Abnormal Variability and Distribution of Functional Maps in Autism: An fMRI Study of Visuomotor Learning
Abstract
OBJECTIVE: Autism is a neurally based psychiatric disorder, but there is no consensus regarding the underlying neurofunctional abnormalities. Previous functional magnetic resonance imaging (fMRI) studies of simple movement suggested individually variable and scattered functional brain organization in autism. The authors examined whether such abnormalities generalize to multimodal processing (visually driven motor sequence learning). METHOD: Eight male autistic patients and eight comparison subjects matched with the patients on age, gender, and handedness were examined by using fMRI while they performed finger press movements prompted by visually presented repeating six-digit sequences. Hemodynamic responses to the six-digit sequences were statistically compared to responses to single-digit stimuli in one experiment and to regular six-digit sequences in another experiment. RESULTS: Both groups showed activations in bilateral premotor, superior parietal, and occipital cortices in both experiments. Task-by-group interactions showed that superior parietal activations were less pronounced in the autism group, whereas prefrontal cortex and more posterior parietal loci showed greater activation in the autism group than in the comparison group. The distances between Individual subjects’ activation peaks and the groupwise peak were greater in the autism group than in the comparison group. CONCLUSIONS: The results support earlier findings of abnormal variability and scatter of functional maps in autism. They are consistent with evidence from other studies suggesting early-onset disturbances in the development of cerebello-thalamo-cortical pathways in autism.
Autism is a neurally based psychiatric disorder, affecting multiple domains of cognitive, sensorimotor, and sociobehavioral function (1–3). Although strong genetic factors are suspected (1), its etiology remains unestablished. Besides convergent—although not uncontested (5–7)—evidence for cerebellar abnormalities (8–18), numerous brain regions have been implicated, but no consensus has been established (19). Functional imaging studies have shown abnormal regional activation profiles in autism and Asperger’s disorder for “theory of mind” tasks (20–22), face perception (23–25), and language (26, 27). However, no clear picture of neurofunctional abnormalities has yet emerged, possibly because abnormal activation for complex tasks could reflect diverse, more elementary abnormalities. In two previous functional magnetic resonance imaging (fMRI) studies (28, 29), we therefore focused on simple motor tasks.
Motor impairments, though not a prominent feature in autism, have been observed in many studies (3, 30–35). Stereotypic behavior is found both on the motor level (e.g., hand flapping) and on the cognitive level (e.g., obsessive interests). Motor abnormalities are seen in the first months of life (i.e., well before sociocommunicative deficits become apparent) and may thus reflect elementary impairments in autism (36). In normal development, simple motor behavior begins in the fetus (37, 38), suggesting that neurofunctional organization of the motor system emerges early in human development.
In a previous fMRI study, we found abnormal spatial variability of activation foci around the central sulcus in autistic individuals during finger movement, with widely scattered frontoparietal activation in some patients (29). These findings resembled those of another autism study showing abnormally scattered cerebellar activity during thumb movement (28).
The present study examined motor functions at a slightly higher level of complexity by applying a visuomotor paradigm with a learning component. Subjects performed visually prompted six-digit sequences of finger movements learned over brief periods of time. We examined two questions: First, do autistic patients as a group show abnormal activation patterns during visuomotor learning? Based on previous structural imaging and event-related potential studies (39–41), we expected reduced or abnormal recruitment of the frontal and parietal regions normally involved in early stages of digit-sequence learning (29). Second, do autistic individuals show similar abnormal neurofunctional variability for polymodal visuomotor learning as previously found for simple motor control?
Method
Subjects
The study subjects were eight male autistic patients (ages 15–41 years) and eight gender-matched healthy comparison subjects (ages 21–43 years). The patients met the DSM-IV criteria for autism and the criteria for a diagnosis of autism according to the Childhood Autism Rating Scale (42) and the Autism Diagnostic Interview—Revised (43), except for patient 6, whose scores fell below the cutoff on the Childhood Autism Rating Scale but were otherwise consistent with a diagnosis of autism (Table 1). None of the autistic patients had fragile X syndrome (as determined by DNA or chromosomal analyses), had used psychotropic medication, or had a history of seizures or any additional psychiatric or neurologic diagnoses. All autistic patients were nonretarded (i.e., full-scale IQ >70), as assessed by either the Wechsler Intelligence Scale for Children—Revised (44) (patients 1 and 4) or the WAIS–R (all other patients). Neuroradiological examination showed no abnormalities in any of the patients besides borderline findings that are often associated with a diagnosis of autism (such as reduced cerebellar or parietal volume [10, 45]).
Comparison subjects were free of any history of developmental, psychiatric, or neurologic disorders. The autistic and healthy comparison groups were matched for age, sex, and handedness. Patient 3, who was ambidextrous, was matched with a left-handed comparison subject. We analyzed data for complete groups, including left-handed subjects, who performed finger movements with the left hand. Motor control conditions, which were also performed with the dominant hand, were expected to remove lateralizing effects related to side of execution. This expectation was empirically confirmed (see the Results section).
We refrained from trying to match the autism and comparison groups for IQ. Intelligence scales tap into multiple cognitive domains that are known to be impaired in autism. Since these impairments are probably an integral component of the disorder (46, 47), they should not be factored out. There appear to be only two alternatives to this approach, both of which are less desirable: 1) the inclusion of comparison groups of (borderline) retarded subjects, which is problematic given that retardation may involve diverse forms of neural pathology, and, thus, retarded subjects do not constitute “normal comparison subjects” for psychiatric populations; and 2) the exclusive study of very high-functioning autistic patients with average IQ, which may not yield results that are representative of more typical autistic populations.
The study was approved by the institutional review boards of the Children’s Hospital and the University of California, San Diego. Written informed consent was obtained from each subject, according to the procedures outlined in the Declaration of Helsinki. The 15-year-old autistic patient gave additional written informed assent.
MR Imaging
Images were acquired on a General Electric Signa 1.5-T scanner (GE Medical Systems, Milwaukee) by using a custom-made head gradient coil. Sagittal and axial localizer scans were used to select sagittal slices for echoplanar image acquisition, ensuring complete coverage of the head. After manual shimming for reduction of magnetic field inhomogeneities, echoplanar images were acquired with a single-shot gradient-recalled pulse sequence (interleaved slice acquisition; TR=2.5 seconds; TE=40 msec; flip angle=90°). The field of view was 24 cm, and 19 sagittal slices were acquired with a thickness of 7 mm (1 mm gap) and a 64×64 matrix. For each subject, the time series contained 98 echoplanar images. Phase maps were acquired for correction of echoplanar image distortions. A high-resolution structural volume was acquired in the same session by using a three-dimensional magnetization prepared rapid gradient echo pulse sequence (TR=30 msec; TE=5 msec; flip angle=45°; matrix=256×256×128; field of view=24 cm; slice thickness=1.2 mm).
Experimental Conditions
Subjects viewed a screen through an individually adjusted mirror inside the head coil. Visual stimuli were displayed on a screen approximately 12 feet from the subject’s head. Control and experimental (or task) conditions were alternated every 40 seconds in a block design (CECECE) for a total of six 40-second blocks. Each block started with the visual instruction “Press,” signaling a change in condition. Subjects viewed a diagram of a hand (roughly corresponding to the position of their own dominant hand on a four-button press device) with a blue dot appearing every 550 msec on one of the four fingers (excluding the thumb). Subjects were instructed to press the corresponding button as soon as the dot was detected. All subjects performed the movements with their preferred hand (right hand in ambidextrous patient 3). The tasks were briefly practiced immediately before the fMRI session, with digit sequences different from those used during scanning.
