A Population-Based Analysis of the Relationship Between Substance Use and Adolescent Cognitive Development
Abstract
Objective:
Alcohol and cannabis misuse are related to impaired cognition. When inferring causality, four nonexclusive theoretical models can account for this association: 1) a common underlying vulnerability model; 2) a neuroplasticity model in which impairment is concurrent with changes in substance use but temporary because of neuroplastic brain processes that restore function; 3) a neurotoxicity model of long-term impairment consequential to substance use; and 4) a developmental sensitivity hypothesis of age-specific effects. Using a developmentally sensitive design, the authors investigated relationships between year-to-year changes in substance use and cognitive development.
Method:
A population-based sample of 3,826 seventh-grade students from 31 schools consisting of 5% of all students entering high school in 2012 and 2013 in the Greater Montreal region were assessed annually for 4 years on alcohol and cannabis use, recall memory, perceptual reasoning, inhibition, and working memory, using school-based computerized assessments. Multilevel regression models, performed separately for each substance, were used to simultaneously test vulnerability (between-subject) and concurrent and lagged within-subject effects on each cognitive domain.
Results:
Common vulnerability effects were detected for cannabis and alcohol on all domains. Cannabis use, but not alcohol consumption, showed lagged (neurotoxic) effects on inhibitory control and working memory and concurrent effects on delayed memory recall and perceptual reasoning (with some evidence of developmental sensitivity). Cannabis effects were independent of any alcohol effects.
Conclusions:
Beyond the role of cognition in vulnerability to substance use, the concurrent and lasting effects of adolescent cannabis use can be observed on important cognitive functions and appear to be more pronounced than those observed for alcohol.
Beyond their acute effects, alcohol and cannabis misuse have been associated with impairments in learning, decision making, and cognitive functioning as well as lower academic performance (1). Meta-analyses have linked cannabis use to poor cognition in the domains of learning, memory, attention, and working memory (2), and regular and heavy alcohol use to poor verbal fluency, processing speed, episodic and working memory, attention, executive functions, inhibition/impulsivity, and visuospatial abilities (3). These effects have been shown in adults and adolescents (2–8). Studies also suggest that impairments in some of these domains persist (e.g., IQ, working memory, inhibition, and others) (3, 4), whereas other domains recover as consumption changes (e.g., processing speed, recall memory, attention, and others) (3, 5). Imaging studies in adolescent substance users also indicate smaller volumes (9) in brain regions responsible for these cognitive functions, such as the prefrontal cortex and the left hippocampus. What has yet to be established in the literature is the extent to which such cognitive impairments represent an underlying vulnerability to misusing substances or are a direct consequence of substance use or misuse.
The literature investigating the relationship between adolescent substance use and brain functions is mixed (3–8, 10, 11) and may depend on how childhood cognitive deficits that exist before the onset of substance use are accounted for in such analyses (12, 13). Few studies have investigated the relationship between cognitive functions and substance use repeatedly over time; most studies simply test differences in cognition at one time point and again after a given follow-up interval. Even fewer studies have considered the continuum of substance use behavior, and studies tend to compare nonusers to occasional or problematic users. It is important that new large-scale studies incorporate developmentally sensitive designs that model the impact of year-to-year changes in substance use on age-related changes in cognition.
In the absence of experimental designs, large-scale longitudinal designs with multiple repeated assessments provide an opportunity to explore inferences about causality between two variables by examining how changes in one domain are related to changes in another over time. As depicted in Figure 1, computational models can test the extent to which changes in one behavior lead to concurrent or lasting changes in another (e.g., after an increase in substance use subsides), but such models require large prospective data sets. To our knowledge, few data sets have been available that might allow dissociation of antecedent cognitive risk factors from the consequences of substance misuse on adolescent cognitive development from this perspective.
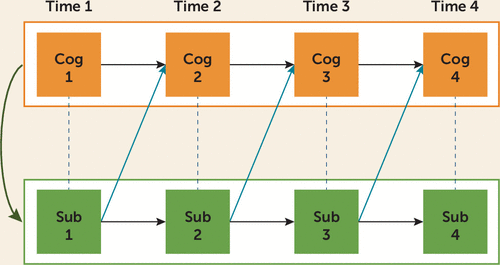
FIGURE 1. Neurotoxicity and Neuroplasticity Models: Multilevel Modeling of Causalitya
a This multilevel computational method tests between-subject differences at one level and then various within-subject processes at a second level, allowing for the investigation of concurrent and time-lagged relationships between sets of variables. Cognitive performance (Cog) and substance use (Sub) were measured at four time points (time 1 represents assessment in the 7th grade, time 2 in 8th grade, and so on). The first hypothesis proposes an underlying cognitive vulnerability that might contribute to early-onset substance use and the likelihood of continued and heavy use over time (green arrow) (14, 15). Three within-subject effects reflect processes that are consequential to substance use. The neurotoxicity hypothesis suggests that past substance use causes impairment in cognitive function in some lasting way, regardless of whether the substance use continues (blue arrows) (16). The neuroplasticity hypothesis suggests that consumption is associated with impaired cognitive performance, but only in the short term, and that through mechanisms of neuroplasticity, abstinence, or reduction in consumption, the cognitive impairment subsides (hashed lines). Finally, in the developmental sensitivity hypothesis, substance use at a critical period in development will lead to neurotoxicity, depending on the neuromaturational state of the particular brain region (with larger neurotoxicity or neuroplasticity effects at earlier times than at later times).
