Prevention of Late-Life Depression in Primary Care: Do We Know Where to Begin?
Abstract
Objective: This study attempted to compare two models for selective (people at elevated risk) and indicated (those with subsyndromal depressive symptoms) prevention and to determine the optimal strategy for prevention of late-life depression. Method: Onset was assessed at 3 years with the Geriatric Mental State AGECAT in a randomly selected cohort of 1,940 nondepressed and nondemented older people in Amsterdam. Risk factors that can easily be identified in primary care were used. Results: The association of risk factors with depression incidence was expressed in absolute and relative risk estimates, number needed to treat, and population-attributable fractions. Prevention models were identified with classification and regression tree analyses. In the indicated prevention model, subsyndromal symptoms of depression were associated with a risk of almost 40% of developing depression and a number needed to treat of 5.8, accounting for 24.6% of new cases. Adding more risk factors raised the absolute risk to 49.3%, with a lower number needed to treat but also lower attributable fraction values. In the selective prevention model, spousal death showed the highest risk, becoming even higher if the subjects also had a chronic illness. Overall, the attributable fraction values in the indicated model were higher, identifying more people at risk. Conclusions: Consideration of the costs and benefits of both models in the context of the availability of evidence-based preventative interventions indicated that prevention aimed at elderly people with depressive symptoms is preferred. The focus on treatment should be readdressed; a new approach is needed, with a stronger emphasis on prevention.
The adverse consequences of depression are well established. Currently, of all illnesses, depressive disorder causes the largest amount of nonfatal burden, accounting for almost 12% of all years lived with disability worldwide (1) . Depression is also associated with excess morbidity and mortality (2) , higher demands on caregivers and higher service use, and has substantial economical implications (3) . In community-dwelling elderly people, the prevalence of depression requiring clinical attention is 13.5% (4) , and more than 50% have a chronic course (5 , 6) . Although effective treatment is available, case finding and adequate treatment in primary care are generally poor (7 , 8) . Still, even if all patients with depression were optimally treated with evidence-based interventions, only 34% of the disease burden in terms of years lived with disability could be averted (9) . From the public health perspective, it is therefore of great interest to consider the possibility of depression prevention (10 , 11) . However, evidence-based preventive strategies aimed at reducing the incidence of late life depression in community living elderly people are sparse.
In prevention, different strategies can be chosen according to the stages or transitions in the development of a disorder (12) ( Figure 1 ). Universal prevention aims to influence the behavior of the whole population to prevent the onset of disease. Examples of universal prevention are programs informing the population about the risk of alcohol intake or the benefits of physical exercise. Selective prevention is aimed at people who are at risk because they have been exposed to certain risk factors. In the case of depression, risk indicators are, for example, spousal loss and physical illness (13) . The third form, indicated prevention, targets people who already have early or subsyndromal symptoms, in whom an intervention may reduce the likelihood of developing a full-blown case of depression (12 , 14) . An example of indicated prevention is cognitive therapy for the prevention of psychosis in people at ultrahigh risk (15 , 16) or pharmacotherapy for people with mild cognitive impairment to delay the onset of Alzheimer’s disease (17) .
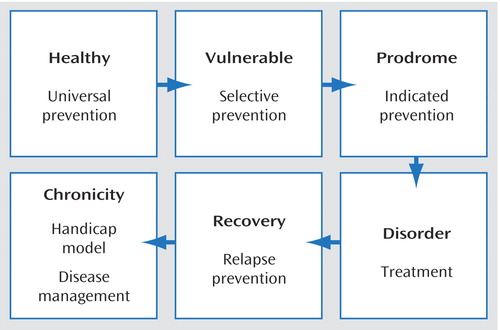
The choice for a specific type of prevention depends on the “untreated” prognosis of the disorder, in combination with the costs, benefits, and possible adverse consequences of different types of intervention. An ethical dilemma is that identifying a healthy person as a possible future case and starting some kind of intervention could carry certain negative consequences and should only be applied if the alternative has a significantly higher probability of adverse consequences. In the case of depression, evidence-based universal prevention of depression is currently nonexistent. Selective prevention may focus on people with certain risk indicators, such as exposure to loss. Indicated prevention would be directed at persons with depressive symptoms below the diagnostic threshold for “clinically relevant depression” (18 , 19) . For both ethical reasons and reasons of cost-effectiveness, preventative measures aimed at reducing the incidence of depression should target subjects with high a priori risk through exposure to multiple risk factors (20) . Furthermore, for practical reasons, persons at risk should be easily identifiable in primary care, and risk profiles have to be simple and unambiguous. From a public health perspective, prevention should be cost-effective and lead to a substantial reduction of total disease burden. This implies that the selected risk indicators should be indicative of a substantial proportion of new cases.