Experiment 1
In the control condition, the blue dot appeared every 550 msec on the representation of the index finger, and subjects pressed the corresponding button at the same frequency. In the experimental condition, a pseudorandom six-digit sequence (e.g., fingers 1-3-4-2-4-1) was repeated 10 times per 40-second block. A novel sequence was used in each block.
Experiment 2
In this experiment, the control condition was a regular sequence (fingers 1-2-3-4-3-2). The experimental condition was identical to that in experiment 1, although different digit sequences were used (i.e., subjects did not encounter any previously learned sequences).
In experiment 1, the control condition was extremely simple and included only minimal components of visuomotor coordination and learning. In experiment 2, the more complex control condition was matched to the experimental condition in terms of complexity of motor execution (use of four fingers). The control condition in experiment 2 involved slightly greater demands on visuomotor coordination than the control condition in experiment 1.
Image Preprocessing
An unwarping algorithm (48) was applied to correct for echoplanar image distortions due to magnetic field inhomogeneities by using phase maps acquired for each session. The first two time points of each series, which are typically affected by signal instability, were discarded. All image volumes were intensity normalized, motion corrected (49, 50), spatially normalized (49), and smoothed with a Gaussian kernel of 6×6×6 mm (full-width half-maximum).
Head movement prior to correction was measured by using a center-of-intensity procedure. In all subjects and on all axes, detected motion was less than 1 mm. Groupwise means for head movement were between 0.23 and 0.49 mm for all axes and both experiments. There were no significant group differences for any axis or for mean motion across all three axes (all p≥0.14, two-tailed t test). The surprisingly limited motion in autistic patients is consistent with our experience from previous studies (25, 29) that high-functioning autistic patients are often extremely cooperative after instructions have been carefully explained.
Group Analyses in Talairach Space
Hemodynamic changes associated with the alternation of task and control periods (“activations”) were statistically analyzed by means of regression against a hemodynamic ideal (51). We used a smoothed boxcar shifted by 5 seconds (to correct for hemodynamic latency) as the hemodynamic ideal. The main effects of task (i.e., fit of observed signal intensity changes with the hemodynamic model) were examined within each group. Using a factorial design, we also tested for task-by-group interactions in each experiment (cf. reference 52, p. 618). All z maps were thresholded at p≤0.05, with Bonferroni correction for multiple comparisons (based on the number of resolution elements).
Individual Analyses
Two types of analyses assessed individual variability of activation foci within each group for the most consistent regions of activation (premotor cortex [Brodmann’s area 6] and superior parietal cortex [area 7] in both hemispheres). First, we calculated the distance in stereotactic space between the strongest groupwise activation peak in a given region and the closest peak found in each individual of the respective group. For example, in experiment 1 the strongest peak in the right premotor cortex (lateral area 6) in the autism group occurred at coordinates x=42, y=–3, z=48 in stereotactic space. In patient 1, the closest significant peak occurred at x=30, y=3, z=51, resulting in a distance of 13.75 mm, calculated as follows:
In fMRI, z scores tend to vary markedly across individuals, and in a few subjects the closest peak was slightly below the Bonferroni-corrected significance threshold (p≤0.05). In these cases, we applied a slightly more liberal threshold of p≤0.0005 (uncorrected). This procedure appeared adequate because it was applied to regions of expected activation. There were no significant group differences in maximum z scores for individual subjects. This finding of generally normal blood-oxygen-level-dependent (BOLD) effects is consistent with previous studies of autistic patients (24, 25, 29).
The distance from groupwise activation peaks may underestimate the scatter of activations in autistic patients, which is expected on the basis of previous studies (25, 28, 29). Even if peaks were randomly scattered across a given region, a few would occur close to a groupwise peak by chance alone. We therefore examined spatial variability in a second way, in terms of the overall group variance of distances from the groupwise peak on each axis.
Results
Experiment 1: Group Analyses
All subjects were task compliant throughout the study, but response times and accuracy varied widely, especially in the autism group. The mean number of errors per block (button presses that did not correspond to the target sequence) was 12.2 in the autism group (SD=8.4) and 3.4 in the comparison group (SD=4.3). The mean response time was 574.1 msec in the autism group (SD=179.9) and 513.9 msec in the comparison group (SD=81.0). Group differences were significant for the number of errors (t=2.63, df=14, p<0.03) but not for response time (t=0.85, df=13, p=0.41). In the control condition, there was no significant difference in the mean number of index finger presses per block (autism group: mean=53.4, SD=24.1; comparison group: mean=59.1, SD=3.0; t=0.66, df=7, p=0.53). The mean number of errors decreased significantly from trial 1 to trial 10 within task blocks in both groups (autism group: p=0.004; comparison group: p=0.01 [paired one-tailed t tests]). Significant response time decreases were also seen in both groups (autism group: p<0.05; comparison group: p=0.003 [paired one-tailed t tests]).
Main effects of task
Both groups showed extensive frontoparietal activation, with peaks in the bilateral premotor and superior parietal, left postcentral, and right inferior frontal cortices (Table 2; Figure 1, upper left panel). Additional clusters were seen in the bilateral temporo-occipital cortex and in the thalamus and basal ganglia. Activation was also observed in the left anterior cerebellum for the comparison group and in the left inferior and middle frontal region for the autism group.
Task-by-group interaction
Several parieto-occipital regions showed significantly better voxel fit (i.e., stronger task-related hemodynamic effects) in the comparison group than in the autism group, in particular, the occipital (areas 17 and 18) and superior parietal (area 7) areas bilaterally, as well as the right middle frontal gyrus (area 9) (Table 3; Figure 1, upper right panel). Inverse effects of significantly greater activation in the autism group were found in the bilateral parietal lobes, about 20 mm inferior and posterior to those described earlier. Other clusters of greater activation in the autism group occurred in the frontal lobes, with peaks in the bilateral premotor and right medial frontal area (area 6) and in the left middle and superior frontal gyri (areas 8 and 10).
Experiment 2: Group Analyses
This experiment included a more complex control condition that was more tightly matched to the experimental condition in terms of complexity of motor execution. Nonetheless, the number of errors was significantly lower in both groups for the control condition (regular sequence) compared to the task condition (sequence learning). There were also significant performance differences between groups, both for the control and the task conditions, with greater numbers of errors in the autism group. In the learning condition, the mean number of errors per block (button presses not corresponding to the target sequence) was 11.1 (SD=9.6) in the autism group and 2.4 (SD=3.3) in the comparison group. The mean response time was 492.9 msec (SD=78.7) in the autism group and 501.1 msec (SD=68.3) in the comparison group. Group differences were significant for the number of errors (t=2.42, df=9, p<0.04) but not for response time (t=0.21, df=12, p=0.84). As in experiment 1, decreases in response time from trial 1 to trial 10 within each task block occurred in both groups (p<0.001, paired one-tailed t tests). Error means also decreased, but these differences did not reach significance (autism group: p=0.18; comparison group: p=0.08 [paired one-tailed t tests]). Mean numbers of errors and mean response times were slightly lower in both groups for experiment 2, compared to experiment 1, but these differences were not significant (all p≥0.33, paired two-tailed t tests).
Main effects of task
The comparison group showed activation clusters in the bilateral premotor, supplementary motor, and superior parietal regions (Table 2; Figure 1, lower left panel). The occipital cortex (areas 17–19) was also activated bilaterally. Smaller clusters were seen in the inferior frontal lobe, thalami, and midbrain bilaterally and in the right anterior cerebellum. In the autism group, activations occurred in the parietal (areas 7 and 40), premotor, and inferior frontal areas bilaterally and in the left prefrontal area (area 10). Peaks were also found in the occipital cortex (areas 18 and 19) and in the right middle temporal gyrus.