Using this multivariate, multilevel framework, the association between substance use and cognition can be investigated with respect to four theoretical hypotheses, also shown in Figure 1. Recognizing that cognitive factors are also implicated in risk for early-onset substance use (17), we hypothesized that working memory and response inhibition (two executive functions of the frontal lobes) would be associated with overall risk for early onset and heavier substance use generally (the vulnerability hypothesis). Consistent with previous research suggesting a relationship between adolescent binge drinking and cognitive functions (7), we hypothesized that further increases in alcohol consumption would predict impaired spatial working memory, recall memory, perceptual reasoning, and inhibition, over and above common vulnerability. The literature also suggests that effects of heavy alcohol consumption on memory recover over time (3). We therefore hypothesized that the relationship between alcohol use and memory functions could be accounted for by common vulnerability and neuroplasticity hypotheses.
The effects of cannabis use should also conform to a vulnerability hypothesis, particularly with respect to inhibitory function (1), but additional cognitive consequences of adolescent cannabis use are hypothesized on measures of memory function and general IQ, such as perceptual reasoning (7, 18). Considering results from animal studies showing that chronic administration of THC causes dose-dependent neurotoxic changes in brain regions that are rich in cannabinoid receptors, such as the hippocampus, amygdala, septum, and cortex (17), and that abnormalities in hippocampal and temporal structures seem particularly linked to human cannabis use (16), we hypothesized that additional visual-spatial memory deficits will be consequential to cannabis use in adolescence, where both neuroplasticity and neurotoxicity models are considered, as the literature relating to the degree to which these effects last beyond the consumption period in humans remains inconclusive. A fourth model will also be tested—the developmental sensitivity model, informed by current neurodevelopmental theories (19) suggesting that cognitive functions linked to the prefrontal cortex (executive cognitive functions such as working memory, response inhibition, and perceptual reasoning) should show age-dependent effects, with earlier onset of substance use being linked to greater impairment.
Using data from a large longitudinal study of adolescents assessed repeatedly on substance use and cognitive functions through the critical developmental period when substance use onset and brain maturation overlap, this study represents a unique opportunity to study the effects of cannabis and alcohol on various cognitive domains with enough power to model the complex nature of these relationships. Results from this highly conservative analysis may help guide drug policy. Results supporting neuroplastic or neurotoxic effects of substance use on adolescent cognitive development may help in advocating for more investment in evidence-based preventive interventions, which currently represent a small fraction of the societal costs resulting from substance abuse in Western societies (20).
Method
Participants
We used data from the Co-Venture trial (21), a longitudinal population-based randomized controlled trial assessing the 5-year efficacy of a personality-targeted drug and alcohol prevention program named Preventure. A total of 3,826 seventh-graders (47% female; mean age, 12.7 years [SD=0.5]; 58% of European origin) were recruited from 31 schools in the Montreal area. This school sample of adolescents, studied annually from seventh grade through 11th grade, is epidemiologically representative of each of its respective school districts with respect to average size and socioeconomic index. The sample of participating schools represents 15% of all schools across all school districts of the Greater Montreal area. The study sampled, on average, 76% of all seventh-grade students in these schools, suggesting that the cohort included 6.0%−11.4% of the entire population of seventh-grade students of the Greater Montreal area in 2012 and 2013. Only two school-level exclusion criteria were specified: the school had to agree to the study protocol, including randomization and 5-year school-based follow-up, and the school could not have more than 50% of its seventh-grade students having official educational codes that indicate special learning needs. Participating schools were randomly assigned either to deliver the Preventure program to seventh-grade high-risk adolescents or to deliver the program to subsequent seventh-grade cohorts 3 years later. There were no exclusion criteria specified for students, other than being able to provide informed assent and parental consent. All participating seventh-grade students in September 2012 or 2013 completed a confidential annual web-based survey during class time (from grades seven through 10) to assess cognition and substance use. Confidentiality was assured by emphasizing that parents and teachers would not have access to survey results and by automatically anonymizing assessments. Ethical approval was obtained from the Sainte-Justine Hospital Ethics Committee in Montreal. Depending on the school, either passive or active parental consent was obtained.
The quality and reliability of the data were evaluated using automated algorithms assessing valid response ranges on cognitive tasks and a sham drug item on substance use self-reports. All participants who consented to the study were included in the analysis if 75% of their data across all items and assessment points were considered complete and reliable. Among the 3,826 adolescents who consented to participate, 3,659 (95.6%) passed the data quality control requirements while also providing the required minimal demographic information (sex and socioeconomic status). Attrition was not predicted by any covariates (sex, p=0.43; socioeconomic status, p=0.88). While the intervention delivered in this trial is expected to have an impact on substance use behavior, there is no reason to expect that the intervention will have an impact on how substance use behavior will subsequently affect cognitive functions, so all participants, regardless of intervention exposure, were included in the analysis.
Predictors
Alcohol and cannabis use were assessed using the Detection of Alcohol and Drug Problems in Adolescents questionnaire (14). Once a year for 4 consecutive years, participants rated their consumption frequency on a 6-point scale (0=never, 5=every day) for each named substance, and for alcohol specifically, they provided the typical number of drinks consumed when they drink alcohol. No quantity measure was used for cannabis, as cannabis quantity assessment remains a challenge in the field (15). Three variables were extracted at each time point: alcohol use frequency, typical quantity of alcohol intake on a typical drinking occasion, and cannabis use frequency. Alcohol consumption frequency and quantity were multiplied at each time point to create a quantity×frequency variable for each of the 4 years of measurement. This variable is sensitive to differences in dose and frequency of consumption, thereby distinguishing frequent light drinkers from frequent heavy drinkers (22). When self-report measures are used in a context that guarantees confidentiality, they are considered more accurate than collateral reports or biologic measures of adolescent substance use because they are better at capturing the episodic and illicit nature of adolescent substance use (23, 24). All participants in this study agreed that parents and school staff would not have access to self-report information unless such information indicated imminent risk of harm. Consumption distributions are summarized in Table 1.