The current study explores selective and indicated prevention models to identify high-risk groups among older geriatric patients, bearing in mind the above-mentioned qualifications. It seeks to identify combinations of exposures that predict the largest health gains in the most cost-effective way when prevention is successful in blocking the adverse effects of these risk factors. These risk factors could then be used in primary care as an easy-to-use checklist or screener to identify patients at elevated risk of becoming a future case of depressive disorder. For indicated prevention, the use of a screening instrument to detect subsyndromal depression would be required. Because this is more time-consuming than recognizing subjects on the basis of more straightforward risk factors needed for selective prevention, the benefits of both approaches are compared. The study is based on data from the Amsterdam Study of the Elderly, a large and prospective cohort study of depression in community-living elderly people incorporating a comprehensive set of risk factors associated with late-life depression (13 , 21) .
Method
Sampling and Nonresponse
The Amsterdam Study of the Elderly is a prospective cohort study on the incidence of depression in a large and representative sample of noninstitutionalized older persons (65+). Informed consent was asked and obtained from each participant before they began the study. The study was approved by the Medical Ethics Committee of the Vrije Universiteit Amsterdam. The population base for Amsterdam Study of the Elderly included all noninstitutionalized individuals in the 65–84-year age bracket who lived in the city of Amsterdam and were registered with a general practitioner at baseline. The study was actively supported by the general practitioners, whose lists were used as the sampling frame. In the Netherlands, general practitioners are the gatekeepers of the health care system, and almost every citizen is on the list of a general practitioner. In this role, general practitioners in the Netherlands generally provide social support and have a long-standing personal relationship with their patients. Thus, the source population consisted of almost all of the noninstitutionalized population. The sample was drawn from a list of 30 general practices spread throughout the city; practices were selected from all of those registered within the city of Amsterdam, 22 randomly and eight by convenience from a network of general practitioners participating in medical research. The profile of the general practice population over age 65 in terms of age and gender, corresponds to the noninstitutionalized population in Amsterdam (22 , 23) . Within each practice, respondents were randomly selected from four age strata spanning 5 years each (65–69 and 80–84). In order to obtain equally sized age strata at follow-up, the older old were oversampled. Out of a total sample of 5,666, 4,051 (71.5%) responded and formed the baseline sample. Interviews with these subjects were conducted between May 1990 and November 1991. The profile of the general practice population over age 65 in terms of age and gender corresponds to the noninstitutionalized Amsterdam population.
At follow-up 3 years later (median=38 months), 2,244 (55.4%) were reinterviewed, 656 (16.2%) people were deceased, 662 (16.3%) refused to cooperate further, 282 (7.0%) were too ill or cognitively impaired to respond, and 207 (5.1%) were not available for interview because of a variety of other reasons. For this study, 523 subjects with depression (12.9%) or 261 with dementia (6.4%) at baseline were excluded. The study cohort consisted of all 1,940 respondents at follow-up who were neither depressed nor demented at baseline for whom complete data were available at follow-up ( Figure 2 ).

Measures
A 1-hour interview was developed to gather information on psychiatric symptoms, demographic and medical status, personal history of depression, and family history. The interview consisted of the Dutch translation of the Mini-Mental State Examination (24) ; all Geriatric Mental State examination items related to organic, affective, and anxiety syndromes (25 , 26) ; the Activities of Daily Living scale (27) ; the Instrumental Activities of Daily Living scale (28) ; and part of the CAMDEX interview (29) . The interview was administered during home visits by lay interviewers who were trained to use video sessions and regularly supervised. The same Geriatric Mental State AGECAT package with an identical algorithm was used in the second wave of the study. When reinterviewing subjects, the raters were unaware of previous data and diagnoses.
Psychiatric Diagnoses
Diagnoses of dementia, depression and generalized anxiety disorder were made according to the Geriatric Mental State AGECAT system. Diagnostic levels 3–5 correspond reliably to cases of depression requiring clinical attention in both the community (30) and in elderly hospital patients (31) . Geriatric Mental State AGECAT has proven reliability in epidemiological studies, as became evident through replication studies (26) . Geriatric Mental State AGECAT generates both a nonhierarchical syndrome level and a more narrowly defined diagnostic level. The diagnostic case level is calculated from the syndrome level with hierarchy from organic to depression to anxiety disorder. To be able to also assess overlapping comorbidity influences, syndrome levels were used in the analyses because otherwise the diagnostic hierarchy would bias the results. Depression “caseness” at baseline and follow-up was defined as a Geriatric Mental State AGECAT level 3 or higher (26) . Subjects not depressed at baseline who had become depressed at follow-up were considered to be incident cases. Subjects with depression case levels 1 or 2 at baseline were classified as having “subsyndromal depression.”