Task-by-group interaction
Greater activation in the comparison group compared to the autism group was found in the bilateral premotor and superior parietal cortices (Table 3; Figure 1, lower right panel). Additional effects occurred in the bilateral anterior inferior parietal and temporo-occipital regions and left anterior cerebellar regions. Inverse effects of greater activation in the autism group occurred in frontal and parieto-occipital regions, mostly in the left hemisphere. The frontal clusters were anterior to the premotor cortex (areas 8 and 10). Parietal clusters were located inferior and posterior to those showing greater activation in the comparison group.
Potential Confounds of Handedness and Performance
We performed additional analyses using data from right-handed subjects (N=5 per group). As Figure 2 shows, task effects were highly similar for the full groups and right-handed subgroups. Handedness is therefore unlikely to account for the task and group effects described earlier.
Group differences in performance constituted a further possible confound. We therefore performed all analyses using data from subjects who were approximately matched on performance, removing the two highest-performing comparison subjects and the two lowest-performing autistic patients (autistic patients 1 and 2 in experiment 1 and autistic patients 1 and 3 in experiment 2). These subgroups did not significantly differ in the number of digit sequence errors (p>0.21 in both experiments, two-tailed t tests) and in response time (p>0.60, two-tailed t tests). Figure 3 shows that the main findings for the frontal and parietal lobes for task-by-group interactions in the full groups and in the performance-matched subgroups were comparable.
Analyses of Individual Variability
As expected, spatial variability (distance between a groupwise peak in a region of interest and the closest significant peak in individual subjects) was consistently higher in the autism group than in the comparison group (Figure 4). Although the in-plane resolution in our study was high (3.75 mm), the effective slice thickness of 8 mm may have limited the detection of spatial distances. However, mean distances per region in the autism group were between 12 and 21 mm and were thus well above the spatial resolution in this study. Across both experiments and the four regions of major activation (left and right premotor and superior parietal cortex), the difference between the comparison group and the autistic group was highly significant (t=4.08, df=107, p<0.001). The difference between groups was also significant when experiment 1 (t=3.94, df=40, p<0.001) and experiment 2 (t=2.09, df=56, p<0.05) were considered separately (for single-region comparisons, see Figure 4). Greater variability of activation loci was also reflected in the greater variance of stereotactic coordinates in the autism group for each of the four regions (Figure 5).
Discussion
We examined hemodynamic effects associated with brief episodes of visually driven and fast-paced digit sequence learning in patients with autism and healthy comparison subjects. Consistent with previous studies (53–55), we identified extensive involvement of the premotor and parietal cortex. Even though activation patterns overlapped across groups, in particular in experiment 1 (Figure 1, upper left panel), significant effects of task-by-group interaction were found, mostly in the frontal and parietal lobes. With the single exception of a site in the right middle frontal gyrus (area 9), which showed greater activation in the comparison subjects in experiment 1, autistic patients showed overall stronger prefrontal involvement during visuomotor learning. This group difference was especially pronounced in experiment 2, which was less sensitive to effects of motor execution. In premotor cortex (area 6), we found similar effects in experiment 1 but inverse effects in experiment 2 (stronger activation in the comparison group).
These apparently inconsistent findings are most likely explained by more distributed and scattered cortical recruitment during simple finger movement, as previously reported (29). In experiment 1, the task was motorically more complex than the control condition. Abnormal scatter of motor activation beyond the primary motor cortex would thus explain the observed greater premotor effects in the autism group. Frontal group differences in experiment 2 can be explained analogously, again assuming scatter of functional maps in autism. The bilateral premotor cortex is a consistent site of activity related to motor learning in normal adults (53, 55–58). In autistic patients, scattering of activity related to motor execution into the premotor cortex (29) would be subtracted out in experiment 2 (because motor execution was fully balanced across the task and control conditions). Scatter of activation more specifically related to visuomotor coordination and learning, however, would be associated with reduced activation in the premotor cortex itself but abnormal recruitment of neighboring prefrontal regions anterior to the premotor cortex. This pattern of group effects was indeed observed, consistent with the general hypothesis of individually variable scatter of functional maps discussed later in this section.
Bilateral activation in the parietal cortex was found in both groups, as expected (53, 59–61) (Figure 1, upper left panel). Involvement of the superior parietal area (area 7) in normal adults can be attributed to visuospatial attention during early stages of digit sequence learning. Our group (58) recently reported bilaterally stronger activation in area 7 for early compared to later stages of digit sequence learning, associated with upregulation of visual processing in the striate and extrastriate cortices, which is also characteristic of early stages of visuomotor learning. The finding of more posterior and inferior activation in the autism group is again consistent with a general hypothesis of scattered maps. Activation scatter would result in reduced activation in the normal superior parietal and occipital foci but abnormal recruitment of cortical tissue between these regions (Figure 6).
It might be argued that the premotor and parietal activations identified in this study are solely linked to visuomotor coordination, rather than to learning (62). Visuomotor coordination is crucial during early stages of visually prompted sequence acquisition. Nonetheless, even with only 10 trials per six-digit sequence, performance improved within blocks in terms of errors and response times in both groups. These findings suggest that learning indeed occurred.
Each group included three non-right-handed subjects. In additional analyses of data for right-handed subgroups (N=5 in each group), the main effects of task were very similar to those for the full groups, albeit slightly weaker (due to reduced statistical power) (Figure 2). Specifically, effects showed no laterality differences, suggesting that our main findings were not affected by handedness or side of movement. Finally, to rule out potential confounds of task performance, we analyzed data for subgroups that were grossly matched for response times and errors (N=6 per group). Despite some minor differences, the main findings for the frontal and parietal lobes described earlier were consistent (Figure 3). It should be noted that similar levels of performance in these subgroups cannot absolutely guarantee absence of confounds regarding, for example, attention, general arousal, or differences in cognitive strategies.
Convergent Evidence for Frontoparietal Abnormalities in Autism
The finding of activation abnormalities in the frontal and parietal lobes in autism is not unexpected. Parietal involvement in autism is suggested by cholinergic abnormalities (63) and by association of parietal hypoplasia (45) with visuospatial attention deficits (40, 64, 65). Our task paradigm required visual attention to a spatially displayed digit sequence. Reduced activity in superior parietal loci that were activated in normal adults could be related to impairments of visuospatial attention in autism. However, a deficit interpretation alone would not account for enhanced activation in more inferior and posterior loci in the autism group (see later discussion).
Frontal involvement in autism has been hypothesized on the basis of neuropsychological evidence of “executive” deficits (47, 66–68), cytoarchitectonic abnormalities (69), neuroimaging studies showing reduced blood flow (26, 70, 71) and serotonin metabolism (15), and abnormal event-related potentials at frontal sites for attentional tasks (41, 72). Again, however, such evidence of frontal impairment in autism does not readily account for our finding of greater-than-normal prefrontal involvement in motor learning.
A Developmental Account
In each of the few published functional mapping studies of autism and Asperger’s disorder (20–23, 25, 29, 65, 73), significant differences from normal hemodynamic responses to a variety of tasks have been observed. Such abnormalities support the expectation that autistic pathogenesis is associated with neurofunctional disturbances. However, ascertaining neurological abnormality in a disorder that by consensus is neurally based is not truly enlightening. Some studies have demonstrated reduced or absent activation in regions that are functionally well characterized in normal brains, such as the fusiform gyrus for face perception (24, 25) or the amygdala for emotional processing (21). Even though such findings may be linked to respective deficits in autism, the view that recruitment of differently localized cortical tissue would necessarily reflect functional impairment remains an assumption. Given our insufficient understanding of neurofunctional organization in autism and the known multipotential qualities of developing cortical tissue (see later discussion), this assumption is speculative. In addition, the vast array of sensorimotor and cognitive disturbances observed in autism makes it unlikely that this disorder can be neurofunctionally described in terms of a localized or “modular” deficit.