Substance and Assessmenta | Frequency or Quantity | |||||
---|---|---|---|---|---|---|
Frequency | Never | Occasionally | Once a Month | Once or Twice Per Week | Three Times or More Per Week | Every Day |
Cannabis use | ||||||
Year 1 | 95.41% | 2.76% | 0.71% | 0.45% | 0.32% | 0.37% |
Year 2 | 90.20% | 6.27% | 1.50% | 1.12% | 0.53% | 0.38% |
Year 3 | 80.09% | 12.29% | 2.20% | 2.95% | 1.17% | 1.30% |
Year 4 | 71.19% | 17.91% | 3.62% | 3.47% | 1.81% | 2.00% |
Alcohol use | ||||||
Year 1 | 63.56% | 31.77% | 2.97% | 1.39% | 0.18% | 0.13% |
Year 2 | 48.66% | 41.62% | 7.18% | 2.21% | 0.24% | 0.10% |
Year 3 | 35.86% | 46.04% | 11.67% | 6.06% | 0.23% | 0.13% |
Year 4 | 23.87% | 44.61% | 18.89% | 11.73% | 0.60% | 0.30% |
Number of drinks on drinking occasion | ||||||
Quantityb | 0 | 1–2 | 3–5 | 5–8 | >8 | |
Alcohol use | ||||||
Year 1 | 85.37% | 11.58% | 1.81% | 0.81% | 0.44% | |
Year 2 | 74.97% | 18.67% | 3.55% | 2.15% | 0.66% | |
Year 3 | 65.44% | 21.51% | 7.22% | 4.33% | 1.49% | |
Year 4 | 59.33% | 19.75% | 10.51% | 8.68% | 1.74% |
TABLE 1. Frequency Distribution for Substance Use Variables in a School Sample of Adolescents Assessed Over 4 Years
Outcomes
All tasks are described in more detail in the study protocol, which has been published elsewhere (21). Spatial working memory was measured with the “find the phone” task, based on the self-order pointing task (25) and the spatial working memory subtest in the Cambridge Neuropsychological Test Automated Battery. Delayed recall memory was assessed with a computerized task based on the dot location test of the Child Memory Scales (26). Participants are asked to reproduce a previously learned pattern of stimuli 30 minutes later. Perceptual reasoning was assessed using a selection of items from the Cattell’s Culture Fair Intelligence Test. Participants completed a sequence of puzzles of progressively increasing difficulty. This nine-item task correlates highly with Raven’s 60-item perceptual reasoning matrices (27).
Inhibitory control was measured using the passive avoidance learning paradigm (28, 29). Participants learn by trial and error to respond to “good” numbers and to withhold responses to “bad” numbers by experiencing rewards to correct presses or omissions and punishments to incorrect presses or omissions. This measure has been used in past research and is correlated with other measures of inhibition and with prefrontal cortical activation during other go/no-go tasks (30).
Covariates
Baseline socioeconomic status was controlled for with the Family Affluence Scale (31). Analyses also controlled for self-reported gender. Additional potential confounding variables were investigated in sensitivity analyses, including an estimate of ethnicity (assessed on the basis of child-reported country of origin of the child and parents) and family intactness (living with both biological parents [69% of the sample], or not).
Statistical Analysis
Multilevel linear models assessed the influence of cannabis (frequency) and alcohol (quantity×frequency) consumption on four domains of cognition. Three multilevel linear models were applied: one for cannabis, one for alcohol, and one combining alcohol and cannabis. The time parameter was coded as wave. Predictors were person-mean centered. Normality and homoscedasticity of residuals were examined for each step of the models. For all three analyses, a first model estimated the intercept and time parameters and a second model evaluated the contribution of three predictors: average use over 4 years (between-subject differences in consumption), change in use this year compared with the participant’s mean use (within-subject difference in consumption), and substance use the year before compared with the participant’s mean use (lagged within-subject difference in consumption). A final model added interaction parameters: interaction of time by average use over 4 years, interaction of time by change in use this year compared with the participant’s mean use, and interaction of time by substance use the year before compared with the participant’s mean use. Effects of between-subject differences were interpreted as a common vulnerability between consumption and poor neurocognitive performance. Within-subject effects (increased consumption that year) were interpreted as neuroplastic effects, and time-lagged within-subject effects (consumption last year) were interpreted as neurotoxic effects. The most parsimonious of three iterative steps for each analysis was identified using the likelihood ratio test. Only effects revealed to be significant in the most parsimonious model were interpreted.
Missing data on the main variables were handled through full information maximum likelihood. School was included as a cluster-level variable. As a sensitivity analysis, and to ensure the robustness of our results, all models were reestimated excluding users at the first year to focus only on those who started substance use later.
The intraclass correlation coefficient (ICC) function from the psych package in the R statistical environment was used to estimate the within-subject stability of cognitive data over time; intraclass correlation coefficients were 0.74 for working memory, 0.80 for perceptual reasoning, 0.58 for delayed memory recall, and 0.68 for response inhibition.
Results
Cannabis Models
Table 2 presents results for the cannabis models. The first model indicated that average frequency of cannabis use over 4 years (between-subject differences) predicted lower performance on working memory (β=0.51, SE=0.25, p=0.04), perceptual reasoning (β=−0.25, SE=0.08, p=0.001), and inhibition (β=1.19, SE=0.48, p<0.01) over the same time period. Over and above the significant between-subject effects, a significant within-subject effect showed that any further increase in cannabis use frequency was associated with impairment in delayed recall memory in the same year (β=−0.14, SE=0.05, p<0.01). A significant within-subject lagged effect revealed that any further increases in cannabis use frequency predicted further impairment on the inhibition task 1 year later (β=1.05, SE=0.41, p=0.01). Similar, but marginal, cannabis lagged effects were revealed for working memory (β=0.36, SE=0.19, p=0.06).