Risk Indicators
Sociodemographic variables included age, gender, and educational level, the latter of which was dichotomized into lower (primary school or less) and higher (more than primary school) education. Living situation was assessed with the associated questions in the Geriatric Mental State AGECAT. A personal history of depression was ascertained by a relevant CAMDEX question.
The question was considered affirmative if treatment had previously been requested. The presence of chronic diseases was assessed with the pertinent CAMDEX questions on myocardial infarction, stroke, cancer, lung disease, diabetes, Parkinson’s disease, arthritis, and epilepsy. Cognitive status was assessed by MMSE score. Cognitive impairment was defined as an MMSE score below 24. Sleeping disorder was determined by two questions from the Geriatric Mental State on “trouble falling asleep” (yes or “I would have problems if I did not use sleeping medication”) and “early wakening” (at least two times per week at least 2 hours earlier than usual). Disability was measured with a combined scale consisting of all activities of daily living and Instrumental Activities of Daily Living items (32 , 33) . Subjects were considered disabled if the total score was two or more points below the maximum. This indicates that subjects were “in need of help” to perform at least two of the tasks mentioned or were “unable to perform’” at least one task.
Data Analysis
The analyses were conducted in several steps. First, standard epidemiological techniques were used to calculate the absolute and relative risk of becoming a case for subjects with or without being exposed to a certain risk indicator.
In a second step, the importance of risk indicators for the onset of disorder at population level was quantified by taking into account the level of exposure to a risk factor in the population, also called the exposure rate, and the population-attributable fraction. The attributable fraction indicates by how many percentage points the incidence rate of depression in the population are lowered when the adverse effect of the risk factor (or combination of risk factors) can be completely blocked. Similarly, a measure of the efficiency of a preventive intervention can be calculated: the number needed to be treated (34) . The number needed to treat can be interpreted as a measure of efficiency, showing how many people must be protected from the adverse influence of a risk factor to avoid one new case of depression. Because the number needed to treat values presented here are based on the assumption that an intervention is able to completely block the adverse influence of a risk factor on the onset of depression, these should be adapted depending on the actual efficacy of each preventative intervention. If an intervention has 50% efficacy in containing the effect of a risk factor, the actual number needed to treat should thus be multiplied by 2. Using Stata (35) statistics such as relative risk, attributable fraction and the number needed to treat were obtained in a multivariate context with control for competing risks (10) .
In the third step, the relative risk, attributable fraction, the number needed to treat and E statistics were used to find the best values for efficiency (i.e., low needed to treat values) and the best values for health gains (i.e., highest attributable fraction values) for combinations of exposures. Targeting preventative interventions on groups that have been exposed to specific combinations of risk factors may yield higher health gains (relative risk, attributable) against less effort (number needed to treat).
To trace the effect of multiple exposures in all their combinations, we used a method known as classification and regression tree analysis (36 , 37) and adapted it for our purposes. Classification and regression tree analysis can graphically be represented as tree-like figures ( Figure 3 ). First, a parent node is selected that optimizes the number needed to treat, the relative risk, and attributable fraction values in preferably the smallest exposure rate groups. This parent node branches off in two directions, creating child nodes in which people are not only exposed to the risk factor in the parent node but also to an additional risk factor in the child node. Therefore, the first generation of child nodes captures the effect of double exposures, the second generation of child nodes represents the effect of triple exposures, and so on. At each node, both branches (for subjects with and subjects without that specific risk indicator) are followed up to determine the number needed to treat, the relative risk, and the attributable fraction values of combined exposures. Thus, the sample is split into smaller subgroups at each step. To determine the optimal child nodes for our purpose, the following hierarchy of decision rules was used:

Child node with the lowest number needed to treat (i.e., the greatest efficiency)
Child node with the highest attributable fraction (i.e., greatest health gain)
When ties occurred, the child node was selected with the smallest exposure (E) rate because targeting smaller groups is logistically and economically less demanding when the number needed to treat and the attributable fraction values are equal.
The following rules were employed for the termination of branches:
Relative risk <1.00 or relative risk not significant (i.e., no risk involved)
Attributable fraction <5% or attributable fraction not significant (i.e., no health gain)
Number needed to treat >50 or number needed to treat not significant (i.e., not efficient)
Results
Sample Characteristics and Response Pattern
The baseline sample characteristics are shown in Table 1 and have been described in more detail elsewhere (13) . In multivariate logistic regression analysis, nonresponse at the follow-up assessment was predicted by higher age, male gender, lower education, living alone, chronic disease(s), disability, organic caseness, and depression (dichotomized as depression case level versus no depression case level). When subjects who died between measurements were excluded from these analyses, only lower education, living alone and baseline organic syndrome predicted nonresponse. At follow-up, 309 subjects (15.9% of the study sample) had become depressed. The following analyses investigate the associations of risk factors with incident depression.
