Genetic Heterogeneity in Depressive Symptoms Following the Death of a Spouse: Polygenic Score Analysis of the U.S. Health and Retirement Study
Abstract
Objective:
Experience of stressful life events is associated with risk of depression. Yet many exposed individuals do not become depressed. A controversial hypothesis is that genetic factors influence vulnerability to depression following stress. This hypothesis is often tested with a “diathesis-stress” model, in which genes confer excess vulnerability. The authors tested an alternative formulation of this model: genes may buffer against depressogenic effects of life stress.
Method:
The hypothesized genetic buffer was measured using a polygenic score derived from a published genome-wide association study of subjective well-being. The authors tested whether married older adults who had higher polygenic scores were less vulnerable to depressive symptoms following the death of their spouse compared with age-matched peers who had also lost their spouse and who had lower polygenic scores. Data were analyzed from 8,588 non-Hispanic white adults in the Health and Retirement Study (HRS), a population-representative longitudinal study of older adults in the United States.
Results:
HRS adults with higher well-being polygenic scores experienced fewer depressive symptoms during follow-up. Those who survived the death of their spouses (N=1,647) experienced a sharp increase in depressive symptoms following the death and returned toward baseline over the following 2 years. Having a higher well-being polygenic score buffered against increased depressive symptoms following a spouse’s death.
Conclusions:
The effects were small, and the clinical relevance is uncertain, although polygenic score analyses may provide clues to behavioral pathways that can serve as therapeutic targets. Future studies of gene-environment interplay in depression may benefit from focus on genetics discovered for putative protective factors.
Depression is a leading cause of disability burden globally (1). Depression is a particular concern among older adults, who are a growing population segment, and in whom depression may contribute to mobility limitation leading to disability and premature mortality (2). Depression in older adults shares etiological features with depression in younger adults, reflecting a combination of genetic and environmental influences (3). A critical question is why events that can trigger depressive episodes may affect some individuals differently than others.
Experience of stressful life events is associated with risk of depression (4, 5). Yet not all stress-exposed individuals become depressed. A controversial hypothesis is that genetic differences between individuals modify the influence of life-stress on depression (6). This “diathesis-stress” hypothesis is supported by family-based genetic studies that find individuals with familial liability to depression are more vulnerable to developing depression following stress exposure (7). Molecular genetic evidence for the diathesis-stress hypothesis from candidate-gene studies is contested, partly because of concerns about the candidate-gene approach (8). Genome-wide association studies (GWAS) offer opportunities to move beyond arguments about candidate genes. GWAS discoveries in depression have yielded mixed results in tests of diathesis-stress models (9–11). A recent GWAS of subjective well-being (12) offers the opportunity to evaluate the opposite end of the continuum of genetic liability.
Subjective well-being represents a dimension of so-called “positive psychology” and may reflect the opposite end of an affective continuum from depression (13). Like depression, subjective well-being is heritable, with a portion of this heritability reflecting genetic influences shared with depression (14). Theory predicts increased subjective well-being should buffer against deleterious consequences of stressful life events (15).
We conducted a polygenic score study to test whether genetic predisposition to greater subjective well-being buffered against risk of depression following experience of a stressful life event: the death of one’s spouse. We studied depressive symptoms in a cohort of older adults and their spouses followed longitudinally as part of the U.S. Health and Retirement Study (HRS). We combined whole-genome single-nucleotide polymorphism (SNP) data already generated for the cohort and results from GWAS of subjective well-being in over 100,000 adults by the Social Science Genetic Association Consortium (12) to calculate cohort members’ subjective well-being polygenic scores. Analysis tested whether higher subjective well-being polygenic scores buffered survivors’ risk of developing depressive symptoms following the death of their spouse. For comparative purposes, we repeated the analysis using polygenic scores based on GWAS of major depressive disorder by the Psychiatric Genomics Consortium (16) and GWAS of depressive symptoms by the Social Science Genetic Association Consortium (12).
Method
Sample
The HRS is a longitudinal survey, initiated in 1992, of a representative sample of Americans aged ≥50 and their spouses (17). The HRS is administered biennially and includes over 26,000 persons in 17,000 households. Respondents are interviewed about economic, social, and health issues (http://hrsonline.isr.umich.edu/index.php).