Working Memory | Perceptual Reasoning | Delayed Recall Memory | Inhibitory Control | |||||||||
---|---|---|---|---|---|---|---|---|---|---|---|---|
Predictor | Estimate | SE | Pr(>|t|) | Estimate | SE | Pr(>|t|) | Estimate | SE | Pr(>|t|) | Estimate | SE | Pr(>|t|) |
Model 1 | ||||||||||||
Intercept | 22.210 | 1.411 | 0.000 | 14.814 | 0.396 | 0.000 | 17.516 | 0.376 | 0.000 | 38.422 | 2.940 | 0.000 |
Time | –6.745 | 0.947 | 0.000 | 1.196 | 0.263 | 0.000 | –9.440 | 0.261 | 0.000 | –8.972 | 1.995 | 0.000 |
Time squared | 0.802 | 0.159 | 0.000 | –0.089 | 0.044 | 0.042 | 1.976 | 0.044 | 0.000 | 0.862 | 0.333 | 0.010 |
Socioeconomic status | 0.124 | 0.079 | 0.118 | –0.041 | 0.025 | 0.097 | –0.017 | 0.016 | 0.277 | 0.286 | 0.151 | 0.058 |
Gender | 1.843 | 0.266 | 0.000 | 0.311 | 0.084 | 0.000 | 0.051 | 0.054 | 0.341 | 0.440 | 0.509 | 0.387 |
Cannabis, between-subjects | 0.505 | 0.246 | 0.040 | –0.251 | 0.077 | 0.001 | –0.044 | 0.057 | 0.443 | 1.912 | 0.482 | 0.000 |
Cannabis, within-subjects | 0.022 | 0.166 | 0.894 | –0.084 | 0.045 | 0.064 | –0.140 | 0.048 | 0.004 | 0.420 | 0.352 | 0.232 |
Cannabis, within-subjects (lagged) | 0.361 | 0.192 | 0.061 | 0.076 | 0.053 | 0.151 | 0.004 | 0.057 | 0.948 | 1.045 | 0.411 | 0.011 |
Model 2 | ||||||||||||
Intercept | 22.104 | 1.494 | 0.000 | 14.729 | 0.420 | 0.000 | 17.485 | 0.399 | 0.000 | 39.075 | 3.104 | 0.000 |
Time | –6.706 | 1.010 | 0.000 | 1.276 | 0.281 | 0.000 | –9.415 | 0.278 | 0.000 | –9.517 | 2.119 | 0.000 |
Time squared | 0.802 | 0.169 | 0.000 | –0.107 | 0.047 | 0.023 | 1.971 | 0.047 | 0.000 | 0.969 | 0.354 | 0.006 |
Socioeconomic status | 0.122 | 0.079 | 0.124 | –0.040 | 0.025 | 0.109 | –0.017 | 0.016 | 0.290 | 0.281 | 0.151 | 0.063 |
Gender | 1.863 | 0.267 | 0.000 | 0.297 | 0.084 | 0.000 | 0.048 | 0.054 | 0.372 | 0.499 | 0.510 | 0.328 |
Cannabis, between-subjects | 1.426 | 0.854 | 0.095 | –0.789 | 0.231 | 0.001 | –0.027 | 0.225 | 0.906 | 3.904 | 1.727 | 0.024 |
Cannabis, within-subjects | 0.576 | 0.785 | 0.463 | –0.661 | 0.221 | 0.003 | –0.323 | 0.194 | 0.096 | 3.660 | 1.665 | 0.028 |
Cannabis, within-subjects (lagged) | 0.191 | 0.950 | 0.841 | 0.084 | 0.266 | 0.754 | 0.107 | 0.234 | 0.648 | 0.712 | 2.082 | 0.732 |
Time×cannabis, between-subjects | –0.315 | 0.265 | 0.235 | 0.177 | 0.072 | 0.014 | –0.006 | 0.084 | 0.944 | –0.639 | 0.543 | 0.240 |
Time×cannabis, within-subjects | –0.126 | 0.249 | 0.612 | 0.159 | 0.071 | 0.025 | 0.061 | 0.067 | 0.360 | –0.927 | 0.529 | 0.080 |
Time×cannabis, within-subjects (lagged) | 0.124 | 0.298 | 0.676 | –0.046 | 0.084 | 0.584 | –0.038 | 0.080 | 0.632 | 0.265 | 0.647 | 0.682 |
TABLE 2. Estimated Parameters for All Cannabis Models in a School Sample of Adolescents Assessed Over 4 Yearsa
Including interactions with time improved model fit only for the perceptual reasoning model and revealed a time-by-within-subject interaction, suggesting stronger within-subject, or concurrent, effects (β=−0.66, SE=0.22, p<0.003) in early adolescence than in late adolescence (β=0.16, SE=0.07, p=0.03). Sensitivity analysis focusing on participants who were drug and alcohol naive at the first assessment showed the same pattern of results.
Alcohol Models
Table 3 presents results for the alcohol models. The first model indicated that average quantity×frequency of alcohol consumption over 4 years was related to lower spatial working memory performance (β=0.09, SE=0.05, p<0.05), lower perceptual reasoning scores (β=−0.06, SE=0.02, p<0.01), and more errors on the inhibitory control task (β=0.27, SE=0.09, p<0.01) over the same time period, which is consistent with a common vulnerability hypothesis between these cognitive domains and alcohol use. No within-subject alcohol effects reached significance for any of the cognitive domains studied. Because the model including time interactions did not significantly improve model fit, no interaction terms are interpreted.