Predictors of Late-Life Depression
Table 2 shows the predictor model and the associations with the onset of depression, with 95% confidence intervals. Statistically significant associations between risk indicators and depression incidence were found for the following risk factors: medical illness, disability, a personal history of depression, recent loss of spouse, sleep disturbance, generalized anxiety disorder, and subsyndromal depression. From these, the absolute risk of developing depression when exposed to a risk factor was calculated. The number needed to treat values for the risk factors in the model ranged from 242 (living alone, 95% CI not statistically significant) to 4.1 (95% CI=2.3–19.6) for subjects with generalized anxiety disorder. For each risk factor, the associated attributable fraction was also calculated, ranging from 24.6 (subsyndromal depression, 95% CI=18.8−30.0) to 1.1 (living alone, 95% CI not statistically significant).
Indicated Prevention Model (classification and regression tree analysis)
Following the aforementioned algorithm, subsyndromal depression clearly was the preferred primary node ( Figure 3 ). Although the number need to treat of 5.8 was higher than that of generalized anxiety disorder (4.1) ( Table 2 ), the associated attributable fraction of depression was far greater (24.6%) and that of generalized anxiety disorder (2.0%) was below the threshold of 5% for the termination of branches. The prevention model was then continued for each branch, starting with subjects who had and those who did not have subsyndromal depressive symptoms. In subjects with subsyndromal depression, the next branching candidate was having a disability. This step reduced the number needed to treat to 3.9, but it also limited the attributable fraction to 9.7%. The model was then further subdivided with living alone and female sex as following risk indicators. Because the attributable fraction was below 5% at the next step (all→subsyndromal symptoms→disability→living alone→female sex→), the branch was terminated here. In subjects with subsyndromal depression who were not disabled, having a chronic illness (absolute risk=30.9%, number needed to treat=6.0) and living alone (absolute risk=32.9%, number needed to treat=5.6, attributable fraction=4.9%) were chosen as subsequent child nodes. In subjects who did not have depressive symptoms, spousal death was the strongest predictor, with an absolute risk of 31.4% of developing depression, a number needed to treat of 6.1, and an attributable fraction of 5.4%. Further branching was not performed because the attributable fraction became too small (<5%).
The way in which the number needed to treat, the attribution fraction, and exposure rate are interrelated is graphically illustrated in Figure 4 . It shows how adding more risk factors to the model results in a higher absolute risk, a lower number needed to treat, but also a lower attributable fraction.

Selective Prevention Model (classification and regression tree analysis)
Figure 5 shows the model without subsyndromal symptoms of depression. Now the focus is on selective prevention and easy case recognition by general practitioners. Following the algorithm described above, the first branching candidate on the basis of number needed to treat and attributable fraction scores is spousal death, with a number needed to treat of 5.3 and an attributable fraction of 8.2%. This was chosen as the primary (or parent) node. The selective-prevention model then yielded medical illness as the next branching candidate in subjects who lost their spouses. This step reduced the number needed to treat to 3.7 but also limited the attributable fraction to 5.8%. Because of this, further branching was not performed. In subjects who did not recently lose their partner, being disabled was the most important risk indicator, with a number needed to treat of 11.5 and an attributable fraction of 9.0%. Further risk indicators were the presence of a chronic medical illness and female gender. The latter step reduced the attributable fraction but only slightly affected the number needed to treat (from 8.6 to 8.3).

Discussion
In this study, high-risk groups for incident depression were identified in a large cohort of elderly patients seen by general practitioners. Because case finding is generally poor, and both the prevalence and persistence of late-life depression are of major concern, the goal was to identify groups in primary care with a high vulnerability for depression according to easily identifiable criteria. Prevention is of interest, bearing in mind that even optimal care can only reduce the burden of depression by 34% (9) . Because the detection of subsyndromal depressive symptoms requires more effort than recognizing other, more easily identifiable risk indicators in primary care, two models were compared that either incorporated or excluded subsyndromal depression as a predictor of the future onset of a full-blown depressive disorder. Thus, the potential health benefits of preventative measures aimed at reducing the incidence of late-life depression could be determined, taking into account the time that should be invested in adequate case finding in primary care. Although this approach, combining clinical, epidemiological, and public health perspectives has been strongly advocated (14) , it is relatively new to the field of common mental disorders.