In view of the pervasive developmental nature of the disorder, the question of abnormal neurofunctional maps observed in autistic adults must be approached developmentally: How do functional maps differentiate in normal development, and how do developmental disturbances in autism interfere with this normal differentiation? Since fMRI studies of autistic patients at the time of initial pathogenesis (prenatal or early postnatal) are impractical, developmental considerations can apply only post hoc. Autism is a behaviorally defined psychiatric disorder, and diagnostic criteria may not correspond to a unique developmental pathology. On the contrary, the diversity of findings in structural (5, 74) and functional imaging (19) and in genetic linkage studies (75, 76) suggests that multiple etiological pathways result in phenotypes captured under the diagnostic umbrella term of autism. In this context, it becomes crucial for neuroimaging studies to examine data on an individual level. To our knowledge, only three studies have attempted this approach.
A study of face perception (25) showed that absence of normal activation (in the fusiform gyrus) on groupwise analyses does not imply absence of activation in individual autistic patients. Instead, the majority of patients in this study showed activation in individually varying loci around the fusiform gyrus. Our previous study of index finger movement (29) demonstrated abnormal individual variability of activations in autism for simple motor control, with individual maps showing scattered activation beyond the perirolandic cortex. Very similar effects were also observed in the cerebellum in a separate study of simple thumb movement (28). Among the three previous studies examining fMRI findings on a patient-by-patient basis, there was thus complete convergence indicating individually variable scatter of activation beyond the foci seen in normal subjects. In the present study, we again found overall significantly greater spatial variability of activation foci in the autism group, compared to the healthy subjects (Figure 5 and Figure 6), which supports our hypothesis that such variability may be a general characteristic of neurofunctional organization in autism and may directly reflect developmental disturbances.
Despite important genetic influences on brain morphology (77) and cognitive abilities (78), normal neocortical differentiation into functionally specialized regions is not strictly predetermined genetically (79, 80). Crossmodal plasticity of developing cortical tissue has been demonstrated in animal models (81, 82) and in human imaging studies (83, 84). Animal studies have further established that thalamocortical afferents play an important role in such differentiation (81), as well as in lesion-induced cortical plasticity (85). Intact thalamocortical afferents are thus a prerequisite for the normal development of cerebral cortical functional maps. There is some, as yet limited, evidence for thalamic abnormalities in autism (15, 26, 86–89). It is known that the thalamus participates in extensive cerebello-thalamo-cortical pathways reaching almost all cerebral cortical regions, including association cortex (90). Among the many sites of neurological abnormality proposed in autism research, the cerebellum has been identified through convergent evidence, including findings of postmortem (17), volumetric (10, 91–93), neurochemical (15, 16, 94), behavioral (95), and activation studies (26, 27, 65). Postmortem histology suggests that the most prominent cerebellar abnormality is a reduction in Purkinje cell numbers (17, 96). Fatemi and colleagues (94) observed reduced levels of reelin and Bcl-2 protein in autistic postmortem cerebella. These proteins play important roles in migration and cortical lamination (reelin) and apoptosis (Bcl-2). The postmortem findings from adult brains do not establish whether similar regulatory protein reduction could explain developmental abnormalities in the autistic cerebellum. However, an absence of “empty baskets” suggests that Purkinje cell reduction in adults reflects maldevelopment rather than post hoc loss (17, 97). Growth interaction between basket cells and Purkinje cells occurs before the differentiation of Purkinje cells is completed (around postnatal day 8 in the rat [98], roughly corresponding to the end of the second trimester in human gestation [99]). Inhibitory synapses of Purkinje cells in deep cerebellar nuclei probably form around the beginning of the third trimester in humans (99, 100). In the thalamus, afferents from deep cerebellar nuclei are present at birth in the rat, i.e., preceding the events described earlier (101). Early Purkinje cell loss in autism is thus likely to affect developing cerebello-thalamo-cortical connectivity and cerebral cortical functional differentiation, which may explain abnormally scattered fMRI activation patterns, as observed here.
Representational scatter in autism (as depicted in Figure 6) may imply phenomena analogous to those of “crowding” suggested for lesion-induced interhemispheric reorganization (102, 103). Currently limited fMRI evidence suggests that ontogenetically earlier functions (e.g., simple motor control, visual perception) invade brain areas normally involved in more complex multimodal processing. The opposite is not observed. For instance, we did not see greater-than-normal activity in the primary motor cortex associated with visuomotor coordination. Instead, scatter was directed toward the prefrontal cortices, i.e., the relatively late-maturing cortices that normally assume higher “executive” functions. Our hypothesis implies that due to impaired cerebral cortical differentiation (caused at least partly by disturbances in thalamocortical afferents), early-developing sensorimotor functions require a larger cortical processing territory. Such territory will subsequently be less available to later-developing complex multimodal processing (e.g., language, executive functions). This hypothesis of hierarchicalcrowding thus implies that more elementary functions impinge on more complex ones in the developing autistic brain.
This scenario is not a fully comprehensive model of autistic neuropathology. Relatively elementary functions (such as motor dexterity, auditory perception, spatial attention) are often impaired in autism, whereas some high-functioning patients, including those with Asperger’s disorder, perform well in higher cognitive domains. The hypothesis of hierarchical crowding does not address potential effects of compensatory neuroplasticity, known from the literature on patients with early brain damage (104, 105). Furthermore, given the plethora of pathological findings in autism, additional etiological mechanisms besides early Purkinje cell reduction and hypothesized cerebello-thalamo-cortical disturbances—such as diffuse growth disturbances (91, 106)—are likely to play important roles.
It should be noted that the proposed model has been prompted by imaging findings in rather small groups of high-functioning autistic patients, and replication will be required. Nonetheless, our model generates a number of hypotheses that may inform future fMRI work. To examine these hypotheses, it will be important to go beyond the customary procedure of groupwise analyses and examine the autistic brain on an individual level. First, our model implies predictions for fMRI studies on elementary and complex cognitive functions in various domains and modalities. Second, the model would predict functional impairment of the thalami beyond the currently limited evidence. Third, it is predicted that axonal pathways connecting cerebellar deep nuclei, the thalamus, and the cerebral cortex are affected.
![]() |
![]() |
![]() |
Presented in part at the First International Meeting for Autism Research, San Diego, Nov. 9–10, 2001. Received Sept. 23, 2002; revision received April 16, 2003; accepted April 24, 2003. From the Department of Psychology, San Diego State University; the Laboratory for the Neuroscience of Autism, Children’s Hospital Research Center, La Jolla, Calif.; the Departments of Cognitive Science and Neurosciences, University of California, San Diego, La Jolla, Calif.; and the Joint Doctoral Program in Clinical Psychology, San Diego State University and University of California, San Diego. Address reprint requests to Dr. Müller, Department of Psychology, San Diego State University, MC1863, San Diego, CA 92120; [email protected]. Supported by NIMH grant MH-36840 (Dr. Courchesne) and grant DC-6155 from the National Institute on Deafness and Other Communication Disorders (Dr. Müller). The authors thank Greg Allen, Natasha Akshoomoff, Marissa Westerfield, Miguel Rubio, Lynn Lord, Lisa Eyler Zorilla, and Craig Stark for their assistance.