Working Memory | Perceptual Reasoning | Delayed Recall Memory | Inhibitory Control | |||||||||
---|---|---|---|---|---|---|---|---|---|---|---|---|
Predictor | Estimate | SE | Pr(>|t|) | Estimate | SE | Pr(>|t|) | Estimate | SE | Pr(>|t|) | Estimate | SE | Pr(>|t|) |
Model 1 | ||||||||||||
Intercept | 22.214 | 1.414 | 0.000 | 14.905 | 0.396 | 0.000 | 17.585 | 0.376 | 0.000 | 38.303 | 2.945 | 0.000 |
Time | –6.797 | 0.952 | 0.000 | 1.104 | 0.264 | 0.000 | –9.477 | 0.261 | 0.000 | –8.905 | 2.003 | 0.000 |
Time squared | 0.814 | 0.160 | 0.000 | –0.071 | 0.044 | 0.108 | 1.980 | 0.044 | 0.000 | 0.871 | 0.335 | 0.009 |
Socioeconomic status | 0.124 | 0.080 | 0.121 | –0.035 | 0.025 | 0.162 | –0.019 | 0.016 | 0.245 | 0.243 | 0.153 | 0.113 |
Gender | 1.834 | 0.267 | 0.000 | 0.313 | 0.084 | 0.000 | 0.058 | 0.054 | 0.280 | 0.400 | 0.511 | 0.434 |
Alcohol, between-subjects | 0.094 | 0.047 | 0.048 | –0.057 | 0.015 | 0.000 | –0.010 | 0.011 | 0.352 | 0.273 | 0.093 | 0.003 |
Alcohol, within-subjects | –0.002 | 0.026 | 0.936 | –0.010 | 0.007 | 0.143 | 0.000 | 0.008 | 0.985 | 0.063 | 0.056 | 0.265 |
Alcohol, within-subjects (lagged) | 0.031 | 0.035 | 0.375 | –0.005 | 0.010 | 0.568 | –0.013 | 0.010 | 0.188 | –0.019 | 0.071 | 0.789 |
Model 2 | ||||||||||||
Intercept | 22.359 | 1.553 | 0.000 | 14.878 | 0.436 | 0.000 | 17.547 | 0.415 | 0.000 | 38.329 | 3.227 | 0.000 |
Time | –6.914 | 1.052 | 0.000 | 1.146 | 0.293 | 0.000 | –9.438 | 0.289 | 0.000 | –8.989 | 2.208 | 0.000 |
Time squared | 0.836 | 0.176 | 0.000 | –0.082 | 0.049 | 0.094 | 1.971 | 0.049 | 0.000 | 0.893 | 0.369 | 0.015 |
Socioeconomic status | 0.124 | 0.080 | 0.121 | –0.035 | 0.025 | 0.162 | –0.018 | 0.016 | 0.253 | 0.251 | 0.153 | 0.102 |
Gender | 1.840 | 0.267 | 0.000 | 0.308 | 0.084 | 0.000 | 0.053 | 0.054 | 0.326 | 0.435 | 0.511 | 0.395 |
Alcohol, between-subjects | 0.123 | 0.164 | 0.453 | –0.148 | 0.046 | 0.001 | –0.019 | 0.042 | 0.649 | 0.101 | 0.339 | 0.767 |
Alcohol, within-subjects | 0.067 | 0.130 | 0.607 | –0.060 | 0.037 | 0.103 | –0.062 | 0.032 | 0.051 | 0.532 | 0.286 | 0.062 |
Alcohol, within-subjects (lagged) | 0.024 | 0.172 | 0.890 | –0.028 | 0.049 | 0.563 | 0.035 | 0.040 | 0.385 | –0.703 | 0.374 | 0.060 |
Time×alcohol, between-subjects | –0.008 | 0.051 | 0.872 | 0.029 | 0.014 | 0.039 | 0.004 | 0.016 | 0.820 | 0.035 | 0.106 | 0.738 |
Time×alcohol, within-subjects | –0.020 | 0.040 | 0.619 | 0.012 | 0.011 | 0.297 | 0.019 | 0.011 | 0.073 | –0.129 | 0.088 | 0.141 |
Time×alcohol, within-subjects (lagged) | 0.004 | 0.052 | 0.937 | 0.002 | 0.015 | 0.898 | –0.018 | 0.013 | 0.183 | 0.212 | 0.113 | 0.061 |
TABLE 3. Estimated Parameters for All Alcohol Models in a School Sample of Adolescents Assessed Over 4 Yearsa
Combined Alcohol-Cannabis Model
Table 4 presents an integrated model accounting for the effect of alcohol and cannabis simultaneously. Specific between-subject effects were revealed for alcohol and perceptual reasoning (β=−0.04, SE=0.02, p=0.03). No within-subject effects of alcohol were detected in the combined model. Specific between-subject effects were also revealed for cannabis and inhibitory control (β=1.48, SE=0.57, p<0.01). Lagged within-subject effects showed that cannabis use frequency in a given year further predicted lower performance on the inhibitory control task a year later (β=1.18, SE=0.44, p<0.01) and marginally predicted working memory performance a year later (β=0.36, SE=0.21, p<0.09), over and above changes in alcohol consumption. Increases in cannabis use frequency in a given year were also related to lower scores on the delayed recall memory task in that same year (β=−0.13, SE=0.05, p=0.01).