Our findings suggest that indicated prevention that involves identifying subsyndromal depressive symptoms as the most important risk indicator is the preferred option for identifying groups at high risk of developing depression. Subjects with depressive symptoms had a risk of almost 30% and accounted for 24.6% of the new cases at follow-up. With a number needed to treat of 5.8, which carries the promise of efficient interventions in this group, these subjects are of major interest for preventative interventions. Further subdivision of the sample according to combinations of risk factors yielded groups with an even higher risk of developing depression and lower numbers needed to treat, but these more narrowly focused approaches have a smaller impact on the incidence rate of depression in the population. For example, disabled women with subsyndromal symptoms who live alone had a 47% risk of becoming depressed, a number needed to treat of only 3.2 but a relatively low attributable fraction (5.9%), indicating that the incidence rate would only fall by 5.9% at best.
In a recent meta-analysis on short-term psychotherapeutic interventions aimed at people with subsyndromal depressive symptoms, these were found to reduce the incidence of depression by 30% (38) . If this were applied to a sample such as ours, the number needed to treat for intervention would be 19.3 (5.8/0.3) for those with subsyndromal depression. For a relatively intensive form of treatment, this may be considered to be too great an effort, and it could be argued that one should start with those subjects who have the highest vulnerability. Further subdivision in the classification and regression tree analysis then leads to smaller subgroups that were exposed to more risk factors. In women with disability who live alone, the adjusted number needed to treat would be 10.7 (3.2/0.3). As an alternative to cognitive behavior therapy, less costly forms of indicated prevention should also be taken into consideration. Both bibliotherapy (39) and the Coping With Depression course, a manualized form of self-help with instructions on mood management, were found to be effective therapies for unipolar depression, with effect sizes that are comparable to those of other treatment modalities for depression (40) . Very recently, minimal contact psychotherapy along these lines has been proven effective in reducing the onset of depression in primary care patients with subthreshold depression (41) . Also in low-cost computerized form, such interventions have proved effective (42 , 43) .
If one considers selective prevention of late-life depression, our analyses show that, in line with earlier findings (44) , elderly persons who recently lost their spouses are at great risk of developing depression, and even more if they also have a chronic medical condition (absolute risk=41.8%, number needed to treat=3.7). Overall, the attributable fractions in this model were somewhat smaller, which means that fewer cases can be prevented. Examples of preventative programs directed at persons who lost their spouses include self-help groups of fellow sufferers that convene for emotional exchange and support, specific courses on competences needed to cope with single life, and “widow-to-widow” programs in which women who had lost their husbands earlier visit recently widowed women for emotional and practical support. Although these programs have shown promising results in terms of postbereavement adaptation (45) and social functioning (46) , the evidence of efficacy in reducing depression onset is limited. In a meta-analysis of eight types of such interventions, Cuijpers et al. (47) found an effect size of 0.34 in relation to control subjects, but the number of published studies is still limited, and this difference was not statistically significant. When we consider the fact that these are low-cost community-based interventions, randomized controlled trials are urgently needed.
Indicated prevention thus has the best chance of detecting large groups of subjects at high risk of developing depression, with numbers needed to treat that could make preventative actions cost-effective by using available evidence-based interventions. Still, in comparison with selective prevention, indicated prevention requires the extra effort of screening subjects for subsyndromal depression. A number of screening instruments have been validated for case finding in community living elderly. These include the Center for Epidemologic Studies Depression Scale, a 30-item questionnaire on depressive symptoms (4 , 48 , 49) but more recently also the 15-item version of the Geriatric Depression Scale (50 – 53) has demonstrated its effectiveness for detecting elderly subjects with depressive symptoms in the community. The screening of large numbers of patients in primary care has been shown to be both feasible and effective (54 , 55) . Therefore, primary care facilities appear to be well equipped to find elderly persons with subsyndromal symptoms.
Strengths and Limitations
The Amsterdam Study of the Elderly is a large prospective cohort study with long follow-up intervals, and it incorporated a wide range of risk factors for late-life depression measured independently of depression onset. The findings are representative for urban populations of community dwelling older persons, and are highly relevant for primary care.
Nonetheless, a number of limitations deserve mentioning. First of all, studies with a limited number of measurements tend to overrepresent chronic forms of disorder. Between assessments, subjects may have had both onset and remission of shorter episodes of depression. At the same time, it may be concluded that even with a 3-year interval, the set of risk factors employed in this study can identify a large number of future cases of depression. Second, although the risk factors cover many domains relevant to depression, biological (genetic) risk indicators are less well represented in the data set. Other factors that would ideally have been available are medication use, substance abuse or dependence, and specific medical conditions, such as thyroid disorders that may be associated with depression onset. Third, it should be concluded that this study does not prove that preventative interventions are successful in community-living elderly. Available studies on indicated prevention were mostly performed in younger adults. However, because other forms of treatment of depression are also effective in the elderly, there would be no reason to doubt the efficacy of preventative interventions in elderly subjects. When considering the potential benefits of prevention, there is an urgent need for randomized, controlled trials to address this matter.