Genetic Data
We linked HRS survey data compiled by Rand Corporation (http://hrsonline.isr.umich.edu/modules/meta/rand/randhrsn/randhrsN.pdf) with genome-wide SNP data downloaded from the U.S. National Institutes of Health Database of Genotypes and Phenotypes [dbGaP] (see the data supplement accompanying the online version of this article). Quality control procedures excluded SNPs missing in >5% of samples, with minor allele frequency <1%, or not in Hardy-Weinberg equilibrium (p<0.001). The final genetic database included approximately 1.7 million SNPs.
Population stratification (the nonrandom patterning of allele frequencies across populations with different ancestries) may limit the generalizability of polygenic scores derived in one population to others (18). To increase comparability of the analysis sample to the population studied in the original GWAS, we focused analysis on HRS respondents identified as non-Hispanic white based on analysis of genome-wide SNP data by the HRS investigators (http://hrsonline.isr.umich.edu/sitedocs/genetics/HRS_QC_REPORT_MAR2012.pdf). The resulting analysis sample included 8,588 individuals (58% female) born between 1905 and 1974, with the average age of 59 years (interquartile range: 53–66) at first interview and participating in a median of eight follow-up assessments (interquartile range: 6–10). The analysis data set comprised a total of 67,805 observations. We also conducted our analysis using the sample of HRS respondents who self-identified as non-Hispanic white. These results are reported in the online data supplement.
Well-Being Polygenic Score
We calculated HRS respondents’ well-being polygenic scores from results from the Social Science Genetic Association Consortium GWAS of subjective well-being (12). That GWAS analyzed measures of life satisfaction, positive affect, and a combination of these scales defined as subjective well-being. The original GWAS included HRS respondents. To calculate polygenic scores for HRS respondents for this analysis, the Social Science Genetic Association Consortium provided revised GWAS weights estimated from data excluding the HRS (restricted GWAS: N=195,024). Therefore, there was no overlap between the samples used to develop the polygenic score and the sample we analyzed. Polygenic scoring was conducted according to the methods described by Dudbridge (19) following the protocol used in previous work (20). Briefly, we matched SNPs in the HRS genotype database with well-being GWAS SNPs. For each SNP, counts of well-being-associated alleles were weighted by GWAS-estimated effect sizes. Weighted counts were summed across SNPs to compute polygenic scores. Polygenic scores were composed from all SNPs matched between the original GWAS and the HRS genotyped SNP database (SNPs: N=773,672). We calculated polygenic scores based on all SNPs in the score for two reasons. First, this is the same method used by the Social Science Genetic Association Consortium in their own polygenic score analysis (12). Second, theory, simulation evidence, and empirical evidence all agree that for genetically complex traits, including all SNPs is the most reliable method to produce a polygenic score with maximum predictive power (19, 21, 22).
To account for residual population stratification in our non-Hispanic white sample, we computed residualized polygenic scores (20). We estimated principal components from the genome-wide SNP data for non-Hispanic white HRS respondents according to the method described by Price et al. (23) using the PLINK command for pca [principal component analysis]. We then residualized the well-being polygenic score for the first 10 principal components (i.e., we regressed HRS respondents’ polygenic scores on the 10 principal component scores and computed residual values from the predictions). Residualized polygenic scores were standardized to a mean of 0 [SD=1] for the analysis.
Depression Polygenic Scores
We used the same methods to calculate two polygenic scores for depression from GWAS of clinical depression conducted by the Psychiatric Genomics Consortium and GWAS of depressive symptoms conducted by the Social Science Genetic Association Consortium (12, 16). The Social Science Genetic Association Consortium GWAS included the Psychiatric Genomics Consortium samples in addition to samples from the United Kingdom Biobank and the Kaiser Permanente Genetic Epidemiology Research on Adult Health and Aging databases.
Death of Spouse
Death of one’s spouse is a well-documented risk factor for developing depressive symptoms (24). During follow-up, 1,647 individuals (22% of those married) experienced the death of their spouse. These survivors (76% female; mean age=73 years [SD=9.72]) completed follow-up surveys 12 months, on average, after their spouse’s death (interquartile range: 6–18 months [also see Figure S1 in the online data supplement]).
