with substance-related principal diagnoses were 32% more costly per day than average; patients under age 65 were 16% less costly.
Costs per day declined during the stay (results not shown). The first full day was 16% more costly than average, and days 2–4 were also more costly than average. By the start of the third week (day 15 and beyond), daily costs were 8% below average.
Two facility characteristics (results not shown) were statistically significant in all models: teaching intensity and average daily census. The regression coefficient for the ratio of resident to average daily census was nearly double the equivalent in the Medicare acute inpatient prospective payment system. The psychiatric average daily census coefficient implied a 1.6% decrease in daily costs for a 10% increase in average daily census.
Increased Administrative Burden
The casemix classification system developed in this study included characteristics not currently collected for Medicare payment. Adopting such a patient classification system would presumably increase provider reporting burden. In a final regulation that implemented a 50-item data collection instrument for certain skilled nursing facilities (25) , CMS estimated 30 minutes of staff time for data collection, including data entry. We estimate that a similar data collection instrument for the patient classification scheme developed here would require far fewer items, perhaps one dozen in total, requiring 10 total minutes of staff (nurse and clerical worker) time.
In the study hospitals, average wages for nurses were $24/hour, and clerical workers were paid about $12/hour. If fringe benefits and other related overhead costs double the wage costs, this would imply a fairly small $6 increased cost per patient. The average cost per case in inpatient psychiatric units in 2000 was $6,155 (26) , so the extra $6 per patient would increase facilities’ per case costs by about 0.1%.
Discussion
Claims-based analyses fail to reflect the disproportionate staff time spent caring for the most difficult patients. The cost measure used in this study, which adjusted for each patient’s relative staffing intensity, permits analyses of cost differences among Medicare patients not previously possible.
Our findings confirm earlier research that diagnosis-related groups are inadequate measures of casemix costliness for Medicare psychiatric and substance use disorder cases, even on a per diem basis (8 , 10) . Limitations of diagnosis-related groups in casemix classification are exacerbated when only administrative data are used for costing because they assign the same routine per diem cost to all patients in a facility. A fully interactive approach that begins with five DSM-IV categories then further stratifies by selected patient characteristics would produce more efficient and equitable payment levels. Our findings support higher payment levels for severe psychiatric and medical diagnoses within schizophrenia and mood disorders. For these two disorders, we also identified small high-cost groups of patients with high levels of dangerousness. These patients would not likely be identified using diagnosis-related groups, age, or even psychiatric comorbidity.
Other key cost drivers included deficits in daily living activities and medical severity. Deficits in daily living activities should be limited to the three areas (assistance with toileting, eating, and bathing) that were most staff-intensive according to our sensitivity analysis of other deficits. Also, our list of severe medical conditions is restricted to those observed in our patient sample; other severe conditions not in our list may also be costly, and a clinical panel of physicians and nurses should refine the list.
ECT has also been shown to contribute materially to daily costs beyond routine staffing needs. ECT treatment can be quite helpful, primarily for patients with refractory depression or mania. Because it is still a relatively rare but costly intervention, ECT could be considered as an add-on payment across all diagnostic categories (as in the new CMS prospective payment system). Concerns over patient safety have been addressed by APA (27) , which issued a set of rigorous guidelines for appropriate ECT candidates.
Also notable is the finding that many other characteristics, inappropriate for use in a payment system, proved to be minor determinants of cost after more important factors were controlled. Cognitive impairment, risk of falls, and Global Assessment of Functioning score were generally minor splitting variables. Furthermore, the explanatory power of the parsimonious principal characteristics model compared favorably to the all characteristics benchmark model, which was based on characteristics inappropriate for a payment system and had more than four times as many patient groups.
We warn against placing too much emphasis on the overall explanatory power of particular casemix categories. A small, but very resource-intensive, group will add little to a model’s explanation of variance, but combining with a large, less intensive group may cause providers to avoid admitting these difficult patients.
The government and industry’s preference for a per diem rather than a per stay basis of payment may encourage providers to extend inpatient stays. Declining block pricing can reduce this incentive. Our findings confirm those of CMS (6 , 7) and other researchers (10 , 11 , 20) that costs during the first few days of a patient’s stay are much higher than subsequent days after other covariates are controlled. Higher payments for the first few days will be more equitable to providers and would encourage providers to accept unstable, high-intensity patients.
The government has several options in light of our findings. In the short term, CMS could recalibrate its model by cross-walking diagnosis-related groups into major DSM-IV categories and interacting with age. Although this approach is more consistent with the way clinicians manage patient care, payment efficiency and equity would be only slightly improved because of the continued absence of within-facility routine cost variation due to claims-based costing.
Another option would be to adopt our relative weights for 14–16 casemix groups and day-of-stay adjustors and require that providers report indicators of dangerousness and deficits in daily living activities. The government could continue to use its facility-level adjustments. However, if CMS required providers to report deficits in daily living activities and dangerousness in our recalibrated model, the relative weights would still be compressed because of claims-based costing. CMS might consider establishing three or four levels of “routine” psychiatric care analogous to routine and ICU cost centers (e.g., general, geriatric, and med-psych units). Unit types would have to be defined in regulations.