Conclusions
The results of this study stress the importance of preventative action for depression as the primary common mental disorder. And to answer the question put forward in the title of this article, “Yes, we do know where to begin.” Indicated prevention of depression is the preferred strategy. Still, because this involves screening patients for subclinical depression, selective prevention of depression may prove to be a good alternative. It will require only the use of a simple checklist of the relevant risk factors, and the expected health gains can also be substantial. Either way, primary care facilities are equipped to perform adequate case finding. Nonetheless, even though minimal contact, low-cost, evidence-based interventions for people with subsyndromal symptoms are available, their cost-effectiveness has to be established in proper randomized trials. Recent data, however, indicate that this may be the case (11 , 41) . Therefore, it is time to reconsider whether the exclusive focus on the treatment of common mental disorders should be adapted and whether an allocation of personnel and resources for prevention may be more effective in reducing both individual suffering and the overall costs of health care.
1. Ustun TB, Ayuso-Mateos JL, Chatterji S, Mathers C, Murray CJL: The global burden of depressive disorders in the year 2000. Br J Psychiatry 2004; 184:386–392Google Scholar
2. Cuijpers P, Schoevers RA: Increased mortality in depressive disorders: a review. Curr Psychiatry Rep 2004; 6:430–437Google Scholar
3. Charney DS, Reynolds CF III, Lewis L, Lebowitz BD, Sunderland T, Alexopoulos GS, Blazer DG, Katz IR, Meyers BS, Arean PA, Borson S, Brown C, Bruce ML, Callahan CM, Charlson ME, Conwell Y, Cuthbert BN, Devanand DP, Gibson MJ, Gottlieb GL, Krishnan KR, Laden SK, Lyketsos CG, Mulsant BH, Niederehe G, Olin JT, Oslin DW, Pearson J, Persky T, Pollock BG, Raetzman S, Reynolds M, Salzman C, Schulz R, Schwenk TL, Scolnick E, Unutzer J, Weissman MM, Young RC: Depression and Bipolar Support Alliance consensus statement on the unmet needs in diagnosis and treatment of mood disorders in late life. Arch Gen Psychiatry 2003; 60:664–672Google Scholar
4. Beekman AT, Copeland JR, Prince MJ: Review of community prevalence of depression in later life. Br J Psychiatry 1999; 174:307–311Google Scholar
5. Cole MG, Bellavance F, Mansour A: Prognosis of depression in elderly community and primary care populations: a systematic review and meta-analysis. Am J Psychiatry 1999; 156:1182–1189Google Scholar
6. Beekman AT, Geerlings SW, Deeg DJ, Smit JH, Schoevers RA, de Beurs E, Braam AW, Penninx BW, Van Tilburg W: The natural history of late-life depression: a 6-year prospective study in the community. Arch Gen Psychiatry 2002; 59:605–611Google Scholar
7. Tiemens BG, Ormel J, Simon GE: Occurrence, recognition, and outcome of psychological disorders in primary care. Am J Psychiatry 1996; 153:636–644Google Scholar
8. Sonnenberg CM, Beekman AT, Deeg DJ, Van Tilburg W: Drug treatment in depressed elderly in the Dutch community. Int J Geriatr Psychiatry 2003; 18:99–104Google Scholar
9. Andrews G, Issakidis C, Sanderson K, Corry J, Lapsley H: Utilising survey data to inform public policy: comparison of the cost-effectiveness of treatment of ten mental disorders. Br J Psychiatry 2004; 184:526–533Google Scholar
10. Smit F, Beekman ATF, Cuijpers P, de Graaf R, Vollebergh W: Selecting key variables for depression prevention: results from a population-based prospective epidemiological study. J Affect Disord 2004; 81:241–249Google Scholar
11. Smit F, Ederveen A, Cuijpers P, Deeg D, Beekman A: Opportunities for cost-effective prevention of late-life depression: an epidemiological approach. Arch Gen Psychiatry 2006; 63:290–296Google Scholar
12. Haggerty RJ, Mrazek PJ: Can we prevent mental illness? Bull NY Acad Med 1994; 71:300–306Google Scholar
13. Schoevers RA, Beekman AT, Deeg DJ, Geerlings MI, Jonker C, Van Tilburg W: Risk factors for depression in later life: results of a prospective community based study (AMSTEL). J Affect Disord 2000; 59:127–137Google Scholar
14. Munoz RF, Mrazek PJ, Haggerty RJ: Institute of Medicine Report on Prevention of Mental Disorders: Summary and Commentary. Am Psychologist 1996; 51:1116–1122Google Scholar