Depressive Symptoms
Depressive symptoms were measured with the 8-item Center for Epidemiological Studies Depression Scale at each HRS follow-up. The Center for Epidemiological Studies Depression Scale is a valid psychometric instrument for assessment of depression in older adults (25). We analyzed the continuous score on this scale. There was substantial within-person variability in depressive symptoms across follow-up (intraclass correlation=0.47, mean=1.16 [SD=1.74]).
Analysis.
We conducted descriptive analysis of HRS respondents’ depressive symptoms before and after the death of their spouses using nonparametric local regression. Local regression fits smoothed curves to data with nonlinear patterns by analyzing “localized” subsets of the data to build up a function iteratively (26). We used local regression to fit symptom data to time from death. The resulting model describes symptom levels leading up to and following spousal death. If the death of one’s spouse produces a change in depressive symptoms, this change will appear as a discontinuity in the local regression line estimated by the model (e.g., depressive symptom levels may “jump” from their trajectory before the death to a higher level after the death).
We conducted hypothesis tests using parametric nonlinear regression (27). Nonlinear regression models were estimated independent of the descriptive local regression analysis. Nonlinear regression estimates a function describing nonlinear patterns in a set of data and then models those nonlinearities as a function of other variables. Our analysis modeled depressive symptoms at the first assessment following death of a spouse as a nonlinear function of time since the death. We tested the hypothesis that well-being polygenic score would buffer against increase in depressive symptoms following death of a spouse using the coefficient for the association between polygenic score and that nonlinear function.
Specifically, we estimated the following model:
The nonlinear regression model focused on the first observations made in HRS respondents following the deaths of their spouses. Because follow-up assessments in the HRS were conducted only every 2 years, repeated observations of individuals are unlikely to observe changes in depressive symptoms related to spousal death. Nevertheless, as a sensitivity analysis, we re-estimated the nonlinear regression model using all observations following spousal death (i.e., multiple observations per HRS respondent). Results are reported in Figure S3 in the data supplement.
Statistical analysis was conducted using the R software. We tested main-effect associations between HRS respondents’ polygenic scores and their depressive symptoms using random-intercept models implemented with the R package lme4. We fit local regression models to repeated measures depressive symptom data using the loess [LOESS] function and the nonlinear regression models using the nls [nonlinear least squares] function.
Results
Higher Well-Being Polygenic Score Was Associated With Reduced Depressive Symptoms
HRS respondents with higher polygenic scores experienced fewer depressive symptoms during follow-up (b=−0.10, confidence interval [CI]=−0.13 to –0.07, p<0.001) (Figure 1; also see Table S1/Model 1 in the online data supplement). This effect size is consistent with previous analysis of the well-being polygenic score and depression (12). The genetic association with depressive symptoms was similar for men and women (p=0.803 for test of interaction between polygenic score and sex).
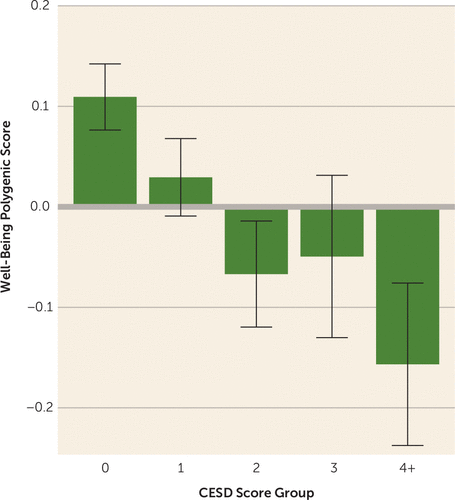
FIGURE 1. Health and Retirement Study (HRS) Respondents With Higher Polygenic Scores Reporting Fewer Depressive Symptoms During Follow-Upa
a The graph shows average subjective well-being polygenic scores (denominated in standard deviation units) for groups of HRS respondents defined by their histories of depressive symptoms. Depressive symptom history was measured by averaging respondents’ Center for Epidemiological Studies Depression (CESD) scores across repeated assessments. For graphing purposes, averaged scores were rounded to the nearest integer value. The resulting groups included N=3,489 respondents with an average score of 0, N=2,565 with a score of 1, N=1,327 with a score of 2, N=605 with a score of 3, and N=602 with a score of 4 or higher.