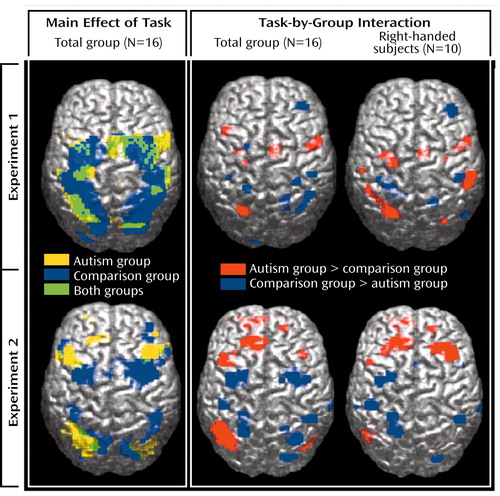
Figure 1. Surface Rendering of Brain Regions With Significant Activation in Healthy Comparison Subjects (N=8) and Patients With Autism (N=8) During Two Visuomotor Sequence Learning Task Experimentsa
aSignificant effects of task and task-by-group interaction (p≤0.05, general linear tests with Bonferroni correction).
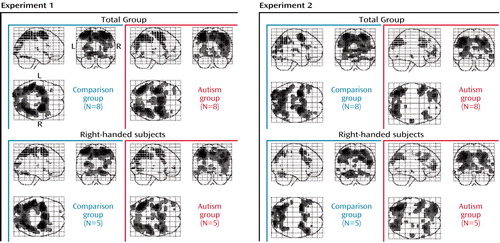
Figure 2. Brain Regions With Significant Activation in Healthy Comparison Subjects and Patients With Autism and in Right-Handed Subgroups During Two Visuomotor Sequence Learning Task Experimentsa
aSignificant effects of task (p≤0.05, general linear tests with Bonferroni correction).
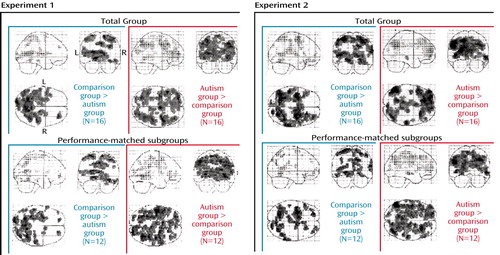
Figure 3. Brain Regions With Relatively Greater Activation in Healthy Comparison Subjects and Patients With Autism and in Performance-Matched Subgroups During Two Visuomotor Sequence Learning Task Experimentsa
aSignificant effects of task-by-group interaction (p<0.01, general linear tests uncorrected).
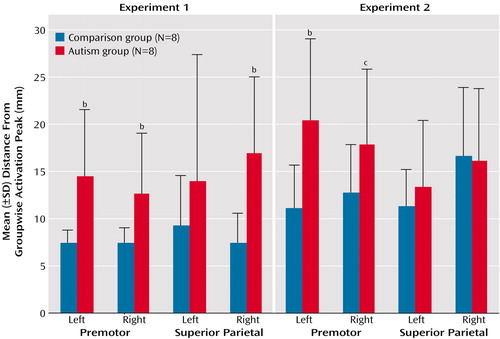
Figure 4. Individual Spatial Variability of Activation Peaks in the Premotor and Superior Parietal Cortices in Healthy Comparison Subjects and Patients With Autism During Two Visuomotor Sequence Learning Task Experimentsa
aSpatial variability calculated as the distance between the groupwise activation peak and the closest significant peak in individual subjects in three-dimensional normalized space. The groupwise reference peaks for each experiment and region are identified in Table 2.
bSignificantly greater spatial variability in the autism group (p<0.05, two-tailed t test).
cGreater spatial variability in the autism group (p<0.08, two-tailed t test).
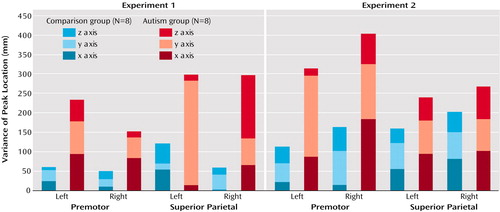
Figure 5. Variance of Stereotactic Coordinates of Activation Peaks in the Premotor and Superior Parietal Cortices in Healthy Comparison Subjects and Patients With Autism During Two Visuomotor Sequence Learning Task Experiments
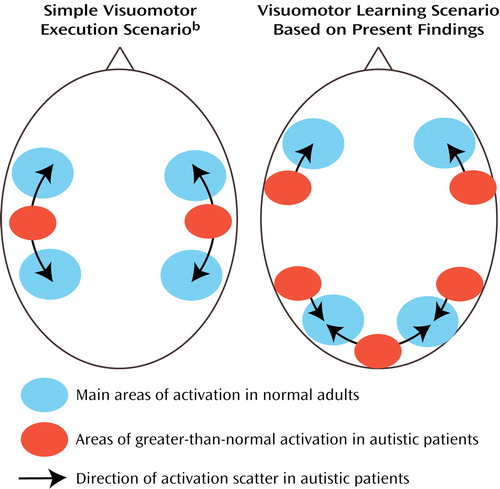
Figure 6. Schematic Representation of Brain Regions With Significant Activation During Visuomotor Execution and Visuomotor Learning Showing Hypothesized Effects of Scattered Functional Maps in Autisma
aActivation scatter in autistic patients results in reduced activation in normal superior parietal and occipital foci and abnormal activation of cortical tissue between these regions.
bBased on earlier findings of Müller et al. (29).
1. Tager-Flusberg H, Joseph R, Folstein S: Current directions in research on autism. Ment Retard Dev Disabil Res Rev 2001; 7:21–29Crossref, Medline, Google Scholar
2. Bryson SE: Epidemiology of autism: overview and issues outstanding, in Handbook of Autism and Pervasive Developmental Disorders. Edited by Cohen DJ, Volkmar FR. New York, John Wiley & Sons, 1997, pp 41–46Google Scholar
3. Rapin I: Autism. N Engl J Med 1997; 337:97–104Crossref, Medline, Google Scholar
4. Folstein SE, Rosen-Sheidley B: Genetics of autism: complex aetiology for a heterogeneous disorder. Nat Rev Genet 2001; 2:943–955Crossref, Medline, Google Scholar
5. Cody H, Pelphrey K, Piven J: Structural and functional magnetic resonance imaging of autism. Int J Dev Neurosci 2002; 20:421–438Crossref, Medline, Google Scholar
6. Piven J, Saliba K, Bailey J, Arndt S: An MRI study of autism: the cerebellum revisited. Neurology 1997; 49:546–551Crossref, Medline, Google Scholar
7. Holttum JR, Minshew NJ, Sanders RS, Phillips NE: Magnetic resonance imaging of the posterior fossa in autism. Biol Psychiatry 1992; 32:1091–1101Crossref, Medline, Google Scholar
8. Haas RH, Townsend J, Courchesne E, Lincoln AJ, Schreibman L, Yeung-Courchesne R: Neurologic abnormalities in infantile autism. J Child Neurol 1996; 11:84–92Crossref, Medline, Google Scholar
9. Hallett M, Lebiedowska MK, Thomas SL, Stanhope SJ, Denckla MB, Rumsey J: Locomotion of autistic adults. Arch Neurol 1993; 50:1304–1308Crossref, Medline, Google Scholar
10. Hashimoto T, Tayama M, Murakawa K, Yoshimoto T, Miyazaki M, Harada M, Kuroda Y: Development of the brainstem and cerebellum in autistic patients. J Autism Dev Disord 1995; 25:1–18Crossref, Medline, Google Scholar
11. Fatemi SH, Halt AR, Stary JM, Realmuto GM, Jalali-Mousavi M: Reduction in anti-apoptotic protein Bcl-2 in autistic cerebellum. Neuroreport 2001; 12:929–933Crossref, Medline, Google Scholar
12. Otsuka H, Harada M, Mori K, Hisaoka S, Nishitani H: Brain metabolites in the hippocampus-amygdala region and cerebellum in autism: an 1H-MR spectroscopy study. Neuroradiology 1999; 41:517–519Crossref, Medline, Google Scholar
13. Mostofsky SH, Goldberg MC, Landa RJ, Denckla MB: Evidence for a deficit in procedural learning in children and adolescents with autism: implications for cerebellar contribution. J Int Neuropsychol Soc 2000; 6:752–759Crossref, Medline, Google Scholar
14. Weber AM, Egelhoff JC, McKellop JM, Franz DN: Autism and the cerebellum: evidence from tuberous sclerosis. J Autism Dev Disord 2000; 30:511–517Crossref, Medline, Google Scholar
15. Chugani DC, Muzik O, Rothermel RD, Behen ME, Chakraborty PK, Mangner TJ, da Silva EA, Chugani HT: Altered serotonin synthesis in the dentatothalamocortical pathway in autistic boys. Ann Neurol 1997; 14:666–669Crossref, Google Scholar
16. Chugani DC, Sundram BS, Behen M, Lee ML, Moore GJ: Evidence of altered energy metabolism in autistic children. Prog Neuropsychopharmacol Biol Psychiatry 1999; 23:635–641Crossref, Medline, Google Scholar
17. Bailey A, Luthert P, Dean A, Harding B, Janota I, Montgomery M, Rutter M, Lantos P: A clinicopathological study of autism. Brain 1998; 121:889–905Crossref, Medline, Google Scholar
18. Murakami JW, Courchesne E, Press GA, Yeung-Courchesne R, Hesselink JR: Reduced cerebellar hemisphere size and its relationship to vermal hypoplasia in autism. Arch Neurol 1989; 46:689–694Crossref, Medline, Google Scholar
19. Rumsey JM, Ernst M: Functional neuroimaging of autistic disorders. Ment Retard Dev Disabil Res Rev 2000; 6:171–179Crossref, Medline, Google Scholar