A longer-term option would be to adopt our relative weights for a few years then recalibrate rates within 5 years on the basis of a larger provider survey. This approach would have the advantage of using a patient-specific measure of routine staff intensity based on a greater number of rural and public psychiatric facilities.
1. Balanced Budget Refinement Act of 1999. HR 3426, Public Law 106–113 (Nov. 29, 1999)Google Scholar
2. Cromwell J, Harrow B, McGuire TG, Ellis RP: Medicare payment for psychiatric facilities: unfair and inefficient. Health Aff (Millwood) 1991; 10:124–134Google Scholar
3. Liu CF, Cromwell J (Health Economics Research, Inc, Waltham, Mass.): Impact of the Balanced Budget Act of 1997 on PPS-Exempt Psychiatric Facilities. Final Report. Washington, DC, National Association of Psychiatric Health Systems, 1998Google Scholar
4. Menke TJ, Ashton CM, Petersen NJ, Wolinsky FD: Impact of an all-inclusive diagnosis-related group payment system on inpatient utilization. Med Care 1998; 36:1126–1137Google Scholar
5. Lave JR: Developing a Medicare prospective payment system for inpatient psychiatric care. Health Aff (Millwood) 2003; 22:97–109Google Scholar
6. Centers for Medicare & Medicaid Services: Medicare program; prospective payment system for inpatient psychiatric facilities; proposed rule. Federal Register 2003; 68(229):66920–66978Google Scholar
7. Centers for Medicare & Medicaid Services: Medicare program; prospective payment system for inpatient psychiatric facilities; final rule. Federal Register 2004; 69(219):66921–67015Google Scholar
8. English JT, Sharfstein SS, Scherl DJ, Astrachan B, Muszynski IL: Diagnosis-related groups and general hospital psychiatry: The APA study. Am J Psychiatry 1986; 143:131–139Google Scholar
9. Horgan C, Jencks SF: Research on psychiatric classification and payment systems. Med Care 1987; 25(suppl 9):S22–S36Google Scholar
10. Mitchell JB, Dickey B, Liptzen B, Sederer LI: Bringing psychiatric patients into the Medicare prospective payment system: alternatives to DRGs. Am J Psychiatry 1987; 144:610–615Google Scholar
11. Freiman MP, Mitchell JB, Rosenbach ML: Simulating policy options for psychiatric care in general hospitals under Medicare’s PPS. Arch Gen Psychiatry 1988; 45:1032–1036Google Scholar
12. Horn SD, Chambers AF, Sharkey PD, Horn RA: Psychiatric severity of illness: a casemix study. Med Care 1989; 27:69–84Google Scholar
13. Ashcraft MLF, Fries BE, Nerenz DR, Falcon SP, Srivastava SV, Lee CZ, Berki SE, Errera P: A psychiatric patient classification system: an alternative to diagnosis-related groups. Med Care 1989; 27:543–554Google Scholar
14. Taube CA, Goldman HH, Lee ES: Use of specialty psychiatric settings in constructing DRGs. Arch Gen Psychiatry 1988; 45:1037–1040Google Scholar
15. Fries BE, Nerenz DR, Falcon SP, Ashcraft MLF, Lee CZ: A classification system for long-staying psychiatric patients. Med Care 1990; 28:311–323Google Scholar
16. Cromwell J, Gage BJ, Drozd EM, Maier J, Osber DS, Richter EM, Goldman HH (RTI International, Waltham, Mass): Psychiatric Inpatient Routine Cost Analysis: Final Report. Baltimore, Centers for Medicare & Medicaid Services, 2003Google Scholar
17. Kish L: Survey Sampling. New York, John Wiley & Sons, 1965Google Scholar
18. Steinberg D, Colla P: CART–Classification and Regression Trees. San Diego, Salford Systems, 1997Google Scholar
19. Breiman L, Friedmen J, Olshen R, Stone C: Classification and Regression Trees. Boca Raton, Fla, Chapman & Hall/CRC, 1984Google Scholar
20. Frank RG, Lave JR: Per case prospective payment for psychiatric inpatients: an assessment and alternatives. J Health Polit Policy Law 1986; 11:83–96Google Scholar
21. Ellis RP, McGuire TG: Hospital response to prospective payment: cost sharing and supply. J Health Economics 1986; 5:129–151Google Scholar
22. Fuller WA: Regression analysis for sample surveys. Sankhy 1975: 37(3) Series C:117–132Google Scholar
23. Eltinge JL, Sribney WM: Estimates of linear combinations and hypothesis tests for survey data. Stata Technical Bulletin 31:31–42Google Scholar
24. Greene WH: Econometric Analysis, 5th Ed. Upper Saddle River, NJ, Prentice Hall, 2002Google Scholar
25. Centers for Medicare & Medicaid Services: Medicare program; prospective payment system and consolidated billing for skilled nursing facilities–update; final rule. Federal Register 2001; 66(147):39562–39607Google Scholar
26. US Department of Health and Human Services: Report to Congress: prospective payment system for inpatient services in psychiatric hospitals and exempt units. Washington, DC, HHS, 2002Google Scholar
27. Weiner RD: Practice of Electroconvulsive Therapy: Recommendations for Treatment, Training, and Privileging (A Task Force Final Report of the American Psychiatric Association), 2nd ed. Washington, DC, American Psychiatric Press, 2001Google Scholar