Well-Being Polygenic Score Was Not Associated With Likelihood of Experiencing Death of a Spouse
Genetic liability to depression may influence exposure to stressful life events in older adults. Therefore, before testing the genetic buffering hypothesis, we evaluated possible gene-environment correlation between subjective well-being polygenic score and likelihood of experiencing spousal death. We found no evidence of such gene-environment correlation; HRS respondents’ polygenic scores were not related to their likelihood of experiencing the death of their spouse (odds ratio=0.97, CI=0.92–1.02, p=0.219).
Death of a Spouse Was Associated With an Immediate Increase in Depressive Symptoms, Followed by a Gradual Return Toward Baseline Over the Following Years
HRS respondents who experienced death of their spouse experienced a discontinuity in depressive symptoms around the time of the death. In local regression analysis, respondents’ depressive symptoms trended slightly upwards in the months immediately preceding the death, rose sharply at the time of death, and gradually returned toward baseline over the following two years (Figure 2). This pattern is consistent with previous reports on the course of depressive symptoms around spousal death (28).
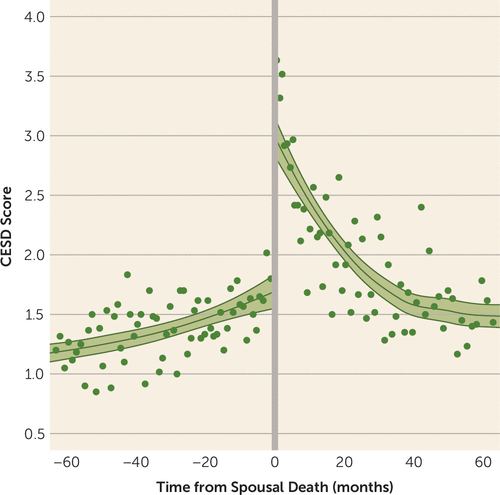
FIGURE 2. Trajectories of Depressive Symptoms in the Months Surrounding the Death of a Spousea
a The scatterplot and local regression plot show depressive symptoms (Center for Epidemiological Studies Depression [CESD] scores) by month of measurement relative to the death of a spouse. The plot reflects data from Health and Retirement Study respondents who experienced spousal death (N=1,647; 14,309 total observations). Plotted points show mean x- and y- coordinates for bins of about 60 observations.
Nonlinear regression analysis estimated the increase in Center for Epidemiological Studies Depression score associated with spousal death to be 1.97 points (95% CI 1.5–2.4, p≤0.001). This increase was smaller for respondents interviewed farther from the time of their spouse’s death. Based on trajectories estimated from the model, the increase in depressive symptoms that occurred following the death of a spouse attenuated by 12 months after the death, with modest further attenuation through 24 months (see Table S1/Model 2 in the online data supplement).
Genetics of Subjective Well-Being Buffered Against Increase in Depressive Symptoms Following Death of a Spouse
HRS respondents with higher polygenic scores experienced flatter trajectories of depressive symptoms around the time of their spouse’s death compared with peers who also lost their spouse and had lower polygenic scores. In local regression analysis, HRS respondents with higher well-being polygenic scores experienced fewer depressive symptoms in the months leading up to the death and a smaller increase in depressive symptoms following the death compared with other survivors who had lower polygenic scores (Figure 3).
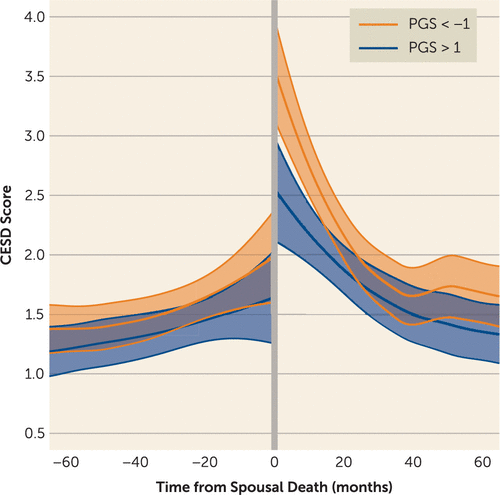
FIGURE 3. Trajectories of Depressive Symptoms for Health and Retirement Study (HRS) Respondents With Low Subjective Well-Being Polygenic Scores and High Subjective Well-Being Polygenic Scoresa
a The graph shows local regression plots of depressive symptoms (Center for Epidemiological Studies Depression [CESD] scores) by month of measurement relative to the death of a spouse. Trajectories for respondents with low subjective well-being polygenic scores are graphed in red (1 or more standard deviations below the mean, N=255 individuals with 2,164 observations). Trajectories for HRS respondents with high subjective well-being polygenic scores are graphed in blue (1 or more standard deviations above the mean, N=242 individuals with 2,048 observations). PGS=polygenic score.