20. Happé F, Ehlers S, Fletcher PC, Frith U, Johansson M, Gillberg C, Dolan RJ, Frackowiak RSJ, Frith CD: “Theory of mind” in the brain: evidence from a PET scan study of Asperger syndrome. Neuroreport 1996; 8:197–201Crossref, Medline, Google Scholar
21. Baron-Cohen S, Ring HA, Wheelwright S, Bullmore ET, Brammer MJ, Simmons A, Williams SC: Social intelligence in the normal and autistic brain: an fMRI study. Eur J Neurosci 1999; 11:1891–1898Crossref, Medline, Google Scholar
22. Castelli F, Frith C, Happé F, Frith U: Autism, Asperger syndrome and brain mechanisms for the attribution of mental states to animated shapes. Brain 2002; 125(part 8):1839–1849Google Scholar
23. Critchley HD, Daly EM, Bullmore ET, William SCR, van Amelsvoort T, Robertson DM, Rowe A, Phillips M, McAlonan G, Howlin P, Murphy DG: The functional neuroanatomy of social behavior: changes in cerebral blood flow when people with autistic disorder process facial expressions. Brain 2000; 123:2203–2212Crossref, Medline, Google Scholar
24. Schultz RT, Gauthier I, Klin A, Fulbright RK, Anderson AW, Volkmar F, Skudlarski P, Lacadie C, Cohen DJ, Gore JC: Abnormal ventral temporal cortical activity during face discrimination among individuals with autism and Asperger syndrome. Arch Gen Psychiatry 2000; 57:331–340Crossref, Medline, Google Scholar
25. Pierce K, Müller R-A, Ambrose JB, Allen G, Courchesne E: Face processing occurs outside the “fusiform face area” in autism: evidence from functional MRI. Brain 2001; 124:2059–2073Crossref, Medline, Google Scholar
26. Müller R-A, Chugani DC, Behen ME, Rothermel RD, Muzik O, Chakraborty PK, Chugani HT: Impairment of dentato-thalamo-cortical pathway in autistic men: language activation data from positron emission tomography. Neurosci Lett 1998; 245:1–4Crossref, Medline, Google Scholar
27. Müller R-A, Behen ME, Rothermel RD, Chugani DC, Muzik O, Mangner TJ, Chugani HT: Brain mapping of language and auditory perception in high-functioning autistic adults: a PET study. J Autism Dev Disord 1999; 29:19–31Crossref, Medline, Google Scholar
28. Allen G, Müller R-A, Courchesne E: Imaging motor function in the autistic cerebellum. Society for Neuroscience Abstracts 1998; 24:1519Google Scholar
29. Müller R-A, Pierce K, Ambrose JB, Allen G, Courchesne E: Atypical patterns of cerebral motor activation in autism: a functional magnetic resonance study. Biol Psychiatry 2001; 49:665–676Crossref, Medline, Google Scholar
30. Rinehart NJ, Bradshaw JL, Brereton AV, Tonge BJ: Movement preparation in high-functioning autism and Asperger disorder: a serial choice reaction time task involving motor reprogramming. J Autism Dev Disord 2001; 31:79–88Crossref, Medline, Google Scholar
31. Hughes C: Brief report: planning problems in autism at the level of motor control. J Autism Dev Disord 1996; 26:99–107Crossref, Medline, Google Scholar
32. Szatmari P, Tuff L, Finlayson MA, Bartolucci G: Asperger’s syndrome and autism: neurocognitive aspects. J Am Acad Child Adolesc Psychiatry 1990; 29:130–136Crossref, Medline, Google Scholar
33. Bauman ML: Motor dysfunction in autism, in Movement Disorders in Neurology and Psychiatry. Edited by Joseph AB, Young RR. Boston, Blackwell, 1992, pp 660–663Google Scholar
34. Jones V, Prior M: Motor imitation abilities and neurological signs in autistic children. J Autism Dev Disord 1985; 15:37–45Crossref, Medline, Google Scholar
35. Ghaziuddin M, Butler E: Clumsiness in autism and Asperger syndrome: a further report. J Intellect Disabil Res 1998; 42(part 1):43–48Google Scholar
36. Teitelbaum P, Teitelbaum O, Nye J, Fryman J, Maurer RG: Movement analysis in infancy may be useful for early diagnosis of autism. Proc Natl Acad Sci USA 1998; 95:13982–13987Crossref, Medline, Google Scholar
37. Hepper PG: The behavior of the fetus as an indicator of neural functioning, in Fetal Development. Edited by Lecanuet J-P, Fifer WP, Krasnegor NA, Smotherman WP. Hillsdale, NJ, Lawrence Erlbaum Associates, 1995, pp 405–417Google Scholar
38. Forssberg H: Neural control of human motor development. Curr Opin Neurobiol 1999; 9:676–682Crossref, Medline, Google Scholar
39. Carper RA, Courchesne E: Frontal lobe volume in children with autism. Brain 2000; 123:836–844Crossref, Medline, Google Scholar
40. Townsend J, Courchesne E: Parietal damage and narrow “spotlight” spatial attention. J Cogn Neurosci 1994; 6:220–232Crossref, Medline, Google Scholar
41. Townsend J, Westerfield M, Leaver E, Makeig S, Jung T, Pierce K, Courchesne E: Event-related brain response abnormalities in autism: evidence for impaired cerebello-frontal spatial attention networks. Brain Res Cogn Brain Res 2001; 11:127–145Crossref, Medline, Google Scholar
42. Schopler E, Reichler RJ, DeVellis RF, Daly K: Toward objective classification of childhood autism: Childhood Autism Rating Scale (CARS). J Autism Dev Disord 1980; 10:91–103Crossref, Medline, Google Scholar
43. Lord C, Rutter M, Le Couteur A: Autism Diagnostic Interview—Revised: a revised version of a diagnostic interview for caregivers of individuals with possible pervasive developmental disorders. J Autism Dev Disord 1994; 24:659–685Crossref, Medline, Google Scholar
44. Wechsler D: Wechsler Intelligence Scale for Children. San Antonio, Tex, Harcourt Brace Jovanovich, 1989Google Scholar
45. Courchesne E, Press GA, Yeung-Courchesne R: Parietal lobe abnormalities detected with MR in patients with infantile autism. AJR Am J Roentgenol 1993; 160:387–393Crossref, Medline, Google Scholar
46. Ozonoff S: Advances in the cognitive neuroscience of autism, in Handbook of Developmental Cognitive Neuroscience. Edited by Nelson CA, Luciana M. Cambridge, Mass, MIT Press, 2001, pp 537–48Google Scholar
47. Minshew NJ, Goldstein G, Siegel DJ: Neuropsychologic functioning in autism: profile of a complex information processing disorder. J Int Neuropsychol Soc 1997; 3:303–316Crossref, Medline, Google Scholar
48. Reber P: Correction of off resonance-related distortion in echo-planar imaging using EPI-based field maps. Magn Reson Med 1998; 39:328–330Crossref, Medline, Google Scholar