Working Memory | Perceptual Reasoning | Delayed Recall Memory | Inhibitory Control | |||||||||
---|---|---|---|---|---|---|---|---|---|---|---|---|
Predictor | Estimate | SE | Pr(>|t|) | Estimate | SE | Pr(>|t|) | Estimate | SE | Pr(>|t|) | Estimate | SE | Pr(>|t|) |
Intercept | 22.201 | 1.418 | 0.000 | 14.892 | 0.397 | 0.000 | 17.532 | 0.377 | 0.000 | 38.509 | 2.843 | 0.000 |
Time | –6.792 | 0.953 | 0.000 | 1.131 | 0.264 | 0.000 | –9.449 | 0.262 | 0.000 | –8.931 | 1.913 | 0.000 |
Time squared | 0.809 | 0.160 | 0.000 | –0.076 | 0.044 | 0.087 | 1.977 | 0.044 | 0.000 | 0.847 | 0.320 | 0.008 |
Socioeconomic status | 0.128 | 0.080 | 0.111 | –0.037 | 0.025 | 0.146 | –0.018 | 0.016 | 0.270 | 0.241 | 0.153 | 0.115 |
Gender | 1.840 | 0.267 | 0.000 | 0.314 | 0.084 | 0.000 | 0.053 | 0.054 | 0.328 | 0.458 | 0.509 | 0.368 |
Alcohol, between-subjects | 0.051 | 0.056 | 0.355 | –0.039 | 0.017 | 0.026 | –0.008 | 0.013 | 0.551 | 0.126 | 0.109 | 0.246 |
Alcohol, within-subjects | –0.008 | 0.027 | 0.760 | –0.008 | 0.008 | 0.277 | 0.006 | 0.008 | 0.469 | 0.017 | 0.060 | 0.773 |
Alcohol, within-subjects (lagged) | 0.006 | 0.037 | 0.862 | –0.008 | 0.010 | 0.457 | –0.010 | 0.011 | 0.364 | –0.098 | 0.077 | 0.200 |
Cannabis, between-subjects | 0.451 | 0.294 | 0.125 | –0.153 | 0.092 | 0.094 | –0.012 | 0.067 | 0.863 | 1.482 | 0.567 | 0.009 |
Cannabis, within-subjects | 0.044 | 0.180 | 0.805 | –0.081 | 0.049 | 0.099 | –0.132 | 0.052 | 0.010 | 0.527 | 0.380 | 0.165 |
Cannabis, within-subjects (lagged) | 0.357 | 0.207 | 0.085 | 0.098 | 0.057 | 0.085 | 0.001 | 0.060 | 0.986 | 1.181 | 0.443 | 0.008 |
TABLE 4. Estimated Parameters for Combined Alcohol and Cannabis Models in a School Sample of Adolescents Assessed Over 4 Yearsa
Discussion
Vulnerability Model
Cannabis and alcohol models yielded evidence in favor of common vulnerability: individuals more likely to use cannabis or alcohol showed lower working memory, perceptual reasoning, and inhibitory control. These findings are in line with previous research (1, 3, 13, 28). In the combined model, our results suggest that the common vulnerability between working memory and cannabis was not significant over and above alcohol use and vice versa, suggesting that poor working memory could constitute a nonspecific common vulnerability to substance misuse in adolescence (3, 13). Novel findings were those suggesting a common vulnerability process that is specific to low perceptual reasoning and alcohol consumption and a common vulnerability that is specific to cannabis and poor inhibitory control.
Neuroplasticity Hypothesis
Our results suggest neuroplastic (concurrent) effects of cannabis and, contrary to our hypotheses, did not reveal such effects for alcohol. Over and above the effect of being prone to cannabis use during adolescence, when increases in cannabis use frequency were observed in a given year, reductions in delayed recall memory and perceptual reasoning were observed in that same year, and these effects were independent of any changes in alcohol quantity×frequency. The transient effects of cannabis on episodic memory have been reported in animal (32) and human studies investigating long-term cognitive outcomes of cannabis-exposed subjects who later achieved abstinence (4). The ability to encode and retrieve memories is regulated by the circuitry of the medial-temporal lobe, including the hippocampus, which is rich in endocannabinoid receptors (17).
Neurotoxicity Hypothesis
Findings were also consistent with a lasting, or neurotoxic, effect of cannabis on two domains of cognition: inhibitory control and working memory. This study showed that cannabis use in a given year was associated with impaired inhibitory control and working memory 1 year later, over and above any common vulnerability. As reviewed by Volkow et al. (2), two meta-analyses summarizing case-control studies comparing users, nonusers, and former users suggest small but broad effects of cannabis on cognitive functioning. Moreover, a longitudinal analysis of adolescent cannabis users reported long-term effects of early onset and persistent cannabis use on measures of executive functioning, verbal IQ, and decision making (18). Functional imaging studies have also shown that adolescent cannabis users show abnormal prefrontal cortex activation during a working memory task and altered patterns of functional connectivity in frontotemporal networks (7). Working memory and response inhibition critically involve a network linking the prefrontal cortex to the posterior parietal cortex and the striatum, and animal studies indicate that the acute effects of cannabis on working memory are mediated through CB1 receptors in the prefrontal cortex and hippocampus (33). Considering that at least one experimental study with animals failed to demonstrate lasting working memory impairments following adolescent exposure to cannabis (32) and the fact that our analyses revealed marginal lagged effects for working memory, it will be important to further explore the nature of the long-term relationship between cannabis and working memory. One possibility worth exploring with available human data is whether these mild effects on working memory may be secondary to the effects of cannabis on other cognitive processes.
In this study, relationships between adolescent cannabis use and response inhibition were robust and consistent with both common vulnerability and neurotoxicity hypotheses. Youths and adults with heavy substance use patterns have been shown to differ from age- and sex-matched control subjects on cognitive, behavioral, and neural measures of disinhibition (12, 13). Longitudinal studies also confirm that some differences exist prior to onset of substance use (28). Our results suggest that poor response inhibition is both implicated in vulnerability to early-onset cannabis use specifically (between-subject effects) and consequential to increases in cannabis use. The novel findings resulting from this study are that changes in response inhibition following onset of cannabis use appear to be specific to cannabis and long lasting. These lasting neurotoxic effects could explain why early-onset substance use is so critically involved in future risk for addiction, as poor response inhibition and its neural correlates have been consistently identified as key risk factors for initiation and maintenance of substance use (30, 34).