In nonlinear regression analysis, each standard deviation increase in polygenic score buffered the increase in depressive symptoms following spousal death by 0.60 Center for Epidemiological Studies Depression points (95% CI=0.19–1.02, p=0.004). In context, this effect estimate suggests that a person with a polygenic score one standard deviation above the population mean should experience about half as much increase in depressive symptoms following death of a spouse compared with a peer whose polygenic score was one standard deviation below the population mean. Respondents’ polygenic scores were not related to the rate of attenuation in depressive symptoms following the death (γ=0.33, p=0.739 [also see Table S1 in the online data supplement]).
Sensitivity Analyses
We conducted sensitivity analyses to evaluate consistency of findings. First, we repeated the analysis including all HRS respondents who self-reported as being of non-Hispanic white race/ethnicity (N=9,453 [N=1,829 who survived the death of a spouse]). Findings were similar to those in the analysis sample determined by genetically defined ancestry (see Table S2 in the data supplement).
Second, we repeated the analysis using polygenic scores derived from two published GWAS of depression, the Psychiatric Genomics Consortium’s GWAS of major depressive disorder (16) and the Social Science Genetic Association Consortium GWAS of depressive symptoms (12). We analyzed genetic overlap among phenotypes using linkage disequilibrium score regression (29) and polygenic score methods. Linkage disequilibrium score regression estimates genetic correlations (rG) from GWAS summary statistics. We implemented linkage disequilibrium score regression using the LDSC Python software package (https://github.com/bulik/ldsc) following the method described by the Social Science Genetic Association Consortium (12). Previous linkage disequilibrium score regression analysis estimated rG between subjective well-being and depressive symptoms to be r=−0.80 (12). Genetic correlation computed from subjective well-being GWAS results excluding the HRS sample was similar (for depressive symptoms: rG=−0.81, SE=0.06; for major depressive disorder: rG=−0.78, SE=0.12). Empirical correlations between polygenic scores were lower (|r|=0.15–0.32). All genetic correlations and polygenic score correlations were statistically significant at a p value <0.001.
Results from analysis of depression polygenic scores paralleled results from analysis of the subjective well-being polygenic score. HRS respondents with higher depression polygenic scores had higher levels of depressive symptoms across HRS follow-up. Among those who survived the death of a spouse, higher depression polygenic scores predicted a greater increase in depressive symptoms following the death (Figure 4). In nonlinear regression analysis, each standard deviation increase in the depressive symptoms polygenic score amplified the increase in depressive symptoms following spousal death by 0.69 Center for Epidemiological Studies Depression points (95% CI=0.24–1.14, p=0.003). The result was similar for the major depressive disorder polygenic score (b=0.63, 95% CI=0.21–1.05, p=0.003). Results from analysis of depression polygenic scores are reported in Table S3 in the online data supplement.
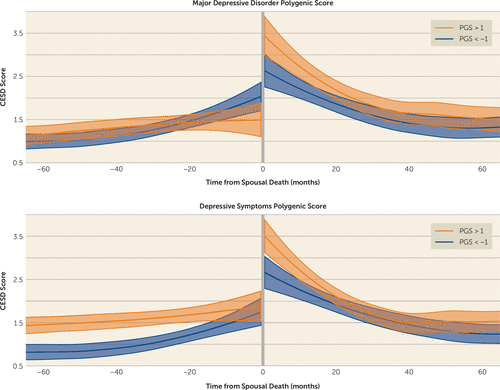
FIGURE 4. Trajectories of Depressive Symptoms for Health and Retirement Study (HRS) Respondents With High Depression Polygenic Scores and Low Depression Polygenic Scoresa
a The charts show local regression plots of depressive symptoms (Center for Epidemiological Studies Depression [CESD] scores) by month of measurement relative to the death of a spouse. Trajectories for respondents with high depression polygenic scores are graphed in red (one or more standard deviations above the mean, N=224 individuals with 1,814 observations for the major depressive disorder polygenic score, N=276 individuals with 2,350 observations for the depressive symptoms polygenic score). Trajectories for HRS respondents with low depression polygenic scores are graphed in blue (one or more standard deviations below the mean, N=260 individuals with 2,165 observations for major depressive disorder polygenic score, N=265 individuals with 2,217 observations for the depressive symptoms polygenic score). PGS=polygenic score.