49. Friston KJ, Ashburner J, Frith CD, Poline J-B, Heather JD, Frackowiak RSJ: Spatial registration and normalization of images. Hum Brain Mapp 1995; 2:165–189Crossref, Google Scholar
50. Friston KJ, Williams S, Howard R, Frackowiak RS, Turner R: Movement-related effects in fMRI time-series. Magn Reson Med 1996; 35:346–355Crossref, Medline, Google Scholar
51. Holmes A, Poline JB, Friston KJ: Characterizing brain images with the general linear model, in Human Brain Function. Edited by Frackowiak RSJ, Friston KJ, Frith CD, Dolan RJ, Mazziotta JC. San Diego, Academic Press, 1997, pp 59–84Google Scholar
52. Friston KJ: Statistical parametric mapping, in Brain Mapping: The Methods. Edited by Toga A, Mazziotta JC. Orlando, Fla, Academic Press, 2002, pp 605–631Google Scholar
53. Jenkins IH, Brooks DJ, Nixon PD, Frackowiak RS, Passingham RE: Motor sequence learning: a study with positron emission tomography. J Neurosci 1994; 14:3775–3790Crossref, Medline, Google Scholar
54. Seitz RJ, Roland PE, Bohm C, Greitz T, Stone-Elander S: Motor learning in man: a positron emission tomography study. Neuroreport 1990; 1:17–20Crossref, Medline, Google Scholar
55. Toni I, Krams M, Turner R, Passingham RE: The time course of changes during motor sequence learning: a whole-brain fMRI study. Neuroimage 1998; 8:50–61Crossref, Medline, Google Scholar
56. Seitz RJ, Canavan AG, Yaguez L, Herzog H, Tellmann L, Knorr U, Huang Y, Homberg V: Successive roles of the cerebellum and premotor cortices in trajectorial learning. Neuroreport 1994; 5:2541–2544Crossref, Medline, Google Scholar
57. Doyon J, Owen AM, Petrides M, Sziklas V, Evans AC: Functional anatomy of visuomotor skill learning in human subjects examined with positron emission tomography. Eur J Neurosci 1996; 8:637–648Crossref, Medline, Google Scholar
58. Müller R-A, Pierce K, Kleinhans N, Kemmotsu N, Courchesne E: Functional MRI of motor sequence learning: effects of learning stage and performance. Brain Res Cogn Brain Res 2002; 14:277–293Crossref, Medline, Google Scholar
59. Deiber MP, Wise SP, Honda M, Catalan MJ, Grafman J, Hallett M: Frontal and parietal networks for conditional motor-learning: a positron emission tomography study. J Neurophysiol 1997; 78:977–991Crossref, Medline, Google Scholar
60. van Mier H, Tempel LW, Perlmutter JS, Raichle ME, Petersen SE: Changes in brain activity during motor learning measured with PET: effects of hand of performance and practice. J Neurophysiol 1998; 80:2177–2199Crossref, Medline, Google Scholar
61. Schlaug G, Knorr U, Seitz R: Inter-subject variability of cerebral activations in acquiring a motor skill: a study with positron emission tomography. Exp Brain Res 1994; 98:523–534Crossref, Medline, Google Scholar
62. Iacoboni M, Woods RP, Mazziotta JC: Bimodal (auditory and visual) left frontoparietal circuitry for sensorimotor integration and sensorimotor learning. Brain 1998; 121(part 11):2135–2143Google Scholar
63. Perry EK, Lee MLW, Martin-Ruiz CM, Court JA, Volsen SG, Merrit J, Folly E, Iversen PE, Bauman ML, Perry RH, Wenk GL: Cholinergic activity in autism: abnormalities in the cerebral cortex and basal forebrain. Am J Psychiatry 2001; 158:1058–1066Link, Google Scholar
64. Townsend J, Courchesne E, Egaas B: Slowed orienting of covert visual-spatial attention in autism: specific deficits associated with cerebellar and parietal abnormality. Dev Psychopathol 1996; 8:563–584Crossref, Google Scholar
65. Allen G, Courchesne E: Differential effects of developmental cerebellar abnormality on cognitive and motor functions in the cerebellum: an fMRI study of autism. Am J Psychiatry 2003; 160:262–273Link, Google Scholar
66. Hughes C, Russell J, Robbins TW: Evidence for executive dysfunction in autism. Neuropsychologia 1994; 32:477–492Crossref, Medline, Google Scholar
67. Ciesielski KT, Harris RJ: Factors related to performance failure on executive tasks in autism. Child Neuropsychol 1997; 3:1–12Crossref, Google Scholar
68. Pennington BF, Ozonoff S: Executive functions and developmental psychopathology. J Child Psychol Psychiatry 1996; 37:51–87Crossref, Medline, Google Scholar
69. Casanova MF, Buxhoeveden DP, Switala AE, Roy E: Minicolumnar pathology in autism. Neurology 2002; 58:428–432Crossref, Medline, Google Scholar
70. Zilbovicius M, Garreau B, Samson Y, Remy P, Barthélémy C, Syrota A, Lelord G: Delayed maturation of the frontal cortex in childhood autism. Am J Psychiatry 1995; 152:248–252Link, Google Scholar
71. George MS, Costa DC, Kouris K, Ring HA, Ell PJ: Cerebral blood flow abnormalities in adults with infantile autism. J Nerv Ment Dis 1992; 180:413–417Crossref, Medline, Google Scholar
72. Ciesielski KT, Courchesne E, Elmasian R: Effects of focused selective attention tasks on event-related potentials in autistic and normal individuals. Electroencephalogr Clin Neurophysiol 1990; 75:207–220Crossref, Medline, Google Scholar
73. Ring HA, Baron-Cohen S, Wheelwright S, Williams SC, Brammer M, Andrew C, Bullmore ET: Cerebral correlates of preserved cognitive skills in autism: a functional MRI study of embedded figures task performance. Brain 1999; 122(part 7):1305–1315Google Scholar
74. Akshoomoff N, Pierce K, Courchesne E: The neurobiological basis of autism from a developmental perspective. Dev Psychopathol 2002; 14:613–634Crossref, Medline, Google Scholar
75. Jones MB, Szatmari P: A risk-factor model of epistatic interaction, focusing on autism. Am J Med Genet 2002; 114:558–565Crossref, Medline, Google Scholar
76. Shao Y, Wolpert CM, Raiford KL, Menold MM, Donnelly SL, Ravan SA, Bass MP, McClain C, von Wendt L, Vance JM, Abramson RH, Wright HH, Ashley-Koch A, Gilbert JR, DeLong RG, Cuccaro ML, Pericak-Vance MA: Genomic screen and follow-up analysis for autistic disorder. Am J Med Genet 2002; 114:99–105Crossref, Medline, Google Scholar
77. Thompson PM, Cannon TD, Narr KL, van Erp T, Poutanen VP, Huttunen M, Lonnqvist J, Standertskjold-Nordenstam CG, Kaprio J, Khaledy M, Dail R, Zoumalan CI, Toga AW: Genetic influences on brain structure. Nat Neurosci 2001; 4:1253–1258Crossref, Medline, Google Scholar