15. McGorry PD, Killackey EJ: Early intervention in psychosis: a new evidence based paradigm. Epidemiol Psychiatr Soc 2002; 11:237–247Google Scholar
16. McGorry PD, Yung AR, Phillips LJ, Yuen HP, Francey S, Cosgrave EM, Germano D, Bravin J, McDonald T, Blair A, Adlard S, Jackson H: Randomized controlled trial of interventions designed to reduce the risk of progression to first-episode psychosis in a clinical sample with subthreshold symptoms. Arch Gen Psychiatry 2002; 59:921–928Google Scholar
17. Jelic V, Winblad B: Treatment of mild cognitive impairment: rationale, present and future strategies. Acta Neurol Scand Suppl 2003; 179:83–93Google Scholar
18. Pincus HA, Davis WW, McQueen LE: “Subthreshold” mental disorders: a review and synthesis of studies on minor depression and other “brand names.” Br J Psychiatry 1999; 174:288–296Google Scholar
19. Cuijpers P, Smit F: Subthreshold depression as a risk indicator for major depressive disorder: a systematic review of prospective studies. Acta Psychiatr Scand 2004; 109:325–331Google Scholar
20. Cuijpers P: Examining the effects of prevention programs on the incidence of new cases of mental disorders: the lack of statistical power. Am J Psychiatry 2003; 160:1385–1391Google Scholar
21. Schoevers RA, Deeg DJ, Van Tilburg W, Beekman AT: Depression and generalized anxiety disorder: co-occurrence and longitudinal patterns in elderly patients. Am J Geriatr Psychiatry 2005; 13:31–39Google Scholar
22. Launer LJ, Dinkgreve MA, Jonker C, Hooijer C, Lindeboom J: Are age and education independent correlates of the Mini-Mental State Examination performance of community-dwelling elderly? J Gerontol 1993; 48:271–277Google Scholar
23. Launer LJ, Wind AW, Deeg DJ: Nonresponse pattern and bias in a community-based cross-sectional study of cognitive functioning among the elderly. Am J Epidemiol 1994:139:803–812Google Scholar
24. Folstein MF, Folstein SE, McHugh PR: “Mini-Mental State”: a practical method for grading the cognitive state of patients for the clinician. J Psychiatr Res 1975; 12:189–198Google Scholar
25. Copeland JR, Dewey ME, Griffiths-Jones HM: A computerized psychiatric diagnostic system and case nomenclature for elderly subjects: GMS and AGECAT. Psychol Med 1986; 16:89–99Google Scholar
26. Copeland JR, Dewey ME, Henderson AS, Kay DW, Neal CD, Harrison MA, McWilliam C, Forshaw D, Shiwach R: The Geriatric Mental State (GMS) used in the community: replication studies of the computerized diagnosis AGECAT. Psychol Med 1988; 18:219–223Google Scholar
27. Katz S, Ford AB, Moskowitz RW, Jackson BA, Jaffe MW: Studies of illness in the aged: the Index of ADL: a standardized measure of biological and psychosocial functioning. J Am Med Assoc 1963; 185:914–919Google Scholar
28. Lawton MP, Brody EM: Assessment of older people: self-maintaining and Instrumental Activities of Daily Living. Gerontologist 1969; 9:179–186Google Scholar
29. Roth M, Tym E, Mountjoy CQ, Huppert FA, Hendrie H, Verma S, Goddard R: CAMDEX: a standardised instrument for the diagnosis of mental disorder in the elderly with special reference to the early detection of dementia. Br J Psychiatry 1986; 149:698–709Google Scholar
30. Copeland JR, Dewey ME, Griffith-Jones HM: Dementia and depression in elderly persons: AGECAT compared with DSM-III and pervasive illness. Int J Geriatr Psychiatry 1990; 5:47–51Google Scholar
31. Ames D, Tuckwell V: Psychiatric disorders among elderly patients in a general hospital. Med J Aust 1994: 160:671–675Google Scholar
32. Kempen GIJM, Suurmeijer TPBM: The development of a hierarchical polychotomous ADL-IADL Scale for noninstitutionalized elders. Gerontologist 1990; 30:497–502Google Scholar
33. Kempen GIJM, Myers AM, Powell LE: Hierarchical structure in ADL and IADL: analytical assumptions and applications for clinician and researchers. J Clin Epidemiol 1995; 48:1299–1305Google Scholar
34. Cook RJ, Sackett DL: The number needed to treat: a clinically useful measure of treatment effect. BMJ 1995: 310:452–454Google Scholar
35. Stata Corporation: Stata statistical software release 7.0. College Station, Tex, Stata Corporation, 2001Google Scholar
36. Everitt BS: Modern Medical Statistics: A Practical Guide. London, Arnold, 2003Google Scholar