Discussion
We tested whether genetics discovered in GWAS of subjective well-being buffered against development of depressive symptoms following death of a spouse. We analyzed data on depressive symptoms in 8,588 individuals followed longitudinally as part of the HRS. During follow-up, 1,647 of these individuals experienced the death of a spouse. HRS respondents with higher polygenic scores experienced fewer depressive symptoms during follow-up. For those whose spouse died during the follow-up period, having a higher polygenic score buffered against increased depressive symptoms following the death. Having a low polygenic score for depression had similar buffering effects on depression risk as having a high polygenic score for subjective well-being. Magnitudes of genetic effects were small.
This evidence of genetic buffering against depression following a stressful life event provides proof of concept for further investigation of the genetics of subjective well-being as a modifier of the life-stress to depression link. Studies of gene-environment interplay are controversial (30). Statistical and theoretical models are contested. The precise nature of any “interaction” between the stress of losing a spouse and well-being-related genetic background remains uncertain. What we find is that individuals who carry more well-being-associated alleles tend to develop fewer additional depressive symptoms following the death of a spouse. This “buffering” is independent of the “main effect” of genetics in the absence of a spousal death. Buffering is also not affected by any “gene-environment correlation” between the genetics of subjective well-being and exposure to death of a spouse; HRS respondents’ subjective well-being polygenic scores were unrelated to their risk of losing a spouse during follow-up. Finally, we detected consistent evidence of buffering using two different statistical specifications, local regression and nonlinear regression, which take into account time elapsed between the stressful event and the measurement of depressive symptoms.
Our findings have implications for relevance of well-being genetics in clinical approaches to bereavement and for research into gene-environment interplay. Clinically, genetic testing based on current knowledge is unlikely to be useful in planning for or managing bereavement. Effect sizes in our study were too small to be useful for predicting outcomes of individual patients. It is possible that larger-scale GWAS will furnish more predictive polygenic scores with greater clinical relevance. However, even with very large GWAS, the predictive power of polygenic scores for common, complex traits will be limited (31). Instead, our results may inform clinical research into candidate intervention targets to promote resilience in bereavement and in the context of other stressful experiences. Specifically, our findings raise questions about what behaviors or psychological processes mediate buffering effects. Do individuals with higher well-being polygenic scores process or cope with stress differently (32)? Do they cultivate different social support networks or interact with them in different ways? Studies to identify such mediators of genetic associations can inform interventions designed to promote resilience. Finally, given that we observed essentially the same result in our analysis of polygenic scores for subjective well-being and depression, research is needed to evaluate whether behavioral and psychological mediators of genetic influences measured by these scores are the same or different.
High genetic correlation between subjective well-being and depression (rG: approximately 0.8) suggests that polygenic scores derived from GWAS of these different phenotypes may measure the same genetic influence. However, the much lower empirical correlation between polygenic scores (r: approximately 0.2–0.3 in our study) means that conducting the same analysis using different scores could yield different results. Parallel findings across different polygenic scores suggest that it is the overlap among these scores that quantifies the substantive genetic influence. Methods are needed to integrate information from GWAS of different phenotypes with high genetic correlation (33). In theory, polygenic scores that measure overlap between GWAS results for genetically correlated phenotypes may provide a more precise estimate of genetic influence.
Regarding research on gene-environment interplay, these results highlight two study design issues. First, temporal resolution of environmental exposure measures may be critical. Depression is an episodic condition, including in older adults (3). Even persistent cases experience temporary remissions. Increases in depressive symptoms following stressful life events may attenuate over time, as is characteristic in bereavement (34). Our study could account for this attenuation because HRS recorded the date when a respondent died, allowing precise quantification of time since exposure for measures of depressive symptoms. We used statistical models designed for this type of data. This difference may account for why our study could detect gene-environment interplay effects in contrast to some previous analyses (10, 11). Future studies to detect gene-environment interplay may benefit from focus on stressful life events that can be located in specific temporal relation to the measurement of depressive symptoms (35).