Developmental sensitivity.
To investigate whether exposure to substances at earlier ages was associated with more severe impairment, we examined the interaction between each of these potential effects and the quadratic effect of time and did not find evidence of developmental sensitivity on three of the four cognitive domains. By contrast, as illustrated in Figure 2, the between-subject and concurrent within-subject relationships between cannabis and perceptional reasoning were more pronounced at earlier stages of adolescent development, indicating that early-onset users are more impaired on perceptual reasoning and the additional effect of their cannabis use in a given year is particularly harmful to their perceptual reasoning abilities during the early adolescent period.
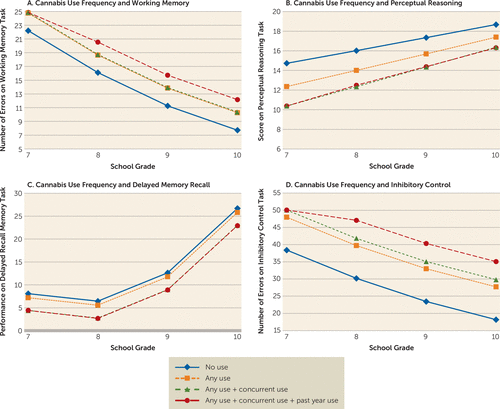
FIGURE 2. Between-Subject and Within-Subject (Concurrent and Lagged) Relationships Between Cannabis Use Frequency and Working Memory Errors, Perceptual Reasoning Performance, Delayed Memory Recall Performance, and Inhibitory Control Errorsa
a Lagged effects were calculated starting in 8th grade. For working memory, performance was measured by counting the number of times a previously chosen stimulus was selected on a given trial (i.e., spatial working memory errors); lower scores indicate better performance. For perceptual reasoning and delayed memory recall, performance was calculated as a score, with higher scores indicating better performance. For inhibitory control, performance was measured by counting the number of commission errors across both conditions of the task; lower scores indicate better performance.
Relevance
The results of this highly conservative and sensitive analysis demonstrate that cannabis is associated with more concurrent and long-term consequences on adolescent cognitive functions than alcohol, even when accounting for the effects of both substances within a single model and any potential underlying common vulnerability to all sets of problems. While previous twin studies (35) examined effects of cannabis on general IQ and only found evidence in favor of a common vulnerability hypothesis, our study was uniquely designed and powered to test year-by-year changes in cognition and substance use, and it was likely more sensitive to within-subject processes. Levels of cannabis use in this sample were low and infrequent (although 76 daily cannabis users were detected at the fourth annual assessment), but analyses nevertheless detected cognitive changes that were consequential to small increases in cannabis use. No such effects were detected for alcohol. It will be important to conduct similar analyses with this cohort or similar cohorts as they transition to young adulthood, when alcohol and cannabis use become more severe. This might be particularly relevant for alcohol effects: while the acute effects of alcohol on response inhibition, working memory, and episodic memory are clearly established, the neurotoxic effects on working memory may be observable only after binge drinking (36), in female drinkers (37), or in older drinking populations (38).
Limitations
Quantity or dose of cannabis exposure could not be assessed in this study, which is not unique to the present sample (15). It is expected that as legal and regulated cannabis markets emerge in North America, youths will eventually be able to refer to their consumption in terms of standard units in the way that alcohol quantity was assessed in this study. Furthermore, while all assessments of cognitive function took place in a school classroom setting under close supervision by research staff, which speaks to the ecological validity of the cognitive data collected, it would be important to link these results to standardized high school leaving examination scores and other meaningful academic outcomes as this cohort transitions to young adulthood. Finally, it will be important to investigate these findings in interaction with important demographic variables, such as gender and ethnicity, as this study was not designed to rigorously investigate such effects.
In summary, this study uniquely contributes to an emerging literature on neurocognitive consequences of alcohol and cannabis use by investigating relationships between year-to-year changes in substance use and cognitive development in a population-based sample of adolescents and by accounting for multilevel effects. In a context where policies and attitudes regarding substance use are being reconsidered, this research may contribute by highlighting the importance of protecting youths from adverse effects of consumption, particularly those substances that appear to have effects consistent with the neurotoxicity hypothesis (39).