37. Lemon SC, Roy J, Clark MA, Friedmann PD, Rakowski W: Classification and regression tree analysis in public health: methodological review and comparison with logistic regression. Ann Behav Med 2003; 26:172–181Google Scholar
38. Cuijpers P, van Straten A, Smit F: Preventing the incidence of new cases of mental disorders: a meta-analytic review. J Nerv Ment Dis 2005; 193:119–125Google Scholar
39. Cuijpers P: Bibliotherapy in unipolar depression: a meta-analysis. J Behav Ther Exper Psychiatry 1997; 28:139–147Google Scholar
40. Cuijpers PA: Psychoeducational approach to the treatment of depression: a meta-analysis of Lewinsohn’s “Coping With Depression” course. Behav Ther 1998; 29:521–533Google Scholar
41. Willemse GR, Smit F, Cuijpers P, Tiemens BG: Minimal-contact psychotherapy for subthreshold depression in primary care: randomised trial. Br J Psychiatry 2004; 185:416–421Google Scholar
42. McCrone P, Knapp M, Proudfoot J, Ryden C, Cavanagh K, Shapiro DA, Ilson S, Gray JA, Goldberg D, Mann A, Marks I, Everitt B, Tylee A: Cost-effectiveness of computerised cognitive-behavioural therapy for anxiety and depression in primary care: randomised controlled trial. Br J Psychiatry 2004; 185:55–62Google Scholar
43. Christensen H, Griffiths KM, Jorm AF: Delivering interventions for depression by using the Internet: randomised controlled trial. Br Med J 2004: 328:265–268AGoogle Scholar
44. Cole MG, Dendukuri N: Risk factors for depression among elderly community subjects: a systematic review and meta-analysis. Am J Psychiatry 2003; 160:1147–1156Google Scholar
45. Vachon ML, Lyall WA, Rogers J, Freedman-Letofsky K, Freeman SJ: A controlled study of self-help intervention for widows. Am J Psychiatry 1980; 137:1380–1384Google Scholar
46. Van Lammeren P, Geelen K: Becoming bitter of becoming better: evaluation of a program for the prevention of loneliness and depression in elderly widows (in Dutch). Netherlands Centre for Mental Health and Addiction, 1995Google Scholar
47. Cuijpers P, Van Gageldonk A, Overtoom K: The effect of preventative interventions on (determinants of) mental health. Volksgezondheidstoekomstverkenningen 2000Google Scholar
48. Radloff LS: The CES-D Scale: a self-report depression scale for research in the general population. Appl Psychiatr Measures 1977; 1:385–401Google Scholar
49. Prince MJ, Reischies F, Beekman AT, Fuhrer R, Jonker C, Kivela SL, Lawlor BA, Lobo A, Magnusson H, Fichter M, van Oyen H, Roelands M, Skoog I, Turrina C, Copeland JR: Development of the EURO-D Scale—a European Union initiative to compare symptoms of depression in 14 European centres. Br J Psychiatry 1999; 174:330–338Google Scholar
50. de Craen AJ, Heeren TJ, Gussekloo J: Accuracy of the 15-item Geriatric Depression Scale (GDS-15) in a community sample of the oldest old. Int J Geriatr Psychiatry 2003; 18:63–66Google Scholar
51. Almeida OP, Almeida SA: Short versions of the Geriatric Depression Scale: a study of their validity for the diagnosis of a major depressive episode according to ICD-10 and DSM-IV. Int J Geriatr Psychiatry 1999; 14:858–865Google Scholar
52. Osborn DP, Fletcher AE, Smeeth L, Stirling S, Nunes M, Breeze E, Siu-Woon, Ng E, Bulpitt CJ, Jones D, Tulloch A, Siu-Woon E: Geriatric Depression Scale scores in a representative sample of 14,545 people aged 75 and over in the United Kingdom: results from the MRC trial of assessment and management of older people in the community. Int J Geriatr Psychiatry 2002; 17:375–382Google Scholar
53. Sheikh JL, Yesavage JA: Geriatric Depression Scale (GDS): recent evidence and development of a shorter version. Clin Gerontol 1986; 37:819–820Google Scholar
54. Bijl D, van Marwijk HWJ, Beekman ATF, de Haan M, Van Tilburg W: A randomized controlled trial to improve the recognition, diagnosis and treatment of major depression in elderly people in general practice: design, first results and feasibility of the West Friesland Study. Primary Care Psychiatry 2003; 8:135–140Google Scholar
55. Bijl D, van Marwijk HW, de Haan M, Van Tilburg W, Beekman AJ: effectiveness of disease management programmes for recognition, diagnosis and treatment of depression in primary care. Eur J Gen Pract 2004; 10:6–12Google Scholar