A second study design issue is the question of whether polygenic scores make good tools for investigating gene-environment interplay in the first place. Polygenic scores have been proposed as a method to investigate gene-environment interplay because, by aggregating information from across the genome, they quantify larger genetic effects compared with individual variants and take on normal distributions (36). These properties match theoretical and empirical models of genetic influence on quantitative traits (37). However, it is argued that polygenic scores derived from GWAS naive to environmental variation may quantify primarily genetic influences that do not vary across environments, although alternative GWAS designs are possible (38). The findings reported above suggest that polygenic scores derived from GWAS naive to environmental variation can predict heterogeneity in response to environmental exposures.
Our study has some limitations. We studied depression in married older adults. The etiology of depression in older adults may differ in some ways from the etiology in younger adults, although the relationship of stressful life events to depression does not seem to vary with age (39). Etiological features of depression, including subjective well-being, may influence the probability of marriage and of remaining married into later life. Replication of results in younger samples and in unmarried adults is needed. We studied depression following death of a spouse. Depression following death of a spouse may be different from depression related to other stressful life events. However, the distinction is unclear, as is reflected in DSM-5 (40). There is evidence that so-called bereavement-related depression shares etiology with depression related to other stressful life events (41). Tests of a well-being polygenic score as a buffer against depressive symptoms following other types of stressful life events are needed. Our analysis was restricted to European-descent HRS participants because of concerns about generalizability of GWAS results across racial/ethnic populations (18). Studies of genetic buffering against depression following stressful life events in non-European populations are needed.
Research into interplay between genes and environments has a contentious history in psychiatry. With the advent of large-scale GWAS, new, more reliable genetic measures of liability to psychiatric disorders and other mental health characteristics are emerging. At the same time, genetic data are becoming available for large, population-based social surveys that have recorded environmental exposure information about participants. Together, these resources make possible a new generation of molecular genetic research into gene-environment interplay in the etiology of psychiatric disorders.
1 : Disability-adjusted life years (DALYs) for 291 diseases and injuries in 21 regions, 1990–2010: a systematic analysis for the Global Burden of Disease Study 2010. Lancet 2012; 380:2197–2223Crossref, Medline, Google Scholar
2 : Depression in the elderly. Lancet 2005; 365:1961–1970Crossref, Medline, Google Scholar
3 : Depression in late life: review and commentary. J Gerontol A Biol Sci Med Sci 2003; 58:M249–M265Crossref, Medline, Google Scholar
4 : The effects of stressful life events on depression. Annu Rev Psychol 1997; 48:191–214Crossref, Medline, Google Scholar
5 : Negative life events and depression in elderly persons: a meta-analysis. J Gerontol B Psychol Sci Soc Sci 2002; 57:87–P94Crossref, Medline, Google Scholar
6 : Diathesis-stress theories in the context of life stress research: implications for the depressive disorders. Psychol Bull 1991; 110:406–425Crossref, Medline, Google Scholar
7 : Genetic moderation of environmental risk for depression and anxiety in adolescent girls. Br J Psychiatry 2001; 179:116–121Crossref, Medline, Google Scholar
8 : Genetic sensitivity to the environment: the case of the serotonin transporter gene and its implications for studying complex diseases and traits. Am J Psychiatry 2010; 167:509–527Link, Google Scholar
9 : Effect of polygenic risk scores on depression in childhood trauma. Br J Psychiatry 2014; 205:113–119Crossref, Medline, Google Scholar
10 : Polygenic interactions with environmental adversity in the aetiology of major depressive disorder. Psychol Med 2016; 46:759–770Crossref, Medline, Google Scholar
11 : Polygenic risk, stressful life events and depressive symptoms in older adults: a polygenic score analysis. Psychol Med 2015; 45:1709–1720Crossref, Medline, Google Scholar