78. Plomin R, Kosslyn SM: Genes, brain and cognition. Nat Neurosci 2001; 4:1153–1154Crossref, Medline, Google Scholar
79. Pallas SL: Intrinsic and extrinsic factors that shape neocortical specification. Trends Neurosci 2001; 24:417–423Crossref, Medline, Google Scholar
80. O’Leary DD, Nakagawa Y: Patterning centers, regulatory genes and extrinsic mechanisms controlling arealization of the neocortex. Curr Opin Neurobiol 2002; 12:14–25Crossref, Medline, Google Scholar
81. Schlaggar B, O’Leary D: Potential of visual cortex to develop an array of functional units unique to somatosensory cortex. Science 1991; 252:1556–1560Crossref, Medline, Google Scholar
82. von Melchner L, Pallas SL, Sur M: Visual behaviour mediated by retinal projections directed to the auditory pathway. Nature 2000; 404:871–876Crossref, Medline, Google Scholar
83. Sadato N, Pascual-Leone A, Grafman J, Deiber M, Ibanez V, Hallett M: Neural networks for Braille reading by the blind. Brain 1998; 121:1213–1229Crossref, Medline, Google Scholar
84. Finney EM, Fine I, Dobkins KR: Visual stimuli activate auditory cortex in the deaf. Nat Neurosci 2001; 4:1171–1173Crossref, Medline, Google Scholar
85. Stojic AS, Lane RD, Killackey HP, Qadri BA, Rhoades RW: Thalamocortical and intracortical projections to the forelimb-stump SI representation of rats that sustained neonatal forelimb removal. J Comp Neurol 1998; 401:187–204Crossref, Medline, Google Scholar
86. Friedman SD, Shaw DW, Artru AA, Richards TL, Gardner J, Dawson G, Posse S, Dager SR: Regional brain chemical alterations in young children with autism spectrum disorder. Neurology 2003; 60:100–107Crossref, Medline, Google Scholar
87. Horwitz B, Rumsey JM, Grady CL, Rapoport SI: The cerebral metabolic landscape in autism: intercorrelations of regional glucose utilization. Arch Neurol 1988; 45:749–755Crossref, Medline, Google Scholar
88. Ryu YH, Lee JD, Yoon PH, Kim DI, Lee HB, Shin YJ: Perfusion impairments in infantile autism on technetium-99m ethyl cysteinate dimer brain single-photon emission tomography: comparison with findings on magnetic resonance imaging. Eur J Nucl Med 1999; 26:253–259Crossref, Medline, Google Scholar
89. Starkstein SE, Vazquez S, Vrancic D, Nanclares V, Manes F, Piven J, Plebst C: SPECT findings in mentally retarded autistic individuals. J Neuropsychiatry Clin Neurosci 2000; 12:370–375Crossref, Medline, Google Scholar
90. Schmahmann JD: From movement to thought: anatomic substrates of the cerebellar contribution to cognitive processing. Hum Brain Mapp 1996; 4:174–198Crossref, Medline, Google Scholar
91. Courchesne E, Karns CM, Davis HR, Ziccardi R, Carper RA, Tigue ZD, Chisum HJ, Moses P, Pierce K, Lord C, Lincoln AJ, Pizzo S, Schreibman L, Haas RH, Akshoomoff NA, Courchesne RY: Unusual brain growth patterns in early life in patients with autistic disorder: an MRI study. Neurology 2001; 57:245–254Crossref, Medline, Google Scholar
92. Courchesne E, Yeung-Courchesne R, Press GA, Hesselink JR, Jernigan TL: Hypoplasia of cerebellar vermal lobules VI and VII in autism. N Engl J Med 1988; 318:1349–1354Crossref, Medline, Google Scholar
93. Hardan AY, Minshew NJ, Harenski K, Keshavan MS: Posterior fossa magnetic resonance imaging in autism. J Am Acad Child Adolesc Psychiatry 2001; 40:666–672Crossref, Medline, Google Scholar
94. Fatemi SH, Stary JM, Halt AR, Realmuto GR: Dysregulation of reelin and Bcl-2 proteins in autistic cerebellum. J Autism Dev Disord 2001; 31:529–535Crossref, Medline, Google Scholar
95. Pierce K, Courchesne E: Evidence for a cerebellar role in reduced exploration and stereotyped behavior in autism. Biol Psychiatry 2001; 49:655–664Crossref, Medline, Google Scholar
96. Bauman ML, Kemper TL: Developmental cerebellar abnormalities: a consistent finding in early infantile autism (abstract). Neurology 1986; 36(suppl 1):190Google Scholar
97. Courchesne E: Brainstem, cerebellar and limbic neuroanatomical abnormalities in autism. Curr Opin Neurobiol 1997; 7:269–278Crossref, Medline, Google Scholar
98. Altman J, Bayer SA: Development of the Cerebellar System. Boca Raton, Fla, CRC Press, 1997Google Scholar
99. Clancy B, Darlington RB, Finlay BL: Translating developmental time across mammalian species. Neuroscience 2001; 105:7–17Crossref, Medline, Google Scholar
100. Garin N, Escher G: The development of inhibitory synaptic specializations in the mouse deep cerebellar nuclei. Neuroscience 2001; 105:431–441Crossref, Medline, Google Scholar
101. Asanuma C, Ohkawa R, Stanfield BB, Cowan WM: Observations on the development of certain ascending inputs to the thalamus in rats, I: postnatal development. Brain Res 1988; 469:159–170Crossref, Medline, Google Scholar
102. Strauss E, Satz P, Wada J: An examination of the crowding hypothesis in epileptic patients who have undergone the carotid Amytal test. Neuropsychologia 1990; 28:1221–1227Crossref, Medline, Google Scholar
103. Nass R, Sadler AE, Sidtis JJ: Differential effects of congenital versus acquired unilateral brain injury on dichotic listening performance: evidence for sparing and asymmetric crowding. Neurology 1992; 42:1960–1965Crossref, Medline, Google Scholar
104. Bates E: Plasticity, localization and language development, in The Changing Nervous System: Neurobehavioral Consequences of Early Brain Disorders, vol 13. Edited by Broman SH, Fletcher JM. New York, Oxford University Press, 1999, pp 214–253Google Scholar
105. Müller R-A, Courchesne E: The duplicity of plasticity: a conceptual approach to the study of early lesion and developmental disorders, in The Foundation and Future of Functional Neuroimaging in Child Psychiatry. Edited by Ernst M, Rumsey J. New York, Cambridge University Press, 2000, pp 335–365Google Scholar
106. Nelson KB, Grether JK, Croen LA, Dambrosia JM, Dickens BF, Jelliffe LL, Hansen RL, Phillips TM: Neuropeptides and neurotrophins in neonatal blood of children with autism or mental retardation. Ann Neurol 2001; 49:597–606Crossref, Medline, Google Scholar