1 : Adolescent cannabis use, change in neurocognitive function, and high-school graduation: a longitudinal study from early adolescence to young adulthood. Dev Psychopathol 2017; 29:1253–1266Crossref, Medline, Google Scholar
2 : Effects of cannabis use on human behavior, including cognition, motivation, and psychosis: a review. JAMA Psychiatry 2016; 73:292–297Crossref, Medline, Google Scholar
3 : Widespread and sustained cognitive deficits in alcoholism: a meta-analysis. Addict Biol 2013; 18:203–213Crossref, Medline, Google Scholar
4 : An evidence based review of acute and long-term effects of cannabis use on executive cognitive functions. J Addict Med 2011; 5:1–8Crossref, Medline, Google Scholar
5 : Non-acute (residual) neurocognitive effects of cannabis use: a meta-analytic study. J Int Neuropsychol Soc 2003; 9:679–689Crossref, Medline, Google Scholar
6 : Impact of adolescent alcohol and drug use on neuropsychological functioning in young adulthood: 10-year outcomes. J Child Adolesc Subst Abuse 2011; 20:135–154Crossref, Medline, Google Scholar
7 : Dare to delay? The impacts of adolescent alcohol and marijuana use onset on cognition, brain structure, and function. Front Psychiatry 2013; 4:53Crossref, Medline, Google Scholar
8 : Effects of emerging alcohol and marijuana use behaviors on adolescents’ neuropsychological functioning over four years. J Stud Alcohol Drugs 2015; 76:738–748Crossref, Medline, Google Scholar
9 : The influence of substance use on adolescent brain development. Clin EEG Neurosci 2009; 40:31–38Crossref, Medline, Google Scholar
10 : Individual differences in decision making and reward processing predict changes in cannabis use: a prospective functional magnetic resonance imaging study. Addict Biol 2013; 18:1013–1023Crossref, Medline, Google Scholar
11 : Are IQ and educational outcomes in teenagers related to their cannabis use? A prospective cohort study. J Psychopharmacol 2016; 30:159–168Crossref, Medline, Google Scholar
12 : Neuropsychological executive functioning in children at elevated risk for alcoholism: findings in early adolescence. J Abnorm Psychol 2004; 113:302–314Crossref, Medline, Google Scholar
13 : Shared predisposition in the association between cannabis use and subcortical brain structure. JAMA Psychiatry 2015; 72:994–1001Crossref, Medline, Google Scholar
14 : La Grille de dépistage de la consommation problématique d’alcool et de drogues chez les adolescents et les adolescentes (DEP-ADO): développement et qualités psychométriques. Drogues Sante Societe 2004; 3:20–37Crossref, Google Scholar
15 : Short scales to assess cannabis-related problems: a review of psychometric properties. Subst Abuse Treat Prev Policy 2008; 3:25Crossref, Medline, Google Scholar
16 : Is cannabis neurotoxic for the healthy brain? A meta-analytical review of structural brain alterations in non-psychotic users. Psychiatry Clin Neurosci 2013; 67:483–492Crossref, Medline, Google Scholar
17 : Does cannabis cause lasting brain damage? in Marijuana and Madness. Edited by Castle D, Murray RM, D’Souza DC. Cambridge, UK, Cambridge University Press, 2012, pp 103–113Google Scholar
18 : Persistent cannabis users show neuropsychological decline from childhood to midlife. Proc Natl Acad Sci USA 2012; 109:E2657–E2664Crossref, Medline, Google Scholar
19 : Neurodevelopmental trajectories of the human cerebral cortex. J Neurosci 2008; 28:3586–3594Crossref, Medline, Google Scholar
20 : Health expenditure by type of service, in Health at a Glance 2017: OECD Indicators. Paris, OECD, 2017, pp 140–141Google Scholar
21 : A cluster-randomised controlled trial evaluating the effects of delaying onset of adolescent substance abuse on cognitive development and addiction following a selective, personality-targeted intervention programme: the Co-Venture trial. Addiction 2017; 112:1871–1881Crossref, Medline, Google Scholar
22 : Alcohol consumption measures, in Assessing Alcohol Problems: A Guide for Clinicians and Researchers, 2nd ed. Edited by Allen JP, Wilson VB. Bethesda, Md, National Institute on Alcohol Abuse and Alcoholism, 2003, pp 75–100Google Scholar
23 : Measuring risks and outcomes in substance use disorders prevention research. J Consult Clin Psychol 2002; 70:1207–1223Crossref, Medline, Google Scholar
24 : Towards the assessment of adolescent problem drinking. J Stud Alcohol 1989; 50:30–37Crossref, Medline, Google Scholar
25 : Self-ordered pointing as a test of working memory in typically developing children. Memory 2007; 15:526–535Crossref, Medline, Google Scholar
26 : CMS: échelle de mémoire pour enfants. Paris, Éditions du Centre de Psychologie Appliquée, 2001Google Scholar
27 : Development of abbreviated nine-item forms of the Raven’s standard progressive matrices test. Assessment 2012; 19:354–369Crossref, Medline, Google Scholar
28 : Response inhibition and reward response bias mediate the predictive relationships between impulsivity and sensation seeking and common and unique variance in conduct disorder and substance misuse. Alcohol Clin Exp Res 2011; 35:140–155Crossref, Medline, Google Scholar
29 : Passive avoidance learning in psychopathic and nonpsychopathic offenders. J Abnorm Psychol 1986; 95:252–256Crossref, Medline, Google Scholar
30 : Adolescent impulsivity phenotypes characterized by distinct brain networks. Nat Neurosci 2012; 15:920–925Crossref, Medline, Google Scholar
31 : The Family Affluence Scale as a measure of national wealth: validation of an adolescent self-report measure. Soc Indic Res 2006; 78:473–487Crossref, Google Scholar
32 : Effects of adolescent cannabinoid self-administration in rats on addiction-related behaviors and working memory. Neuropsychopharmacology 2017; 42:989–1000Crossref, Medline, Google Scholar
33 : Acute cannabinoids impair working memory through astroglial CB1 receptor modulation of hippocampal LTD. Cell 2012; 148:1039–1050Crossref, Medline, Google Scholar
34 : Annual research review: On the developmental neuropsychology of substance use disorders. J Child Psychol Psychiatry 2016; 57:371–394Crossref, Medline, Google Scholar
35 : Impact of adolescent marijuana use on intelligence: results from two longitudinal twin studies. Proc Natl Acad Sci USA 2016; 113:E500–E508Crossref, Medline, Google Scholar
36 : Binge drinking affects attentional and visual working memory processing in young university students. Alcohol Clin Exp Res 2009; 33:1870–1879Crossref, Medline, Google Scholar
37 : Adolescent binge drinking linked to abnormal spatial working memory brain activation: differential gender effects. Alcohol Clin Exp Res 2011; 35:1831–1841Crossref, Medline, Google Scholar
38 : Acute effects of moderate alcohol on psychomotor, set shifting, and working memory function in older and younger social drinkers. J Stud Alcohol Drugs 2014; 75:870–879Crossref, Medline, Google Scholar
39 : Developing public health regulations for marijuana: lessons from alcohol and tobacco. Am J Public Health 2014; 104:1021–1028Crossref, Medline, Google Scholar