12 : Genetic variants associated with subjective well-being, depressive symptoms, and neuroticism identified through genome-wide analyses. Nat Genet 2016; 48:624–633Crossref, Medline, Google Scholar
13 : Subjective well-being. The science of happiness and a proposal for a national index. Am Psychol 2000; 55:34–43Crossref, Medline, Google Scholar
14 : Happiness is a personal (ity) thing the genetics of personality and well-being in a representative sample. Psychol Sci 2008; 19:205–210Crossref, Medline, Google Scholar
15 : Well-being and the risk of depression under stress. PLoS One 2013; 8:e67395Crossref, Medline, Google Scholar
16 Major Depressive Disorder Working Group of the Psychiatric GWAS Consortium, , et al: A mega-analysis of genome-wide association studies for major depressive disorder. Mol Psychiatry 2013; 18:497–511Crossref, Medline, Google Scholar
17 : Cohort profile: the Health and Retirement Study (HRS). Int J Epidemiol 2014; 43:576–585Crossref, Medline, Google Scholar
18 : Generalization and dilution of association results from European GWAS in populations of non-European ancestry: the PAGE study. PLoS Biol 2013; 11:e1001661Crossref, Medline, Google Scholar
19 : Power and predictive accuracy of polygenic risk scores. PLoS Genet 2013; 9:e1003348Crossref, Medline, Google Scholar
20 : Assortative mating and differential fertility by phenotype and genotype across the 20th century. Proc Natl Acad Sci USA 2016Crossref, Google Scholar
21 : Prediction of individual genetic risk to disease from genome-wide association studies. Genome Res 2007; 17:1520–1528Crossref, Medline, Google Scholar
22 Schizophrenia Working Group of the Psychiatric Genomics Consortium: Biological insights from 108 schizophrenia-associated genetic loci [Internet]. Nature 2014; 511:421–427. http://www.nature.com/nature/journal/vaop/ncurrent/full/nature13595.htmlGoogle Scholar
23 : Principal components analysis corrects for stratification in genome-wide association studies. Nat Genet 2006; 38:904–909Crossref, Medline, Google Scholar
24 : Depression through the first year after the death of a spouse. Am J Psychiatry 1991; 148:1346–1352Link, Google Scholar
25 : Screening for depression in the older adult: criterion validity of the 10-item Center for Epidemiological Studies Depression Scale (CES-D). Arch Intern Med 1999; 159:1701–1704Crossref, Medline, Google Scholar
26 : Robust locally weighted regression and smoothing scatterplots. J Am Stat Assoc 1979; 74:829–836Crossref, Google Scholar
27 : Nonlinear mixed effects models for repeated measures data. Biometrics 1990; 46:673–687Crossref, Medline, Google Scholar
28 : Conjugal loss and syndromal depression in a sample of elders aged 70 years or older. Am J Psychiatry 1999; 156:1596–1601Link, Google Scholar
29 : An atlas of genetic correlations across human diseases and traits. Nat Genet 2015; 47:1236–1241Crossref, Medline, Google Scholar
30 : Gene-environment interactions in severe mental illness. Front Psychiatry 2014; 5:48Crossref, Medline, Google Scholar
31 : Pitfalls of predicting complex traits from SNPs. Nat Rev Genet 2013; 14:507–515Crossref, Medline, Google Scholar
32 : Understanding familial risk for depression a 25-year perspective. Perspect Psychol Sci 2014; 9:94–108Crossref, Medline, Google Scholar
33 : Identification of risk loci with shared effects on five major psychiatric disorders: a genome-wide analysis. Lancet 2013; 381:1371–1379Crossref, Medline, Google Scholar
34 : The many faces of depression following spousal bereavement. J Affect Disord 1997; 45:85–95Crossref, Medline, Google Scholar
35 : A prospective cohort study investigating factors associated with depression during medical internship. Arch Gen Psychiatry 2010; 67:557–565Crossref, Medline, Google Scholar
36 : Genetics in population health science: strategies and opportunities. Am J Public Health 2013; 103(Suppl 1):S73–S83Crossref, Medline, Google Scholar
37 : Common disorders are quantitative traits. Nat Rev Genet 2009; 10:872–878Crossref, Medline, Google Scholar
38 : Is the gene-environment interaction paradigm relevant to genome-wide studies? The case of education and body mass index. Demography 2014; 51:119–139Crossref, Medline, Google Scholar
39 : Does the impact of major stressful life events on the risk of developing depression change throughout life? Psychol Med 2003; 33:1177–1184Crossref, Medline, Google Scholar
40 : Diagnostic and Statistical Manual of Mental Disorders, 5th ed. Washington, DC, American Psychiatric Publishing, 2013Crossref, Google Scholar
41 : Does bereavement-related major depression differ from major depression associated with other stressful life events? Am J Psychiatry 2008; 165:1449–1455Link, Google Scholar